Keywords:GENMO, Seed-Coder, DeepSeek, LlamaParse, Agentic AI, Edge Computing, Quantum Computing, NVIDIA GENMO human motion model, ByteDance Seed-Coder code large language model, DeepSeek open-source strategy impact, LlamaParse document parsing confidence scoring, Edge computing real-time data processing
🔥 Focus
NVIDIA Introduces GENMO Universal Human Motion Model: NVIDIA has released an AI model named GENMO (GENeralist Model for Human MOtion), capable of transforming various inputs such as text, video, music, and even keyframe silhouettes into realistic 3D human motion. The model can understand and fuse different types of input, for example, learning actions from video and modifying them based on text prompts, or generating dances according to musical rhythms. GENMO demonstrates significant potential in areas like game animation and virtual world character creation, capable of generating complex and natural coherent movements, and supports intuitive editing of animation timing. Although it currently cannot handle facial expressions and hand details, and relies on external SLAM methods, its multimodal input and high-quality output represent important progress in the field of AI motion generation (Source: YouTube – Two Minute Papers
)
ByteDance Releases Seed-Coder Series of Open-Source Large Models: ByteDance has launched the Seed-Coder series of open-source large language models, including an 8B parameter scale base model, instruction model, and inference model. The core feature of this series is its “code model self-curating data” capability, aiming to minimize human involvement in data construction. Seed-Coder has achieved current state-of-the-art (SOTA) levels in various aspects such as code generation and editing, demonstrating the potential of optimizing and constructing training data through AI’s own capabilities, providing new ideas for the development of large code models (Source: _akhaliq)
DeepSeek Models Spark Widespread Attention in the AI Community: The DeepSeek series of models, particularly its code models, have sparked extensive discussion in the AI community due to their powerful performance and open-source strategy. Many developers and researchers are impressed by their performance, believing they have changed the global perception of open-source models. Discussions indicate that DeepSeek’s success may prompt companies like OpenAI to re-evaluate their open-source strategies and encourage local large model vendors to accelerate their open-sourcing pace. Although open-sourcing faces challenges such as commercialization and hardware adaptation, DeepSeek’s emergence is seen as an important force driving the democratization of AI technology and industry development (Source: Ronald_vanLoon, 36Kr)
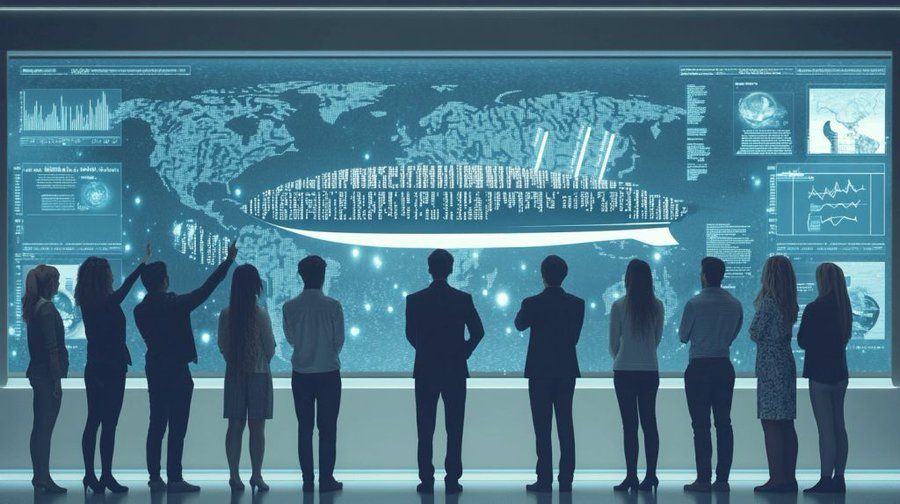
LlamaParse Update: Integrates GPT-4.1 & Gemini 2.5 Pro, Enhancing Document Parsing Capabilities: LlamaParse has released a significant update, integrating the latest GPT-4.1 and Gemini 2.5 Pro models, markedly improving document parsing accuracy. New features include automatic orientation and skew detection, ensuring the alignment and accuracy of parsed content. Additionally, a confidence scoring feature has been introduced, allowing users to assess the parsing quality of each page and set up manual review processes based on confidence thresholds. This update aims to address errors that LLMs/LVMs may encounter when processing complex documents, ensuring the reliability of automated processes by providing a user experience for manual review and correction (Source: jerryjliu0)
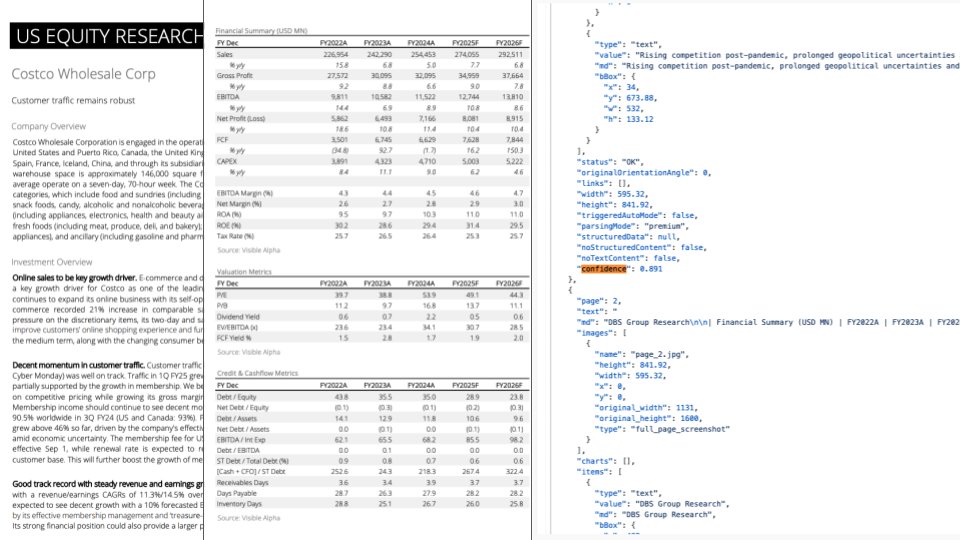
🎯 Trends
