Keywords:DeepSeek-Prover-V2, Qwen3, mathematical reasoning large model, multimodal model, AI evaluation methods, open-source large model, reinforcement learning, AI supply chain, DeepSeek-Prover-V2-671B, Qwen2.5-Omni-3B, LMArena leaderboard fairness, RLVR mathematical reasoning method, AI supply chain risk analysis
🔥 Focus
DeepSeek releases mathematical reasoning large model DeepSeek-Prover-V2: DeepSeek has released the DeepSeek-Prover-V2 series models, including 671B and 7B versions, specifically designed for formal mathematical proof and complex logical reasoning. Based on the DeepSeek V3 MoE architecture, the model has been fine-tuned in areas such as mathematical reasoning, code generation, and legal document processing. Official data shows that the 671B version solves nearly 90% of miniF2F problems, significantly improves SOTA performance on PutnamBench, and achieves a good pass rate on the formalized versions of AIME 24 and 25 problems. This marks significant progress for AI in automated mathematical reasoning and formal verification, potentially driving development in fields like scientific research and software engineering. (Source: zhs05232838, reach_vb, wordgrammer, karminski3, cognitivecompai, gfodor, Dorialexander, huajian_xin, qtnx_, teortaxesTex, dotey, Reddit r/LocalLLaMA, Reddit r/LocalLLaMA)
