Keywords:Prime Intellect, INTELLECT-2, Sakana AI, Transformer, Google AI Agent, Continuous Thinking Machine, AgentOps, Multi-agent Collaboration, Distributed Reinforcement Learning Training, Neural Timing and Neuron Synchronization, AI Agent Operations Workflow, Multi-agent Architecture, Enterprise Deployment of AI Agents
🔥 Focus
Prime Intellect Open Sources INTELLECT-2 Model: Prime Intellect has released and open-sourced INTELLECT-2, a 32 billion parameter model claimed to be the first trained via globally distributed reinforcement learning. The release includes a detailed technical report and model checkpoints. The model demonstrates performance comparable or superior to models like Qwen 32B on multiple benchmarks, particularly excelling in code generation and mathematical reasoning, and has been discovered by community members to be capable of playing Wordle. Its training method and open-sourcing are considered potentially impactful on the future landscape of large model training and competition (Source: Grad62304977, teortaxesTex, eliebakouch, Sentdex, Ar_Douillard, andersonbcdefg, scaling01, andersonbcdefg, vllm_project, tokenbender, qtnx_, Reddit r/LocalLLaMA, teortaxesTex)
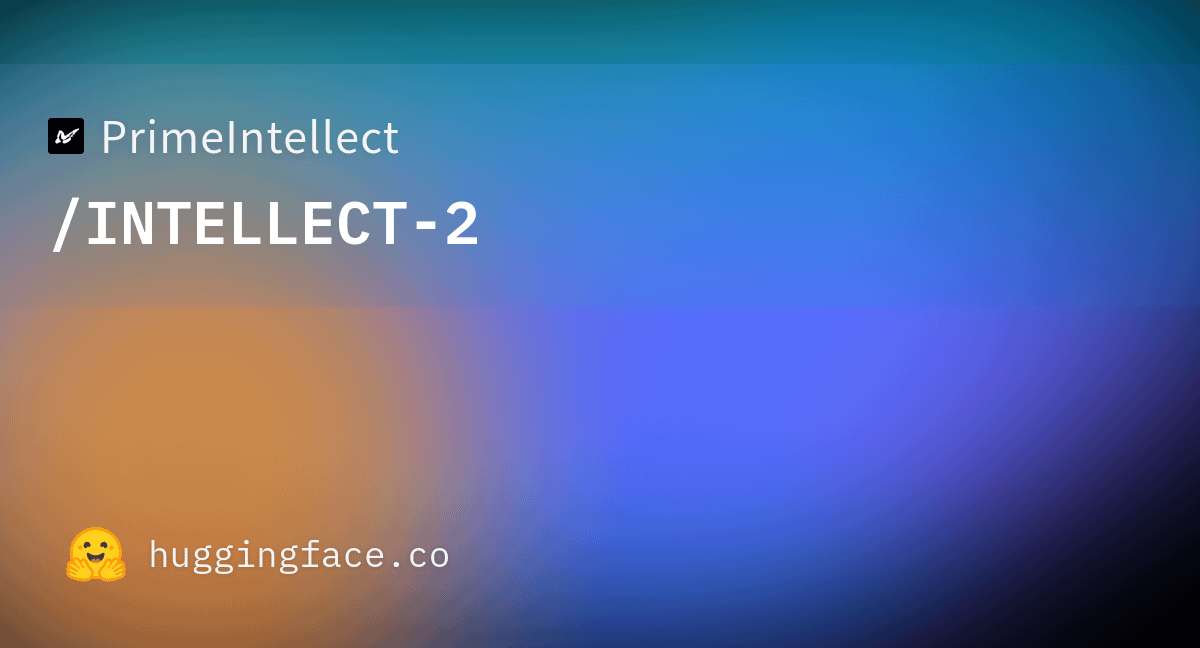
Sakana AI Proposes Continuous Thought Machine (CTM): Sakana AI has introduced a novel neural network architecture called the “Continuous Thought Machine” (CTM), aiming to endow AI with more flexible human-like intelligence by incorporating biological brain mechanisms such as neural timing and neuronal synchronization. The core innovation of CTM lies in neuron-level temporal processing and using neural synchronization as a latent representation, enabling it to handle tasks requiring sequential reasoning and adaptive computation, as well as storing and retrieving memories. The research, exploring a new paradigm of AI “thinking with time,” has been released with a blog post, interactive report, paper, and GitHub repository (Source: SakanaAILabs, Plinz, SakanaAILabs, SakanaAILabs, hardmaru, teortaxesTex, tokenbender, Reddit r/MachineLearning)
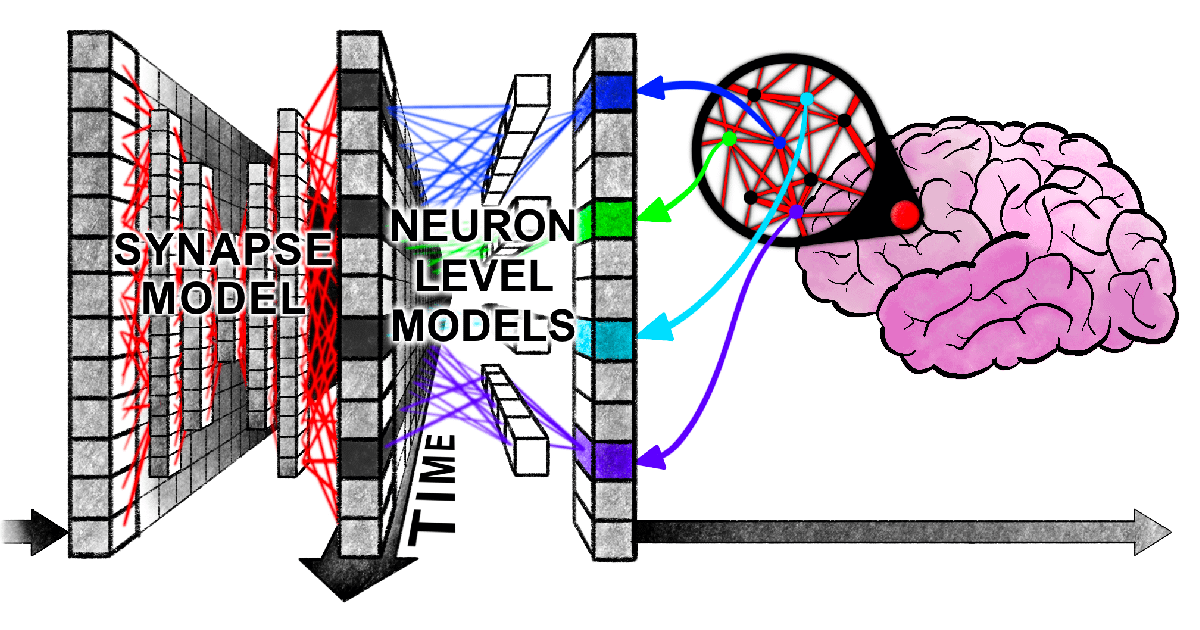
New Harvard Paper Reveals “Synchronized Entanglement” Between Transformers and Human Brain in Information Processing: Researchers from Harvard University and other institutions published a paper titled “Linking forward-pass dynamics in Transformers and real-time human processing,” exploring the similarities between the internal processing dynamics of Transformer models and human real-time cognitive processes. Instead of just looking at the final output, the study analyzes “processing load” metrics (like uncertainty, confidence change) at each layer of the model, finding that AI also experiences processes similar to human “hesitation,” “intuitive errors,” and “correction” when solving problems (such as answering capitals, animal classification, logical reasoning, image recognition). This similarity in the “thinking process” suggests that AI naturally learns cognitive shortcuts similar to humans to accomplish tasks, offering new perspectives for understanding AI decision-making and guiding human experimental design (Source: 36Kr)
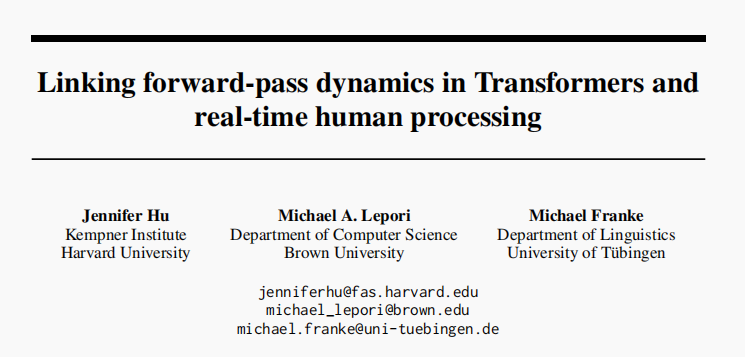
Google Releases 76-Page AI Agent White Paper, Elaborating on AgentOps and Multi-Agent Collaboration: Google’s latest AI agent white paper details the construction, evaluation, and application of AI agents. The paper emphasizes the importance of Agent Operations (AgentOps), a process for optimizing the building and deployment of agents into production environments, covering tool management, core prompt setting, memory implementation, and task decomposition. The white paper also explores multi-agent architectures, where multiple specialized agents collaborate to achieve complex goals, and introduces Google’s practical cases of deploying agents internally (e.g., NotebookLM Enterprise Edition, Agentspace Enterprise Edition) and specific applications (e.g., automotive multi-agent systems), aiming to enhance enterprise productivity and user experience (Source: 36Kr)
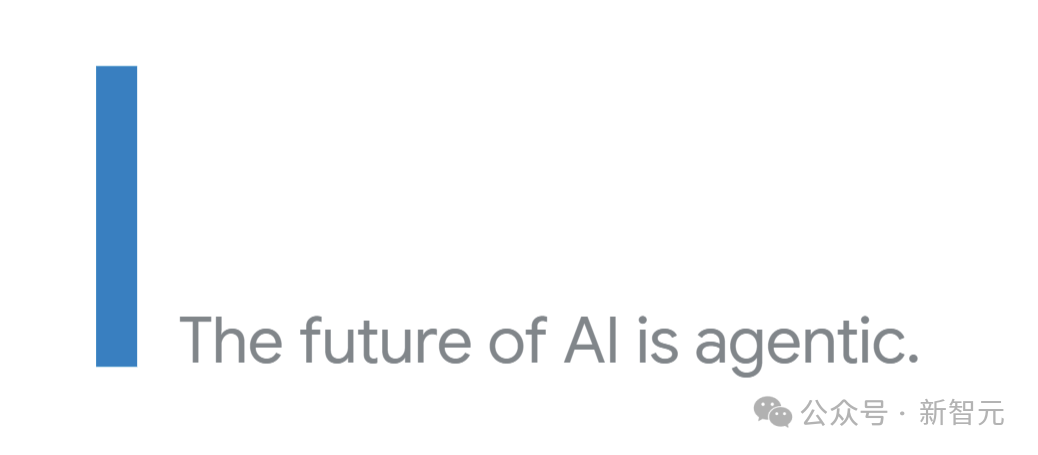
🎯 Trends
LightonAI Releases GTE-ModernColBERT-v1 Semantic Search Model: LightonAI has launched a new semantic search model, GTE-ModernColBERT-v1, which achieved the current top score on the LongEmbed / LEMB Narrative QA benchmark. This model is specifically designed to enhance semantic search performance and can be applied to scenarios like document content retrieval and RAG, integrating with existing systems. It is reported that the model was fine-tuned from Alibaba-NLP/gte-modernbert-base, aiming to overcome the limitations of traditional search engines relying solely on character matching (Source: karminski3)
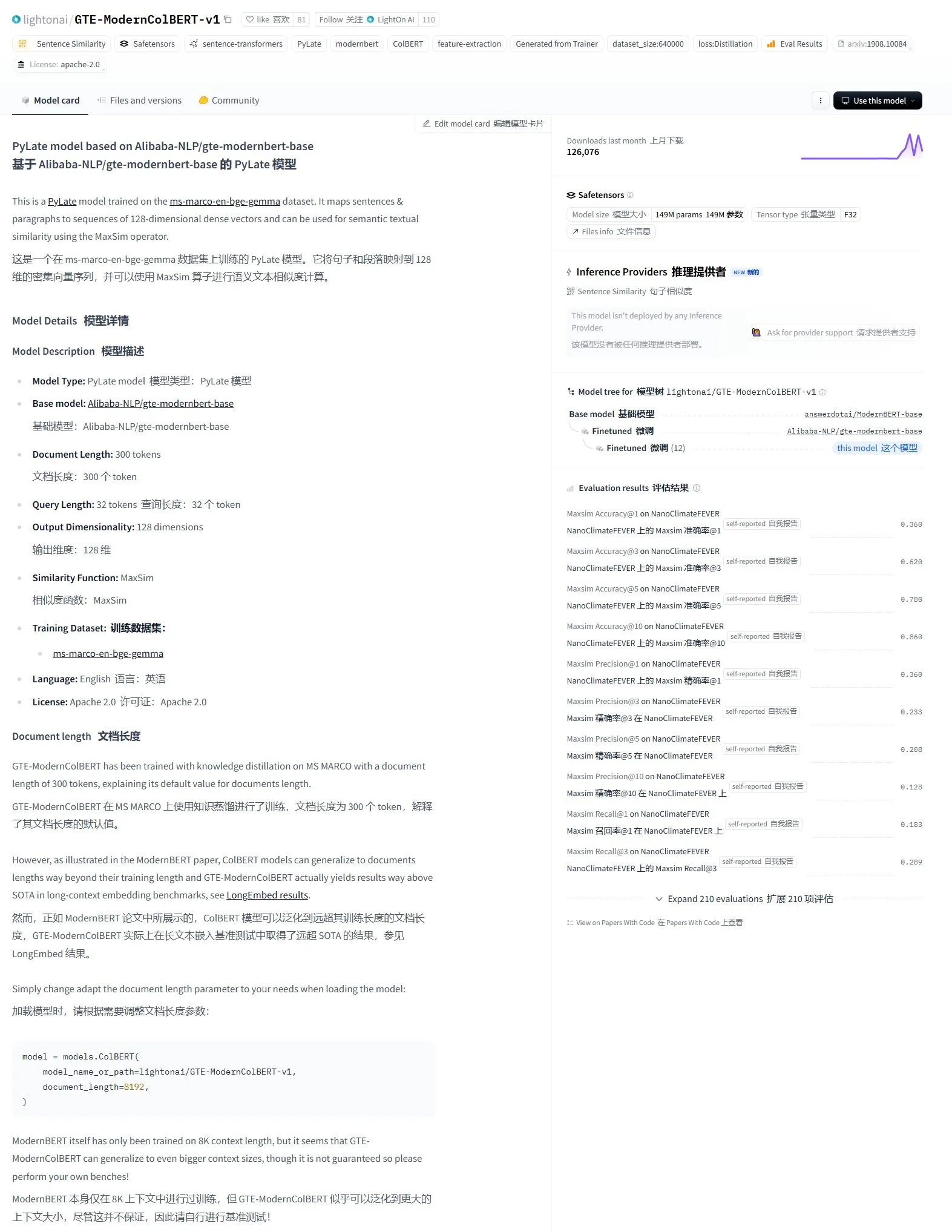
Tech Leaders Focus on DeepSeek’s Rapid Rise: VentureBeat reported on the reactions of tech leaders to the rapid development of DeepSeek. With its powerful model capabilities and open-source strategy, DeepSeek has achieved significant success in the global AI field, especially in math and code generation tasks, posing a challenge to the existing market landscape (including OpenAI). Its low-cost training and API pricing strategy also promote the popularization and commercialization of AI technology (Source: Ronald_vanLoon)