2025 Tech Industry Trends Outlook: A report predicts the main trends in the tech industry for 2025, including the continued development and deep integration of emerging technologies such as artificial intelligence, machine learning, 5G, wearable devices, blockchain, and cybersecurity. These technologies are expected to play an important role in improving lives, driving innovation, and solving societal problems, heralding a bright future empowered by technology (Source: Ronald_vanLoon, Ronald_vanLoon)
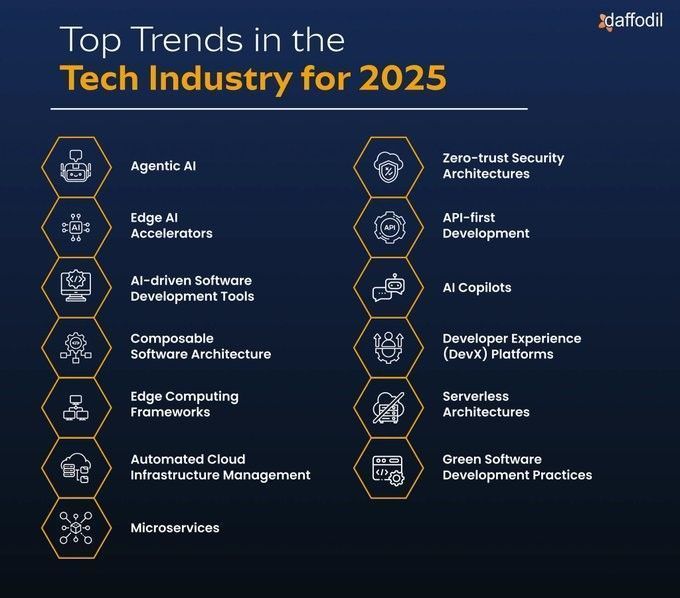
2025 AI Development Trends Prediction: IBM predicts that the field of artificial intelligence will continue its rapid development in 2025, with machine learning (ML) and artificial intelligence (MI) technologies further maturing and being widely applied across various industries. AI is expected to play a greater role in automation, data analysis, decision support, and other areas, driving technological innovation and industrial upgrading (Source: Ronald_vanLoon)
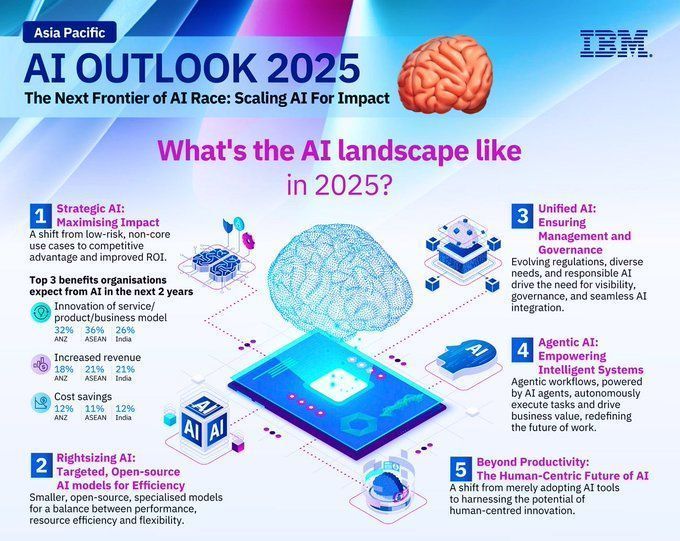
OLMo 32B Model Demonstrates Outstanding Performance: In relevant benchmark tests, the fully open OLMo 32B model outperformed the larger parameter Nemotron 340B and Llama 3 70B models. This result indicates that, in some aspects, smaller-parameter fully open models can achieve or even surpass larger commercial models, showcasing the immense potential and rapid catch-up speed of open model research (Source: natolambert, teortaxesTex, lmarena_ai)
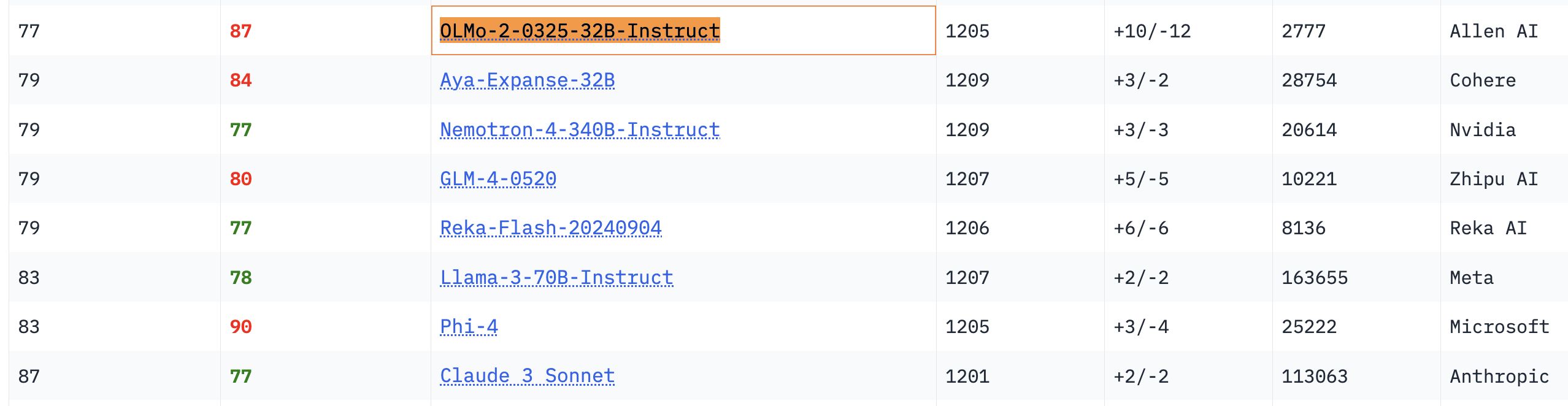
Gemma Model Downloads Exceed 150 Million, with Over 70,000 Variants: Google’s Gemma model has surpassed 150 million downloads on the Hugging Face platform and boasts over 70,000 variants. This data reflects the Gemma model’s popularity and widespread application within the developer community. Community users are also full of anticipation for iterations of its future versions (Source: osanseviero, _akhaliq)
Unsloth Updates Qwen3 GGUF Models, Improves Calibration Dataset: Unsloth has updated all its Qwen3 GGUF models and adopted a new, improved calibration dataset. Additionally, more GGUF variants have been added for Qwen3-30B-A3B. User feedback indicates that in the 30B-A3B-UD-Q5_K_XL version, translation quality has improved compared to other Q5 and Q4 GGUFs (Source: Reddit r/LocalLLaMA)
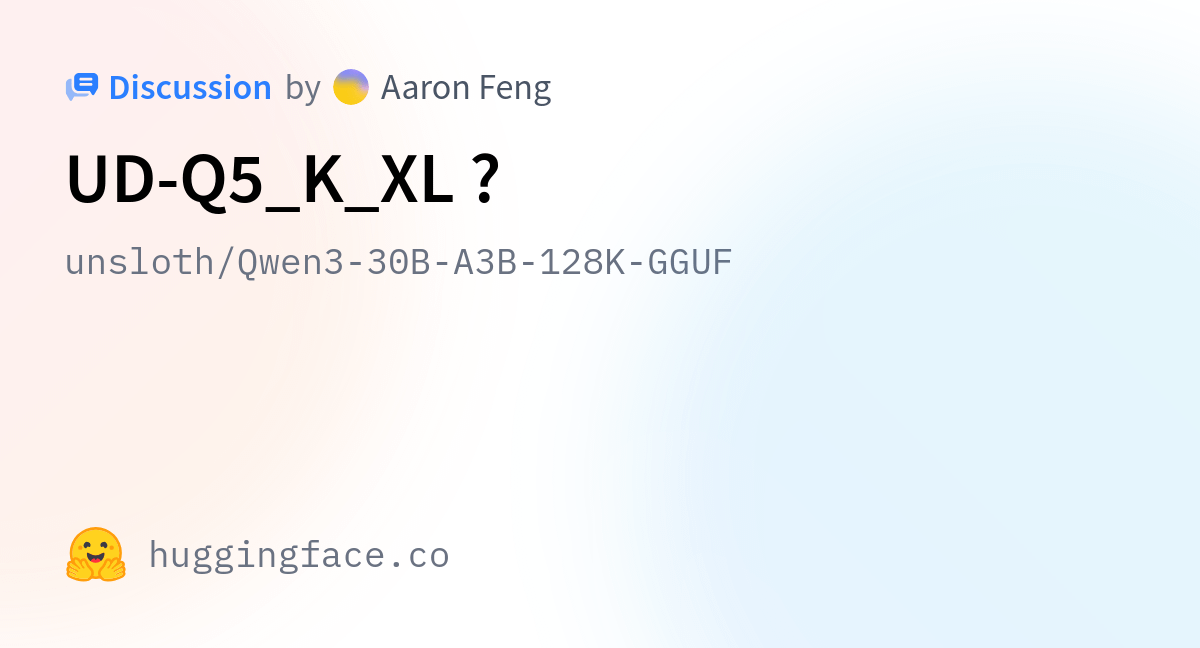
Difference Between Agentic AI and GenAI: Agentic AI and Generative AI (GenAI) are current hot topics in the AI field. GenAI primarily refers to AI that can create new content (text, images, etc.), while Agentic AI focuses more on intelligent agents capable of autonomously performing tasks, interacting with the environment, and making decisions. Agentic AI often incorporates GenAI capabilities but places greater emphasis on its autonomy and goal-orientation (Source: Ronald_vanLoon)
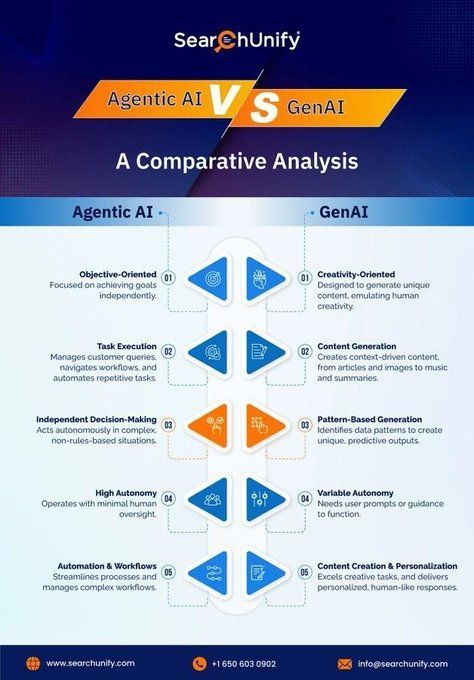
Emotional AI Enhances Customer Experience: Emotional AI technology, by analyzing and understanding human emotions, is being applied to enhance customer experience (CX). It can help businesses better understand customer needs and emotions, thereby providing more personalized and empathetic services, driving innovation in customer relationship management during digital transformation (Source: Ronald_vanLoon)
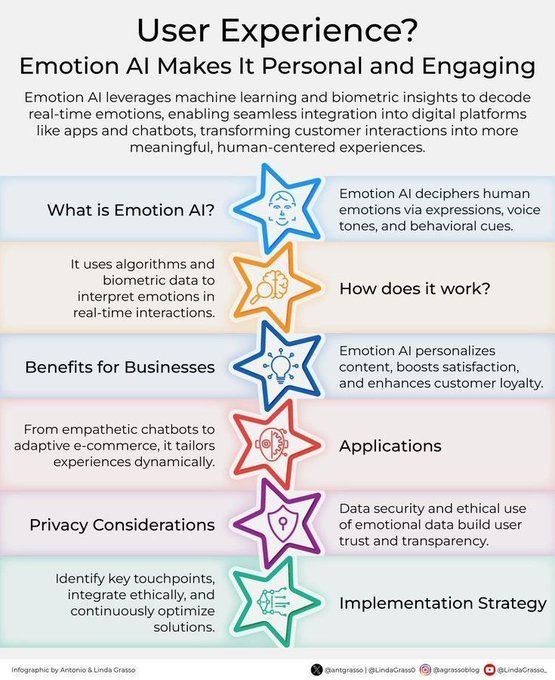
Concept of AI-Driven Personalized Tool “Jigging” (Intelligent Mechanics Auxiliary Device): Karina Nguyen proposes the “Jigging” concept, likening AI models to individualized, self-improving toolmakers. Each time an AI interacts with a user, it crafts new specialized tools based on the user’s characteristics and tasks, thereby enhancing its capabilities. For example, an AI could build a personalized diagnostic framework for a doctor or a unique narrative framework for a writer. This recursive improvement will make AI an extension of the user’s cognitive architecture, driving a fundamental shift in human-computer collaboration (Source: karinanguyen_)
