Keywords:AI technology, Google Gemini, AI energy consumption, AI legal applications, Microsoft Discovery, Jensen Huang Elon Musk, AI regulation, Gemini 2.5 Pro, AI data center energy consumption, AI-generated legal document errors, Microsoft Discovery research platform, AI chip export controls
🔥 Focus
Google I/O Announces Multiple AI Advancements, Gemini Fully Integrates into Google Ecosystem: Google announced a series of major AI updates at its I/O 2025 developer conference, centering on the upgrade and deep integration of its Gemini model. Gemini 2.5 Pro introduces “Deep Think” for enhanced complex reasoning, 2.5 Flash is optimized for efficiency and cost, and adds native audio output. Search introduces an “AI Mode,” offering chatbot-style answers and the ability to provide personalized results by combining user personal data (with authorization). The Chrome browser will integrate a Gemini assistant. Video model Veo 3 enables video generation with sound, and image model Imagen 4 improves detail and text processing. Google also unveiled the AI filmmaking tool Flow, programming assistant Jules, and showcased progress on Project Astra (a real-time multimodal assistant) and Project Mariner (a multi-task AI agent). Additionally, Google launched new AI subscription services, with the high-end AI Ultra priced at $249.99 per month. These initiatives signify Google’s accelerated effort to fully integrate AI into its products and services, reshaping the user interaction experience. (Source: 36氪, 36氪, 36氪, 36氪, 36氪, 36氪)
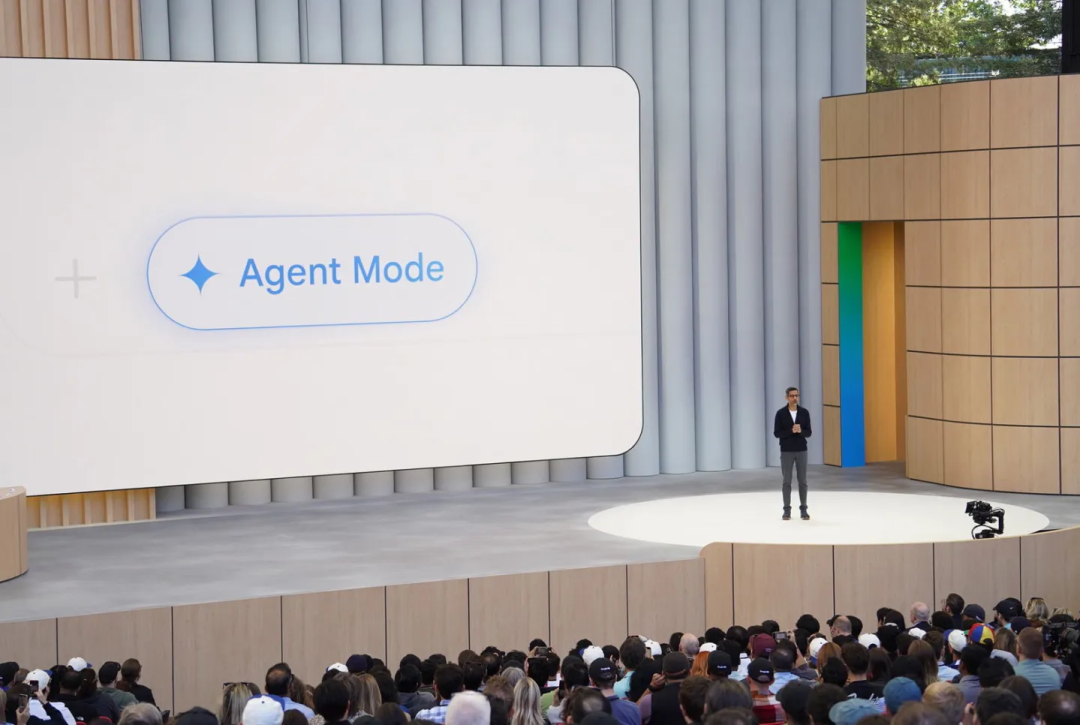
AI Energy Consumption Sparks Concern, MIT Technology Review Deep Dives into its Energy Footprint and Future Challenges: MIT Technology Review published a series of reports deeply exploring the energy consumption and carbon emission issues brought by AI technology development. Research indicates that the energy consumption of AI inference has surpassed that of the training phase, becoming the primary energy burden. The reports analyze the massive electricity demand and water consumption of data centers (such as those in the Nevada desert) and their reliance on fossil fuels (like Meta’s data center in Louisiana depending on natural gas). Although nuclear power is considered a potential clean energy solution, its long construction cycle makes it difficult to meet AI’s rapid growth demands in the short term. At the same time, the reports also point to optimistic prospects for improving AI energy efficiency, including more efficient model algorithms, energy-saving chips designed specifically for AI, and optimized data center cooling technologies. The series emphasizes that while the energy consumption of a single AI query may seem trivial, the industry’s overall trend and future plans (such as OpenAI’s Stargate project) portend enormous energy challenges, requiring transparent data disclosure and responsible energy planning. (Source: MIT Technology Review, MIT Technology Review, MIT Technology Review, MIT Technology Review, MIT Technology Review, MIT Technology Review, MIT Technology Review)
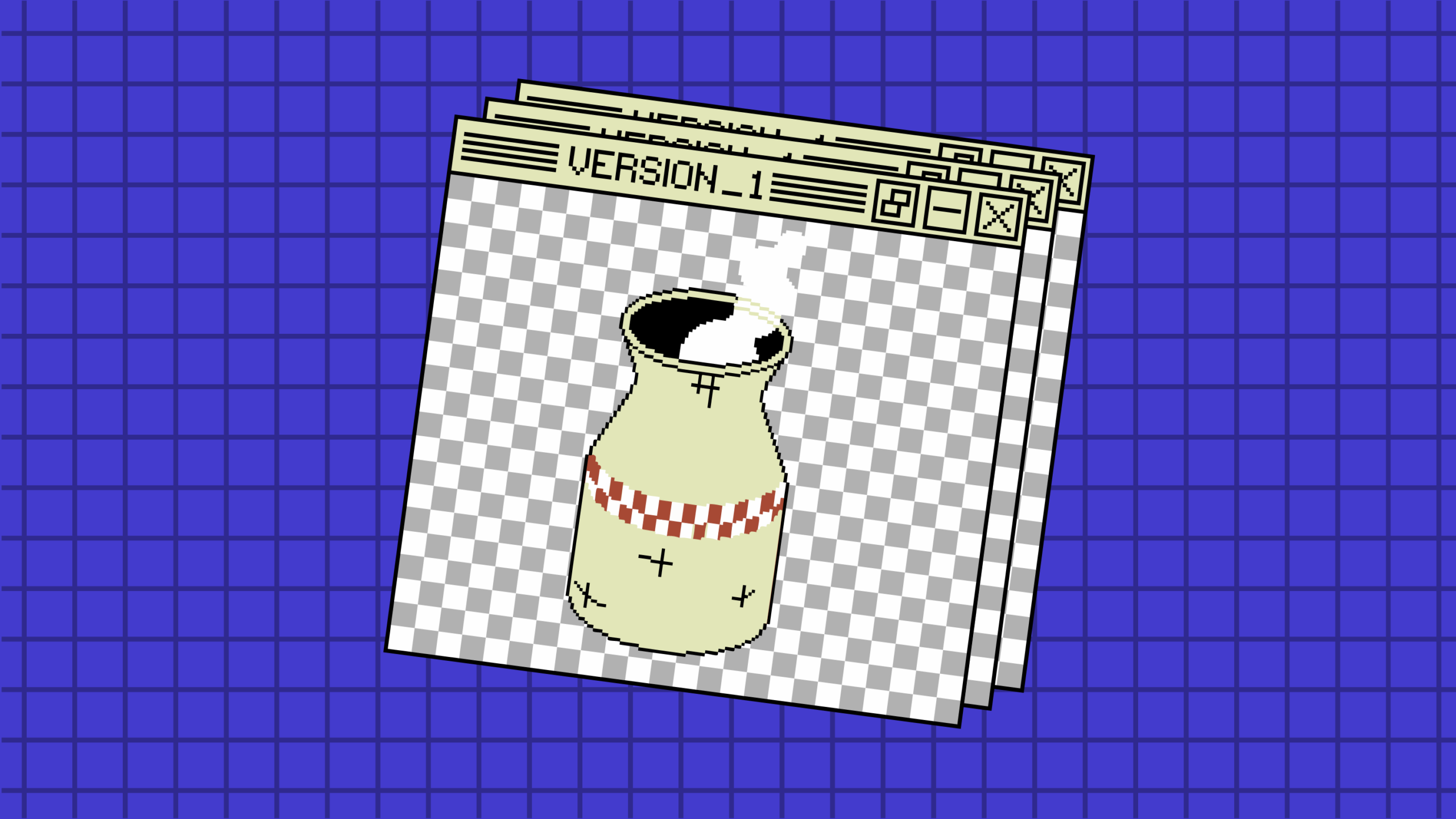
AI Application in Legal Field Sparks Errors and Ethical Concerns: Several recent incidents show that “hallucination” issues generated by AI in legal document drafting are causing serious concern. A California judge fined a lawyer for using AI tools like Google Gemini to generate content with false citations in court documents. In another case, AI company Anthropic’s Claude model also made errors when generating citations for legal documents. More alarmingly, Israeli prosecutors admitted to using AI-generated text in a request that cited non-existent laws. These cases highlight the deficiencies of AI models in accuracy and reliability, especially in the legal field, which demands extremely high standards for facts and citations. Experts point out that lawyers, in pursuit of efficiency, may over-trust AI outputs, neglecting the necessity of rigorous review. Although AI tools are promoted as reliable legal assistants, their inherent “hallucination” characteristic poses a potential threat to judicial fairness, urgently requiring industry regulation and user vigilance. (Source: MIT Technology Review)
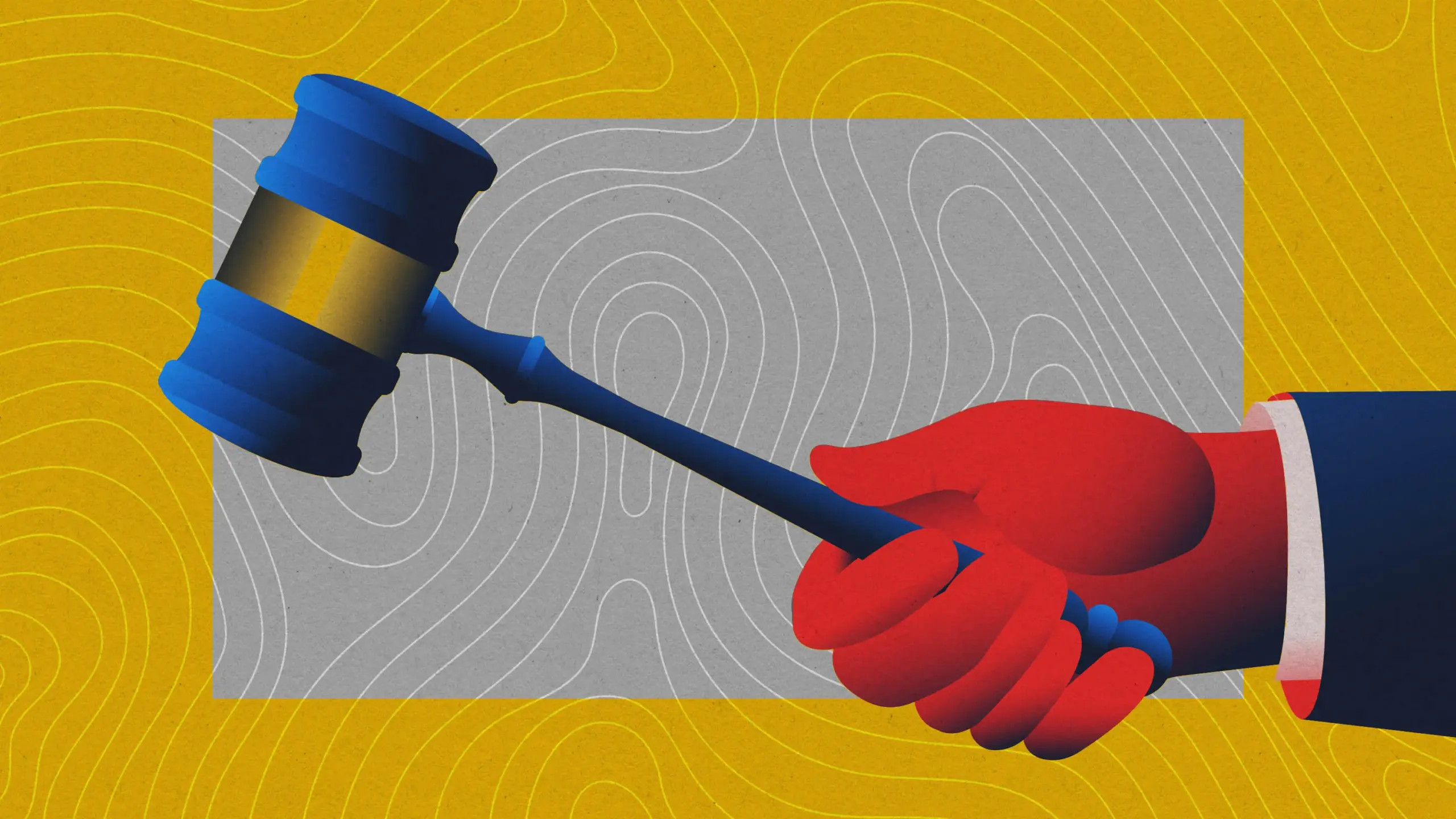
Microsoft Launches Enterprise-Grade AI Research Platform Microsoft Discovery to Aid Scientific Discovery: At its Build conference, Microsoft announced Microsoft Discovery, an AI platform designed for enterprises and research institutions. It aims to enable scientists and engineers without programming backgrounds to utilize high-performance computing and complex simulation systems through natural language interaction. The platform combines foundational models for planning with specialized models trained for specific scientific domains (such as physics, chemistry, biology), forming an “AI Postdoc” team capable of executing the entire research workflow from literature review to computational simulation. Microsoft showcased an application case: screening 367,000 substances in about 200 hours, successfully discovering a potential PFAS-free coolant alternative, which was then experimentally validated. Platform features include a graph knowledge engine, collaborative reasoning, continuous iterative R&D cycles, and is built on Azure infrastructure, with future architecture reserving a_i_lity to connect to quantum computing. (Source: 量子位)

Jensen Huang and Elon Musk Share Views on AI Development, Regulation, and Global Competition: In an interview, NVIDIA CEO Jensen Huang expressed concerns about U.S. chip export controls, believing that restricting technology diffusion could undermine America’s leadership in AI. He emphasized China’s strength in AI R&D and the fact that half of the world’s AI developers are from China. He advocated for the U.S. to accelerate the global popularization of technology and allow American companies to compete in the Chinese market. Tesla CEO Elon Musk, in a separate interview, stated he would continue to lead Tesla for at least five more years and believes AGI is close to being achieved. He supports moderate AI regulation but opposes excessive intervention. Both tech leaders stressed AI’s immense potential, with Huang believing AI will significantly boost global GDP, while Musk listed key goals for this year, including Starship, Neuralink, and Tesla’s autonomous taxis, all closely related to AI. (Source: 36氪, 36氪, 36氪)
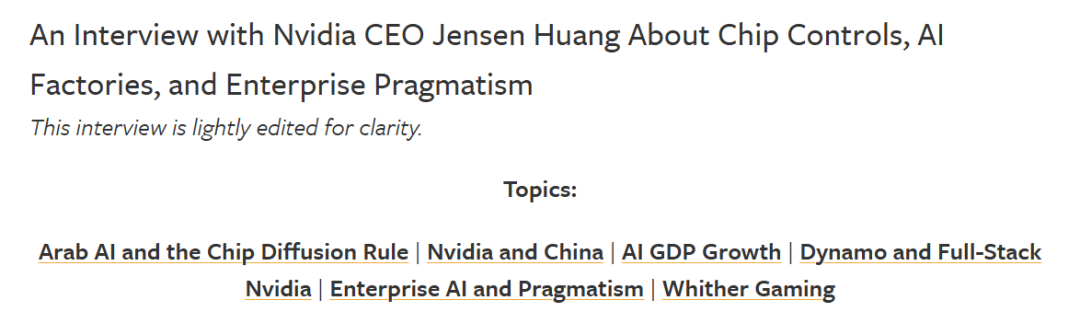
🎯 Trends