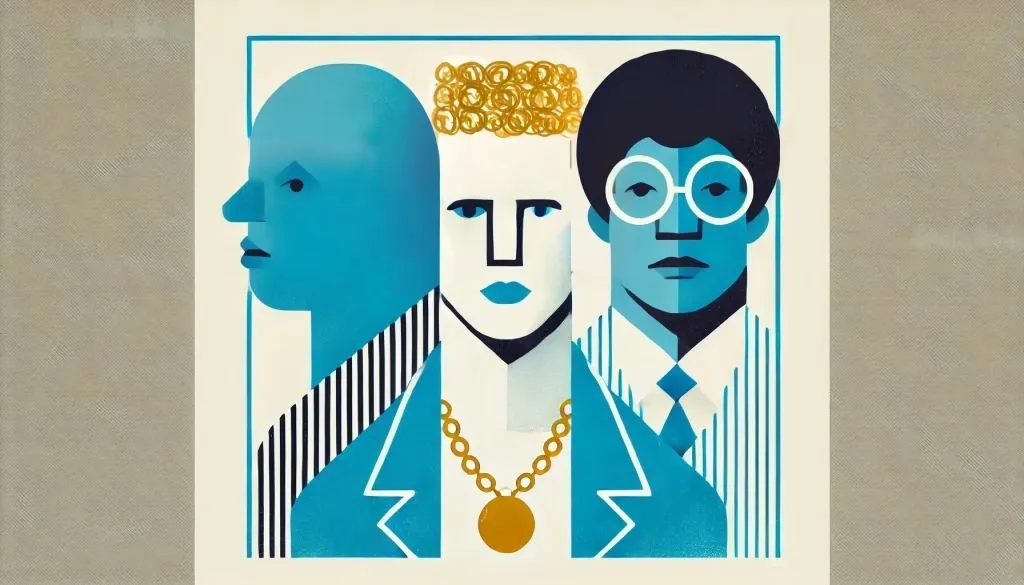
ByteDance and Peking University Jointly Release DreamO, a Unified Image Customization Framework Supporting Multi-Condition Combination: ByteDance, in collaboration with Peking University, has launched DreamO, a framework that enables free combination of multiple conditions such as subject, identity, style, and clothing reference for customized image generation using a single model. Built upon Flux-1.0-dev, the framework introduces specialized mapping layers to handle conditional image inputs and employs progressive training strategies and routing constraints for reference images to enhance generation quality and consistency. With only 400M training parameters, DreamO can generate a customized picture in 8-10 seconds, performing excellently in maintaining consistency. The relevant code and model have been open-sourced (Source: WeChat)
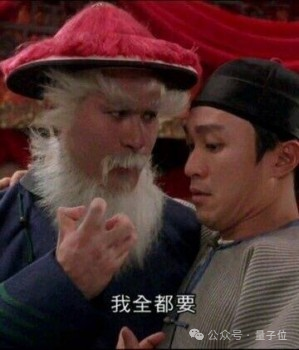
VITA Team Open Sources Real-Time Large Speech Model VITA-Audio, Significantly Improving Inference Efficiency: The VITA team has introduced the end-to-end speech model VITA-Audio, which achieves direct generation of decodable Audio Token Chunks in a single forward pass by incorporating a lightweight Multi-modal Cross-modal Token Prediction (MCTP) module. At the 7B parameter scale, the model takes only 92ms from receiving text to outputting the first audio segment (53ms excluding the audio encoder), achieving inference speeds 3-5 times faster than models of similar scale. VITA-Audio supports both Chinese and English, is trained solely on open-source data, performs excellently in tasks like TTS and ASR, and its code and model weights have been open-sourced (Source: WeChat)

Tsinghua, BAAI, etc. Propose “Absolute Zero” Training Method, Enabling Large Models to Unlock Reasoning Ability Through Self-Play: Researchers from Tsinghua University, Beijing Academy of Artificial Intelligence (BAAI), and other institutions proposed the “Absolute Zero” training method, allowing pre-trained large models to learn reasoning by generating and solving tasks through self-play without external data. This method uniformly represents reasoning tasks as (program, input, output) triplets, with the model playing the roles of Proposer and Solver, learning through abduction, deduction, and induction task types. Experiments show that models trained with this method achieve significant improvements in code and mathematical reasoning tasks, outperforming models trained with expert-annotated samples (Source: WeChat)
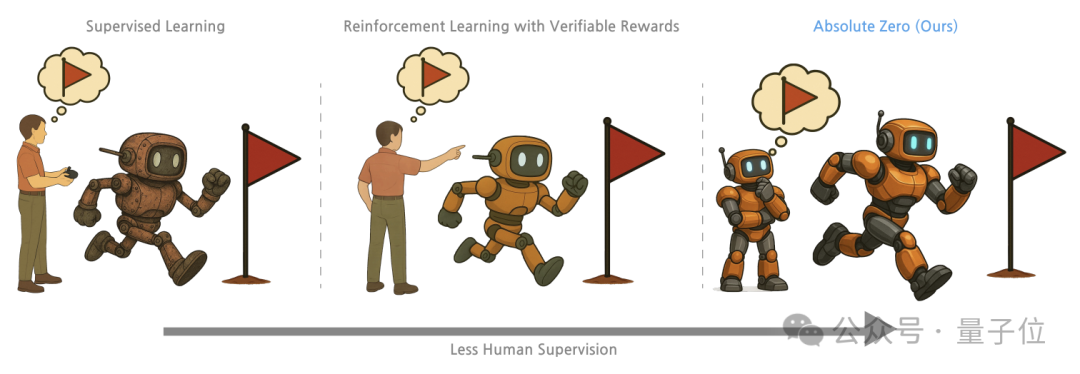
AI PC Development Accelerates, Lenovo and Huawei Launch New AI Terminal Products: Lenovo and Huawei have recently launched PC products integrated with AI agents, such as Lenovo’s Tianxi Personal Super Agent and Huawei’s HarmonyOS PC equipped with the Xiaoyi Agent. Although the market penetration rate of AI PCs is still low, the growth rate is rapid. Canalys data shows that AI PC shipments in mainland China accounted for 15% of the overall PC market in 2024, and it is expected to reach 34% in 2025. Industry insiders believe that the AI PC industry chain will take another 2-3 years to mature, with current main challenges being the cost and scale of supply chains for memory, chips, etc., and the fragmentation of the domestic AI PC ecosystem. Future trends include agents becoming the core interaction portal, localized deployment of AI, and the diversification of AI application scenarios into education, health, etc. (Source: 36Kr)
Global AI App Downloads Surge, Domestic Market Cools Down, Doubao Grows Against Trend: In April 2025, global AI app downloads on both platforms reached 330 million, a month-on-month increase of 27.4%. ChatGPT, Google Gemini, DeepSeek, Genius, and Doubao ranked in the top five. ChatGPT saw a surge in downloads driven by the release of GPT-4o. In contrast, the download volume of AI apps on the Apple platform in mainland China decreased by 24.0% month-on-month. Doubao grew against the trend, ranking first, followed by DeepSeek and Jimi AI (即梦AI). In terms of ad buying, Tencent Yuanbao and Kuark invested heavily, accounting for the majority of ad creatives, while Doubao’s spending declined. Overall, the domestic AI market heat has somewhat cooled, with competition returning to technology and operations (Source: 36Kr)
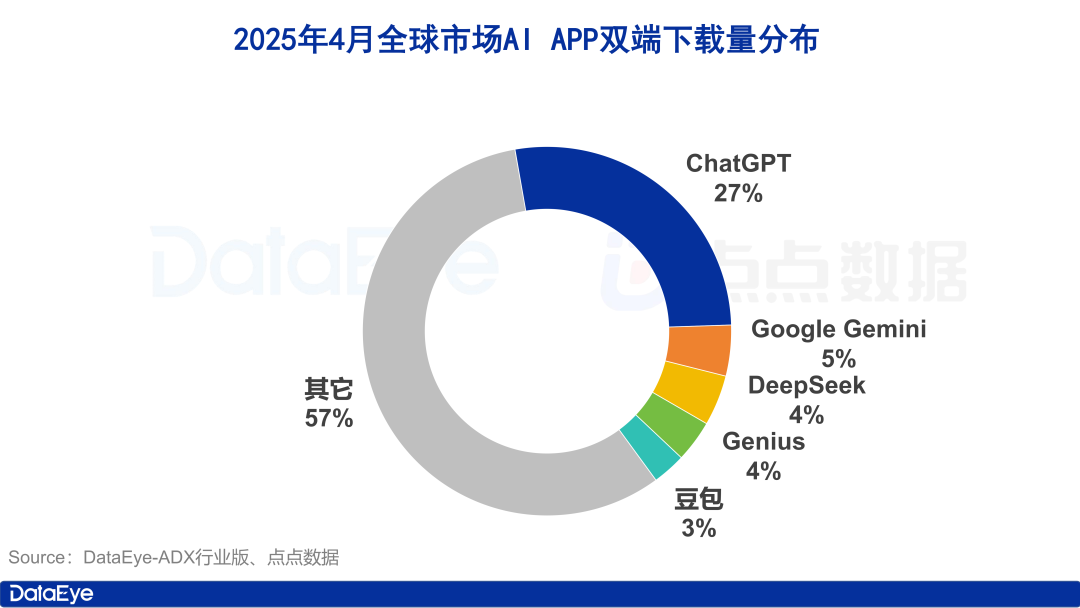