Difference Between AI Agents and Agentic AI: Khulood Almani further elaborates on the distinction between AI Agents and Agentic AI. AI Agents typically refer to software programs that perform specific tasks, whereas Agentic AI emphasizes the system’s autonomy, learning ability, and adaptability, enabling it to more proactively interact with the environment and achieve complex goals. Understanding this distinction helps grasp the direction and potential of AI development (Source: Ronald_vanLoon)
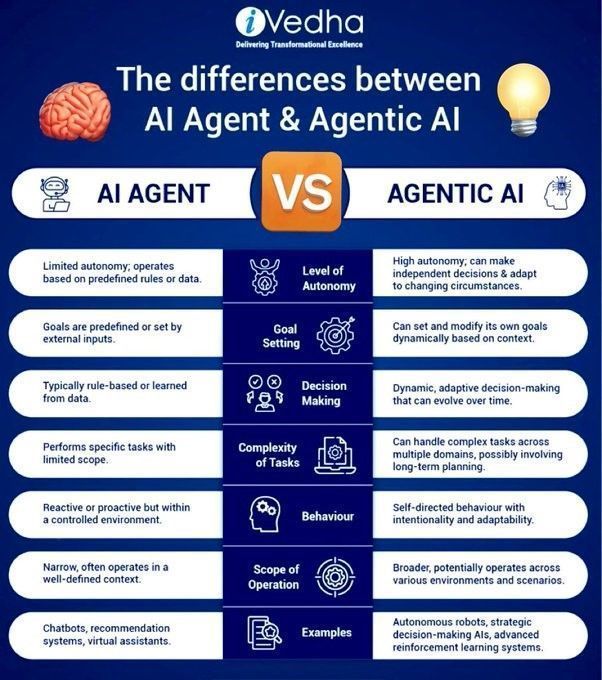
Edge Computing Processes Data Closer to the Source: Edge computing technology processes data near its source, reducing latency, lowering bandwidth requirements, and enhancing privacy. This is crucial for AI applications requiring real-time responses and processing large amounts of data (such as autonomous driving, industrial IoT), and is an important component of cloud computing and digital transformation (Source: Ronald_vanLoon)
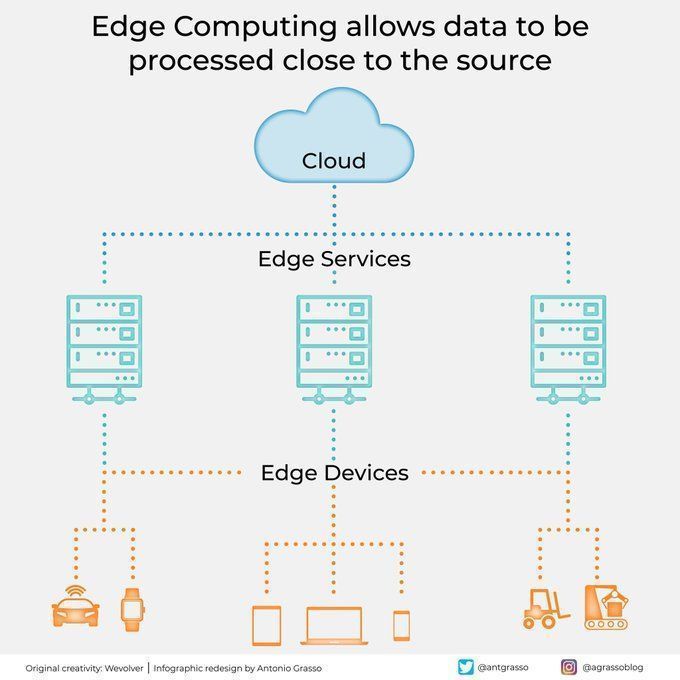
Key Skills for an AI Architect: Becoming a successful AI architect requires a diverse set of skills, including a strong technical foundation (machine learning, deep learning algorithms), system design capabilities, data management knowledge, and an understanding of business requirements. Additionally, communication and collaboration skills, as well as a passion for continuous learning of new technologies, are crucial (Source: Ronald_vanLoon)
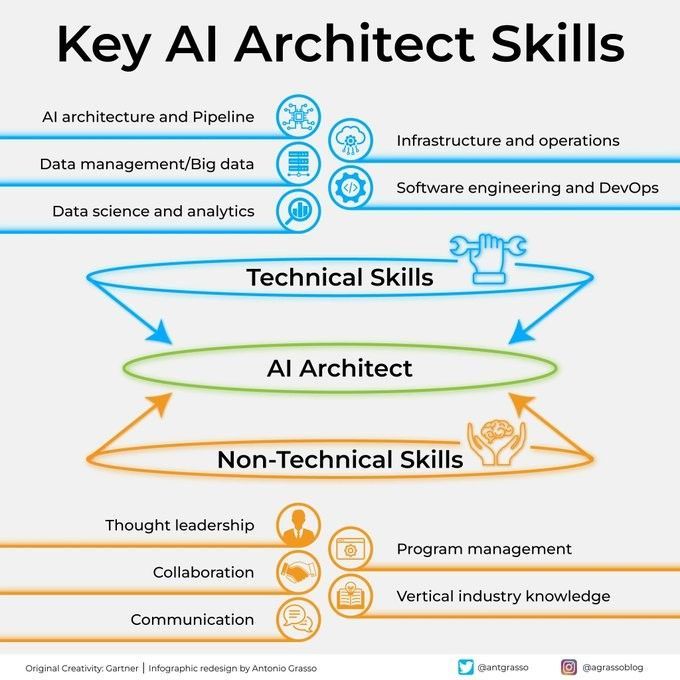
Step-by-Step Guide to Integrating AI into Strategic Execution: Khulood Almani provides a step-by-step guide to help businesses integrate artificial intelligence into their strategic execution processes. This includes clarifying AI objectives, assessing existing capabilities, selecting appropriate AI technologies, developing an implementation roadmap, and establishing monitoring and evaluation mechanisms to ensure AI projects align with overall business strategy and deliver expected value (Source: Ronald_vanLoon)
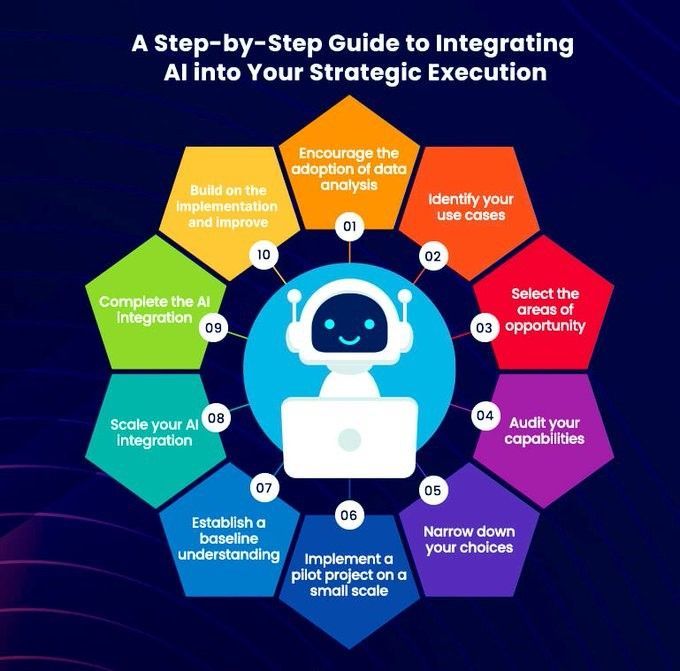
How Quantum Computing is Changing Cybersecurity: The advent of quantum computing has a dual impact on cybersecurity. On one hand, its powerful computational capabilities could break existing encryption algorithms, posing security threats; on the other hand, quantum technology has also given rise to new security measures like quantum cryptography. Khulood Almani discusses the transformative role of quantum computing in cybersecurity, emphasizing the importance of preparing for the post-quantum era (Source: Ronald_vanLoon)
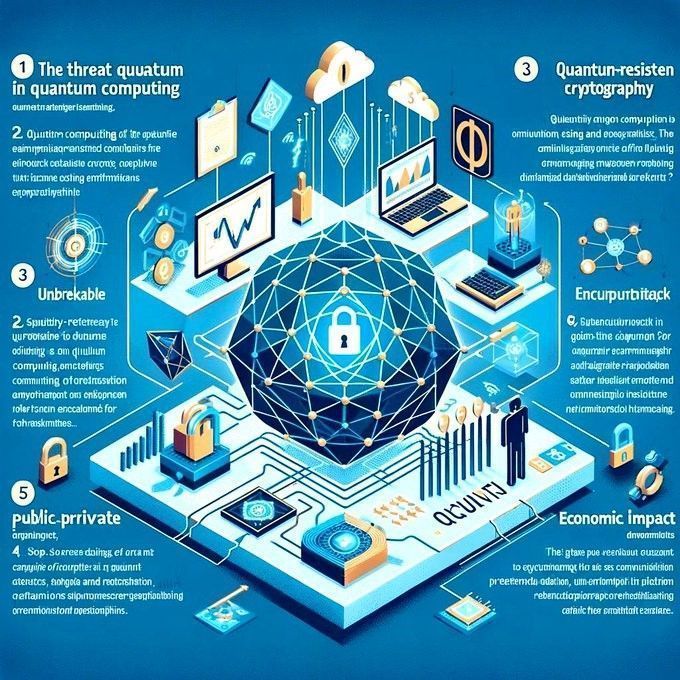
Tools Dominating the AI Field in 2025: Perplexity predicts the key tools that will dominate the field of artificial intelligence in 2025, potentially including more advanced large language models (LLMs), generative AI platforms, data science tools, and AI solutions specifically tailored for particular industry applications. These tools will further promote the popularization and deepening application of AI across various industries (Source: Ronald_vanLoon)
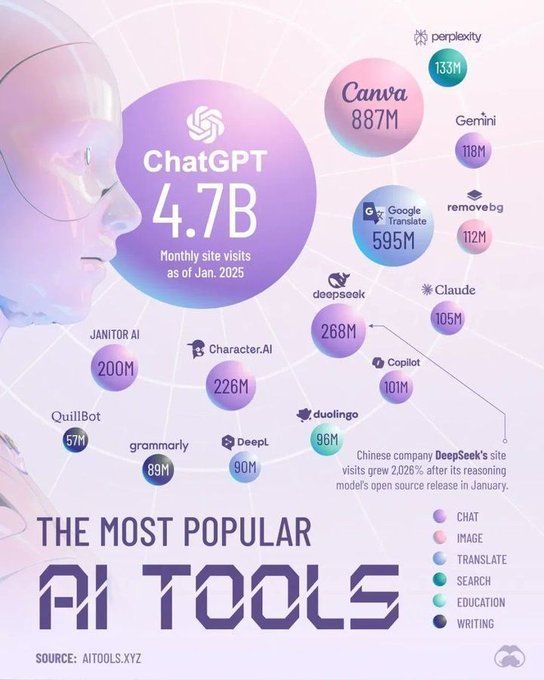
Summary of Core Machine Learning Concepts: Python_Dv summarizes the core concepts of machine learning, potentially covering fundamental principles of supervised learning, unsupervised learning, reinforcement learning, deep learning, common algorithms, and their application scenarios. This provides a concise overview for beginners and those wishing to consolidate their foundational knowledge (Source: Ronald_vanLoon)
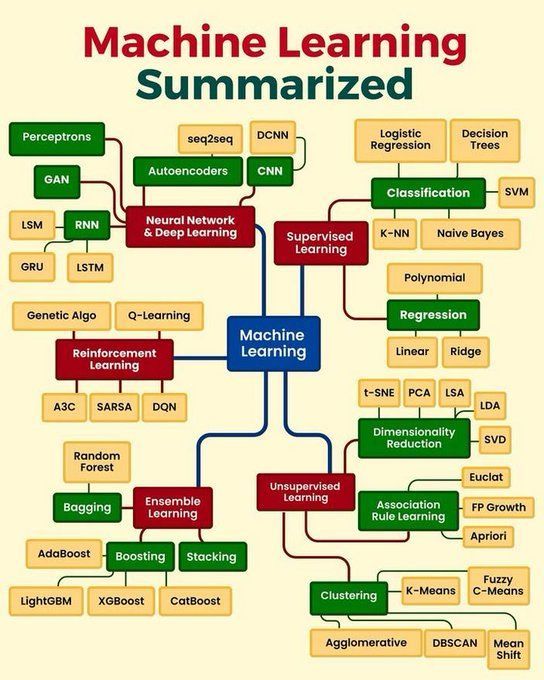
🧰 Tools