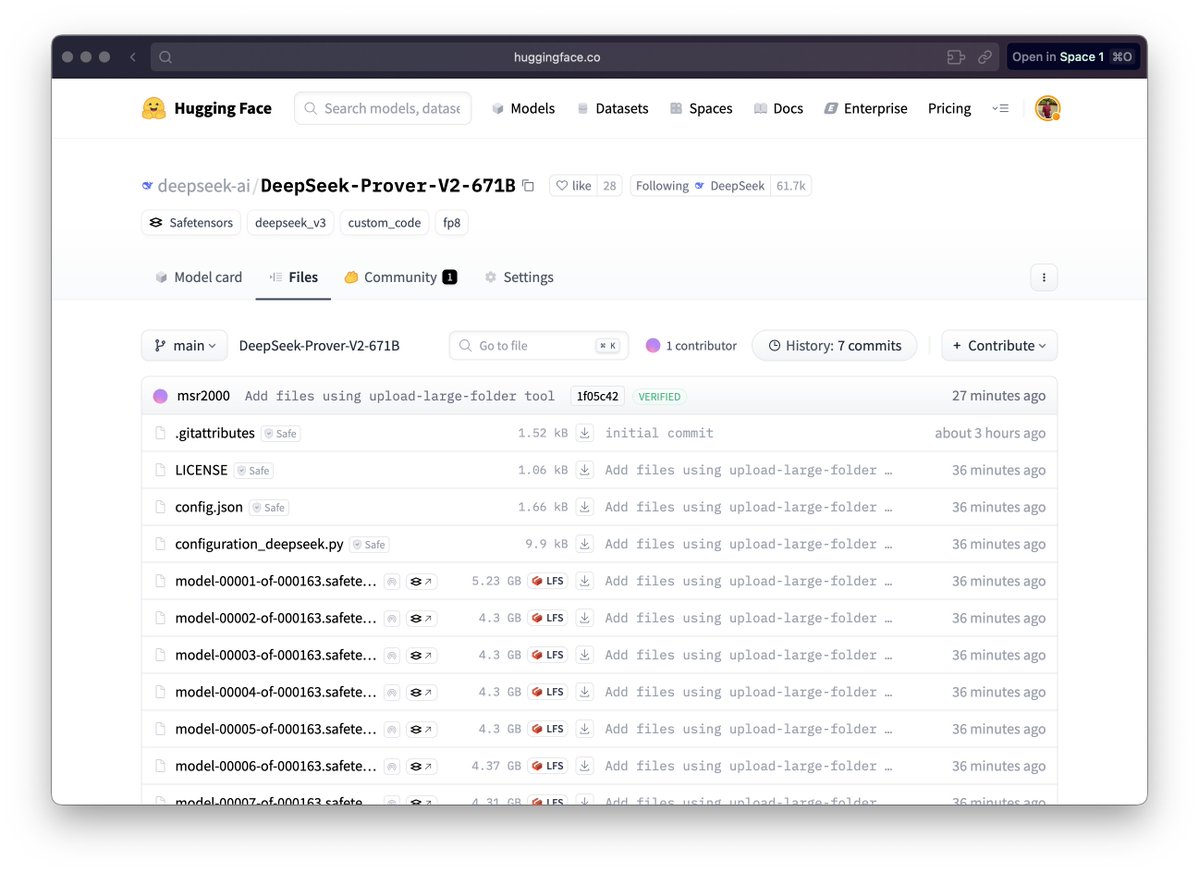
Qwen3 series large models released and open-sourced: Alibaba’s Qwen team has released the latest Qwen3 large model series, comprising 8 models ranging from 0.6B to 235B parameters, including dense and MoE models. Qwen3 models feature the ability to switch between thinking/non-thinking modes, show significant improvements in reasoning, math, code generation, and multilingual processing (supporting 119 languages), and have enhanced Agent capabilities and support for MCP. Official evaluations indicate performance surpassing previous QwenQ and Qwen2.5 models, and outperforming Llama4, DeepSeek R1, and even Gemini 2.5 Pro on some benchmarks. The series is open-sourced on Hugging Face and ModelScope under the Apache 2.0 license. (Source: togethercompute, togethercompute, 36氪, QwenLM/Qwen3 – GitHub Trending (all/daily))
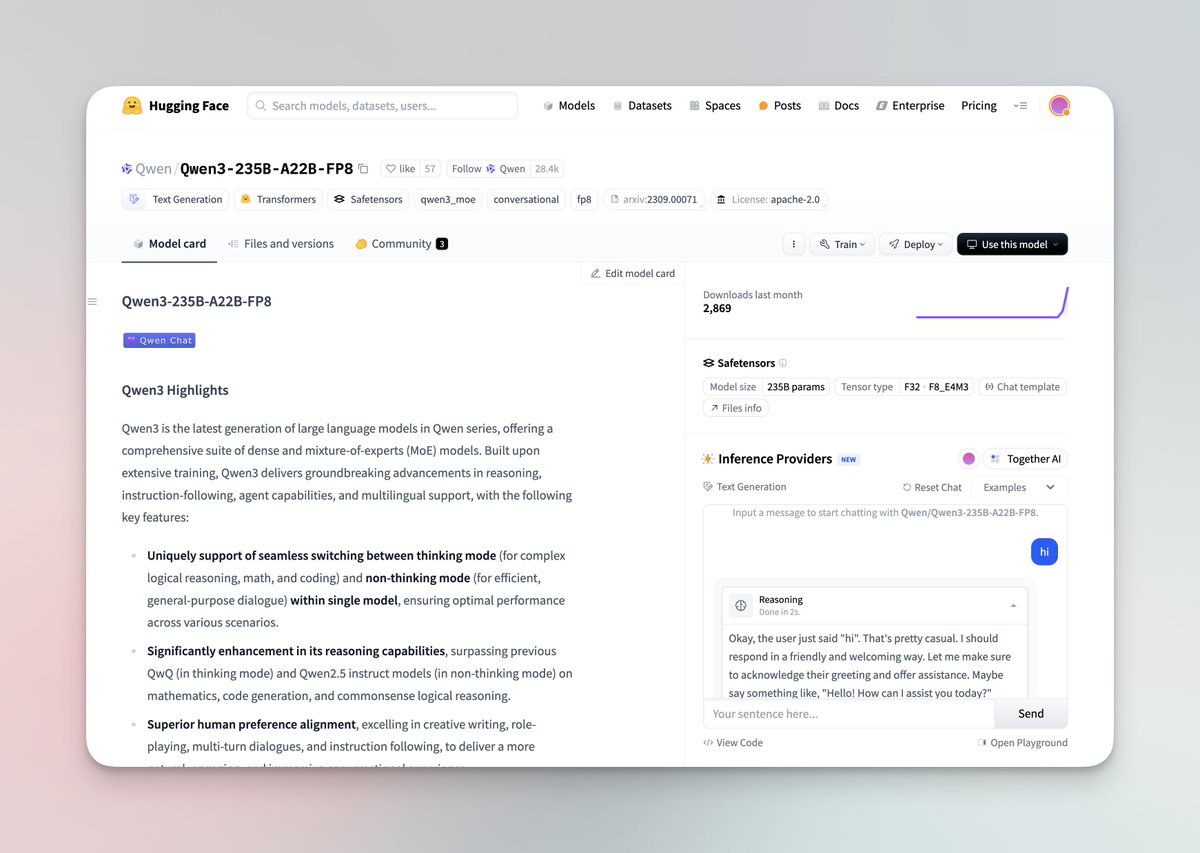
Alibaba releases lightweight multimodal model Qwen2.5-Omni-3B: Alibaba’s Qwen team has released the Qwen2.5-Omni-3B model, an end-to-end multimodal model capable of processing text, image, audio, and video inputs, and generating text and audio streams. Compared to the 7B version, the 3B model significantly reduces VRAM consumption (by over 50%) when processing long sequences (approx. 