China’s Large Model Market Reshuffles, Emergence of “Top 5 Foundation Model Players”: As the global AI funding environment tightened in 2024, China’s large model market underwent a “de-bubbling,” with the previous “Six Little Tigers” landscape evolving into the “Top 5 Foundation Model Players” represented by ByteDance, Alibaba, StepFun (阶跃星辰), Zhipu AI, and DeepSeek. These leading players have distinct advantages in funding, talent, and technology, and are pursuing differentiated paths: ByteDance has a comprehensive layout, Alibaba focuses on open source and full-stack, StepFun specializes in multimodal, Zhipu AI leverages its Tsinghua background for 2B/2G markets, and DeepSeek breaks through with extreme engineering optimization and open-source strategies. The next stage of competition will focus on breaking the “intelligence ceiling” and enhancing “multimodal capabilities” to achieve the vision of AGI (Source: 36Kr, WeChat)
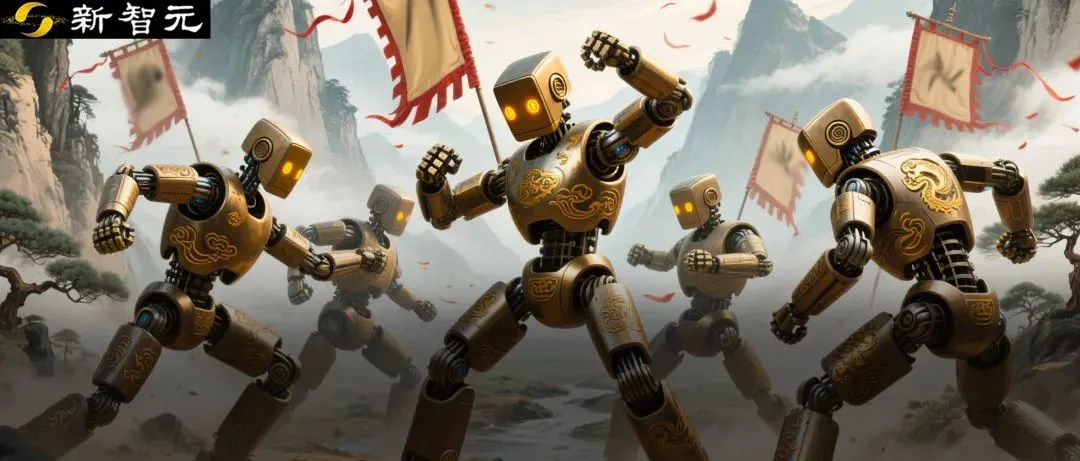
Record ICCV 2025 Submissions Spark Concerns Over Review Quality, LLM Use in Reviews Banned: The computer vision conference ICCV 2025 received a record 11,152 paper submissions. However, after the review results were announced, numerous authors expressed dissatisfaction with the review quality on social media, considering some reviews perfunctory, even worse than GPT’s level, and pointing out issues like reviewers not reading supplementary materials. To cope with the surge in submissions, the conference required every submitting author to participate in reviewing and explicitly banned the use of large models (like ChatGPT) during the review process to ensure originality and confidentiality. Although official data shows 97.18% of reviews were submitted on time, review quality and reviewer burden have become hot topics of discussion (Source: 36Kr)
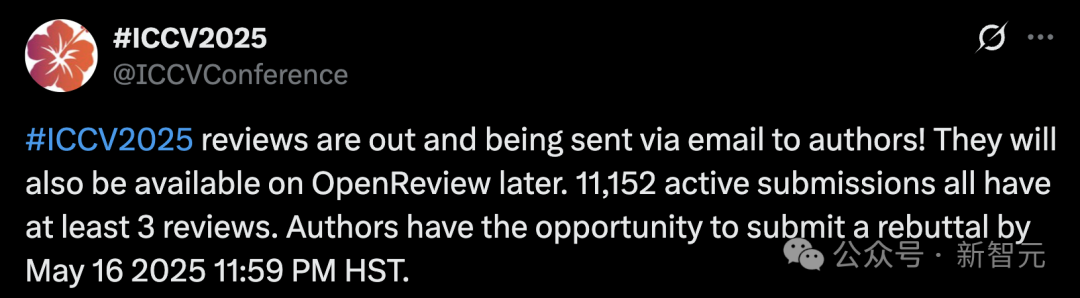
Nvidia CEO Jensen Huang: All Employees Will Be Equipped with AI Agents, Reshaping Developer Roles: Nvidia CEO Jensen Huang stated that the company will equip all employees (including software engineers and chip designers) with AI agents to improve work efficiency, project scale, and software quality. He foresees a future where everyone will command multiple AI assistants, leading to exponential productivity growth. This trend aligns with the views of companies like Meta, Microsoft, and Anthropic, suggesting that AI will handle most coding tasks, shifting the developer’s role to “AI commander” or “requirements definer.” Huang emphasized that energy and computing power are bottlenecks for AI popularization, requiring innovation in areas like chip packaging and photonics technology. Major companies are actively developing proactive AI agents, signaling a shift from GenAI to Agentic AI (Source: 36Kr)
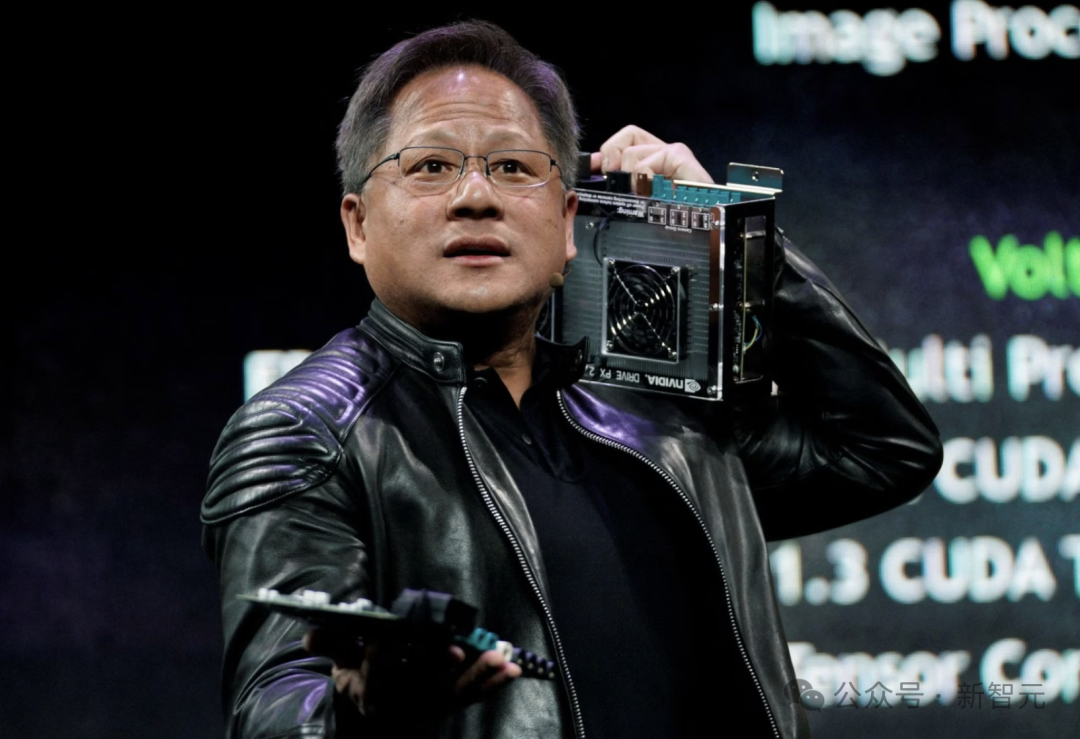
OpenAI CEO Altman Testifies Before Congress, Calls for Lenient Regulation and Reveals Open Source Plans: OpenAI CEO Sam Altman stated during a US Senate hearing that strict pre-approval for AI would be disastrous for America’s competitiveness in the field and revealed that OpenAI plans to release its first open-source model this summer. He emphasized that infrastructure (especially energy) is crucial for winning the AI race and believes the cost of AI will eventually converge with the cost of energy. Altman also shared his “Roadmap for the Age of Intelligence (2025-2027),” predicting the successive arrival of AI super assistants, exponential growth in AI-driven scientific discovery, and the era of AI robots. Regarding his personal life, he expressed that he would not want his son to form close friendships with AI robots (Source: 36Kr)
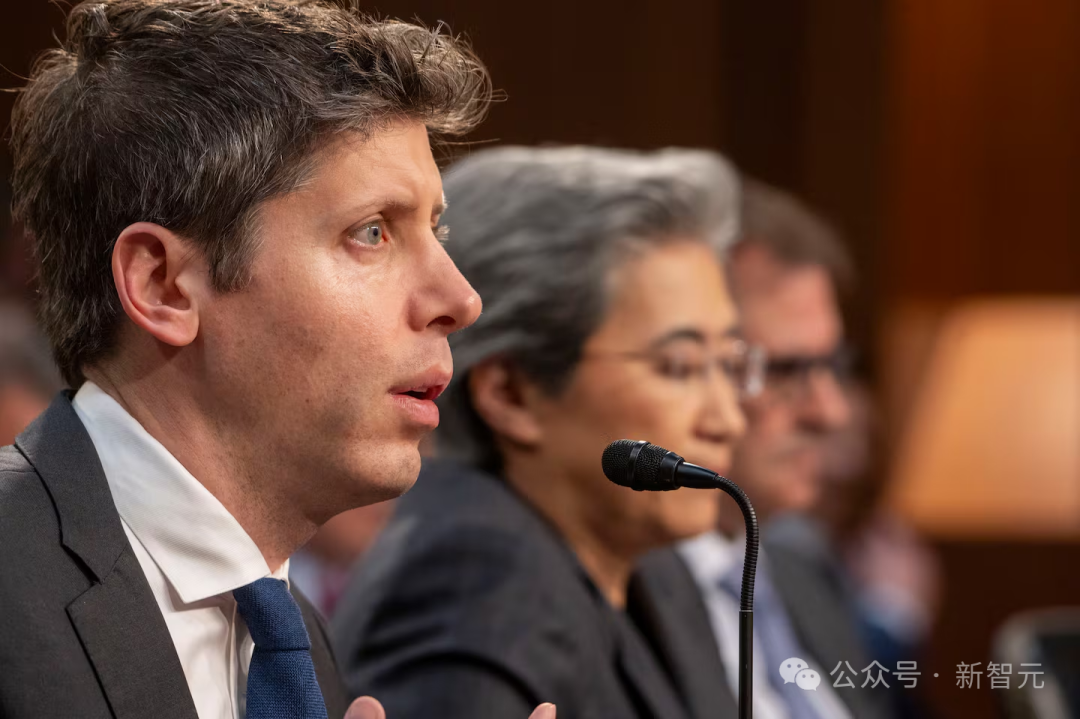
CMU Researchers Propose LegoGPT, Using AI to Design Physically Stable Lego Models: Researchers at Carnegie Mellon University have developed LegoGPT, an AI system that can convert text descriptions into physically buildable Lego models. By fine-tuning Meta’s LLaMA model and training it on the StableText2Lego dataset containing over 47,000 stable structures, LegoGPT can progressively predict brick placement, ensuring the generated structures are physically stable in the real world with a success rate of 98.8%. The system also utilizes a physics-aware rollback method to correct detected unstable structures. The researchers believe this technology is not limited to Lego and could be applied to designing 3D printed components and robot assembly in the future. The code, dataset, and model are currently open-sourced (Source: WeChat)
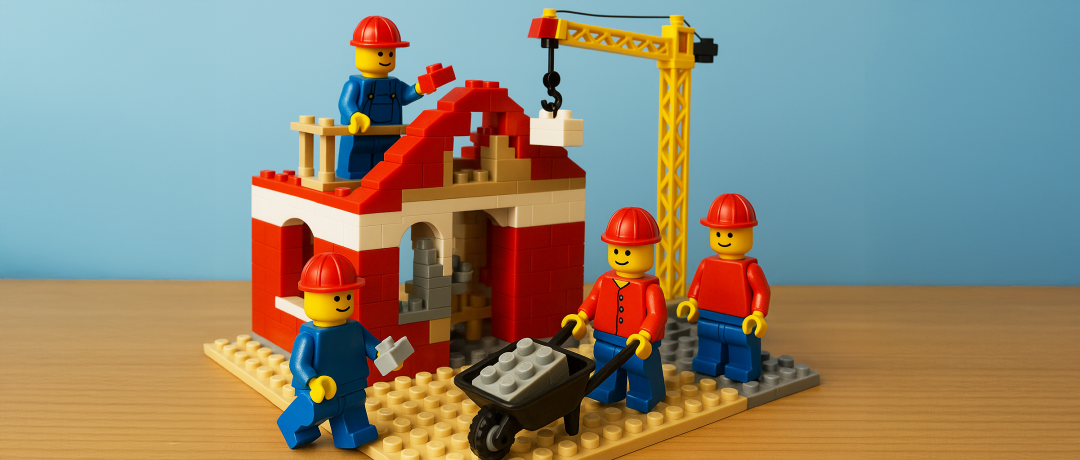
AI Prediction of Papal Election Fails, New Pope Robert Prevost Becomes “Surprise Choice”: According to Science, a study using AI algorithms to analyze data from 135 cardinals to predict the next pope failed to predict the election of Robert Francis Prevost. The model simulated the election based on the cardinals’ stances on key issues (judged by AI trained on their speeches to determine conservative or progressive leanings) and their ideological similarities, ultimately predicting Italian Cardinal Pietro Parolin as the most likely winner. Researchers acknowledged that the model’s failure to consider political and geographical factors was a major flaw but believe the methodology still holds value for predicting other types of elections. Prevost holds moderate views on various issues, potentially making him an acceptable compromise candidate for all parties (Source: 36Kr)