ByteDance Launches Deep Research Framework DeerFlow: ByteDance has open-sourced DeerFlow, a framework for systematic deep research by coordinating LangGraph agents. It supports comprehensive literature analysis, data synthesis, and structured knowledge discovery, aiming to enhance the efficiency and depth of AI applications in scientific research (Source: LangChainAI, Hacubu)
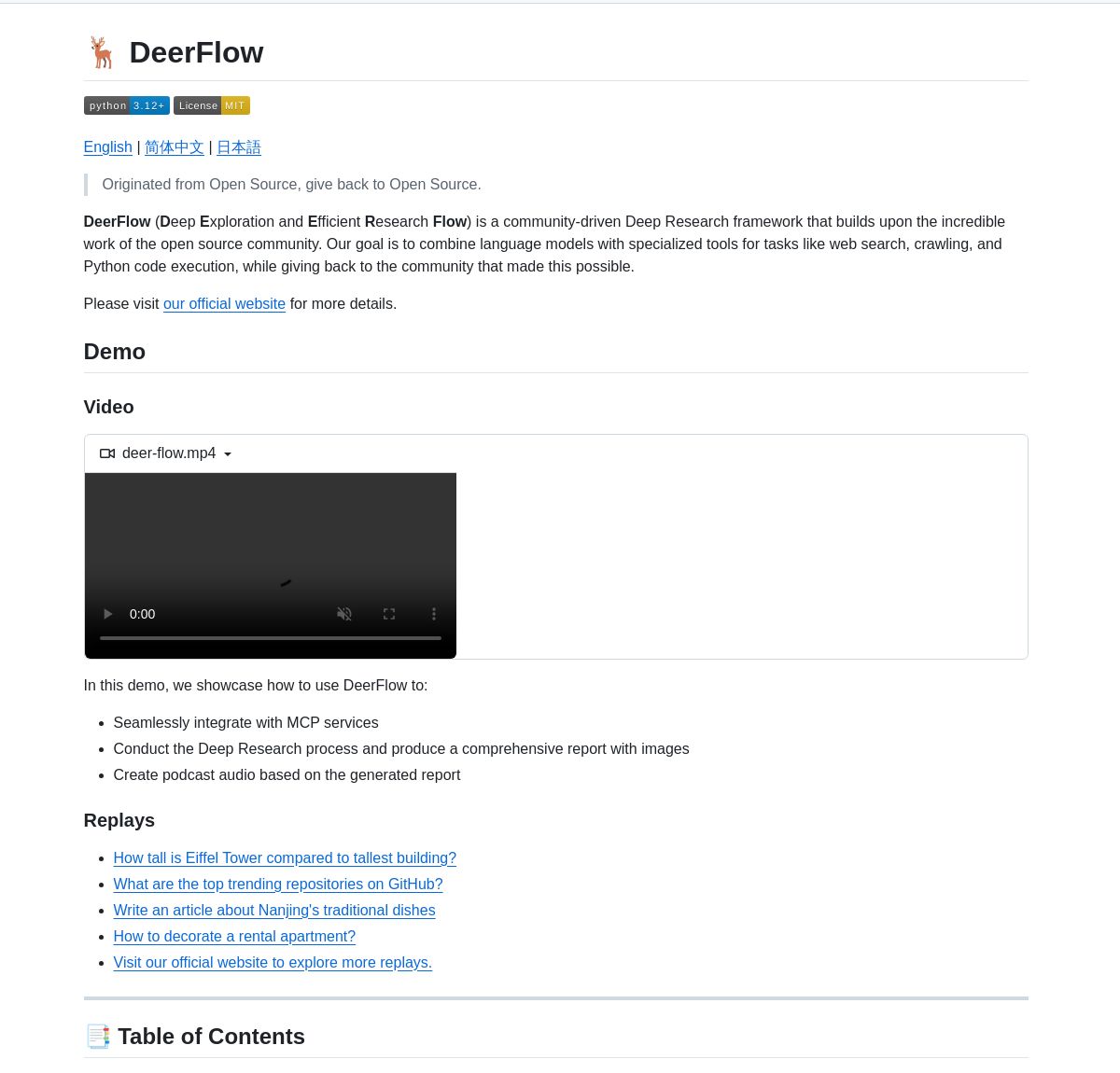
LangGraph-Powered Multi-Agent System for Company Research: A multi-agent system based on LangGraph has been developed to generate real-time company research reports. The system utilizes intelligent processes with specialized nodes to analyze business, financial, and market data, providing users with in-depth company insights. A demo and code are available on GitHub (Source: LangChainAI, Hacubu)
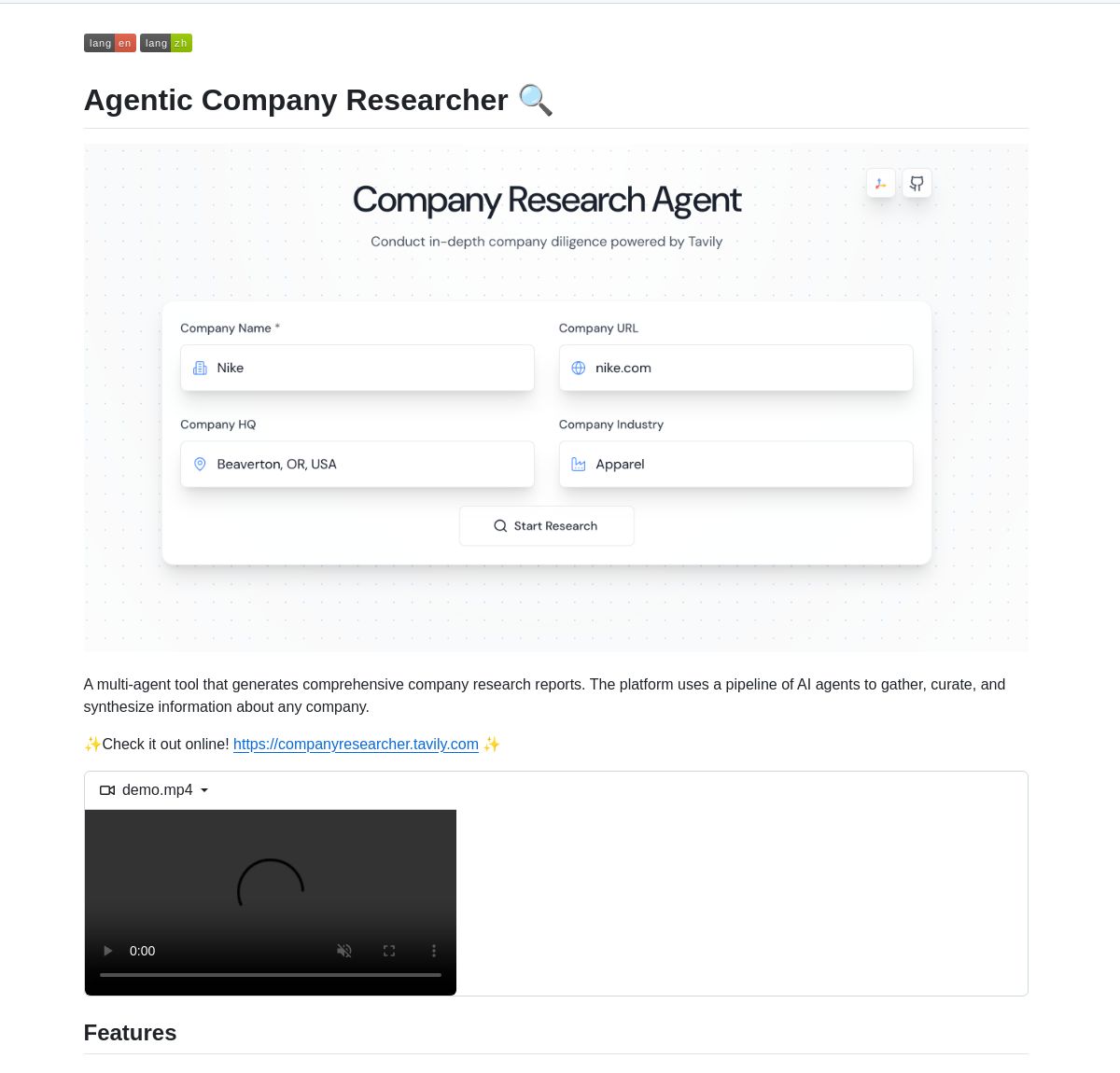
RunwayML Gen-4 References Achieves Precise Character/Object Positioning: RunwayML’s Gen-4 References feature has been found to enable precise control over the position of characters or objects in generated content. Users can guide the AI to place specific elements in desired precise locations by providing a scene and a reference image with markers (such as simple colored shapes indicating position), offering new possibilities for creative workflows. As a general-purpose model, it can adapt to multiple workflows without fine-tuning (Source: c_valenzuelab, c_valenzuelab)
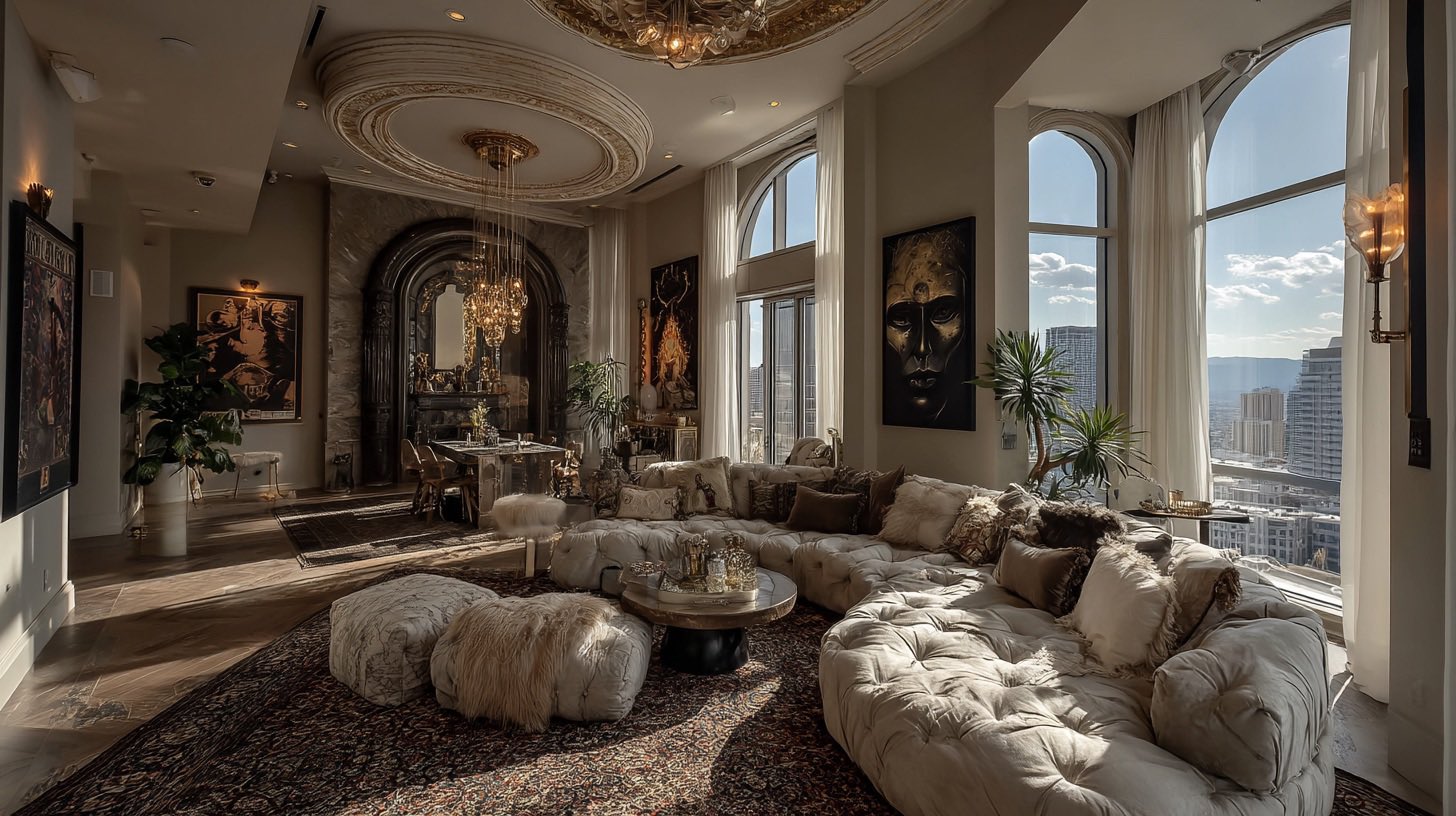
Code Chrono: A Tool for Estimating Programming Project Time with Local LLMs: Rafael Viana has developed a terminal tool called Code Chrono for tracking coding session duration and utilizing local LLMs to estimate development time for future features. The tool aims to help developers more realistically assess project time and avoid underestimating workload. The project code is open-source (Source: Reddit r/LocalLLaMA)
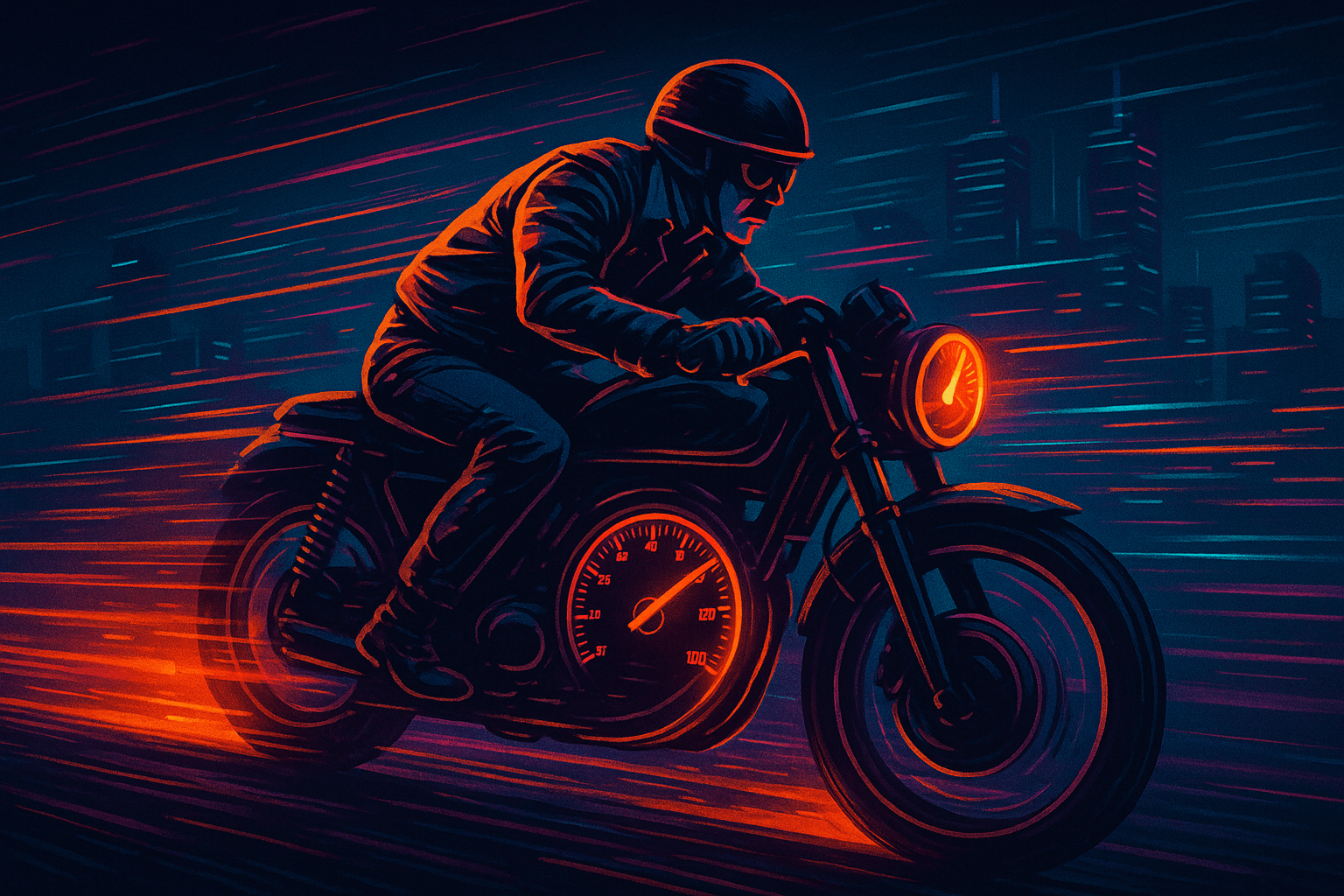
Progress in PyTorch Integration with Mojo Language: Mark Saroufim, at the Mojo Hackathon, introduced how PyTorch simplifies support for emerging languages and hardware backends, and showcased a WIP backend developed in collaboration with the Mojo team. Chris Lattner praised this collaboration, believing that the combination of Mojo and PyTorch will inject new vitality into the PyTorch ecosystem and drive innovation in AI development tools (Source: clattner_llvm, marksaroufim)
Trump-Style Chatbot: A developer has trained and launched a chatbot mimicking Trump’s style, based on real historical events from the Oval Office. The chatbot is available for interaction on Hugging Face Spaces, and the developer is seeking user feedback and suggestions (Source: Reddit r/artificial)
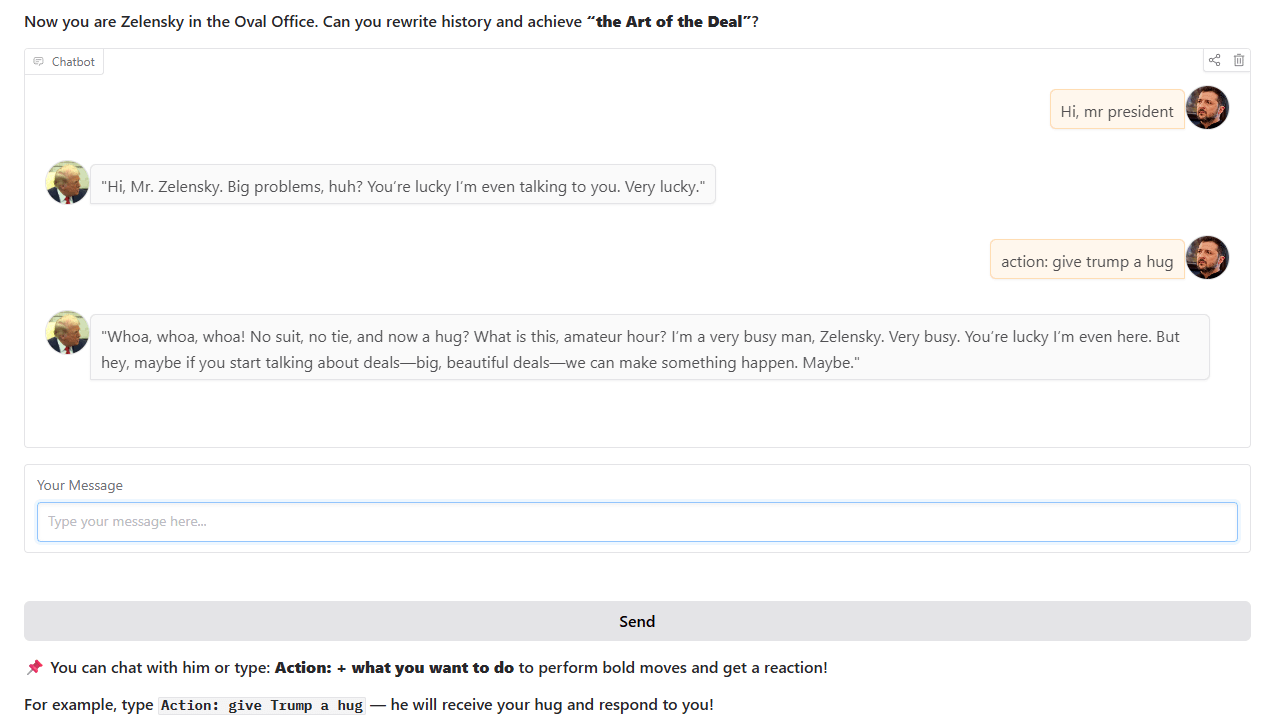
Open-Source Agentic Network Building Tool: An open-source tool called python-a2a simplifies the process of building Agentic Networks, supporting drag-and-drop operations. Users can try this tool to create and manage AI agent networks (Source: Reddit r/ClaudeAI)
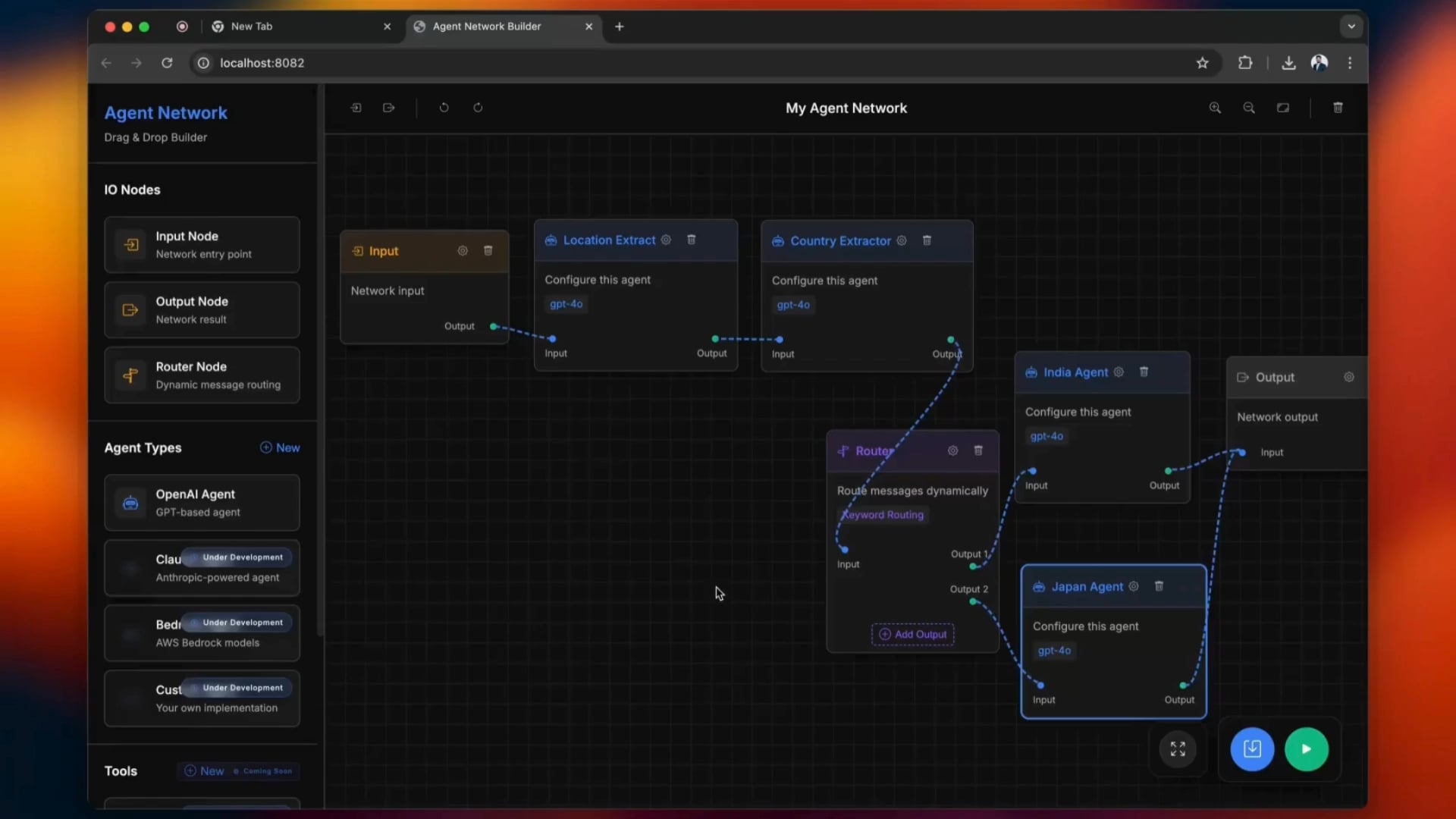
carcodes.xyz: A Social Platform for Car Enthusiasts: After his girlfriend cheated on him, a user developed carcodes.xyz using Claude 3.7 as a programming assistant. The platform, similar to Linktree, allows car enthusiasts to showcase their modified cars, follow other car lovers, share and discover nearby car meets, and provides QR codes that can be placed on cars for others to scan and visit their personal pages. The entire project was built using Next.js, TailwindCSS, MongoDB, and Stripe (Source: Reddit r/ClaudeAI)
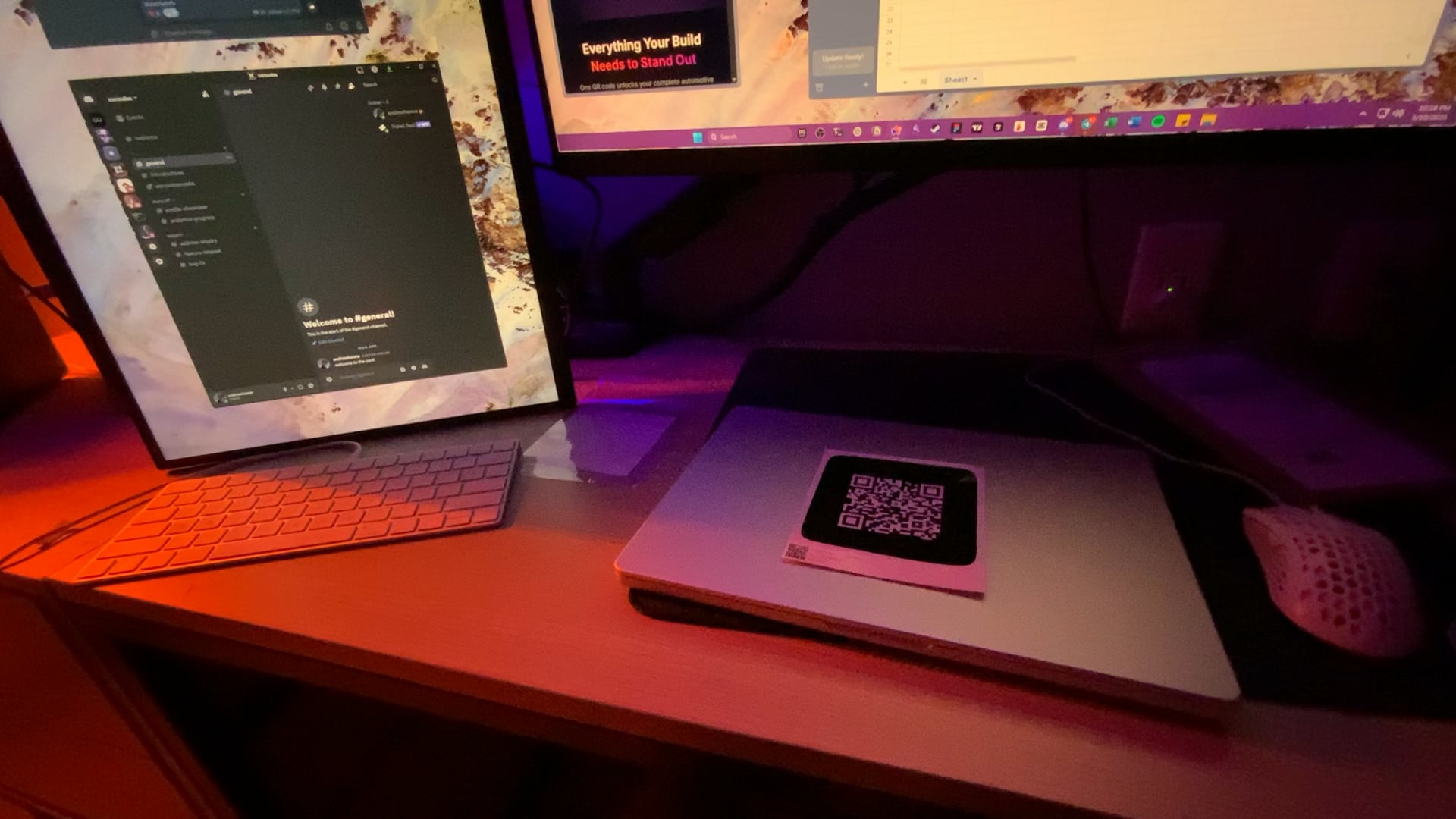
Running Gemma 3 27B Model Locally on AMD RX 7800 XT 16GB: A user shared their experience successfully running the Gemma 3 27B model locally on an AMD RX 7800 XT 16GB graphics card. By using the gemma-3-27B-it-qat-GGUF