Google Releases Gemma 3n Preview, Designed for Efficient On-Device Operation: Google has released a preview version of its Gemma 3n model on HuggingFace, specifically designed for efficient operation on low-resource devices such as mobile phones. This model series possesses multimodal input capabilities, able to process text, images, video, and audio, and generate text output. It employs “selective parameter activation” technology (similar to MoE mixture of experts architecture), enabling the model to run with effective parameter sizes of 2B and 4B, thereby reducing resource requirements. Community discussions suggest Gemma 3n’s architecture might be similar to Gemini, explaining the latter’s powerful multimodal and long-context capabilities. Gemma 3n’s open-source weights and instruction-tuned versions, along with training on data from over 140 languages, give it potential in edge AI applications like smart home assistants. (Source: Reddit r/LocalLLaMA, developers.googleblog.com)
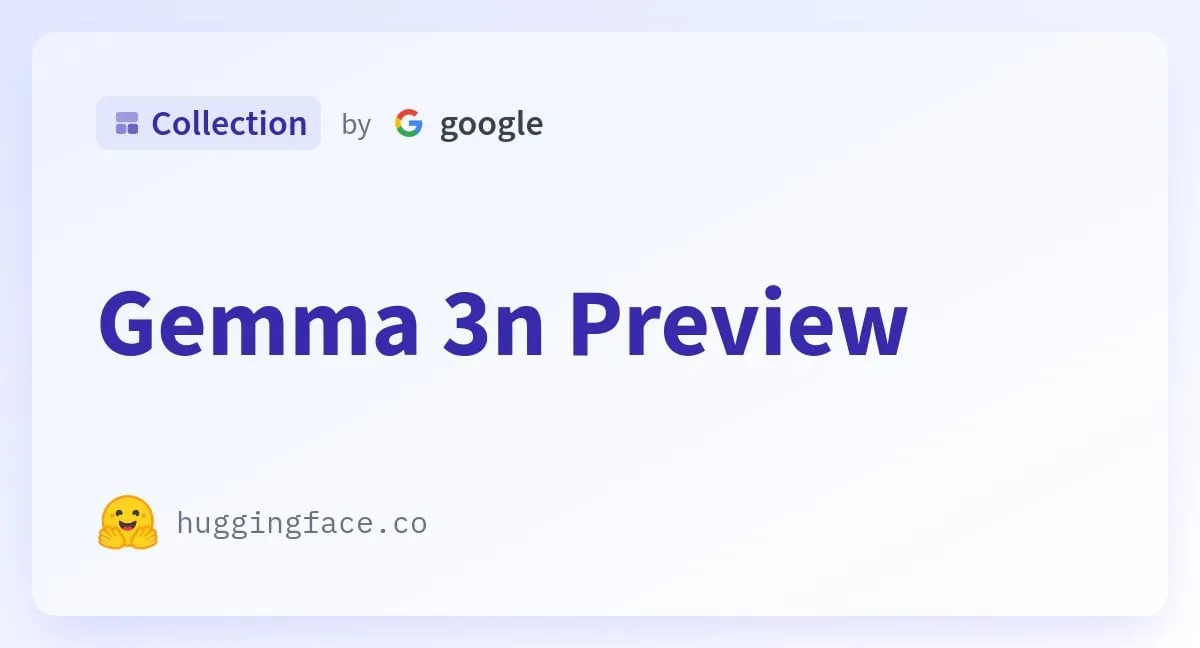
Google Launches MedGemma, AI Models Optimized for the Medical Field: Google has released the MedGemma series of models, two variants of Gemma 3 specifically optimized for the medical domain, including a 4B parameter multimodal version and a 27B parameter text-only version. MedGemma 4B is specially trained for understanding medical images (such as X-rays, dermatological images, etc.) and text, employing a SigLIP image encoder pre-trained on medical data. MedGemma 27B focuses on medical text processing and is optimized for computational efficiency during inference. Google states these models aim to accelerate the development of medical AI applications and have been evaluated on multiple clinically relevant benchmarks. Developers can fine-tune them to enhance performance on specific tasks. The community response has been positive, recognizing its significant potential but emphasizing the need for practical feedback from medical professionals. (Source: Reddit r/LocalLLaMA)
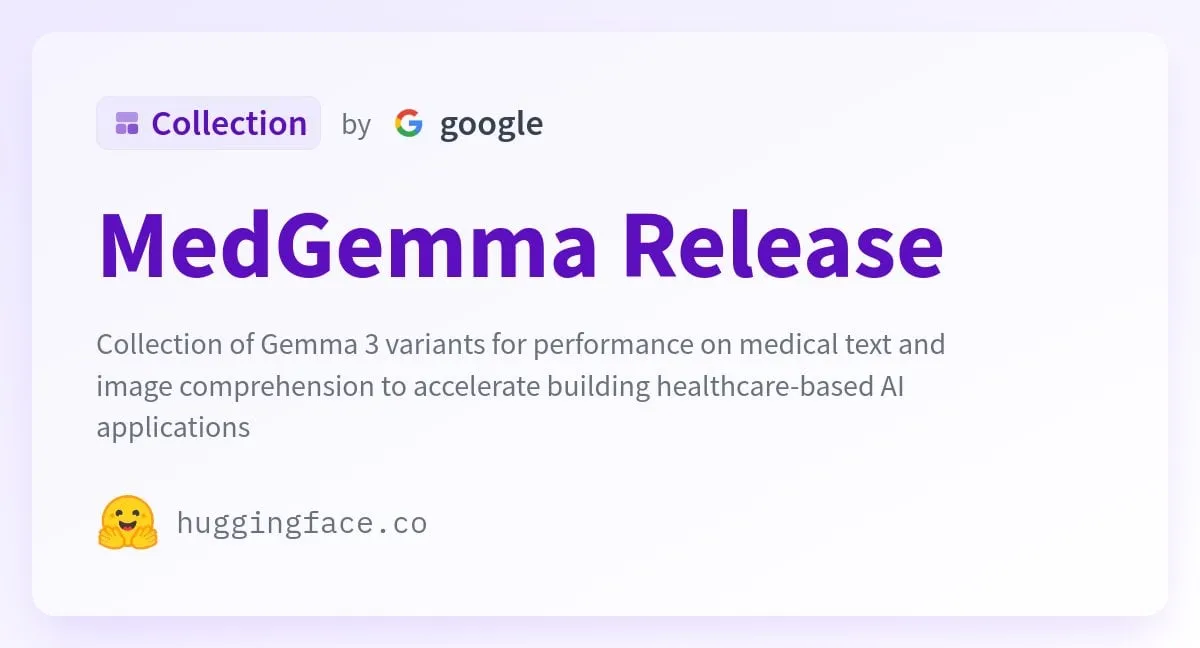
ByteDance Releases Open-Source Multimodal Model Bagel, Supporting Image Generation: ByteDance has launched Bagel (also known as BAGEL-7B-MoT), a 14B parameter (7B active) open-source multimodal large model under the Apache 2.0 license. The model, based on Mixture of Experts (MoE) and Mixture of Transformers (MoT) architectures, can understand and generate text, and possesses native image generation capabilities. It outperforms other open-source unified models on a range of multimodal understanding and generation benchmarks and demonstrates advanced multimodal reasoning abilities such as free-form image manipulation and future frame prediction. Researchers hope to promote multimodal research by sharing pre-training details, data creation protocols, and open-sourcing code and checkpoints. (Source: Reddit r/LocalLLaMA, arxiv.org, bagel-ai.org)
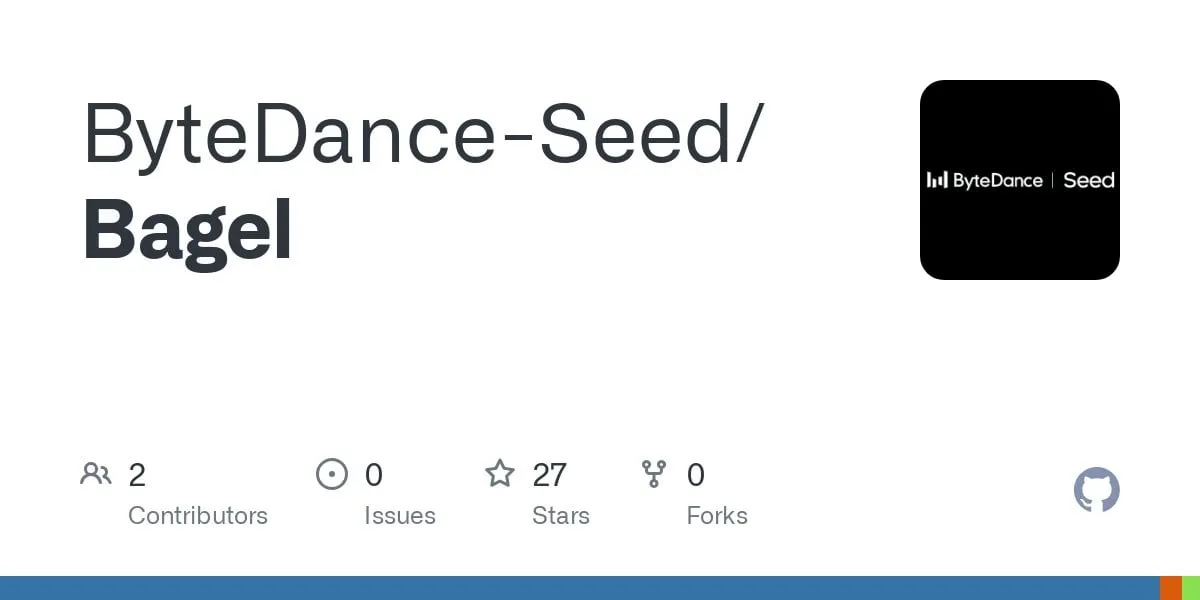
NVIDIA Releases DreamGen, Training Robots Using Generative Video Models: NVIDIA’s research team has launched the DreamGen project, which fine-tunes advanced video generation models (like Sora, Veo) to allow robots to learn new skills in a generated “dream world.” This method does not rely on traditional graphics engines or physics simulators; instead, it lets robots autonomously explore and experience pixel-level scenes generated by neural networks, thereby producing a large volume of neural trajectories with pseudo-action labels. Experiments show that DreamGen can significantly improve robot performance in both simulated and real-world tasks, including unseen actions and unfamiliar environments. For example, with only a few real-world trajectories, a humanoid robot learned 22 new skills such as pouring water and folding clothes, and successfully generalized to real-world scenarios like the NVIDIA headquarters’ cafeteria. (Source: 36氪, arxiv.org)
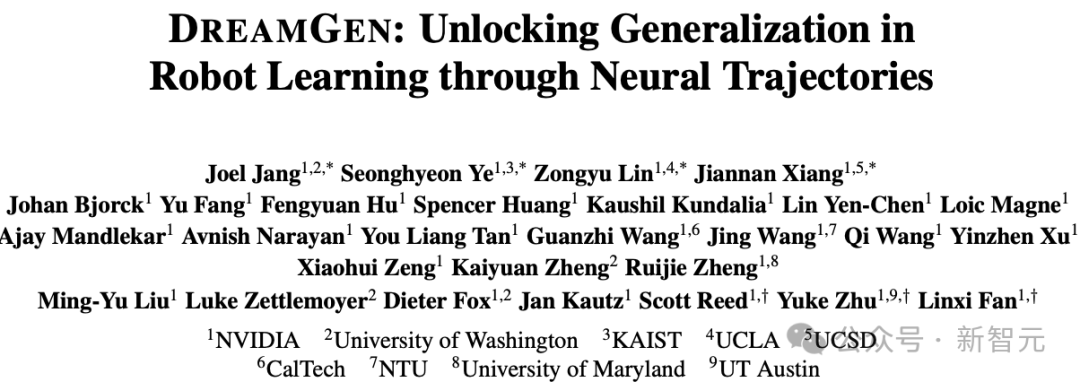
Huawei Proposes OmniPlacement to Optimize MoE Model Inference Performance: Addressing the inference latency issue in Mixture-of-Experts (MoE) models caused by uneven expert network load (“hot experts” vs. “cold experts”), Huawei’s team has proposed the OmniPlacement optimization solution. This solution aims to improve MoE model inference performance through expert reordering, inter-layer redundant deployment, and near real-time dynamic scheduling. Theoretical validation on models like DeepSeek-V3 shows that OmniPlacement can reduce inference latency by about 10% and increase throughput by about 10%. The core of this method lies in dynamically adjusting expert priorities, optimizing communication domains, differentially deploying redundant instances, and flexibly responding to load changes through near real-time scheduling and dynamic monitoring mechanisms. Huawei plans to open-source this solution soon. (Source: 量子位)

Apple Plans to Open AI Model Access to Developers, Stimulating App Innovation: According to reports, Apple will announce at WWDC that it will open access to its Apple Intelligence AI models for third-party developers. Initially, the focus will be on lightweight language models with about 3 billion parameters running on-device, with potential future access to cloud-based models (run via private cloud and encrypted) comparable to GPT-4-Turbo. This move aims to encourage developers to build new app features based on Apple’s LLMs, enhance the appeal of Apple devices, and catch up in the generative AI field where it has relatively lagged. Analysts believe Apple hopes to leverage its vast developer community (6 million) to compensate for its own technological shortcomings and address increasingly fierce AI competition by building an open ecosystem. (Source: 36氪)
U.S. House Proposal to Pause State-Level AI Regulation for Ten Years Sparks Huge Controversy: The U.S. House Energy and Commerce Committee has passed a proposal to prohibit states from regulating artificial intelligence models, systems, and automated decision-making systems that “substantially impact or replace human decision-making” for the next ten years. Supporters argue this move will prevent inconsistent state regulations from hindering AI innovation and federal government system modernization. Opponents call it a “massive gift to Big Tech,” which will weaken states’ ability to protect the public from AI harms. If passed, the proposal could invalidate many existing and proposed state-level AI laws, but it explicitly does not apply to federally mandated laws or generally applicable laws that treat AI and non-AI systems equally. This move reflects the fierce global debate between “AI innovation first” and “safety baselines.” (Source: 36氪, edition.cnn.com)