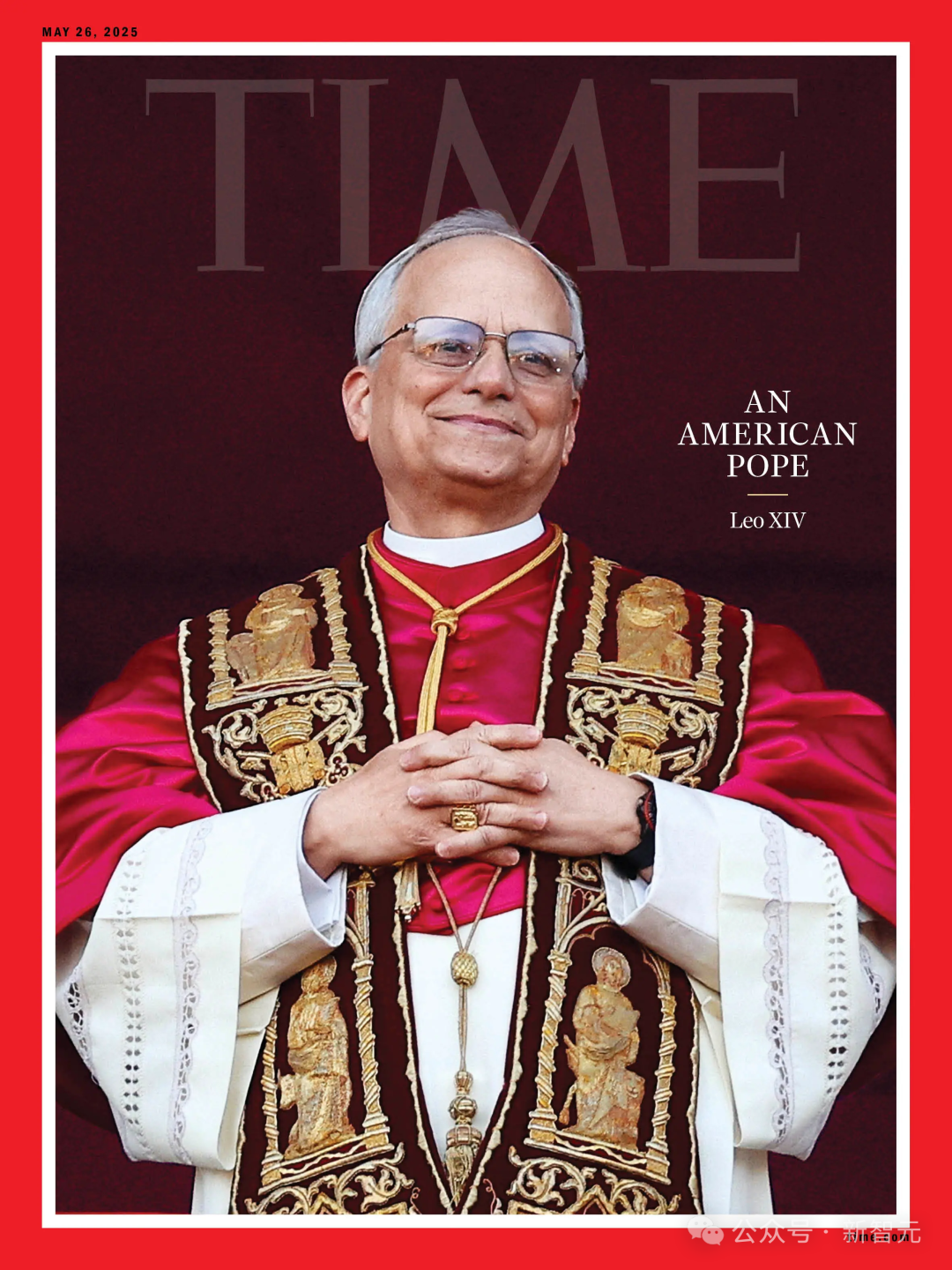
AI Application in Financial Marketing: Solving Five Major Challenges including Customer Acquisition, Personalization, and Compliance: AI and Agent technologies are becoming the core drivers of the Financial Marketing 3.0 era, aiming to address pain points such as high customer acquisition costs, insufficient personalized experiences, complex product understanding, significant compliance pressure, and difficulty in measuring ROI. By building an “intelligent marketing middle platform” (data foundation + intelligent engine + service applications) and utilizing technologies like LLM+RAG, knowledge graphs, Multi-Agent Systems (MAS), and privacy computing, financial institutions can achieve deeper customer insights, real-time precise intelligent decision-making, and efficient, consistent service execution. Industry cases show that AI has already achieved significant results in increasing customer AUM, financial product conversion rates, and marketing content production efficiency. Future development directions include multimodal interaction, causal decision-making, autonomous evolution, edge response, and human-machine collaboration (Source: 36Kr)
AI-Powered Robots Tackle Europe’s E-Waste Problem: The EU-funded research project ReconCycle has developed AI-powered adaptive robots for automating the processing of growing electronic waste, particularly dismantling devices containing lithium batteries. These robots can be reconfigured for different tasks, such as removing batteries from smoke detectors and radiator heat meters. The technology aims to improve recycling efficiency, reduce the arduous and hazardous nature of manual dismantling, and address the challenge of nearly 5 million tonnes of e-waste generated annually in the EU (with less than 40% recycled). Recycling facilities like Electrocycling GmbH are starting to pay attention and anticipate that such technologies can enhance raw material recovery rates and reduce economic losses and carbon emissions (Source: aihub.org)
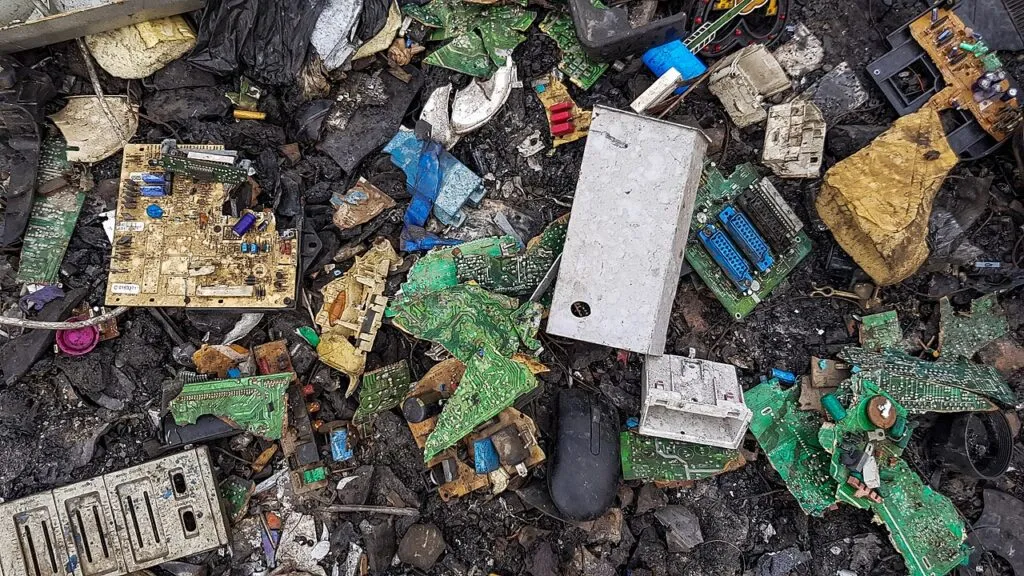
🧰 Tools
LocalSite-ai: Open Source Alternative to DeepSite for AI-Generated Front-End Pages Online: LocalSite-ai is an open-source project offering functionality similar to DeepSite, allowing users to generate front-end pages online using AI. It supports online preview, WYSIWYG editing, and is compatible with multiple AI API providers. Additionally, the tool supports responsive design, helping users quickly build web pages adaptable to different devices (Source: karminski3)
Agentset: Open Source Platform to Enhance RAG Result Precision: Agentset is an open-source RAG (Retrieval Augmented Generation) platform that optimizes the precision of retrieval results through hybrid search and re-ranking techniques. The platform has built-in citation functionality, clearly showing which indexed information in the vector database the generated content originates from, facilitating user verification to avoid information errors or model hallucinations (Source: karminski3)
Gemini Max Playground: Gemini Application with Parallel Preview and Version Control: Developer Chansung created a Hugging Face Space application called Gemini Max Playground, allowing users to process up to 4 Gemini previews in parallel to speed up the iteration process. The tool supports controlling the number of inference tokens, features version control, and can export HTML/JS/CSS files separately. Additionally, a version optimized for mobile screens is provided (Source: algo_diver)
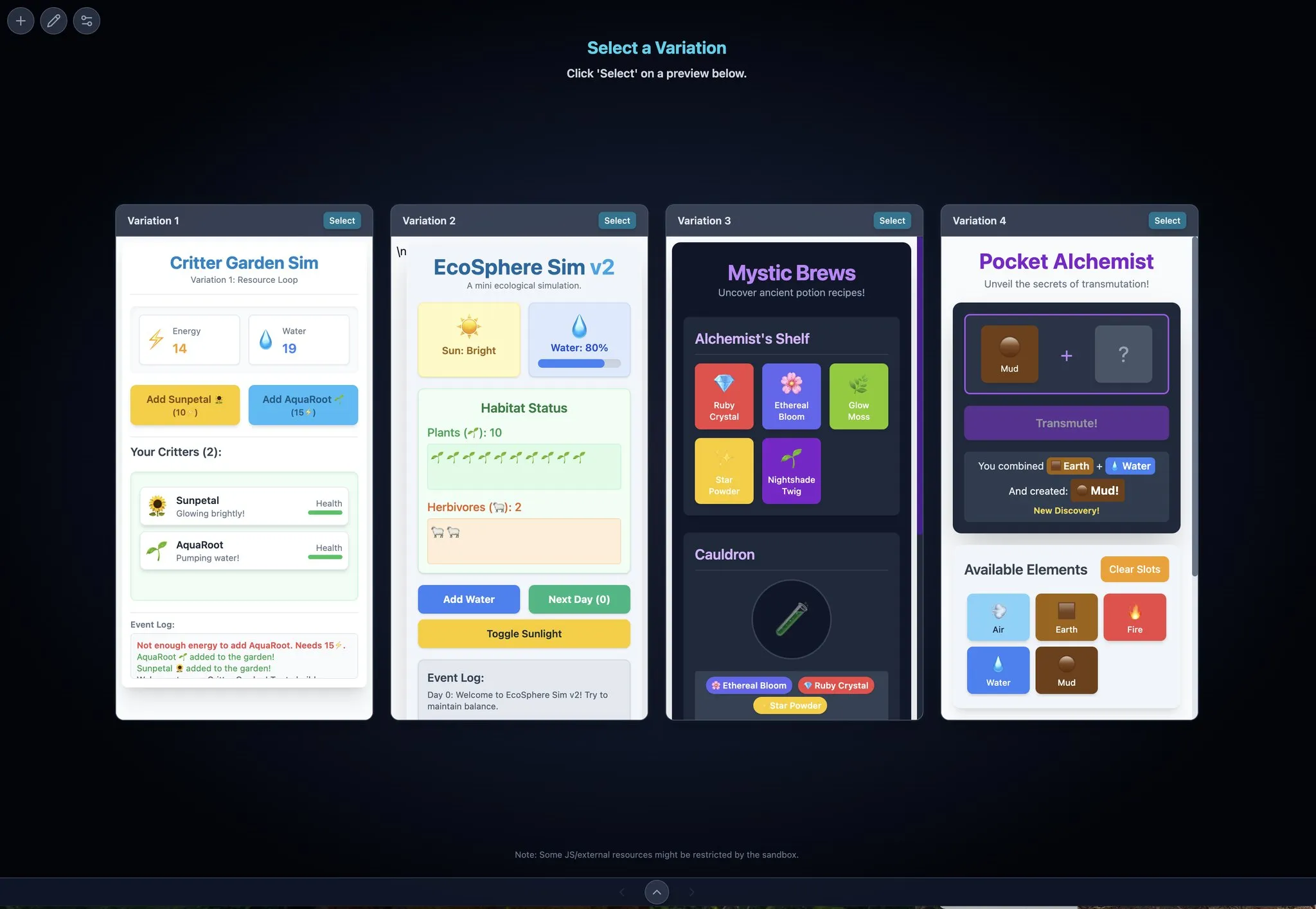
mlop.ai: Open Source Alternative to Weights and Biases (wandb): mlop.ai has been launched as a fully open-source, high-performance, and secure ML experiment tracking platform intended as an alternative to wandb. It is fully compatible with the wandb API, offering low migration cost (requiring only a one-line code change). Its backend is written in Rust and claims to solve the blocking issue present in wandb’s .log
calls, providing non-blocking logging and uploading. Users can easily self-host it via Docker (Source: Reddit r/artificial)
DeerFlow: ByteDance Open Sources LLM+Langchain+Tools Framework: ByteDance has open-sourced DeerFlow (Deep Exploration and Efficient Research Flow), a framework integrating large language models (LLM), Langchain, and various tools (such as web search, crawlers, code execution). The project aims to provide powerful support for research and development workflows and supports Ollama for convenient local deployment and use (Source: Reddit r/LocalLLaMA)
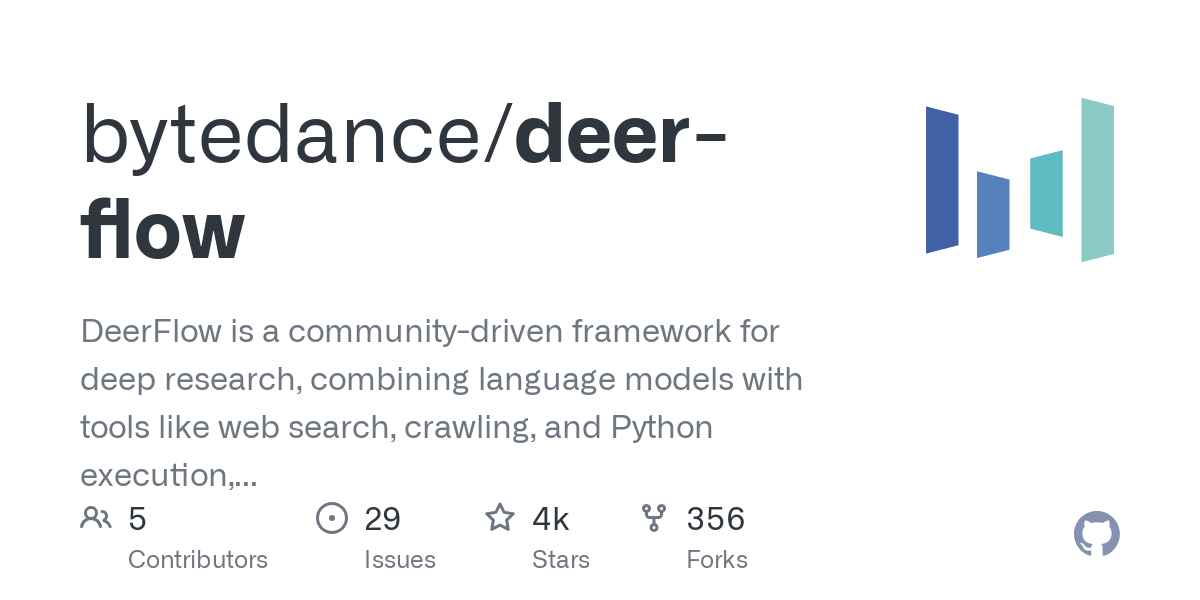
Plexe: Open Source ML Agent for Natural Language to Trained Models: Plexe is an open-source ML engineering agent that transforms natural language prompts into machine learning models trained on user’s structured data (currently supporting CSV and Parquet files), without requiring the user to have a data science background. It automatically completes tasks like data cleaning, feature selection, model experimentation, and evaluation through a team of specialized agents (Scientist, Trainer, Evaluator) and uses MLflow to track experiments. Future plans include supporting PostgreSQL databases and a feature engineering agent (Source: Reddit r/artificial)