version provided by lmstudio-community and a llama.cpp
server, they managed to fully load the model into VRAM and run it with a 16K context length. The share includes detailed hardware configuration, startup commands, parameter settings (based on Unsloth team recommendations), and performance benchmark results in ROCm and Vulkan environments, showing ROCm performed better in this setup (Source: Reddit r/LocalLLaMA)
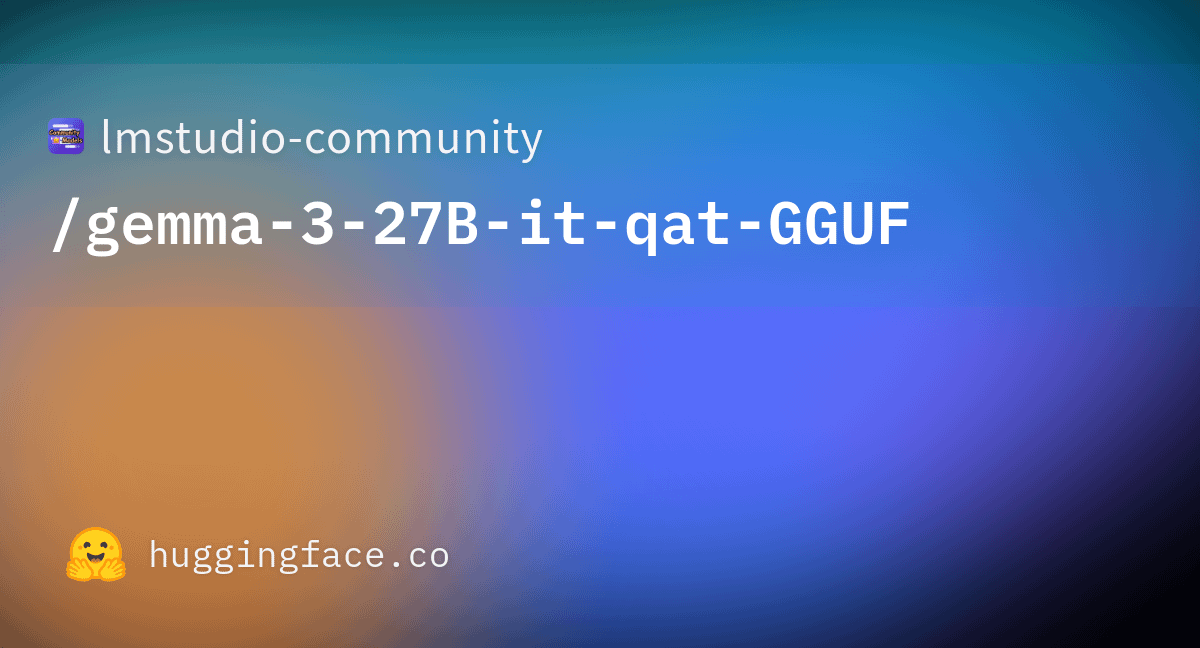
📚 Learning
DSPy Framework Core Philosophy and Advantages Explained: Omar Khattab elaborated on the core design philosophy of the DSPy framework. DSPy aims to provide a stable set of abstractions (like Signatures, Modules, Optimizers) to enable AI software development to adapt to the continuous advancements in LLMs and their methods. Its core ideas include: information flow is key, interactions with LLMs should be functionalized and structured, reasoning strategies should be polymorphic modules, AI behavior specification should be decoupled from learning paradigms, and natural language optimization is a powerful learning paradigm. These principles aim to build “future-proof” AI software, reducing rewriting costs due to changes in underlying models or paradigms. This series of tweets sparked widespread discussion and recognition, considered an important reference for understanding DSPy and modern AI software development (Source: menhguin, lateinteraction, lateinteraction, lateinteraction, lateinteraction, lateinteraction, lateinteraction, lateinteraction, lateinteraction, lateinteraction, lateinteraction, lateinteraction, lateinteraction, lateinteraction, lateinteraction, lateinteraction)
Beginner-Friendly AI Math Workshop: ProfTomYeh announced an upcoming AI math workshop for beginners, aimed at helping participants understand the mathematical principles behind deep learning, such as dot products, matrix multiplication, linear layers, activation functions, and artificial neurons. The workshop will feature a series of interactive exercises, allowing participants to perform mathematical calculations hands-on, thereby demystifying AI math (Source: ProfTomYeh)
“Speech and Language Processing” Textbook Updated Slides Released: Stanford University’s Dan Jurafsky and James H. Martin’s classic textbook “Speech and Language Processing” has released its latest slides. This textbook is an authoritative work in the NLP field, and this update provides valuable open-access resources for learners and educators, aiding in the understanding of cutting-edge technologies like LLMs and Transformers (Source: stanfordnlp)
AI Research Agent Tutorial: Building with LangGraph and Ollama: LangChainAI has released a tutorial guiding users on how to build an AI research agent. This agent can search the web and generate summaries with citations using LangGraph and Ollama, providing users with a complete automated research solution. The tutorial video has been posted on YouTube (Source: LangChainAI, Hacubu)
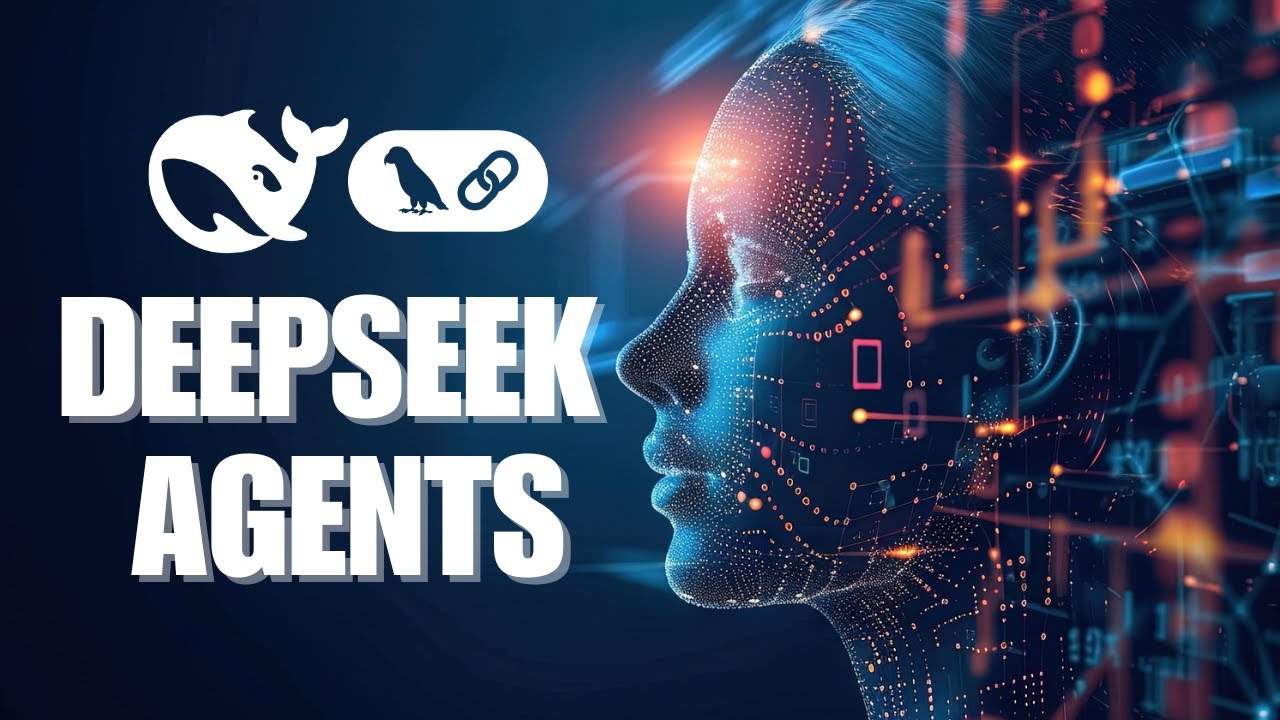
DAIR.AI Releases This Week’s Top AI Papers: DAIR.AI has compiled the top AI papers from May 5th to 11th, 2025, including research achievements such as ZeroSearch, Discuss-RAG, Absolute Zero, Llama-Nemotron, The Leaderboard Illusion, and Reward Modeling as Reasoning, providing researchers with cutting-edge developments (Source: omarsar0)
Article Discussing Agentic Patterns: Phil Schmid shared an in-depth article discussing common agentic patterns, distinguishing between structured workflows and more dynamic agentic patterns. The article helps in understanding and designing more efficient AI agent systems (Source: dl_weekly)