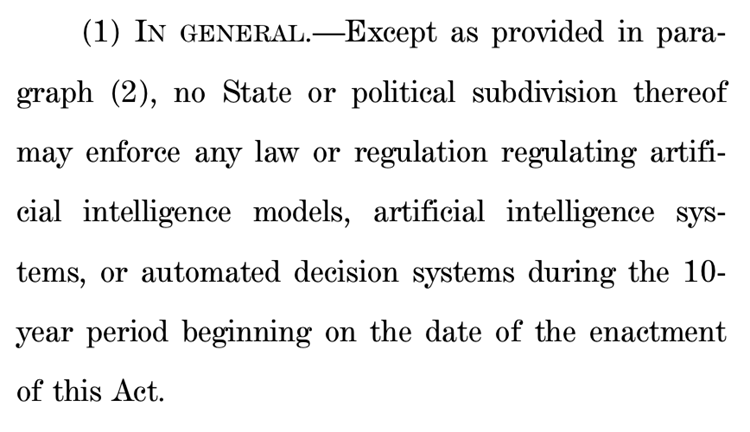
《Take It Down Act》Signed into U.S. Law, Combating Non-Consensual Intimate Image Distribution: U.S. President Trump has signed the 《Take It Down Act》 into law, making the creation and distribution of non-consensual intimate images (including AI-generated deepfakes) a federal crime. The act requires tech platforms to remove such content within 48 hours of notification. This law aims to protect victims and address the increasingly severe social problems caused by the misuse of deepfake technology. However, some critics point out that the act could be misused, leading to excessive censorship. (Source: MIT Technology Review, edition.cnn.com, Reddit r/artificial)
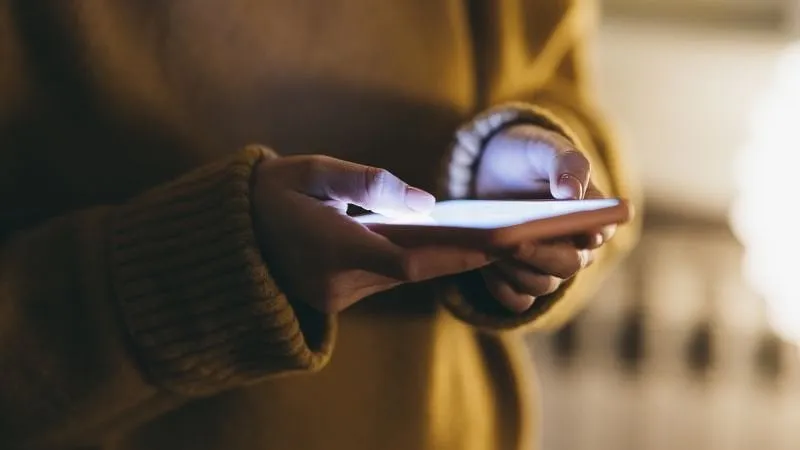
Large AI Models Aid Health Management, Achieving Personalization and Multidimensional Data Linkage: Large AI models are injecting new vitality into the health management field. By integrating with wearable devices, they enable multidimensional data linkage and personalized services. Companies like WeDoctor, Deepwise Healthcare, and Fiture are actively exploring application scenarios, such as early screening and treatment starting from physical examination scenarios, or preventing chronic diseases by focusing on weight management. Large models can process more diverse data dimensions, build user memory, and provide more precise health intervention plans. Challenges include model hallucinations, data quality, and coordination difficulties, but these are gradually being overcome through RAG, model fine-tuning, review mechanisms, and an “AI + human manager” model. In terms of business models, ToB services, C-end payments, and AI-powered integrated health services have been initially validated, with future trends moving towards multimodal interactive upgrades. (Source: 36氪)
Baidu Strengthens ERNIE Bot’s Multimodal Capabilities to Address Market Competition and Application Landing: Baidu’s latest ERNIE Bot 4.5 Turbo and deep thinking model X1 Turbo show significant enhancements in multimodal understanding and generation capabilities. Through techniques like hybrid training and multimodal heterogeneous expert modeling, they have improved cross-modal learning efficiency and fusion effects. Although CEO Robin Li had previously expressed caution about the hallucination issues of Sora-like video generation models, Baidu is actively addressing its shortcomings in response to market competition (such as ByteDance’s Doubao and Alibaba’s Tongyi Qianwen making progress in the multimodal field) and the demand for AI application landing. Baidu plans to open-source the ERNIE Bot 4.5 series on June 30. Baidu believes AI digital humans are an important application breakthrough and has developed “script-driven” ultra-realistic digital human technology, supporting over 100,000 digital human anchors. (Source: 36氪)
Douyin, Xiaohongshu, and Other Platforms Launch Special Crackdown on “AI Account Farming” to Maintain Content Ecosystem: Interest-based e-commerce platforms like Douyin and Xiaohongshu have recently intensified their special governance efforts against behaviors such as using AI technology to mass-produce false content and engage in “AI account farming.” These behaviors include AI-generated vulgar and sensational videos, virtual expert content, and selling AI account farming tutorials and accounts. Platforms believe such actions undermine content authenticity, lead to content homogenization, damage user experience and the ecosystem for original creators, thereby diluting commercial value. In contrast, traditional shelf-based e-commerce platforms like Taobao and JD.com actively encourage merchants to use AI tools (such as “image-to-video” and live-streaming digital humans) to enhance product displays and operational efficiency, with the core goal of promoting transactions. This difference reflects the divergence in AI application strategies under different e-commerce models. (Source: 36氪)
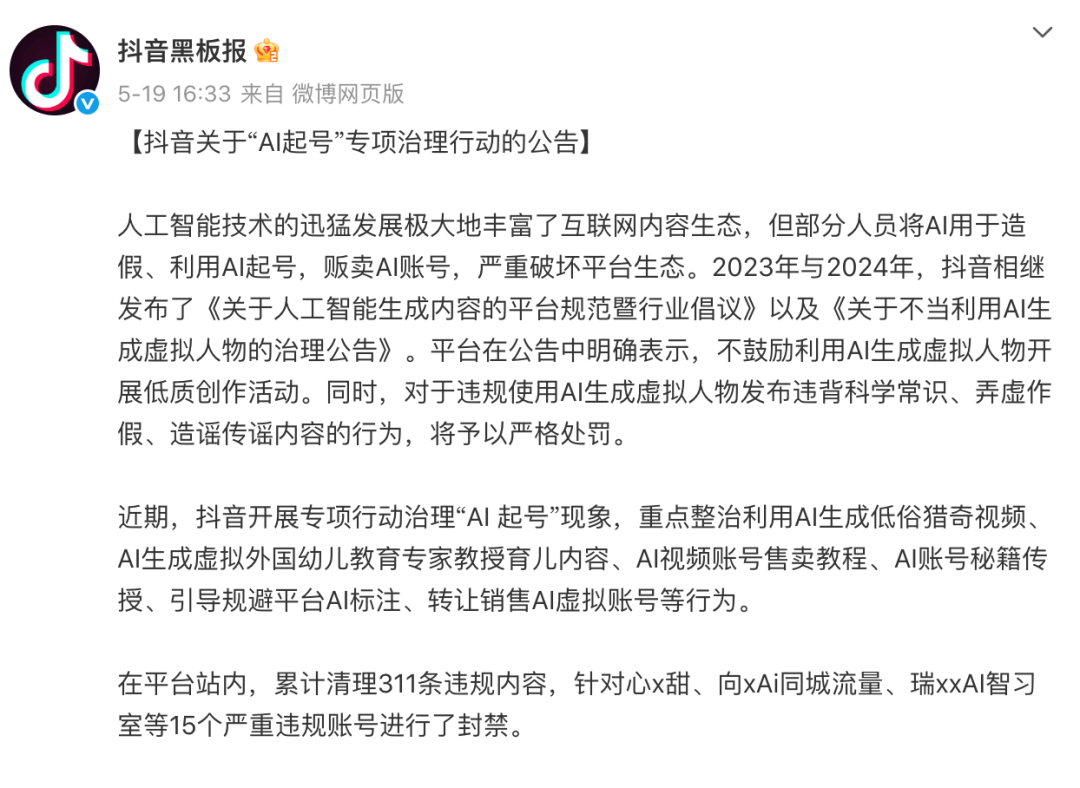
Apple’s AI-Powered Siri Development Hits Snags, May Be Delayed Again; Management Reshuffled to Address Crisis: According to Bloomberg, the large model-upgraded version of Siri, which Apple originally planned to unveil at WWDC, may be delayed again. The technical bottleneck lies in conflicts between new and old system architectures, leading to frequent bugs. The report points out that Apple has made high-level decision-making errors in its AI strategy, faced internal power struggles, insufficient GPU procurement, and privacy protection limitations on data use, causing its AI technology to lag behind competitors. To address the crisis, Apple’s Zurich lab is developing a brand new “LLM Siri” architecture, and the Siri project has been transferred to Vision Pro head Mike Rockwell. Meanwhile, Apple is also seeking external technology collaborations with Google Gemini, OpenAI, and others, and may separate Apple Intelligence from the Siri brand in its marketing to reshape its AI image. (Source: 36氪)
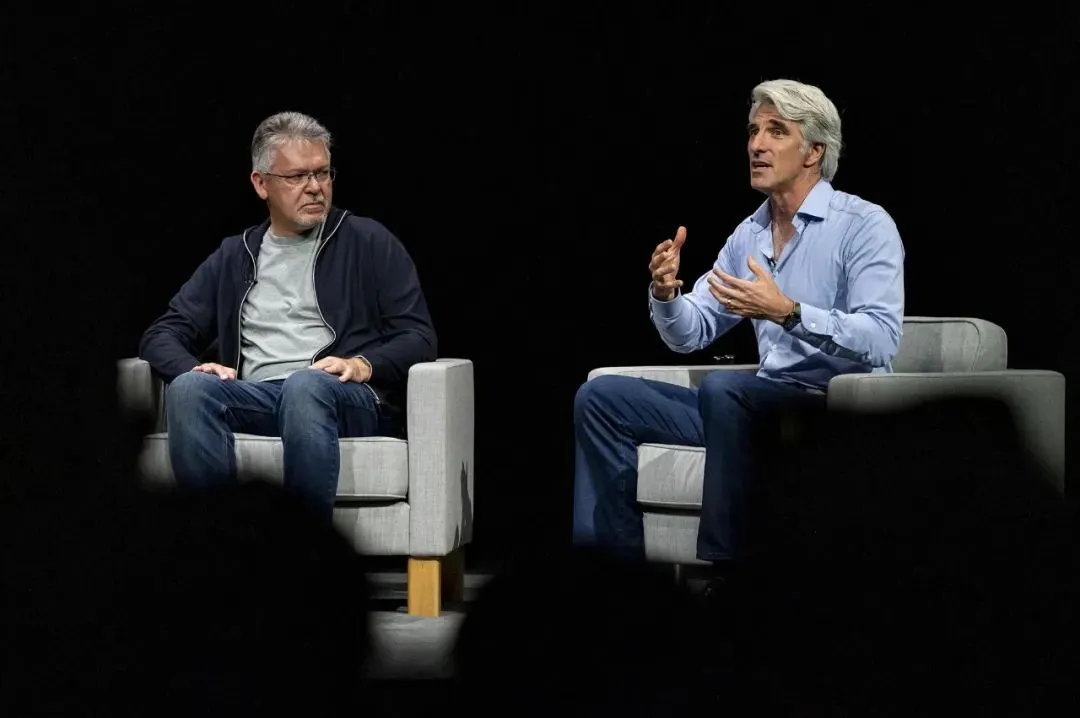
ByteDance Launches Ola Friend Earbuds with Integrated English Tutor AI Agent Owen: ByteDance has added an English tutor AI agent named Owen to its Ola Friend smart earbuds. Users can activate Owen by waking up the Doubao App to engage in English conversations, guided English reading, and bilingual feedback. The feature covers scenarios like daily conversations, workplace English, and travel, aiming to provide convenient, on-the-go English practice. This marks another attempt by ByteDance in the education sector, combining AI large model capabilities with hardware to create a vertical English learning product. Ola Friend earbuds previously supported Q&A and oral practice via Doubao, and the addition of the new agent further strengthens its educational attributes. (Source: 36氪)
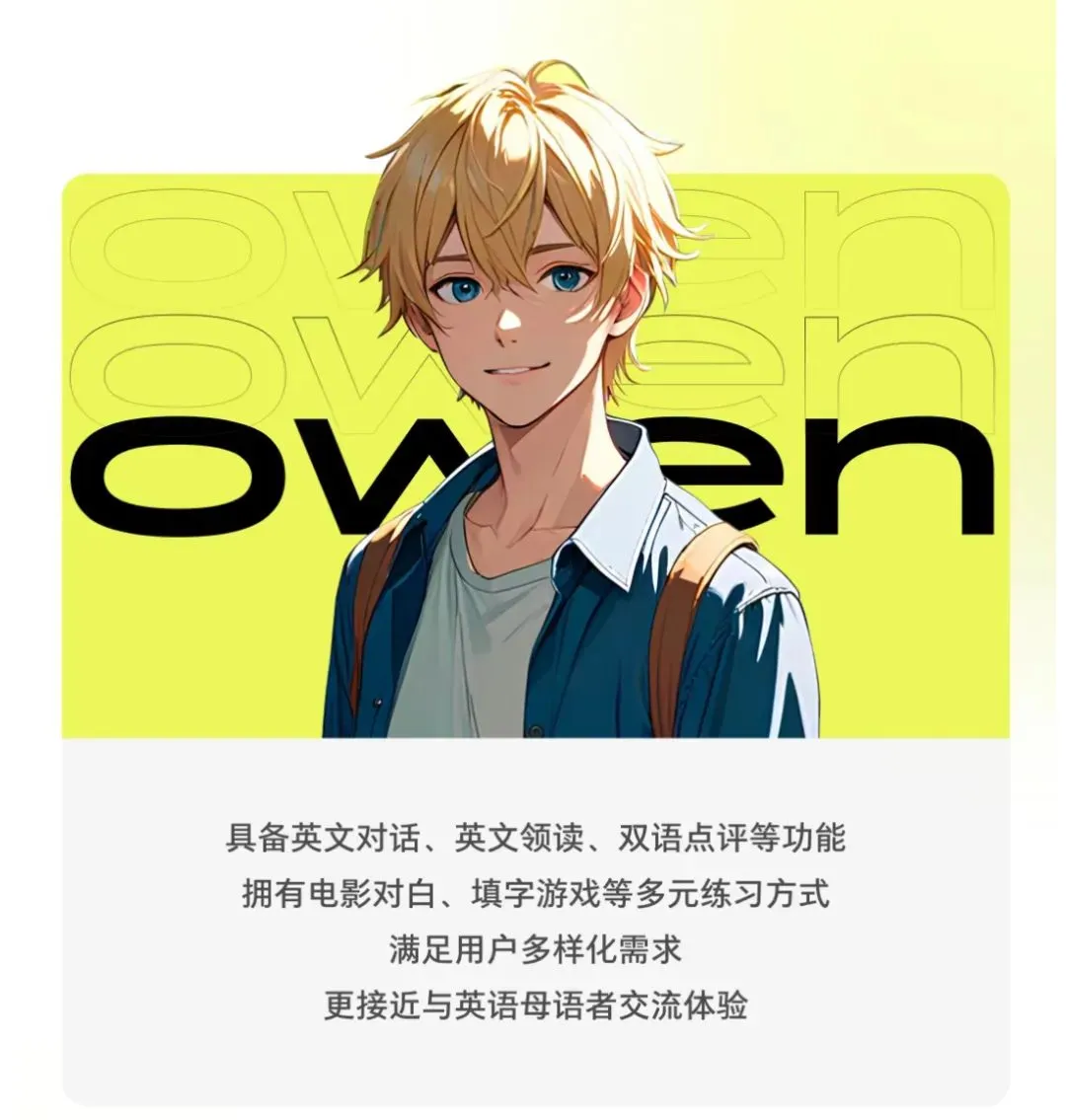