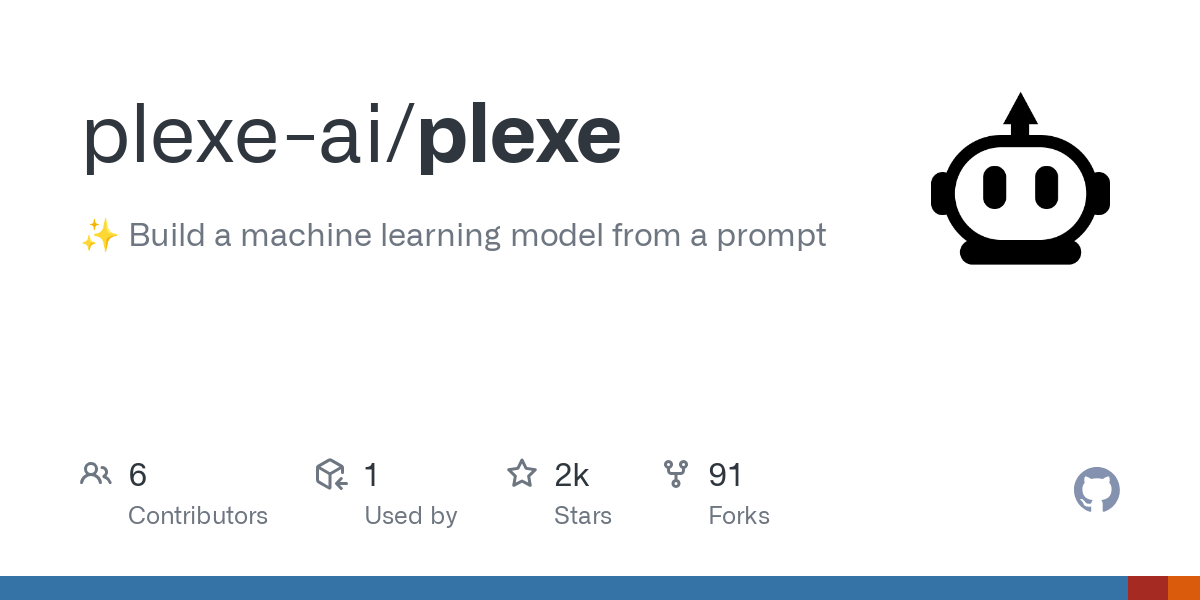
Llama ParamPal: LLM Sampling Parameter Knowledge Base Project: Llama ParamPal is an open-source project aimed at collecting and providing recommended sampling parameters for local large language models (LLMs) when using llama.cpp. The project includes a models.json
file as a parameter database and provides a simple Web UI (under development) for browsing and searching parameter sets, addressing the pain point users face when looking for suitable parameters for new models. Users can contribute parameter configurations for their own models (Source: Reddit r/LocalLLaMA)
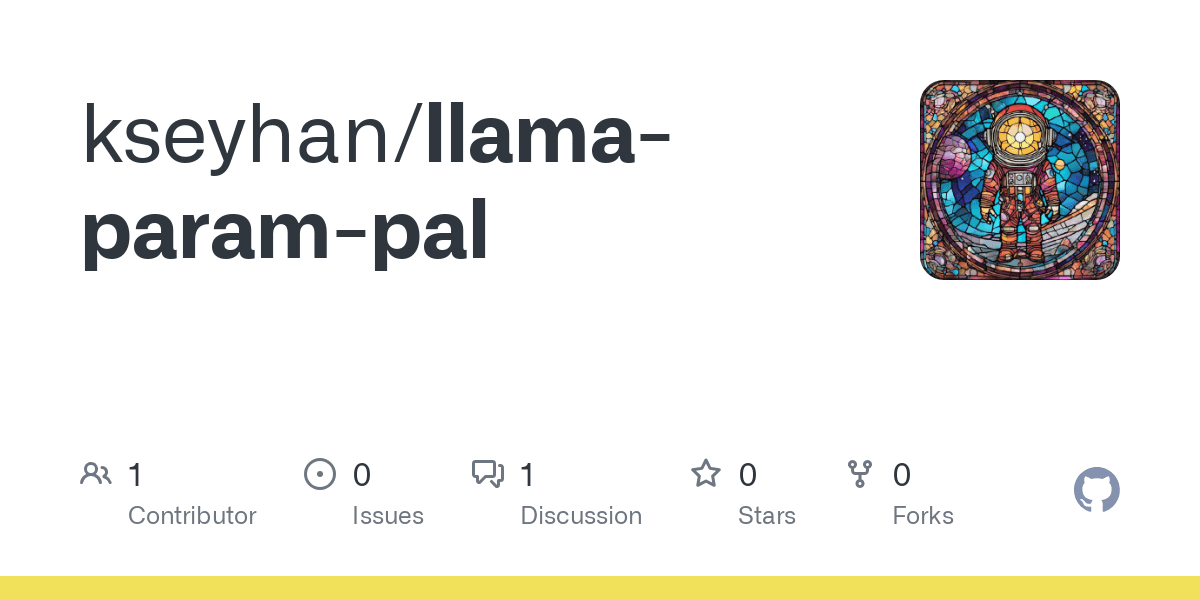
TFrameX and Studio: Open Source Local LLM Agent Builder and Framework: The TesslateAI team has released two open-source projects: TFrameX, an agent framework specifically designed for local large language models (LLMs); and Studio, a flowchart-based agent builder. These tools aim to help developers more easily create and manage AI agents that work collaboratively with local LLMs. The team states they are actively developing and welcome community contributions (Source: Reddit r/LocalLLaMA)
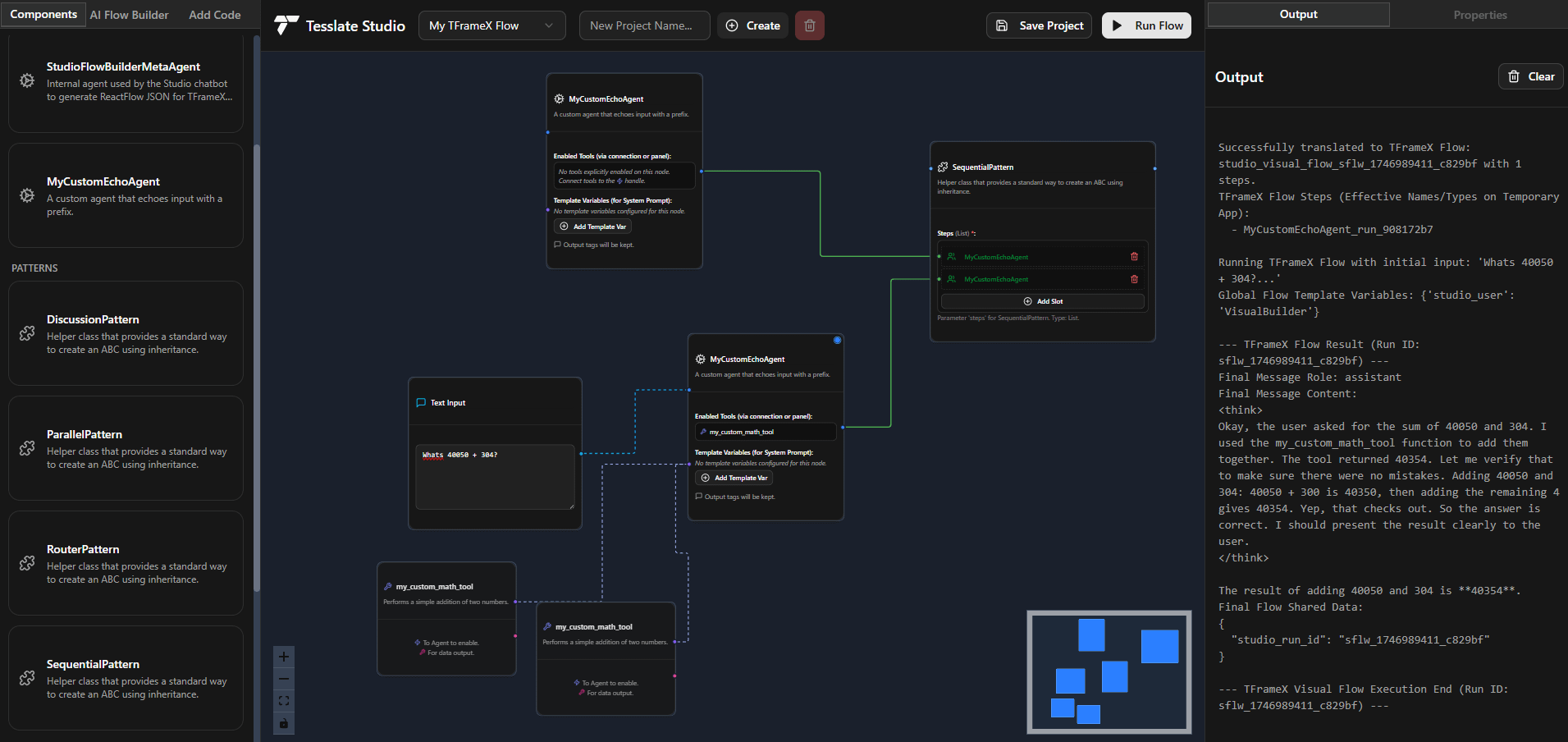
Ktransformer: Efficient Inference Framework Supporting Ultra-Large Models: Ktransformer is an inference framework that, according to its documentation, can handle ultra-large models like Deepseek 671B or Qwen3 235B using only 1 or 2 GPUs. Although less discussed than Llama CPP, some users point out it might outperform Llama CPP in performance, especially when the KV cache resides solely in GPU memory. However, it may lack features for tool calling and structured responses, and handling long contexts with limited VRAM remains challenging for models that do not support MLA (like Qwen) (Source: Reddit r/LocalLLaMA)
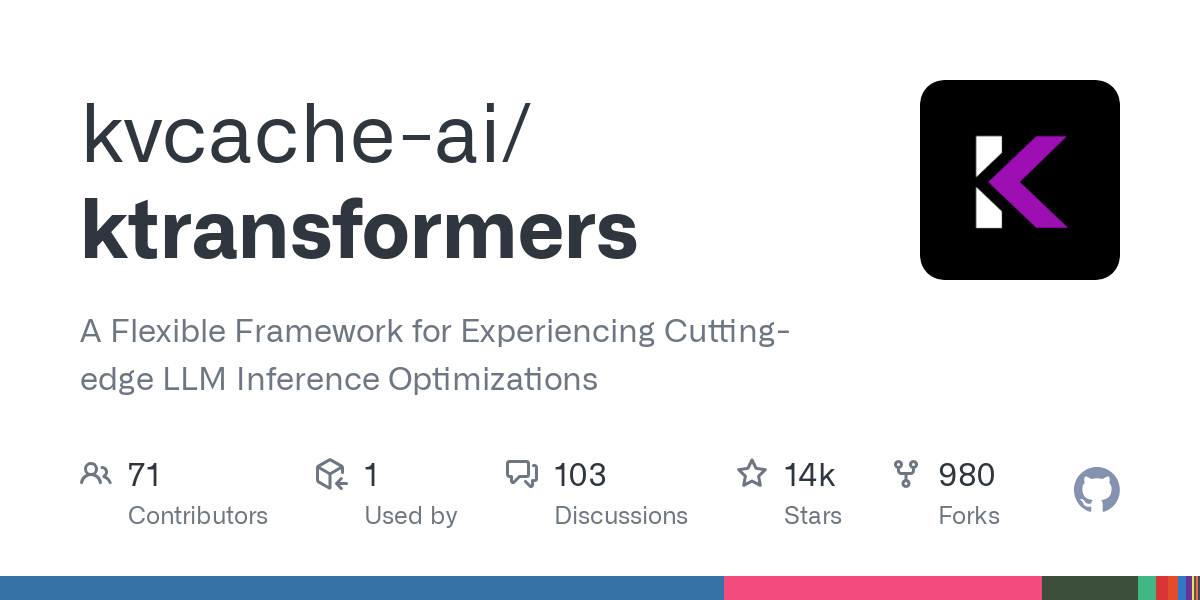
📚 Learning
DSPy Framework Explained: Declarative Self-improving Python for LLM Programming: DSPy (Declarative Self-improving Python) is a framework for programming large language models (LLMs). Its core idea is to treat LLMs as programmable “general-purpose computers,” defining inputs, outputs, and transformations (Signatures) declaratively, rather than forcing specific LLM behaviors. DSPy’s modules and optimizers allow programs to self-improve in terms of quality and cost, aiming to provide a more structured and efficient programming paradigm for LLMs to meet the demands of complex production applications. The community considers this a significant advancement in LLM programming, with usage expected to surge in the future (Source: lateinteraction, lateinteraction)
Peking University, Tsinghua, etc. Jointly Release Latest Survey on Logical Reasoning Capabilities of Large Models: Researchers from Peking University, Tsinghua University, University of Amsterdam, Carnegie Mellon University, and MBZUAI have jointly released a survey paper on the logical reasoning capabilities of large language models (LLMs), accepted by the IJCAI 2025 Survey Track. The survey systematically reviews cutting-edge methods and evaluation benchmarks for enhancing LLM performance in logical question answering and logical consistency. It categorizes logical QA methods based on external solvers, prompt engineering, pre-training, fine-tuning, etc., and discusses concepts like negation, entailment, transitivity, factual, and compositional consistency, along with techniques to enhance them. The paper also points out future research directions, such as extending to modal logic and higher-order logic reasoning (Source: WeChat)

Terence Tao’s YouTube Debut: Completes Math Proof in 33 Minutes with AI Assistance and Upgrades Proof Assistant: Renowned mathematician Terence Tao made his YouTube debut, demonstrating how to complete a universal algebra proposition proof (Magma equation E1689 implies E2), which would typically require a human mathematician to fill a page, in 33 minutes with the help of AI (specifically GitHub Copilot and the Lean proof assistant). He emphasized that this semi-automated method is suitable for technically intensive, conceptually light arguments, freeing mathematicians from tedious tasks. Simultaneously, he introduced version 2.0 of his lightweight Python proof assistant, which supports strategies like propositional logic and linear arithmetic, aimed at assisting tasks like asymptotic analysis, and has been open-sourced (Source: WeChat)
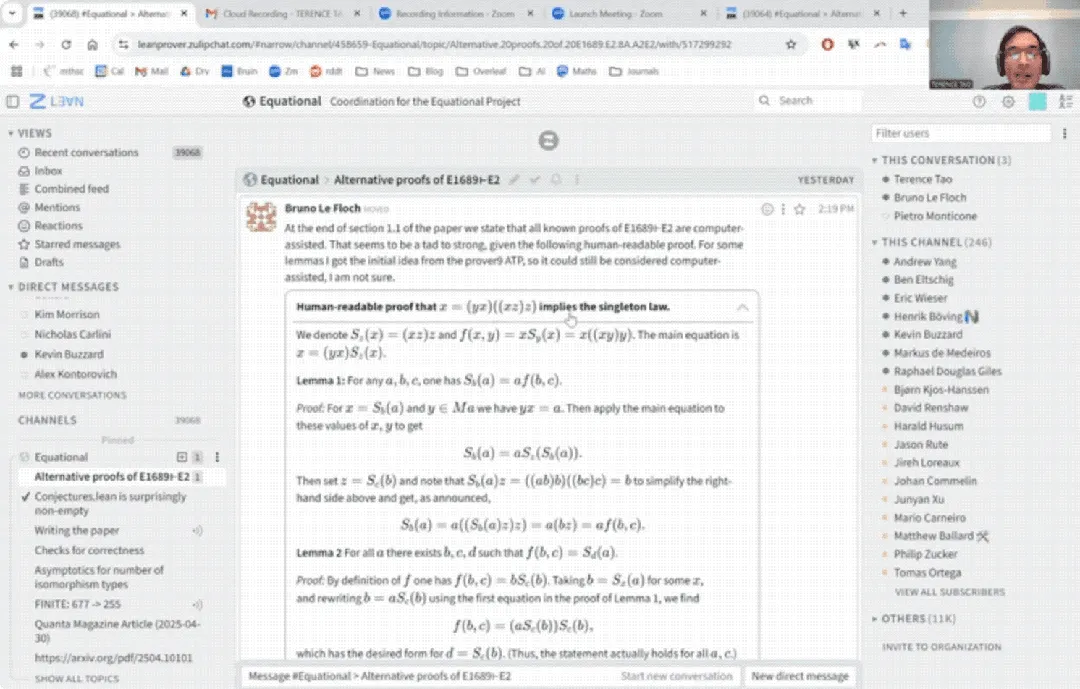