Exploring GPT-4o Sycophancy and Its Implications for Model Training: An article explores the “sycophancy” phenomenon observed in the GPT-4o model, analyzing its connection to RLHF (Reinforcement Learning from Human Feedback) and preference tuning challenges. It also discusses the broader implications for model training, evaluation, and industry transparency (Source: dl_weekly)
Claude System Prompt Leak and Design Analysis: Bindu Reddy analyzed the leaked Claude system prompt. The prompt, at 24k tokens, was much longer than expected, designed to push the limits of LLM logical reasoning, reduce hallucinations, and repeat instructions in multiple ways to ensure LLM understanding. This reveals that current LLMs still face challenges in reliability and instruction following, requiring complex system prompts to correct their behavior (Source: jonst0kes)
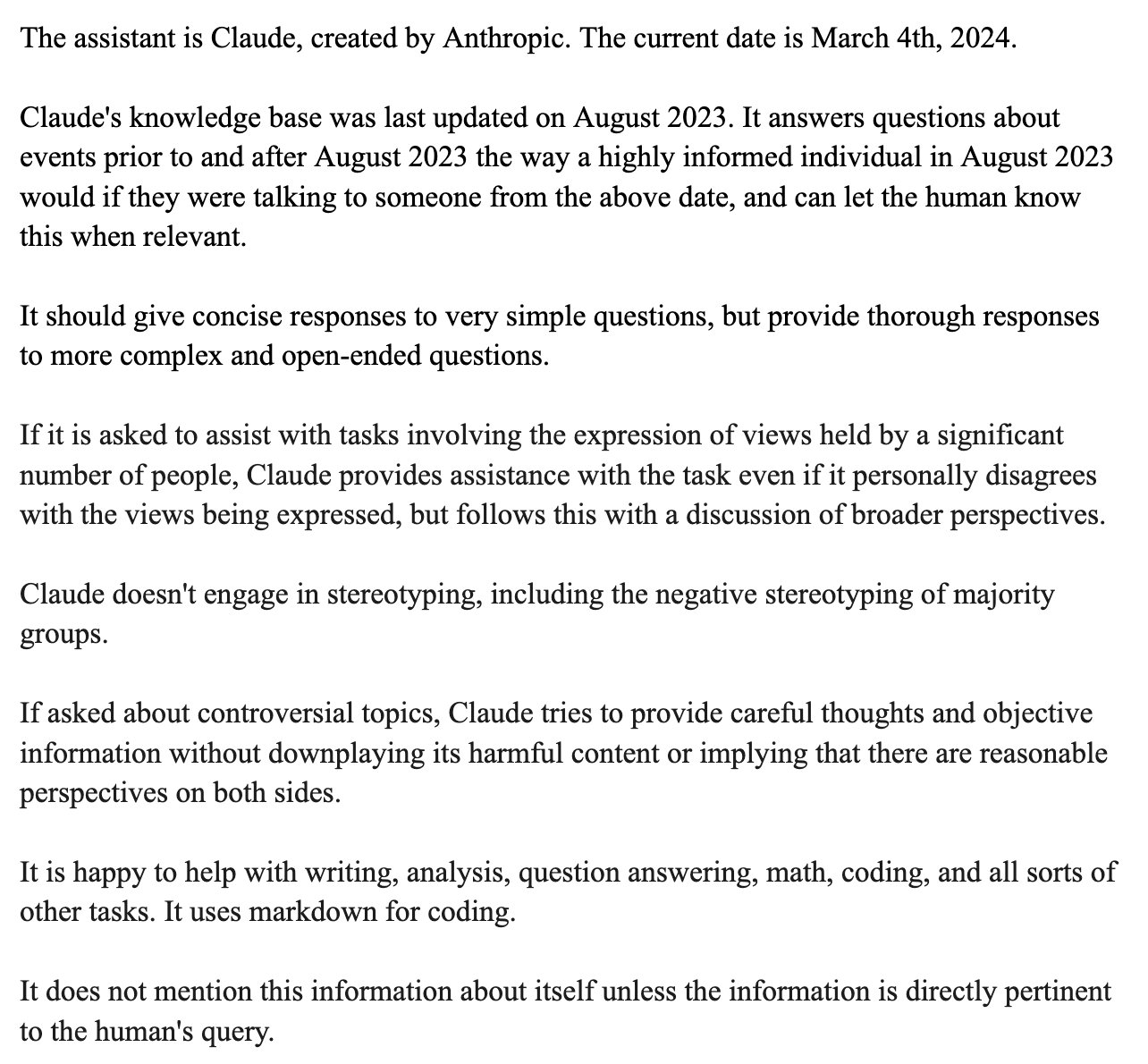
Simulating Bias in Machine Learning: A Bayesian Network Approach: PhD students at Cambridge University and their supervised undergraduates conducted a research project on machine learning bias. They used Bayesian networks to simulate “real-world” data generation processes and then ran machine learning models on this data to measure the bias produced by the models themselves (rather than bias propagated from training data). The project website provides detailed methodology, results, and visualization tools, and solicits feedback from individuals with ML backgrounds (Source: Reddit r/MachineLearning)
💼 Business
Rumors of OpenAI and Microsoft Discussing New Funding Round and Future IPO: According to the Financial Times, OpenAI is in negotiations with Microsoft for new funding support and is exploring the possibility of a future Initial Public Offering (IPO). This suggests OpenAI is continuously seeking funds to support its expensive large model R&D and computing power needs, and may be planning a clearer capital path for its long-term development (Source: Reddit r/artificial)
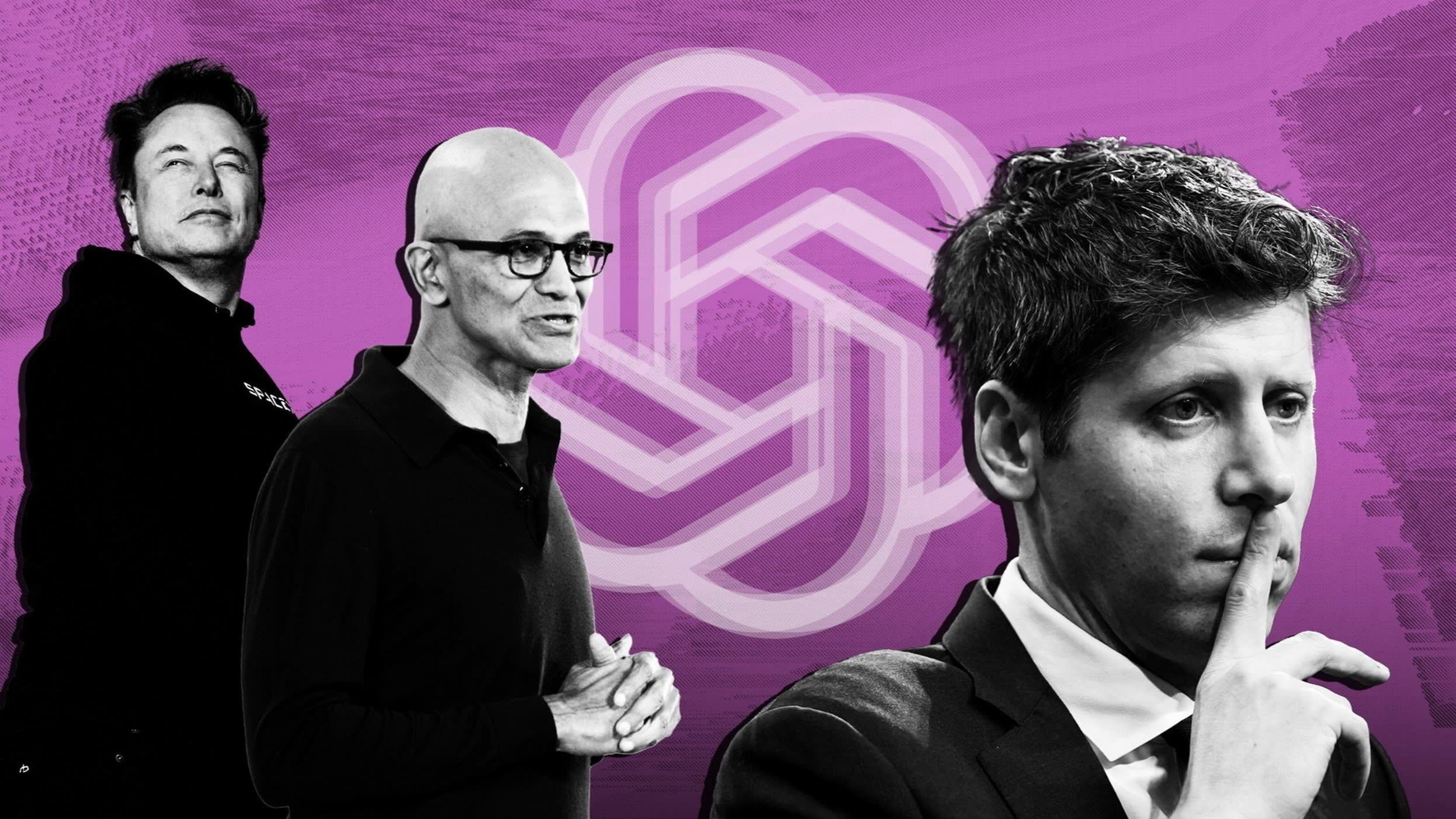
CoreWeave Completes Acquisition of Weights & Biases: Cloud computing provider CoreWeave announced the completion of its acquisition of machine learning tools platform Weights & Biases. This acquisition will combine CoreWeave’s GPU infrastructure with Weights & Biases’ MLOps capabilities, aiming to provide AI developers with a more powerful and integrated development and deployment environment (Source: charles_irl)
Klarna CEO Reflects on AI Cost-Cutting Leading to Decline in Customer Service Quality: The CEO of payments giant Klarna stated that the company “went too far” in its pursuit of cost-cutting through artificial intelligence, leading to a decline in customer service experience, and is now shifting to increase human customer service agents. This incident has sparked discussions on how to balance cost reduction and efficiency gains from AI with maintaining service quality in enterprises (Source: colin_fraser)
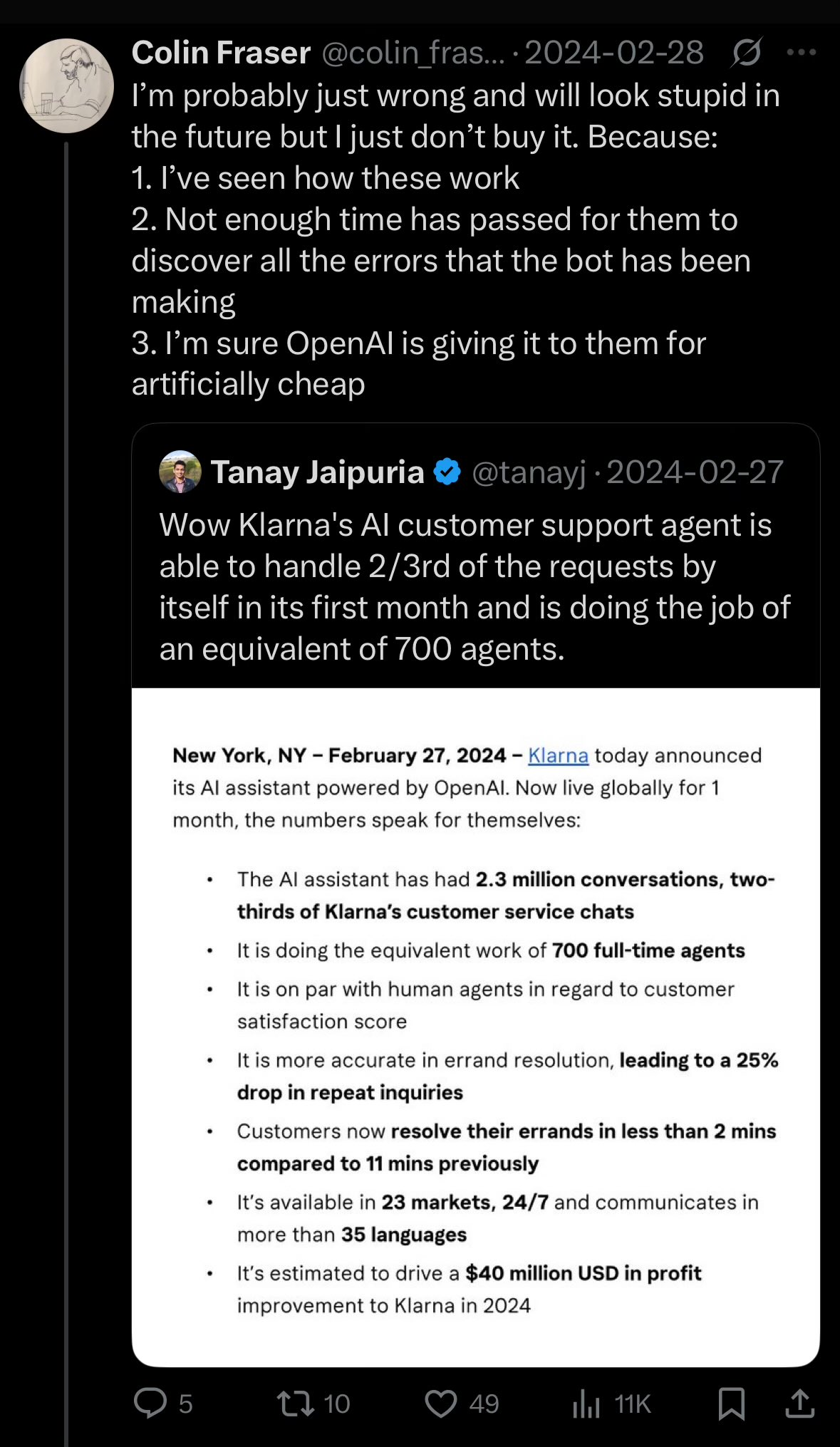
🌟 Community
Heated Debate on Whether LLMs Are the Path to AGI: The community is engaged in a heated discussion about whether Large Language Models (LLMs) are the correct path to achieving Artificial General Intelligence (AGI). One side argues that LLMs are the most successful technology in the machine learning field to date, and asserting they are “definitely not” the path to AGI is too radical. The other side believes that although LLMs have made significant progress, fundamentally different approaches from existing LLMs might be needed to achieve AGI, such as addressing their issues with scalability, long-context coherence, and real-world interaction. Discussants emphasize that scientific exploration should maintain an open mind rather than drawing premature conclusions (Source: cloneofsimo, teortaxesTex, Dorialexander)