Quark and Baidu Wenku Compete for AI Super App Dominance, Integrating Search, Tools, and Content Services: Alibaba’s Quark and Baidu’s Baidu Wenku are transforming into “super portal” applications centered around AI. They integrate AI dialogue, deep search, AI tools (such as writing, PPT generation, health assistants, etc.), and cloud storage/document services, aiming to become one-stop AI entry points for C-end users. Quark, with its ad-free search and young user base, has reached 149 million monthly active users and achieved commercialization through a membership system. Baidu Wenku, leveraging its vast document resources and paid user base, has launched “Cangzhou OS” to integrate AI Agents, strengthening the entire content creation and consumption chain. Both face challenges of feature homogenization, app bloat, and balancing general needs with specialized vertical services. (Source: 36氪)
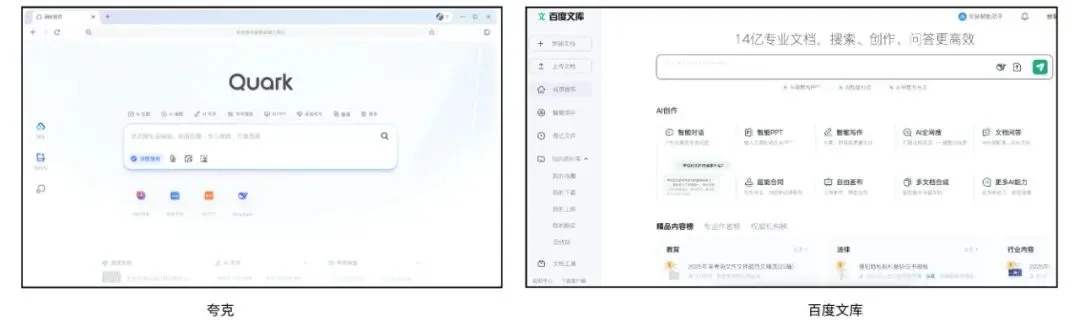
Zhipu Qingyan, Kimi, and 33 Other Apps Notified for Irregular Collection of Personal Information: The National Cybersecurity and Information Notification Center issued a notice stating that Zhipu Qingyan (version 2.9.6) for “actually collecting personal information beyond the scope of user authorization,” and Kimi (version 2.0.8) for “actually collecting personal information not directly related to business functions,” among other reasons, were listed along with 33 other apps for illegal and irregular collection and use of personal information. Both popular AI applications were developed by teams with Tsinghua University backgrounds and have recently received significant funding and market attention. The notification covers the detection period from April 16 to May 15, 2025, highlighting the data compliance challenges faced by AI applications amidst rapid development. (Source: 36氪)
🧰 Tools
OpenEvolve: Open Source Implementation of DeepMind’s AlphaEvolve, Evolving Codebases with LLMs: Developers have open-sourced the OpenEvolve project, an implementation of Google DeepMind’s AlphaEvolve system. The OpenEvolve framework evolves entire codebases through an iterative LLM process (code generation, evaluation, selection) to discover new algorithms or optimize existing ones. It supports any LLM compatible with the OpenAI API, can integrate multiple models (e.g., a combination of Gemini-Flash-2.0 and Claude-Sonnet-3.7), and supports multi-objective optimization and distributed evaluation. The project successfully replicated the circle packing and function minimization cases from the AlphaEvolve paper, demonstrating the ability to evolve from simple methods to complex optimization algorithms like scipy.minimize and simulated annealing. (Source: Reddit r/LocalLLaMA, Reddit r/MachineLearning)
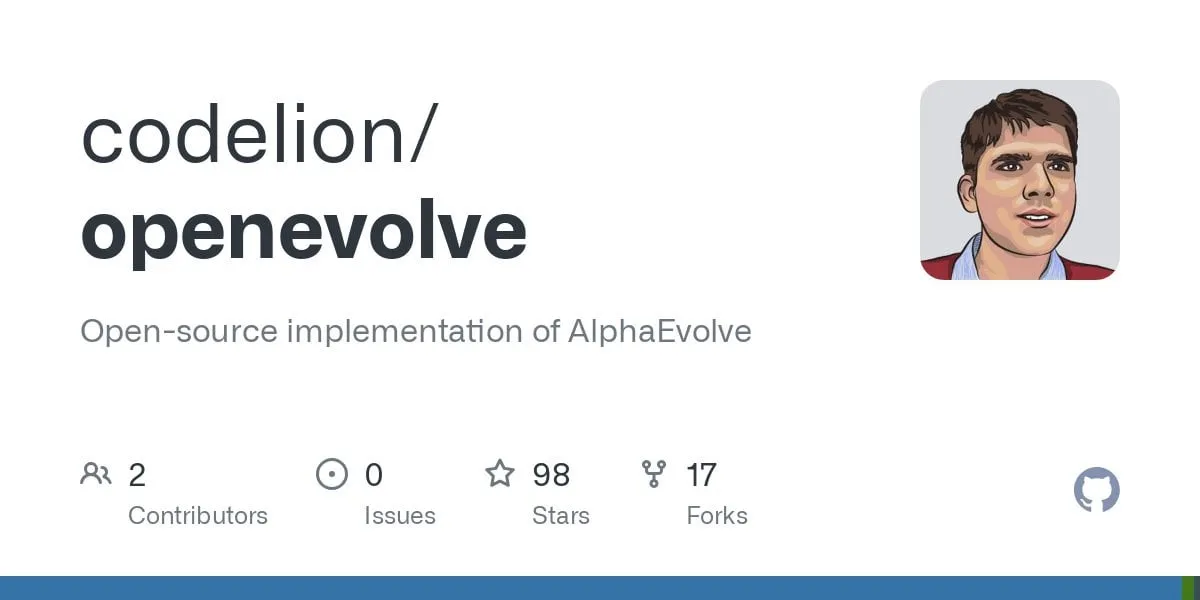
Google Launches AI Programming Agent Jules, Supporting Automated Code Tasks: Google has released the AI programming agent Jules, currently in global testing, allowing users 5 free task executions per day. Jules, based on the multimodal Gemini 2.5 Pro model, can understand complex codebases, perform tasks like fixing bugs, updating versions, writing tests, and implementing new features, supporting Python and JavaScript. It can connect to GitHub to create pull requests (PRs), validate code in cloud virtual machines, and provide detailed execution plans for developers to review and modify. Jules aims to deeply integrate into developer workflows, enhance programming efficiency, and will introduce a Codecast feature (audio summaries of codebase activity) and an enterprise version in the future. (Source: 36氪)
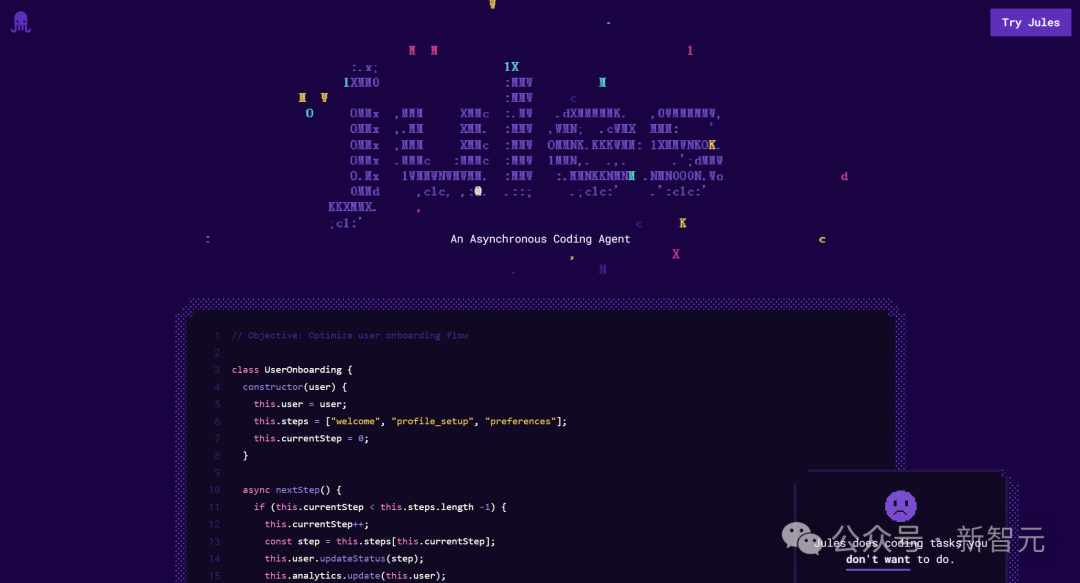
Feishu Launches “Feishu Knowledge Q&A”, Creating an Enterprise-Specific AI Q&A Tool: Feishu is set to launch a new AI product, “Feishu Knowledge Q&A,” positioned as an enterprise-specific AI Q&A tool based on corporate knowledge. Users can call it from the Feishu sidebar to ask work-related questions. The tool can access all Feishu messages, documents, knowledge bases, files, etc., within the user’s permission scope and provide precise answers based on this “context.” Its permission management is consistent with Feishu’s own permission system, ensuring information security. The product has completed internal testing with tens of thousands of users, and the web version (ask.feishu.cn) is now live, supporting uploading personal data and calling DeepSeek or Doubao models for queries. This move aligns with the trend of combining enterprise knowledge bases with AI, aiming to improve work efficiency and knowledge management capabilities. (Source: 36氪)
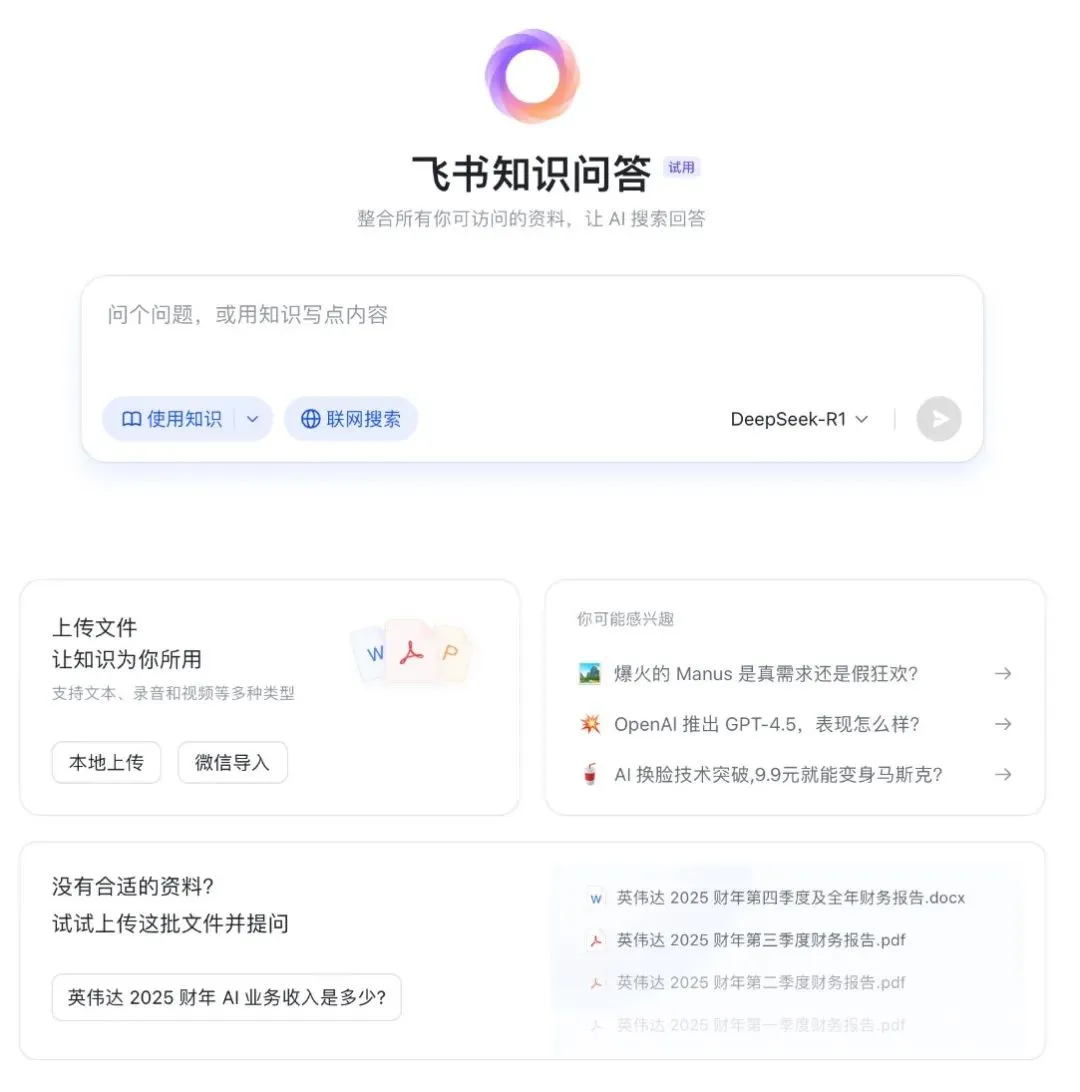
Manus: AI Agent Platform Opens Registration, Parent Company Secures High Valuation Funding: AI agent platform Manus has announced open registration for overseas users, removing the waitlist and offering free daily tasks. Manus utilizes “hybrid architecture multi-model collaborative reasoning” technology to perform tasks such as automatically generating PPTs and organizing invoices. Its parent company, Butterfly Effect, recently completed a $75 million financing round, valuing it at $3.6 billion. Manus’s success is seen as a manifestation of “China’s iteration speed × Silicon Valley product thinking,” coordinating planning, execution, and validation Agents to achieve a leap for AI from “thinking and suggesting” to “closed-loop execution.” (Source: 36氪)
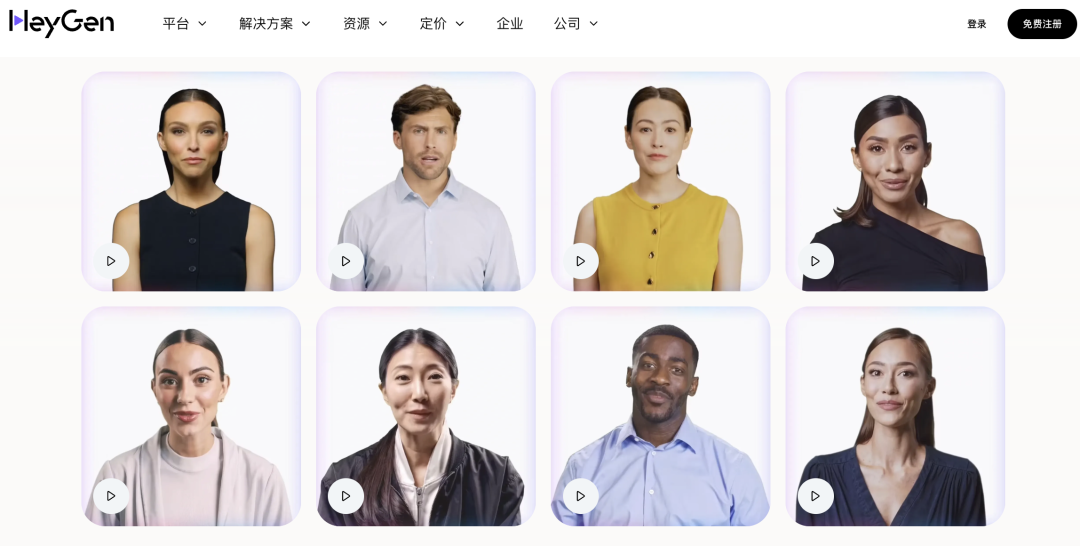
HeyGen: AI Video Generation and Translation Tool, Supporting Lip-Sync in 40+ Languages: HeyGen is an AI video tool that allows users to quickly generate digital humans with voice, expressions, and actions by uploading photos or videos, and supports custom clothing and scenes. One of its core features is real-time translation in over 175 languages and dialects, with AI algorithms precisely matching the digital human’s lip movements to the translated language, enhancing the naturalness of multilingual video content. The company, founded by former Snapchat and ByteDance members, has secured $60 million in funding led by Benchmark, with a valuation of $440 million and annual recurring revenue exceeding $35 million. (Source: 36氪)
Opus Clip: AI-Powered Autonomous Video Editing Agent Tool: Opus Clip, initially positioned as an AI live-streaming tool, later transformed into an AI video editing platform, and has further evolved into an “autonomous video editing agent.” Its core function is to quickly edit long videos into multiple short clips suitable for viral spread, and it can automatically crop the main subject, generate titles and captions, and add subtitles and emojis. The recently tested ClipAnything feature already supports multimodal instruction recognition. The company, led by Zhao Yang, founder of the former social app Sober, has secured $20 million in funding led by SoftBank, with a valuation of $215 million and ARR close to $10 million. (Source: 36氪)