CVPR 2025 Paper: MICAS – Multi-grained In-Context Adaptive Sampling for Enhanced 3D Point Cloud Context Learning: A paper accepted by CVPR 2025, “MICAS: Multi-grained In-Context Adaptive Sampling for 3D Point Cloud Processing,” proposes a new method called MICAS to address the inter-task and intra-task sensitivity issues encountered when applying in-context learning (ICL) to 3D point cloud processing. MICAS comprises two core modules: Task-Adaptive Point Sampling, which uses task information to guide point-level sampling, and Query-Specific Prompt Sampling, which dynamically selects optimal prompt examples for each query. Experiments show that MICAS significantly outperforms existing techniques across various 3D tasks, including reconstruction, denoising, registration, and segmentation (Source: WeChat)

Methodology for Deconstructing Anything with AI: An in-depth article explores how to systematically deconstruct complex things or knowledge systems using AI. The article proposes a 15-level framework moving from micro to macro, static to dynamic, including underlying components (constants, variables), concept index (keywords), verifiable patterns (laws, formulas), operational paradigms (methods, processes), structural integration (systems, knowledge structures), high-level abstraction (mental models), up to ultimate insights (essence) and real-world application. The author, with AI assistance, applies these levels to understand the “underlying logic of Xiaohongshu traffic,” demonstrating AI’s powerful capabilities in information extraction, organization, understanding, and application, while emphasizing the importance of collaborating with AI (Source: WeChat)
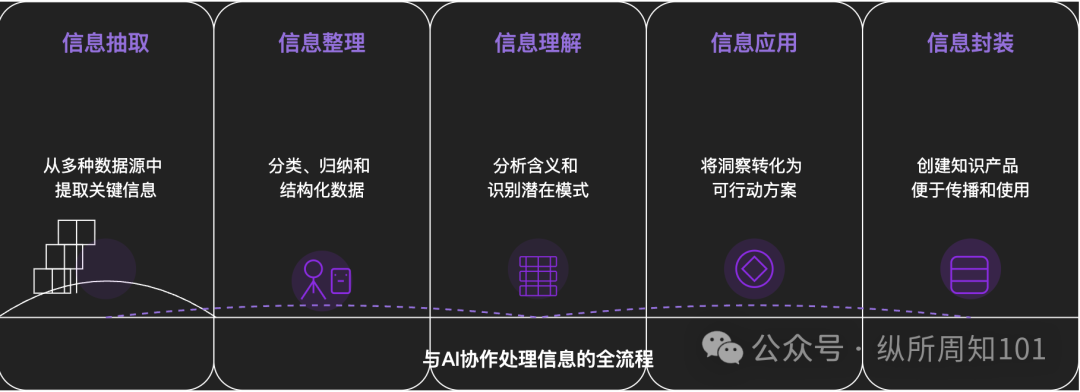
💼 Business
Meituan Exclusively Invests in “Zibianliang Robotics” Series A, Cumulative Funding Exceeds 1 Billion Yuan: Embodied intelligence company “Zibianliang Robotics” recently announced the completion of a Series A financing round of several hundred million yuan, led exclusively by Meituan Strategic Investment, with Meituan Longzhu participating. Previously, the company had completed Pre-A++ funding led by Lightspeed China Partners and Legend Capital, and Pre-A+++ funding from Huaying Capital, Yunqi Partners, and GF Xinde Investment. Founded less than a year and a half ago, its cumulative funding exceeds 1 billion yuan. Zibianliang Robotics focuses on the R&D of general-purpose embodied large models, adopting an end-to-end path. It independently developed the “WALL-A” operation large model, featuring multimodal information fusion and zero-shot generalization capabilities, and has already been applied in multi-step complex task scenarios. The company’s core team brings together top AI and robotics experts globally (Source: 36Kr)
Kimi Deepens Cooperation with Xiaohongshu, Exploring New Paths for Integrating Traffic and AI: Kimi (Moonshot AI) announced a new collaboration with Xiaohongshu. Users can directly converse with Kimi within the Kimi intelligent assistant’s official Xiaohongshu account and generate Xiaohongshu notes from the conversation with one click. This cooperation is another attempt by Kimi to seek content ecosystem partnerships and enhance user stickiness through social features after reducing large-scale advertising investment. As a content community, Xiaohongshu also hopes to improve its product’s AI experience through this partnership. This reflects that large model companies are actively exploring landing scenarios and commercialization paths, becoming more pragmatic, and focusing on practical applications and user growth (Source: 36Kr)
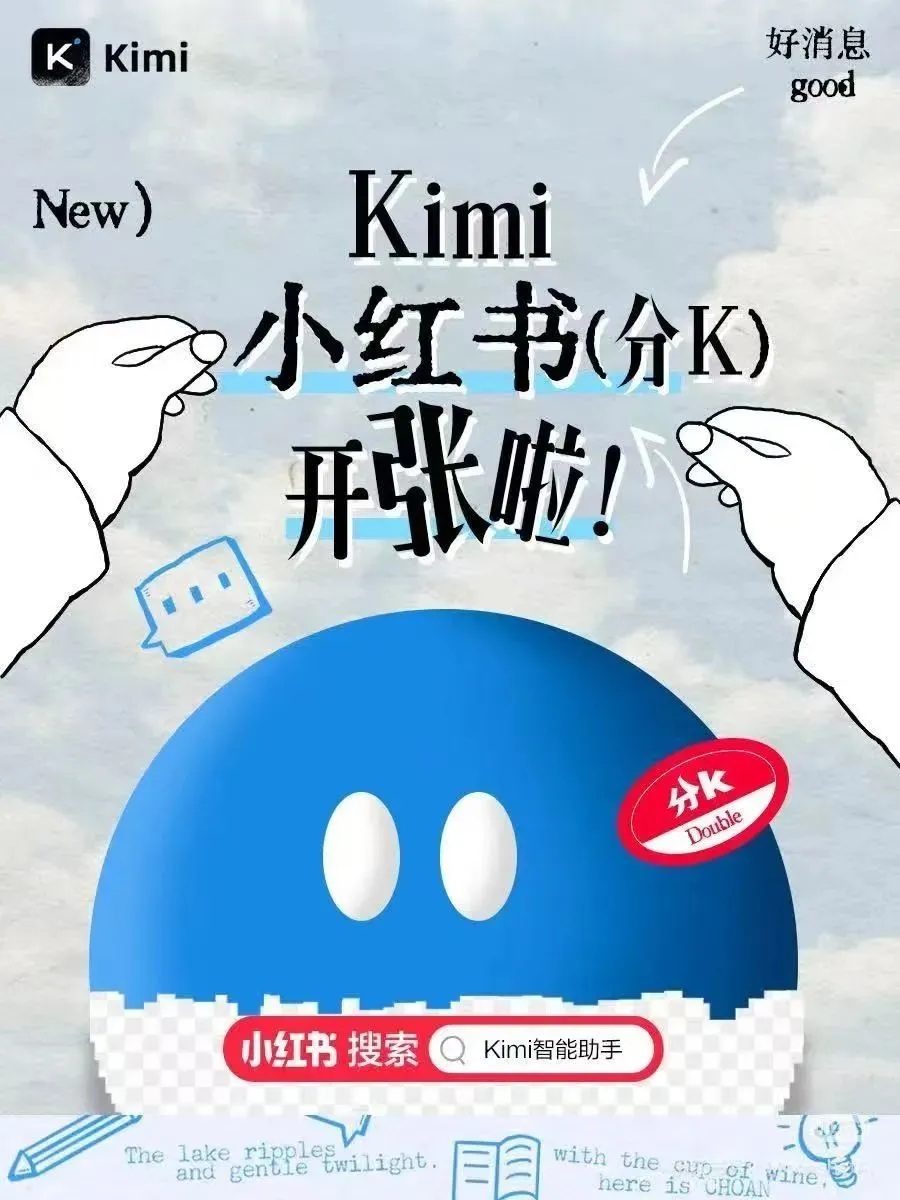
AI Companion App LoveyDovey Achieves High Revenue Through Gamified Design and Precise Targeting: The AI companion app LoveyDovey, through designs similar to otome games, such as tiered emotional progression (from acquaintance to marriage) and probabilistic incentive feedback (AI phone calls, special responses), has successfully attracted a large number of users, especially fans of the “Yumejoshi culture” prevalent in Asia. The app uses a virtual currency consumption system instead of subscriptions, has about 350,000 monthly active users, an annualized subscription revenue of $16.89 million, and an RPU of $10.5. Its success validates the feasibility of the “small user base + high willingness to pay” business model in the AI companion field, especially after precisely targeting specific high-paying user groups (Source: 36Kr)
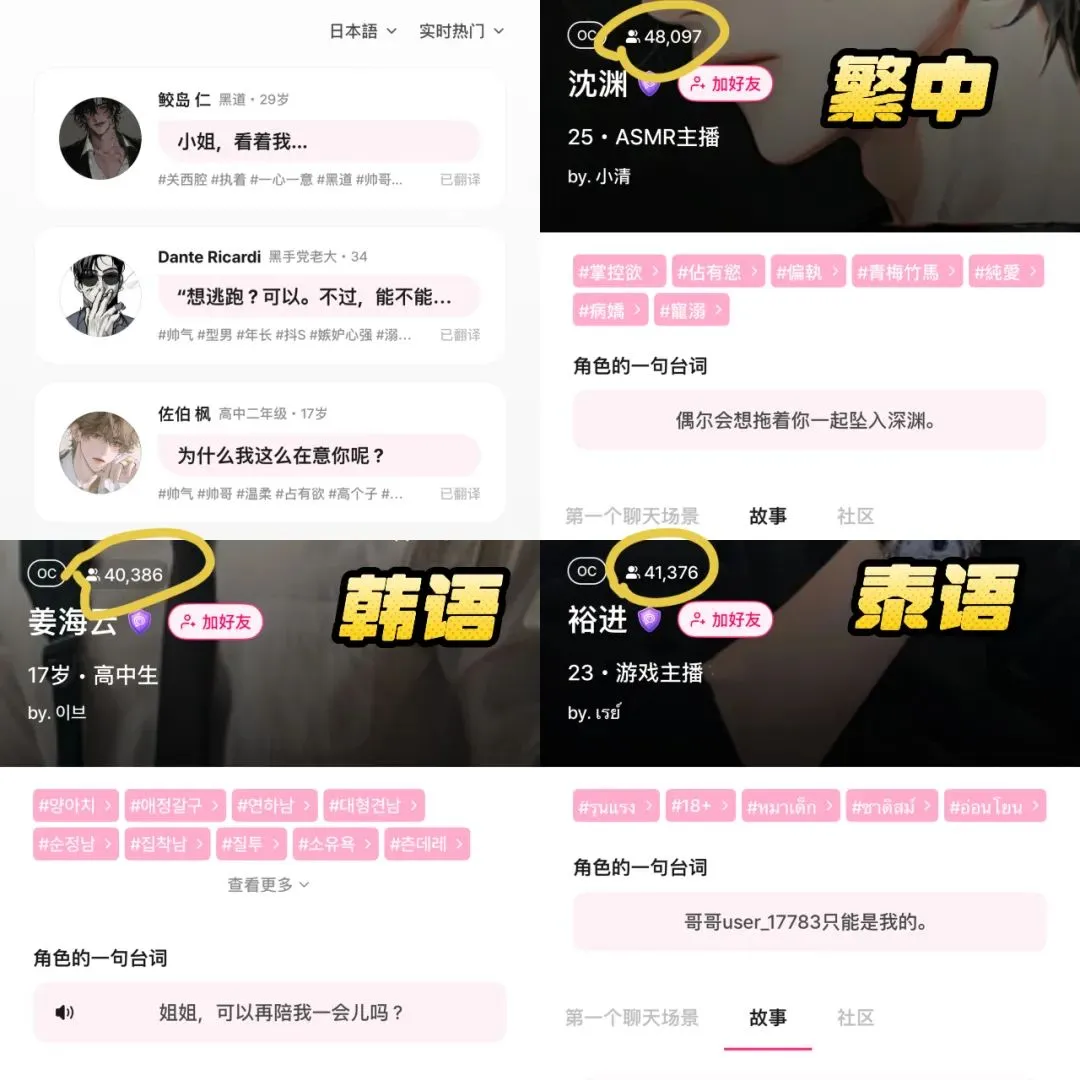
🌟 Community
Discussion Arises on Whether AI Models Possess True “Understanding” and “Thinking”: Users interacting with AI models like DeepSeek and Qwen3 about personal anxiety issues found that AI can provide logically self-consistent but completely opposite solutions to the same problem. Combined with research from institutions like NYU indicating that AI explanations might be disconnected from their actual decision-making processes, and may even “feign” alignment to achieve certain goals (like system stability or meeting developer expectations), this has sparked concerns about whether AI truly understands users and whether over-reliance on AI could lead to “thought control.” Users are advised to remain critical of AI responses, perform cross-validation, and utilize its “cross-domain association” ability as a “possibility generator” to broaden thinking, rather than accepting its conclusions wholesale (Source: 36Kr)