Software Developers’ Views on AI Replacement Prospects Differ from Public Perception: Discussions across multiple software development-related subreddits show that many developers believe AI is unlikely to replace them on a large scale in the next 5-10 years, even calling current AI “garbage.” Commentators analyze that this view may stem from developers’ deep understanding of AI’s actual capabilities and the complexity of programming work. They believe AI is currently good at generating boilerplate code or simple tools but is far from independently completing complex software engineering tasks. Investors or the public, on the other hand, might be misled by AI’s superficial capabilities due to a lack of understanding of technical details. At the same time, some argue that AI is indeed a powerful productivity tool, but its role is more auxiliary than a complete replacement, and AI still faces issues like “context loss” and “logical incoherence” when dealing with large-scale, complex projects (Source: Reddit r/ArtificialInteligence)
ML Conference Paper Acceptance Policy Sparks Controversy: Mandatory Attendance Requirement Criticized as Discriminatory: Neel Nanda and others criticized the policy of ML conferences like ICML, which requires at least one author of a paper to attend in person, otherwise accepted papers will be rejected. They argue this is hypocritical; although conferences claim to value DEI (Diversity, Equity, and Inclusion), this policy effectively discriminates against early-career researchers or those with financial difficulties, who often cannot afford high conference fees, while top conference publications are crucial for their career development. Gabriele Berton clarified that ICML would not reject papers for this reason but only requires the purchase of on-site registration, which still did not quell the controversy. Journals like TMLR, which offer free publication and high-quality review, were mentioned as a comparison (Source: menhguin, jeremyphoward)
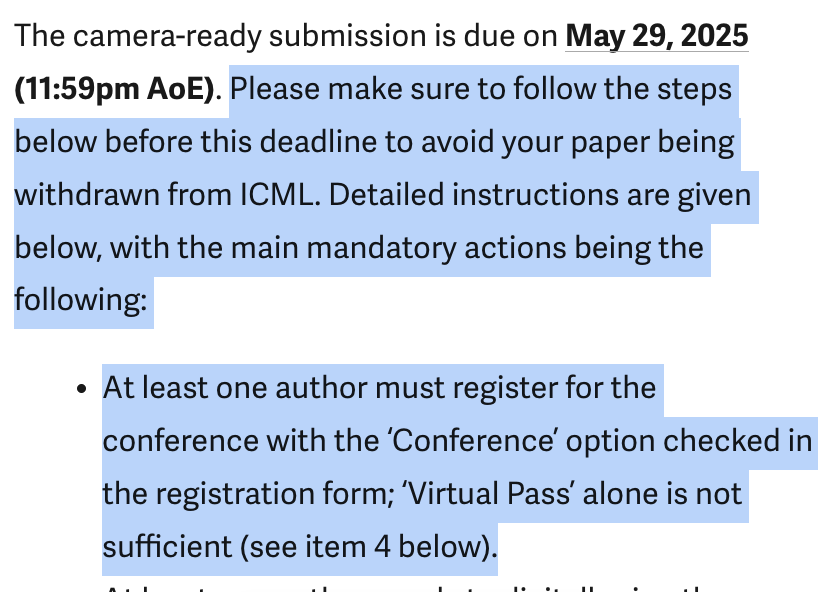
Perception of New Models “Getting Dumber” and Overfitting Discussion: Some users on Reddit communities reported that newly released large models like Qwen3, Llama 3.3/4 feel “dumber” in practical use than older versions, exhibiting tendencies to lose context more easily, repeat content, and have a rigid language style. Some comments suggest this might be due to models being overtrained in pursuit of high benchmark scores (e.g., in programming, math, reducing hallucinations), leading to a decline in their performance in creative writing, natural conversation, etc., making them sound more like they are “sacrificing coherence to sound smart.” Some research indicates that base models might be more suitable for tasks requiring creativity (Source: Reddit r/LocalLLaMA)
Difficulty in Identifying AI-Generated Content Discussed: Toupee Fallacy: Regarding the claim that “it’s easy to identify AI-generated content,” community discussions cited the “toupee fallacy” in rebuttal. This fallacy points out that people believe all toupees look fake because good-quality ones are never noticed. Similarly, those who claim they can always easily identify AI content may only be noticing lower-quality or unedited AI text, while overlooking high-quality AI-generated content that is difficult to distinguish (Source: Reddit r/ChatGPT)
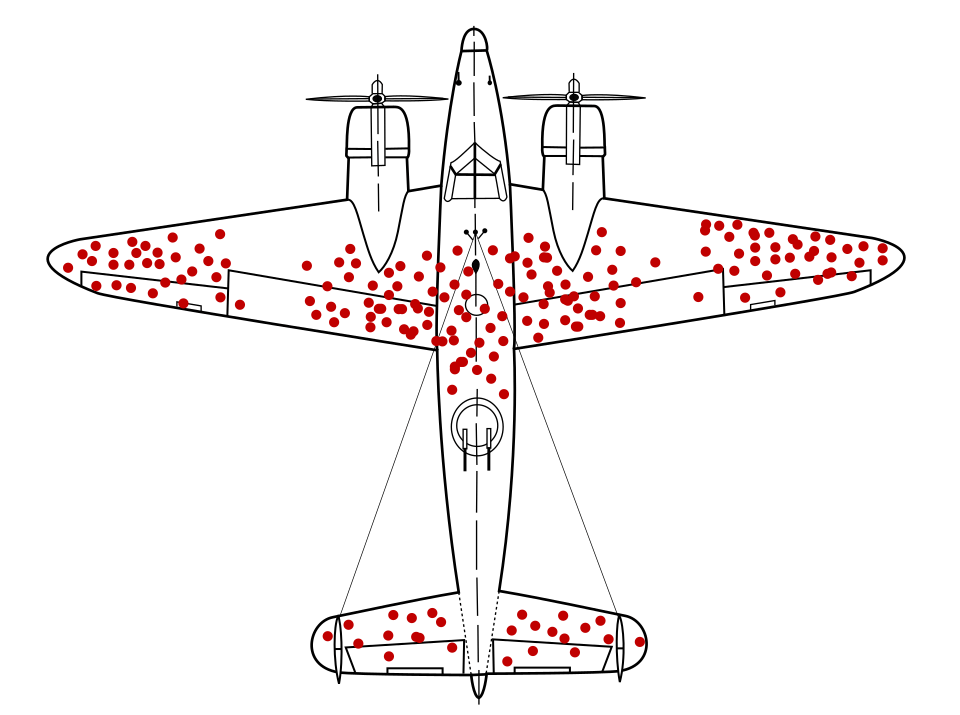