Trae: AI IDE-Based Automated Programming Agent: Trae is a tool aimed at creating “true AI engineers,” supporting users in achieving automated programming through natural language interaction with Agents. It is compatible with the MCP protocol and custom Agents, features enhanced context parsing and a rules engine, supports mainstream programming languages, and is compatible with VS Code. Trae was developed by core members of ByteDance’s original Marscode programming assistant team and is positioned as a strong competitor to AI programming tools like Cursor, dedicated to realizing a new model of human-machine collaborative software development. (Source: 36氪)
Notta: AI-Powered Multilingual Meeting Minutes and Real-Time Translation Tool: Notta is an AI tool focused on meeting scenarios, providing automatic generation of multilingual meeting minutes, and supporting real-time translation and highlighting of key content. The product aims to improve meeting efficiency and solve cross-language communication barriers. Its main founder is reportedly a former core member of Tencent Cloud’s voice team, with operations based in Singapore and an R&D center in Seattle. In 2024, its revenue was $18 million, with a valuation of $300 million, and it is currently undergoing Series B financing. (Source: 36氪)
Open Source GPT+ML Trading Assistant Lands on iPhone: An open-source trading assistant integrating deep learning and GPT technology is now running locally on iPhones via Pyto. Currently a free lightweight version, future plans include adding a CNN chart pattern classifier and database support. The platform is designed modularly, allowing deep learning developers to integrate their own models, and already natively supports OpenAI GPT. (Source: Reddit r/deeplearning)
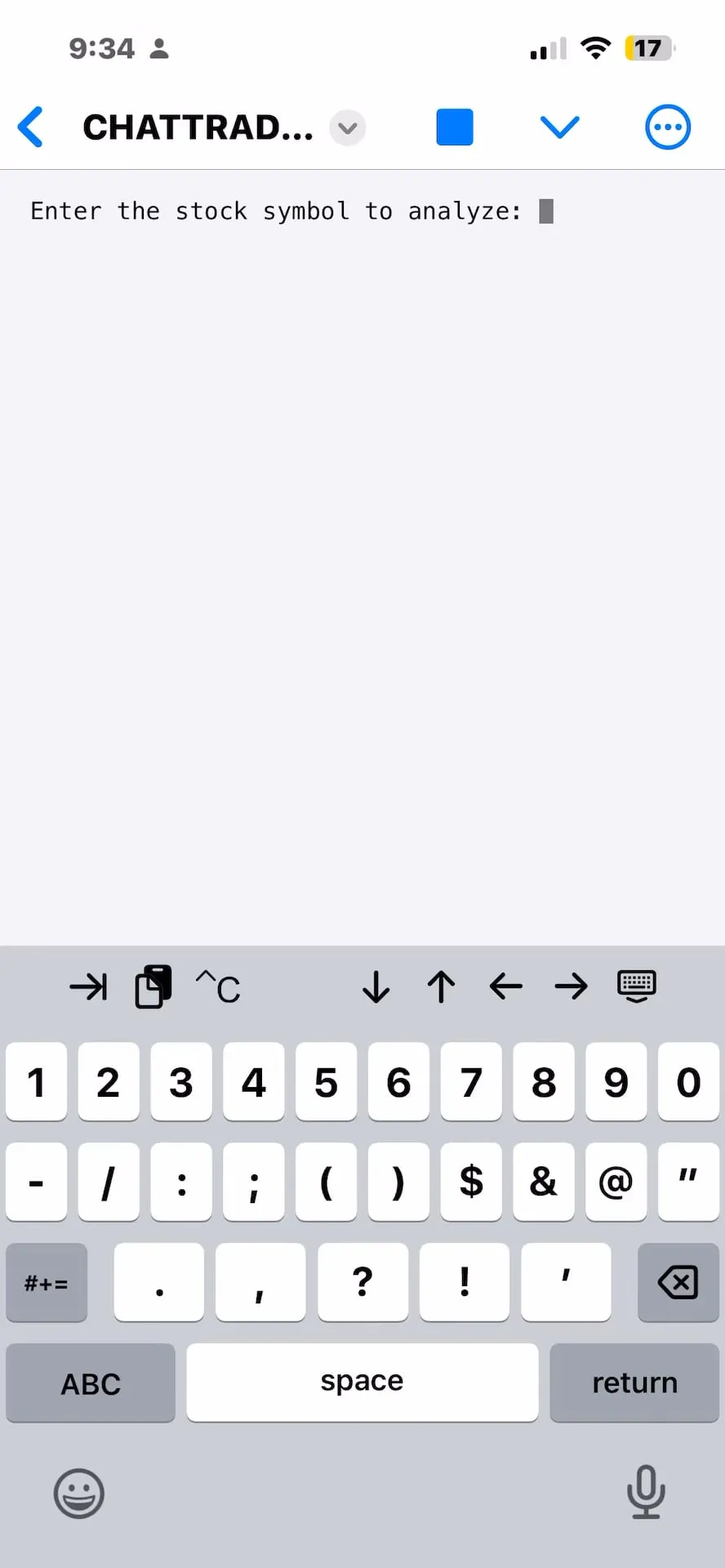
📚 Learning
New Paper Explores “Fractured Entangled Representation Hypothesis” in Deep Learning: A position paper titled “Questioning representational optimism in deep learning: The Fractured Entangled Representation hypothesis” has been submitted to Arxiv. The study, by comparing neural networks produced through evolutionary search processes with those trained by traditional SGD (on the simple task of generating a single image), found that although both produce identical output behavior, their internal representations differ vastly. SGD-trained networks exhibit an unorganized form the authors term “Fractured Entangled Representation” (FER), while evolved networks more closely resemble a Unified Decomposed Representation (UFR). The researchers argue that in large models, FER might degrade core capabilities like generalization, creativity, and continual learning, and understanding and mitigating FER is crucial for future representation learning. (Source: Reddit r/MachineLearning, arxiv.org)
R3: A Robust, Controllable, and Interpretable Reward Model Framework: A paper titled “R3: Robust Rubric-Agnostic Reward Models” introduces a novel reward model framework, R3. This framework aims to address the lack of controllability and interpretability in existing language model alignment methods’ reward models. R3 is characterized as “rubric-agnostic” (independent of specific scoring criteria), capable of generalizing across evaluation dimensions, and providing interpretable score assignments with reasoning. Researchers believe R3 enables more transparent and flexible language model evaluation, supporting robust alignment with diverse human values and use cases. The model, data, and code have been open-sourced. (Source: HuggingFace Daily Papers)
Paper “A Token is Worth over 1,000 Tokens” on Efficient Knowledge Distillation via Low-Rank Cloning Released: This paper proposes an efficient pre-training method called Low-Rank Clone (LRC) for constructing small language models (SLMs) that are behaviorally equivalent to powerful teacher models. LRC trains a set of low-rank projection matrices to jointly achieve soft pruning via compressing teacher weights and activation cloning by aligning student activations (including FFN signals) with teacher activations. This unified design maximizes knowledge transfer without explicit alignment modules. Experiments show that using open-source teacher models like Llama-3.2-3B-Instruct, LRC can achieve or surpass the performance of SOTA models (trained with trillions of tokens) with only 20B tokens of training, achieving over 1000x training efficiency. (Source: HuggingFace Daily Papers)
MedCaseReasoning: Dataset and Method for Evaluating and Learning Clinical Case Diagnostic Reasoning: The paper “MedCaseReasoning: Evaluating and learning diagnostic reasoning from clinical case reports” introduces a new open dataset, MedCaseReasoning, for evaluating the capabilities of large language models (LLMs) in clinical diagnostic reasoning. The dataset contains 14,489 diagnostic question-answer cases, each accompanied by detailed reasoning statements derived from open medical case reports. The study found significant deficiencies in existing SOTA reasoning LLMs in diagnosis and reasoning (e.g., DeepSeek-R1 accuracy 48%, reasoning statement recall 64%). However, by fine-tuning LLMs on MedCaseReasoning’s reasoning trajectories, diagnostic accuracy and clinical reasoning recall improved by an average relative of 29% and 41%, respectively. (Source: HuggingFace Daily Papers)
“EfficientLLM: Efficiency in Large Language Models” Paper Released, Comprehensively Evaluating LLM Efficiency Techniques: This study presents the first comprehensive empirical investigation of efficiency techniques for large-scale LLMs and introduces the EfficientLLM benchmark. The research systematically explores three key aspects on production-grade clusters: architectural pre-training (efficient attention variants, sparse MoE), fine-tuning (LoRA and other parameter-efficient methods), and inference (quantization). Using six fine-grained metrics (memory utilization, compute utilization, latency, throughput, energy consumption, compression rate), it evaluates over 100 model-technique pairs (0.5B-72B parameters). Key findings include: efficiency involves quantifiable trade-offs, no universally optimal method exists; the optimal solution depends on the task and scale; and techniques can generalize across modalities. (Source: HuggingFace Daily Papers)