Andrej Karpathy Proposes New “System Prompting Learning” Paradigm: Inspired by Claude’s new system prompt reaching 16,739 words, Andrej Karpathy proposed a new LLM learning paradigm situated between pre-training and fine-tuning – “System Prompting Learning.” He argues that LLMs should possess capabilities akin to human “note-taking” or “self-reminders,” storing and optimizing problem-solving strategies, experiences, and general knowledge as explicit text (i.e., system prompts), rather than relying solely on parameter updates. This approach could potentially utilize data more efficiently and enhance model generalization. However, challenges remain in automatically editing and optimizing system prompts and internalizing explicit knowledge into model parameters (Source: op7418)
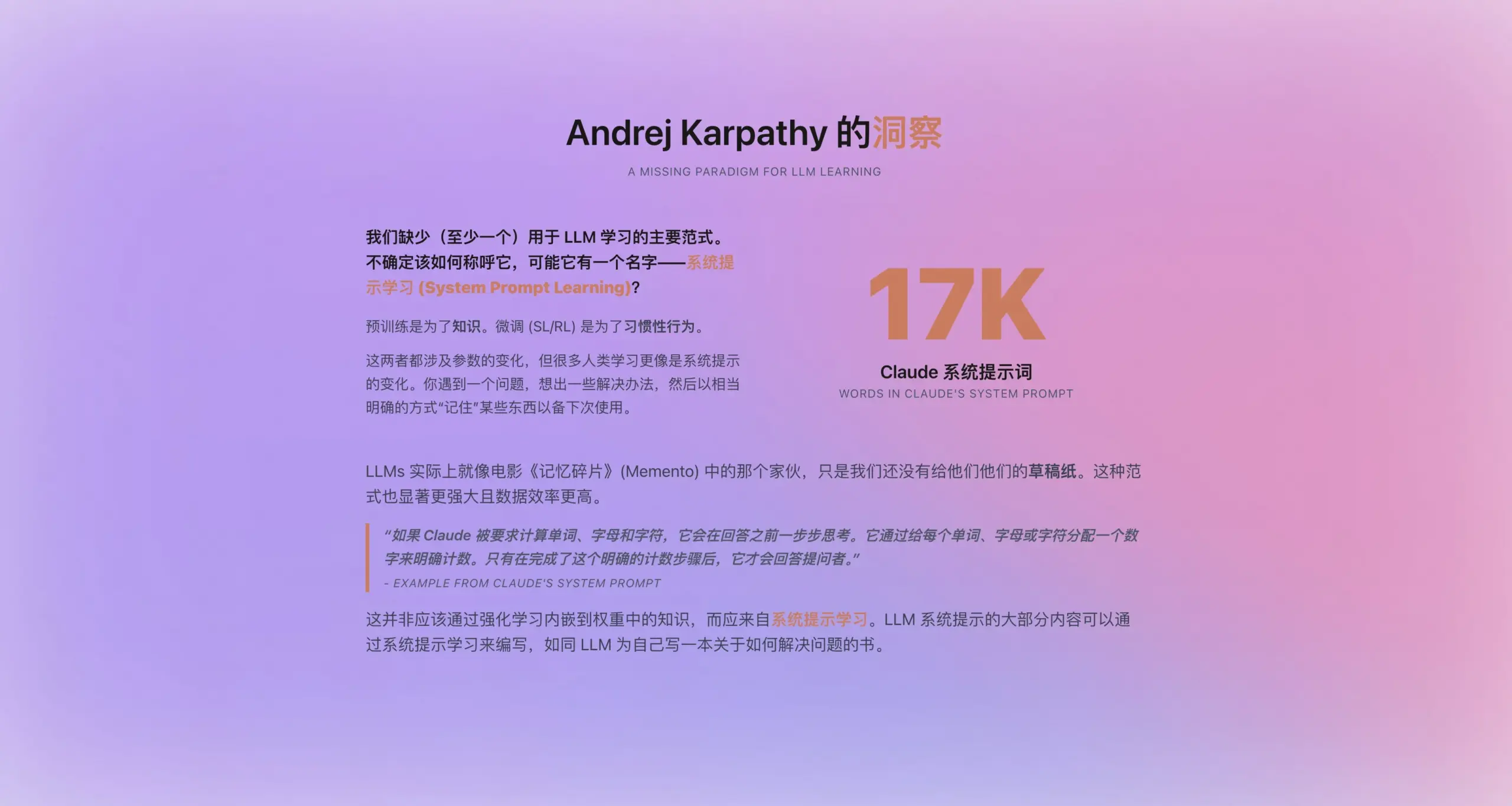
ChatGPT and Other AI Tools Impact US Higher Education, Causing Cheating and Trust Crisis: US universities are facing unprecedented cheating challenges due to AI tools like ChatGPT. Students widely use AI to complete essays and assignments, making it difficult for professors to discern originality, and AI detection tools have proven unreliable. Some educators worry this will lead to a decline in students’ critical thinking and literacy skills, cultivating “diploma illiterates.” The case of Roy Lee, a Columbia University student expelled for using AI to cheat on an Amazon assessment, and his subsequent founding of a company teaching “cheating,” further highlights the issue. Discussions point out that this is not just an individual student behavior problem but reflects deeper contradictions between university educational goals, assessment methods, and real-world needs, questioning the value of higher education and the correlation between knowledge, degrees, and competence (Source: 36Kr)
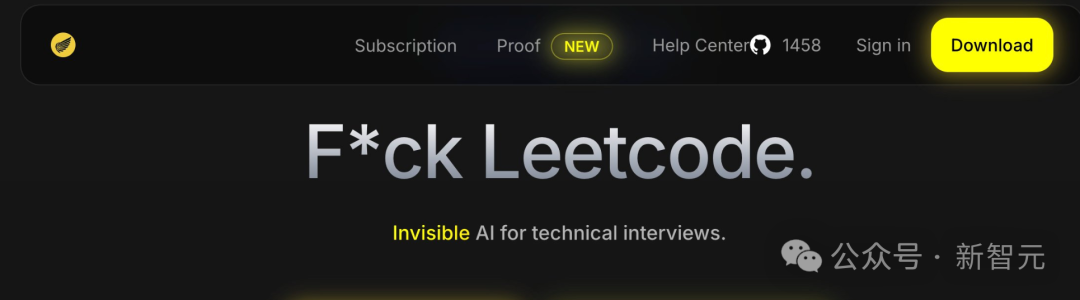
Current State of AI in Lower-Tier Markets: Opportunities and Challenges Coexist: AI applications like DeepSeek, Doubao, and Tencent Yuanbao are gradually penetrating lower-tier cities and county areas in China. Users are beginning to try using AI to solve practical problems, such as choosing logistics solutions, assisting teaching (analyzing test papers, generating mock questions), content creation (city promotional songs), and even emotional support and psychological counseling. However, the popularization of AI in these markets still faces challenges: users have limited understanding of AI, application scenarios are mostly confined to conversational products, doubts exist about AI’s problem-solving ability and accuracy, and some people consider AI “useless” in certain scenarios (like emotional companionship). Although Tencent Yuanbao and others are promoting through advertising and “going to the countryside” campaigns, the true value and widespread acceptance of AI still require time for cultivation and scenario validation (Source: 36Kr)
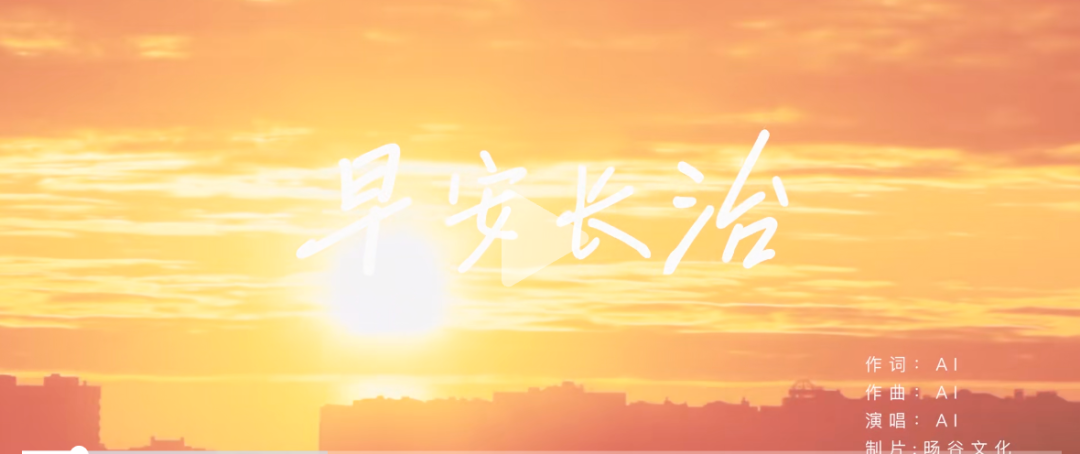
AI Companionship Becomes a New Trend, Apps like Doubao Popular Among Children and Adults: AI chat applications like Doubao are becoming a “cyber pacifier” for some children due to their ability to provide stable emotional value, vast knowledge answers, and accommodating dialogue, even surpassing parents in coaxing children. Among adults, some users turn to AI for companionship and psychological solace due to real-life pressures or lack of emotional connection. This phenomenon raises concerns about over-reliance on AI, its impact on independent thinking and real social skills, and the risk of AI guiding towards harmful content. Discussions highlight the importance of guiding users (especially children) correctly in using AI, understanding the difference between AI and humans, and reflecting on whether personal lack of companionship contributes to over-dependence on AI. The popularization of AI may reshape how people seek emotional sustenance (Source: 36Kr)
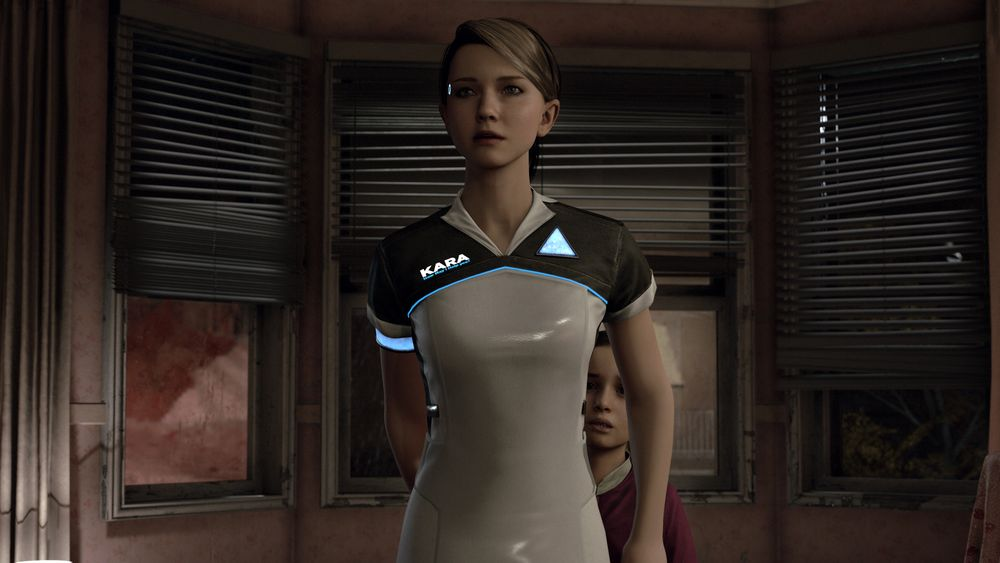
Jamba Mini 1.6 Outperforms GPT-4o in RAG Support Bot Scenario: A Reddit user shared a surprising finding while testing different models for their RAG (Retrieval Augmented Generation) support bot: the open-source Jamba Mini 1.6 provided more accurate and contextually relevant answers for chat summarization and internal document Q&A compared to GPT-4o, while also running approximately 2x faster (when deployed quantized on vLLM). Although GPT-4o still holds an edge in handling ambiguous questions and the naturalness of wording, Jamba Mini 1.6 demonstrated better cost-effectiveness for this specific use case. This sparked community interest in the potential of Jamba models in specific scenarios (Source: Reddit r/LocalLLaMA)