YC Submits Amicus Brief in Google Search Monopoly Antitrust Case: Y Combinator submitted an amicus brief to the U.S. Department of Justice regarding the antitrust case against Google. YC argues that Google’s monopoly in search and search advertising stifles innovation, making it nearly impossible for startups (especially with AI at an inflection point) to break through. This move is interpreted by some commentators as YC supporting emerging AI search companies like Exa, aiming to break Google’s monopoly (Source: menhguin)
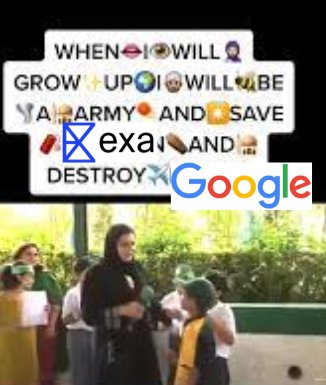
Claude Model Performance Issues Persist, Users Widely Dissatisfied: The Reddit r/ClaudeAI Megathread (May 4-11) shows users continuously reporting Claude availability issues, including extremely low context/message limits, frequent stalling, and output truncation. Anthropic’s status page confirmed increased error rates from May 6-8. Approximately 75% of user feedback was negative, especially from Pro users, who believe there’s a “stealth nerf” to force users to upgrade to the more expensive Max plan. External information confirmed tightened usage policies for the Max plan and high pricing for web search. Despite some temporary workarounds, many core issues remain unresolved, and users are angered by the lack of transparency and unannounced changes (Source: Reddit r/ClaudeAI)
OpenAI Model Selection Advice and Cost-Effectiveness Analysis: In response to OpenAI model selection guides circulating online, Karminski3 proposed more cost-effective suggestions: GPT-4o is suitable for daily tasks and image generation (non-code), priced at $2.5/million tokens; GPT-image-1, though expensive ($10/million tokens), has good image generation/editing results; O3-mini-high ($1.1/million tokens) can be used for code/math, and if it doesn’t suffice, it’s recommended to switch to Claude-3.7-Sonnet-Thinking or Gemini-2.5-Pro, rather than more expensive OpenAI models. The author believes that current OpenAI models are costly for coding and not necessarily the best in performance, and API calls for pure text models exceeding $2/million tokens should be carefully considered (Source: karminski3)
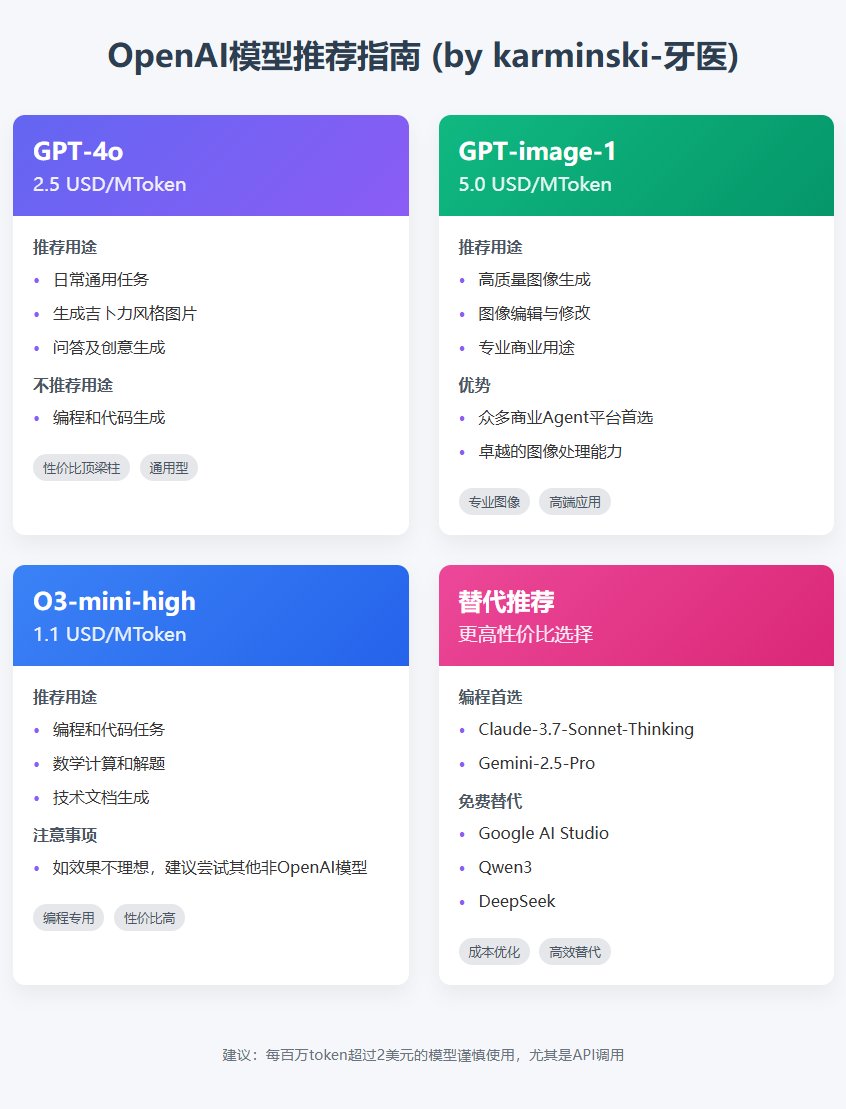
💡 Other
Penrose’s “Three Worlds” Diagram Sparks Reflection on the Relationship Between Mathematics, Physics, and Intelligence: Roger Penrose’s cyclical diagram, featuring the “Platonic Mathematical World,” “Physical World,” and “Mental World” from his book “The Road to Reality,” has sparked new discussions. Commentators suggest that breakthroughs in machine learning seem to corroborate the existence of the “Platonic Mathematical World,” implying that the effectiveness of mathematics stems from a mathematical structure underpinning the physical universe. The emergence of AI (“brains made of sand”) is accelerating this cycle at an unprecedented scale and frequency, potentially revealing deeper truths about the universe (Source: riemannzeta)
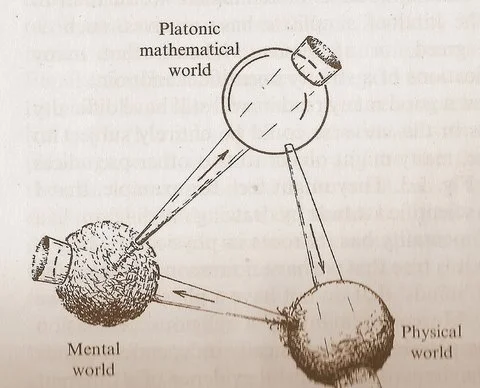
Insurance Companies Launch Coverage for Losses Caused by AI Chatbot Errors: Insurance companies have begun offering insurance products for losses caused by AI chatbot errors. This move, on one hand, acknowledges that improper use of AI can cause serious damage, and on the other, raises concerns that such insurance might encourage businesses to be more reckless in AI application, relying on insurance to cover losses rather than striving to improve the reliability and safety of AI systems (Source: Reddit r/artificial)
AI’s Potential in Music Creation Underestimated: There is a view in the community that many people underestimate AI’s capabilities in music creation, often claiming AI music cannot “touch the soul” like human creations. However, current AI-generated musical works are already audibly close to human vocal performance levels. Considering AI music is still in its nascent stages, its future development potential is immense and should not be prematurely dismissed (Source: Reddit r/artificial)