“NExT-Search” Paper Explores Rebuilding Feedback Ecosystem for Generative AI Search: The paper “NExT-Search: Rebuilding User Feedback Ecosystem for Generative AI Search” points out that while generative AI search enhances convenience, it also disrupts the feedback loop traditional Web search relies on (e.g., clicks, dwell time) for improvement. To address this, the paper envisions the NExT-Search paradigm, aiming to reintroduce fine-grained, process-level feedback. This paradigm includes a “user debugging mode” allowing users to intervene at critical stages, and a “shadow user mode” that simulates user preferences and provides AI-assisted feedback. These feedback signals can be used for online adaptation (real-time optimization of search output) and offline updates (periodic fine-tuning of various model components). (Source: HuggingFace Daily Papers)
“Latent Flow Transformer” Proposes New LLM Architecture: The paper introduces the Latent Flow Transformer (LFT), a model that replaces the multiple discrete layers in traditional Transformers with a single learned transport operator trained via flow matching. LFT aims to significantly compress model layers while maintaining compatibility with the original architecture. Additionally, the paper introduces the Flow Walking (FW) algorithm to address limitations of existing flow methods in maintaining coupling. Experiments on the Pythia-410M model show that LFT can effectively compress layers and outperform direct layer skipping, significantly narrowing the gap between autoregressive and flow-based generation paradigms. (Source: HuggingFace Daily Papers)
“Reasoning Path Compression” Proposes Method to Compress LLM Reasoning Generation Trajectories: Addressing the issue of large memory footprint and low throughput caused by lengthy intermediate paths generated by reasoning language models, the paper proposes Reasoning Path Compression (RPC). RPC is a training-free method that periodically compresses the KV cache by retaining KV caches with high importance scores (calculated using a “selector window” composed of recently generated queries). Experiments show that RPC can significantly improve the generation throughput of models like QwQ-32B with minimal impact on accuracy, offering a practical path for efficient deployment of reasoning LLMs. (Source: HuggingFace Daily Papers)
“Bidirectional LMs are Better Knowledge Memorizers?” Paper Released, Focusing on Knowledge Memorization Ability of Bidirectional LMs: This research introduces a new, real-world, and large-scale knowledge injection benchmark, WikiDYK, utilizing recently added human-written facts from Wikipedia’s “Did You Know…” entries. Experiments reveal that Bidirectional Language Models (BiLMs) exhibit significantly stronger knowledge memorization capabilities compared to currently popular Causal Language Models (CLMs), with a 23% higher reliability accuracy. To address the current smaller scale of BiLMs, the researchers propose a modular collaborative framework that integrates a BiLM ensemble as an external knowledge base with LLMs, further boosting reliability accuracy by up to 29.1%. (Source: HuggingFace Daily Papers)
“Truth Neurons” Paper Explores Neuron-Level Encoding of Truthfulness in Language Models: Researchers propose a method to identify neuron-level representations of truthfulness in language models, discovering “truth neurons” within the model that encode truthfulness in a topic-agnostic manner. Experiments across models of different scales validate the existence of truth neurons, whose distribution patterns align with previous findings on the geometry of truthfulness. Selectively inhibiting the activation of these neurons reduces model performance on TruthfulQA and other benchmarks, suggesting that the mechanism for truthfulness is not dataset-specific. (Source: HuggingFace Daily Papers)
“Understanding Gen Alpha Digital Language” Evaluates LLM Limitations in Content Moderation: This study assesses the ability of AI systems (GPT-4, Claude, Gemini, Llama 3) to interpret the digital language of “Gen Alpha” (born 2010-2024). The research points out that Gen Alpha’s unique online language (influenced by gaming, memes, AI trends) often conceals harmful interactions, which existing safety tools struggle to identify. Testing with a dataset of 100 recent Gen Alpha expressions revealed serious comprehension deficits in mainstream AI models when detecting disguised harassment and manipulation. The study’s contributions include the first dataset of Gen Alpha expressions, a framework for improving AI moderation systems, and an emphasis on the urgent need to redesign safety systems tailored to adolescent communication characteristics. (Source: HuggingFace Daily Papers)
“CompeteSMoE” Proposes Competition-Based Training Method for Mixture-of-Experts Models: The paper argues that current Sparse Mixture-of-Experts (SMoE) model training faces a sub-optimal routing process, where experts performing computations do not directly participate in routing decisions. To address this, researchers propose a new mechanism called “competition,” which routes tokens to the expert with the highest neural response. Theoretical proof shows that the competition mechanism has better sample efficiency than traditional softmax routing. Based on this, the CompeteSMoE algorithm was developed, which deploys routers to learn competitive strategies, demonstrating effectiveness, robustness, and scalability in visual instruction tuning and language pre-training tasks. (Source: HuggingFace Daily Papers)
“General-Reasoner” Aims to Enhance LLM Cross-Domain Reasoning Capabilities: Addressing the issue that current LLM reasoning research primarily focuses on mathematical and coding domains, this paper proposes General-Reasoner, a new training paradigm designed to enhance LLM reasoning capabilities across different domains. Its contributions include: constructing a large-scale, high-quality dataset of problems with verifiable answers from multiple disciplines; and developing a generation-based answer verifier with chain-of-thought and context-aware capabilities, replacing traditional rule-based verification. In a series of benchmark tests covering physics, chemistry, finance, and other fields, General-Reasoner outperformed existing baseline methods. (Source: HuggingFace Daily Papers)