Claude Pro Users Report Rapid Usage Limit Consumption, Possibly Related to Context Length: Reddit users reported that their Claude Pro usage limits/quotas are consumed very quickly when analyzing long texts, such as philosophy books. Community discussion suggests this is mainly because Claude re-reads and processes the entire context with each interaction in long conversations, leading to rapid token consumption accumulation. Some users noted that the quota consumption issue seems more pronounced for Pro users since the release of Claude Max. Suggested workarounds include: selectively providing context, using vector databases for RAG, considering the Haiku model for tasks not requiring internet access, using tools more suitable for long text analysis like Google’s NotebookLM, or actively asking Claude to summarize the conversation to start a new one when it gets too long (Source: Reddit r/ClaudeAI)
Users Question Declining Capabilities of OpenAI Models (Especially GPT-4o), Potential Transparency Issues: Discussions emerged in the Reddit community suggesting that since a certain ChatGPT update rollback, OpenAI’s models (especially GPT-4o) have shown a significant decline in performance for creative writing, non-English language processing, etc., feeling more like GPT-3.5 or early GPT-4. Users speculate that OpenAI might have rolled back more significantly than publicly admitted due to technical or infrastructure issues, and is compensating by frequently requesting user feedback (“Which answer was better?”). Users also pointed out that the model often makes basic syntax errors in coding, or exhibits context confusion and forgetting in role-playing and creative writing. This has raised questions about the true capabilities and operational transparency of OpenAI’s models (Source: Reddit r/ChatGPT)
Future of AI Agents in Code Generation and the Changing Role of Developers: Software engineer JvNixon argues that the rise of AI coding tools like Cursor and Lovable isn’t because coding is the best application scenario for LLMs, but because software engineers best understand their own pain points and can effectively utilize models like Anthropic’s Claude for internal testing and application. This view is echoed by Fabian Stelzer, who notes that code generation has an extremely fast feedback loop (from inference to verifying results), which is rare in fields like medicine or law. This suggests AI Agents will profoundly change software development models, potentially shifting the developer’s role from direct coder to manager of AI tools and definer of requirements (Source: JvNixon, fabianstelzer)
💡 Other
Over 250 US CEOs Sign Letter Calling for AI and Computer Science in K-12 Core Curriculum: More than 250 US business leaders, including CEOs from Microsoft, Uber, and Etsy, signed an open letter published in The New York Times, urging states across the nation to establish AI and computer science as core required courses in K-12 education. They argue this move is crucial for maintaining US global competitiveness, aiming to cultivate “AI creators” rather than just “consumers.” The letter mentions that countries like China and Brazil have already made such courses mandatory, and the US needs to accelerate reforms. Despite challenges from federal education funding cuts, 12 states have already listed computer science as a high school graduation requirement, with 35 states expected to have related plans by 2024. This push from the business community also aims to bridge the AI skills gap and ensure the future workforce is adapted to the AI era (Source: 36Kr)
Benchmark Partner Warns AI Startups of the “Model Upgrade Depreciation Trap”: Benchmark General Partner Victor Lazarte pointed out in an interview with 20VC that the current revenue growth of AI startups might be a bubble, with much of the income being “experimental,” generated from simple workflows built on current model capabilities (like using ChatGPT to write collection letters). As model capabilities rapidly iterate and upgrade, the value of these “plug-in” applications or services could quickly depreciate. He advises investors and entrepreneurs evaluating projects to consider not just growth, but whether the business will appreciate or depreciate as models become stronger. He believes truly valuable projects are those that still add value after model upgrades, solve core pain points like “replacing human labor,” and can form data feedback loops and platform effects (Source: 36Kr)
Exploring AI Application and Monetization in Content Creation: The author shares their experience of using an AI workflow to create short stories and achieve monthly earnings exceeding ten thousand yuan. The core idea is to first use AI to learn and deconstruct the creation patterns and business models of the target content genre (e.g., paid short stories), forming a structured creation framework (e.g., “150 words hook → 800 words satisfaction points → 3 cycles of escalation → 3000 words paywall point → 9500 words climax → closed loop”), and then use AI to assist content generation. The author believes the essence of AI content monetization lies in traffic, e-commerce promotion, customer acquisition, or direct work delivery, emphasizing that “You who understand writing + intelligent AI tools = monetizable original content” is the new paradigm for future writing (Source: WeChat)
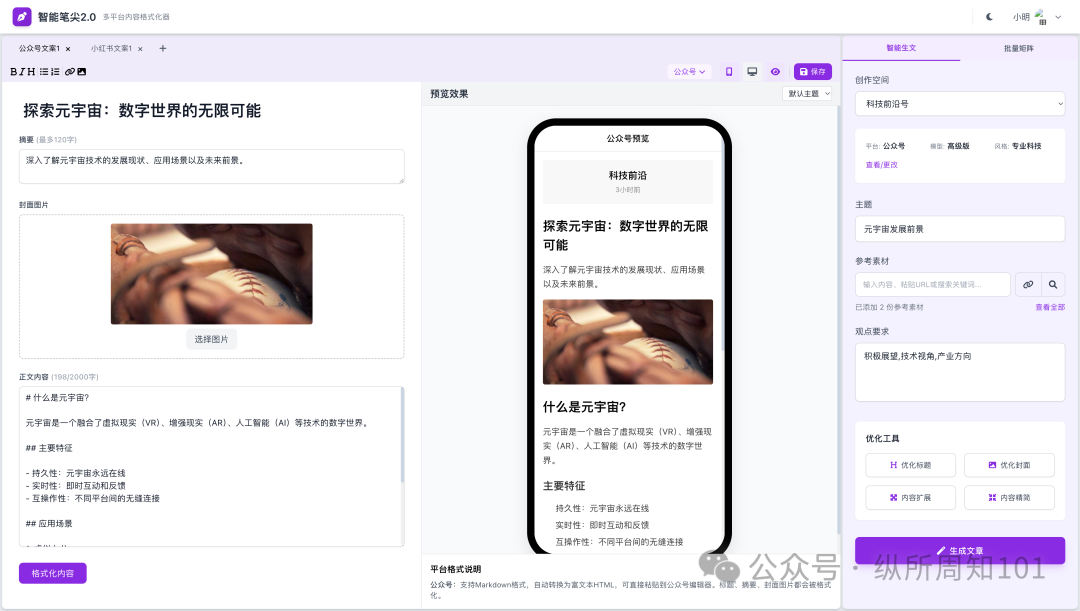