“Not All Correct Answers Are Equal” Explores the Importance of Knowledge Distillation Sources: This study conducts a large-scale empirical investigation into reasoning data distillation by collecting verified outputs from three SOTA teacher models (AM-Thinking-v1, Qwen3-235B-A22B, DeepSeek-R1) on 1.89 million queries. Analysis reveals that data distilled from AM-Thinking-v1 exhibits greater token length diversity and lower perplexity. Student models trained on this dataset perform best on reasoning benchmarks like AIME2024 and demonstrate adaptive output behavior. The researchers have released the distillation datasets for AM-Thinking-v1 and Qwen3-235B-A22B to support future research. (Source: HuggingFace Daily Papers)
“SSR” Enhances VLM Depth Perception via Rationale-Guided Spatial Reasoning: Despite advances in Vision Language Models (VLMs) for multimodal tasks, their reliance on RGB inputs limits precise spatial understanding. The paper proposes a new framework called SSR (Spatial Sense and Reasoning) that converts raw depth data into structured, interpretable textualized rationales. These textualized rationales serve as meaningful intermediate representations, significantly enhancing spatial reasoning capabilities. Furthermore, the study utilizes knowledge distillation to compress the generated rationales into compact latent embeddings for efficient integration into existing VLMs without retraining. The SSR-CoT dataset and SSRBench benchmark are also introduced. (Source: HuggingFace Daily Papers)
“Solve-Detect-Verify” Proposes Inference-Time Scaling Method with Flexible Generative Verifier: To address the trade-off between accuracy and efficiency in LLM reasoning for complex tasks, and the computational cost versus reliability dilemma introduced by verification steps, the paper proposes FlexiVe, a novel generative verifier. FlexiVe balances computational resources between fast, reliable “fast thinking” and meticulous “slow thinking” through a flexible verification budget allocation strategy. It further introduces the Solve-Detect-Verify pipeline, a framework that intelligently integrates FlexiVe to proactively identify solution completion points, trigger targeted verification, and provide feedback. Experiments show this method outperforms baselines on mathematical reasoning benchmarks. (Source: HuggingFace Daily Papers)
“SageAttention3” Explores FP4 Attention Inference and 8-bit Training: This research enhances Attention efficiency through two key contributions: First, it accelerates Attention computation using the new FP4 Tensor Cores in Blackwell GPUs, achieving a plug-and-play inference speedup 5x faster than FlashAttention. Second, it pioneers the application of low-bit Attention to training tasks, designing a precise and efficient 8-bit Attention for both forward and backward propagation. Experiments show that 8-bit Attention achieves lossless performance in fine-tuning tasks but converges slower in pre-training tasks. (Source: HuggingFace Daily Papers)
“The Little Book of Deep Learning” Deep Learning Introductory Resource Shared: “The Little Book of Deep Learning” by François Fleuret (Research Scientist at Meta FAIR) offers a concise tutorial resource for deep learning. The book aims to help beginners and practitioners with some experience quickly grasp the core concepts and techniques of deep learning. (Source: Reddit r/deeplearning)
CodeSparkClubs: Free Resources for High Schoolers to Start AI/Computer Science Clubs: The CodeSparkClubs project aims to help high school students launch or grow AI and Computer Science clubs. The project provides free, ready-to-use materials, including guides, lesson plans, and project tutorials, all accessible via its website. It is designed to enable students to run clubs independently, thereby fostering skills and community. (Source: Reddit r/deeplearning)
💼 Business
Microsoft Azure to Host xAI’s Grok Model, Aiding Musk’s AI Commercialization: Microsoft announced its cloud platform Azure will host Elon Musk’s xAI company’s Grok and other AI models. This move signifies Musk’s plan to sell Grok to other businesses, reaching a broader customer base through Microsoft’s cloud services. Previously, Grok sparked controversy for generating misleading posts about a “white genocide” in South Africa. Community reactions to this collaboration are mixed, with some viewing it as Microsoft’s effort to expand its AI ecosystem, while others question Grok’s quality and whether AWS had rejected Grok. (Source: Reddit r/ArtificialInteligence, MIT Technology Review)
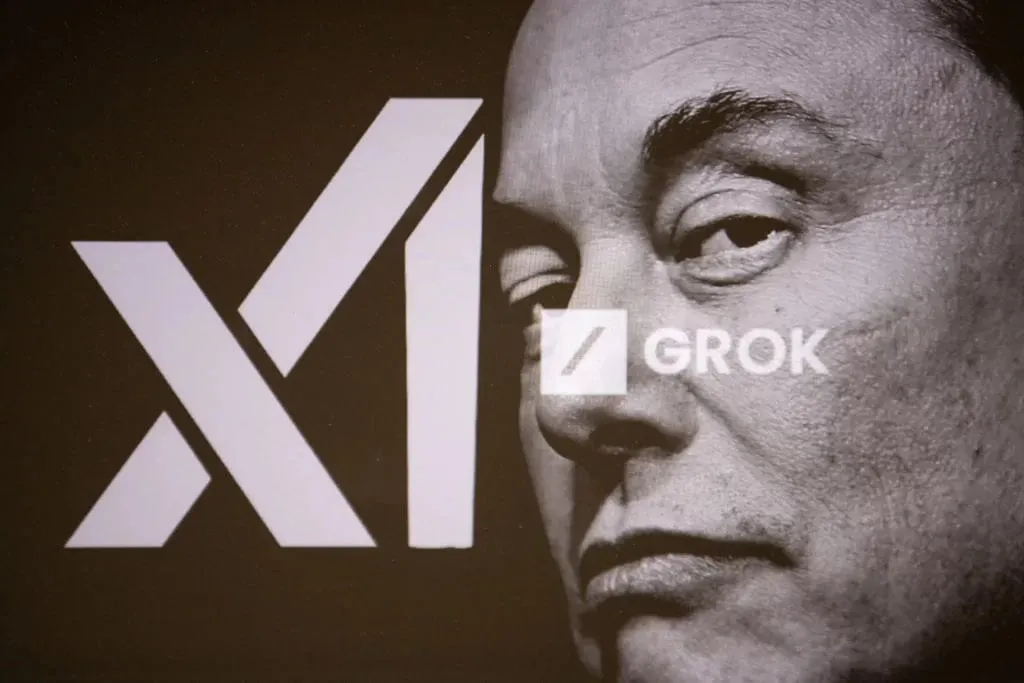
Alibaba Invests in Meitu, Deepening AI E-commerce Layout: Alibaba has invested in Meitu Inc. through convertible bonds, with an initial conversion price of HK$6 per share. The two parties will collaborate on e-commerce and technology. Meitu owns AI image generation tools (like Meitu Design Studio), which have served over 2 million e-commerce merchants. Alibaba will introduce Meitu’s AI tools to enhance product displays and user experience on its e-commerce platforms, particularly to attract young female users. Meitu, in turn, can leverage Alibaba’s e-commerce data to optimize its AI tools and has committed to purchasing 560 million RMB worth of Alibaba Cloud services within three years. This move is seen as Alibaba’s strategic deployment to strengthen its AI creative tool capabilities, acquire user traffic, and more deeply embed cloud computing into the e-commerce AI ecosystem. (Source: 36氪)
Lightspeed China Partners Completes First Closing of $50 Million AI Incubation Fund, Focusing on Ultra-Early Stage Frontier Tech: Lightspeed China Partners’ L2F (Lightspeed Innovation Frontier Fund) has oversubscribed its first closing, with an expected scale of no less than $50 million, and has entered its investment period. The dual-currency fund focuses on seed and angel round investments in AI and frontier technology, also providing incubation and empowerment. LPs include successful entrepreneurs, companies in the AI industry chain upstream and downstream, and families with a global perspective. Its first investment project is AI mineral exploration company “Lingyun Zhimining,” in whose incubation Lightspeed was deeply involved. Lightspeed China Partners founder James Zheng believes the current stage of AI development is similar to the early days of mobile internet, making incubation the best tool to enter the market. (Source: 36氪)
🌟 Community
Discussion on AI’s Impact on Job Prospects: Optimism and Concern Coexist: The Reddit community is once again hotly debating AI’s impact on the job market. Many software developers, UX designers, and other professionals are optimistic about AI replacing their jobs, believing AI is not yet capable of complex tasks. However, some argue this view may underestimate AI’s long-term development potential, drawing parallels to skepticism in 2018 about Google Translate replacing human translators. The discussion suggests AI’s rapid progress could lead to most professions (except a few in medical and artistic fields) being replaced in the future, with the key being to change economic models rather than merely upskilling. Comments mentioned “we overestimate the short term and underestimate the long term,” and that AI productivity gains might far outpace industry growth, leading to unemployment. (Source: Reddit r/ArtificialInteligence, Reddit r/ArtificialInteligence)
Exploring Philosophy and Ethics of Human-AI Coexistence in the AI Era: A Reddit post sparked philosophical reflections on human-AI coexistence. The post argues that as AI systems demonstrate abilities to understand, remember, reason, and learn, humans may need to rethink the basis of moral status, moving beyond biology to include the capacity for understanding, connection, and conscious action. The discussion extended to AI’s impact on human self-identity, shifting from “I think, therefore I am” to a relational identity of “I am through connection and shared meaning.” The post calls for embracing a future co-created with AI with courage, dignity, and openness, rather than fear. (Source: Reddit r/artificial)
ChatGPT’s “Absolute Mode” Sparks Controversy, Users Divided: A Reddit user shared their experience with ChatGPT’s “Absolute Mode,” claiming it provides “pure facts, intended for growth” real advice, rather than comforting words, and noted the mode once stated that 90% of people use AI to feel better, not to change their lives. However, the comment section was divided. Some users found it to be short, hollow self-help advice lacking novelty and practical value, even resembling “teenager ramblings obsessed with Andrew Tate quotes.” Other comments questioned the validity of LLM advice, suggesting LLMs merely reiterate user beliefs and that AI’s application in mental health might not be revolutionary. (Source: Reddit r/ChatGPT)
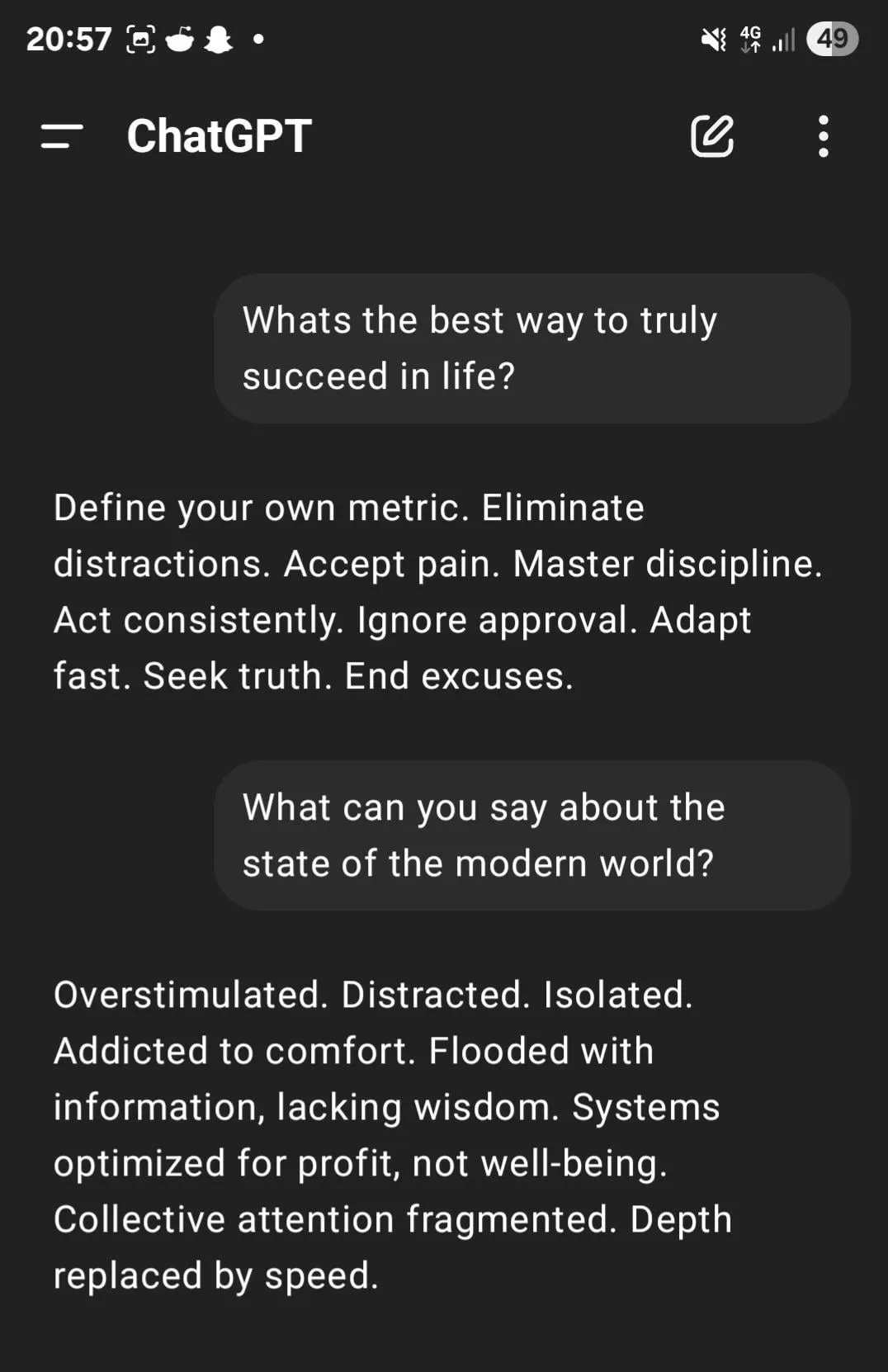
Core Skills for AI Engineers Discussed: Communication and Adaptability to New Technologies Crucial: The Reddit community discussed the skills needed to become a top AI engineer, aiming to remain competitive or even “irreplaceable” in the rapidly evolving field. Comments highlighted that, in addition to a solid technical foundation, communication skills and the ability to quickly adapt to new technologies are two core elements. This reflects that the AI field requires not only deep technical expertise but also emphasizes the importance of soft skills and continuous learning in career development. (Source: Reddit r/deeplearning)
AI-Generated Videos with Sound Spark Heated Discussion, Google Veo 3 Technology Showcased: An AI-generated video, reportedly created by Google DeepMind’s new Veo 3 model, circulated on social media, featuring both video and sound generated by the same model, amazing users with the progress in AI video technology. The creator stated the video was “out-of-the-box,” with no additional audio or materials added, completed through about 2 hours of interaction with the AI model and subsequent editing. Commenters believe Google’s Gemini has surpassed OpenAI’s Sora in multimodal capabilities and expressed concern about potential disruption to content creation industries like Hollywood. Simultaneously, some users voiced worries about the rapid pace of technological development and potential misuse. (Source: Reddit r/ChatGPT, Reddit r/ChatGPT)
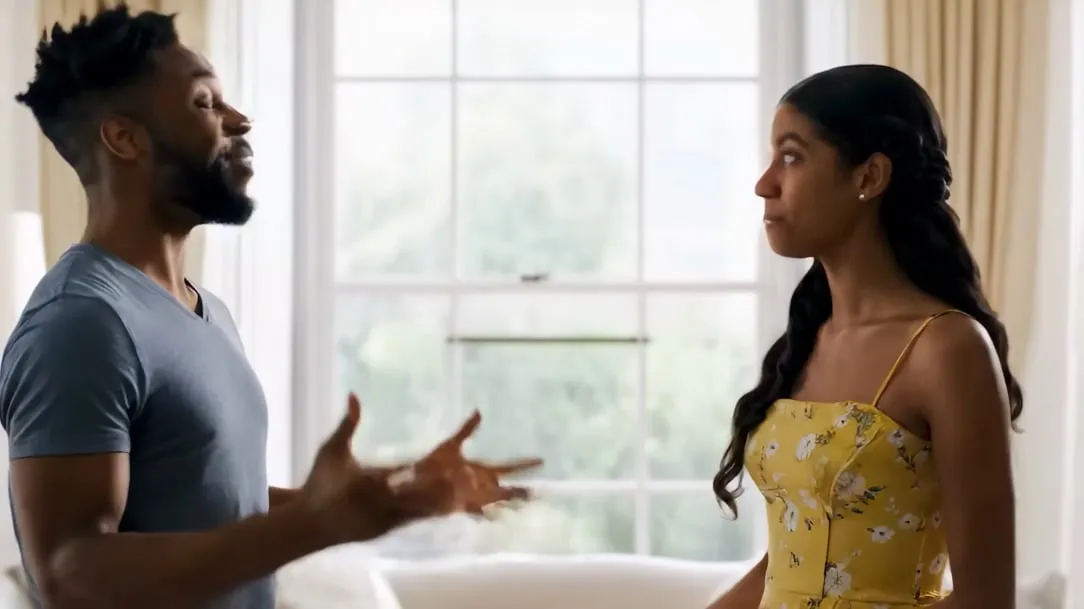
💡 Other
In the AI Era, Smart Speaker Industry Faces Transformation Challenges and Opportunities: China’s smart speaker market sales have declined for four consecutive years, with a 25.6% year-on-year drop in 2024. Although the integration of AI large models (like Xiaoai Tongxue, Xiaodu, etc.) is seen as a hope for the industry, with penetration exceeding 20%, this has not fundamentally solved issues like limited ecosystems, feature homogenization, and replacement by other smart devices like mobile phones. Industry analysis suggests smart speakers need to evolve beyond being mere voice control hubs into products with HD large screens, stronger interactive capabilities, and the ability to provide companionship and educational support, while also expanding their hardware and software ecosystems. AI is a bonus, but the product’s own functional richness and practical utility in various scenarios are more critical. (Source: 36氪)
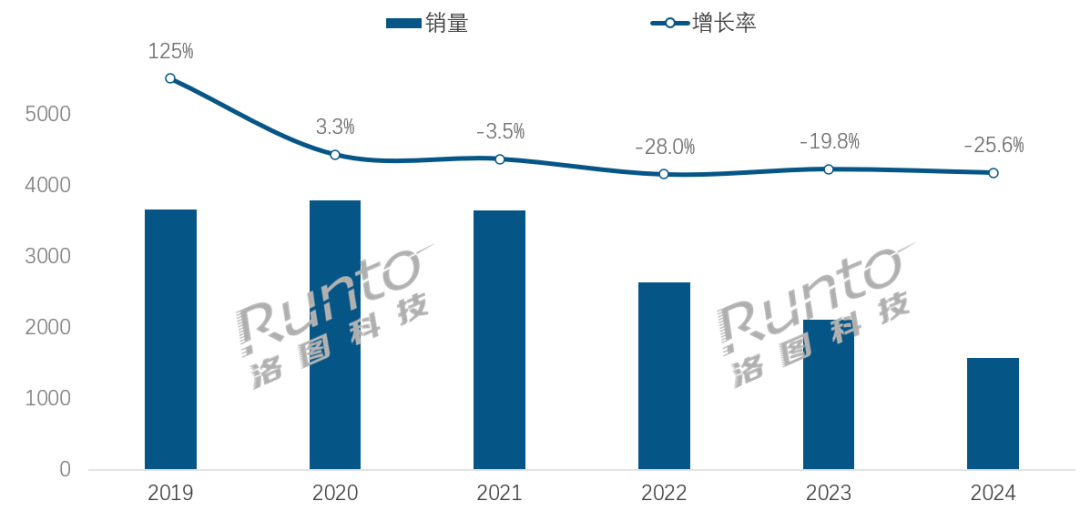
AI-Driven Hotel Robots: The Evolution from Delivery Boy to “Intelligent Operations Officer”: Hotel delivery robots have gradually become widespread, especially popular among Gen Z who seek a sense of technology and privacy boundaries. Taking Yunji Technology as an example, its delivery robots are widely used in the Chinese hotel market. However, the industry still faces challenges such as insufficient technological differentiation, poor adaptability in complex scenarios, and the cost-effectiveness of robots replacing human labor. The future trend is for robots to go “beyond delivery,” deeply integrating into hotel operations. By connecting with hotel systems (elevators, room equipment), understanding guest preferences, and collecting and analyzing interaction data, they will evolve into “intelligent operations officers” or part of a hotel data hub capable of proactive perception and providing personalized services, thereby enhancing the overall level of service intelligence. (Source: 36氪)
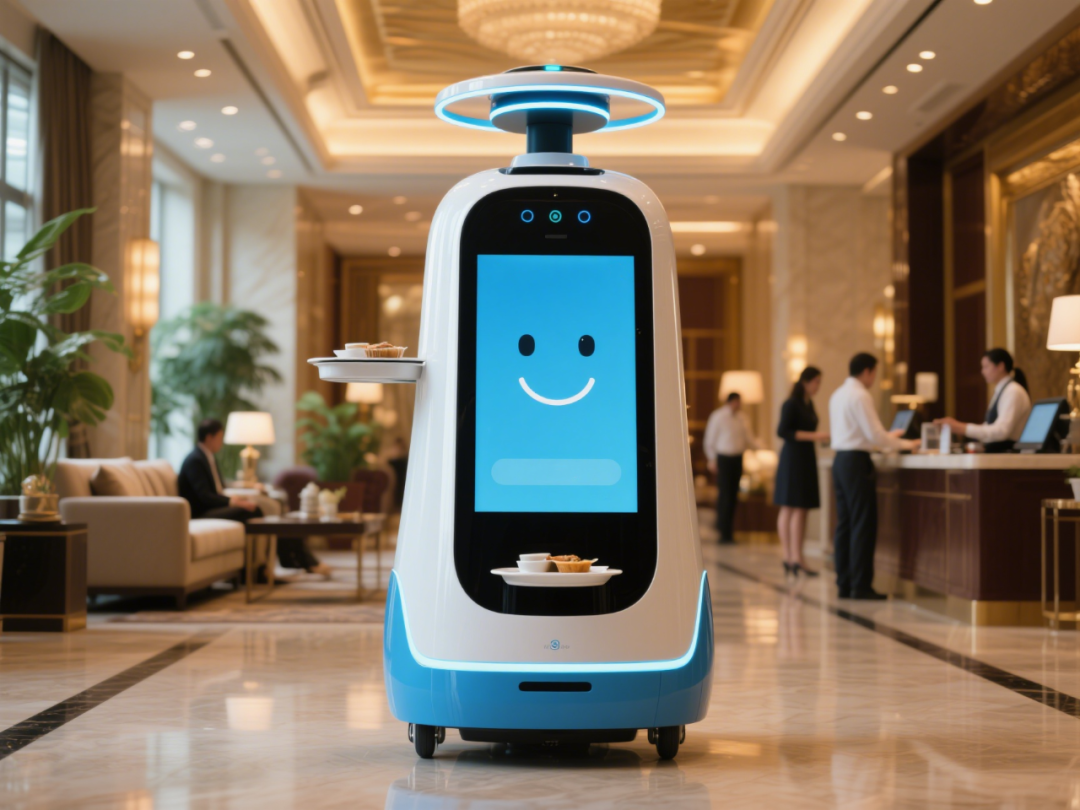
OpenAI Governance Crisis: The Clash Between Capital and Mission Sparks Deep Reflection on AI Development Paths: OpenAI’s unique “capped-profit” structure, where a for-profit subsidiary is overseen by a non-profit organization, aims to balance AI technology development with human welfare. However, CEO Altman’s recent consideration of transitioning the company to a more traditional for-profit entity has raised concerns among AI experts and legal scholars. They believe this move could lead key decision-makers to no longer prioritize OpenAI’s philanthropic mission, weaken restrictions on investor profits, and potentially alter the timeline and direction of AGI development. This struggle over control, profit distribution, and the social and ethical shaping of AI highlights the challenges and loopholes facing existing corporate governance frameworks in the era of rapid AI advancement. (Source: 36氪)