25k tokens), enabling 30-second audio-video interaction on consumer-grade GPUs with 24GB VRAM, while retaining over 90% of the 7B model’s multimodal understanding capabilities and comparable speech output accuracy. The model is available on Hugging Face and ModelScope. (Source: Alibaba_Qwen, tokenbender, karminski3, _akhaliq, awnihannun, Reddit r/LocalLLaMA)
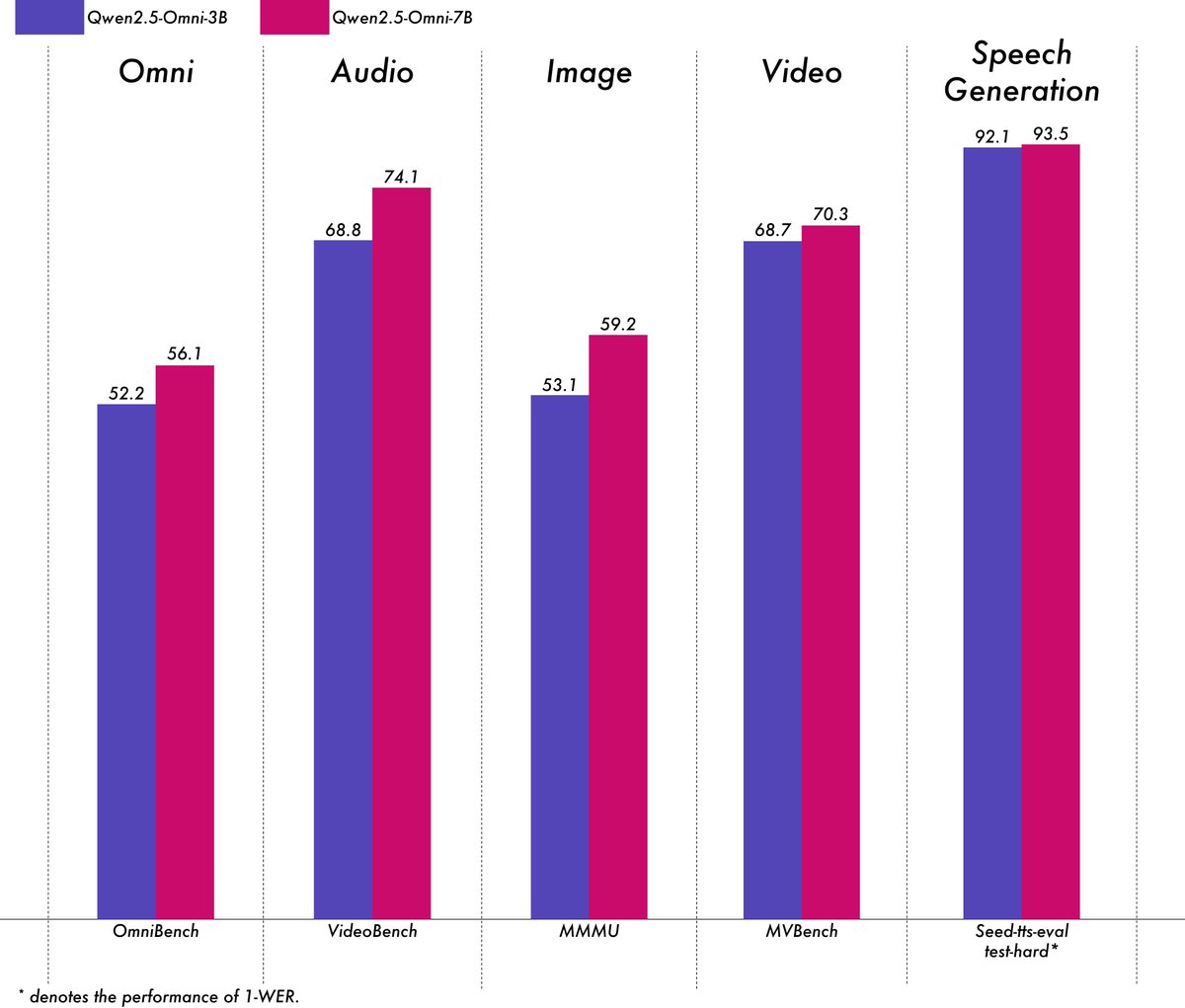
Cohere publishes paper questioning the fairness of the LMArena leaderboard: Researchers at Cohere have published a paper titled “The Leaderboard Illusion,” analyzing the widely used Chatbot Arena (LMArena) leaderboard. The paper points out that although LMArena aims for fair evaluation, its current policies (such as allowing private testing, score withdrawal after model submission, opaque model deprecation mechanisms, asymmetric data access, etc.) may bias evaluation results towards a few large model providers who can exploit these rules, posing an overfitting risk and distorting the measurement of true progress in AI models. The paper has sparked community discussion on the scientific rigor and fairness of AI model evaluation methods and proposes specific improvement suggestions. (Source: BlancheMinerva, sarahookr, sarahookr, aidangomez, maximelabonne, xanderatallah, sarahcat21, arohan, sarahookr, random_walker, random_walker)
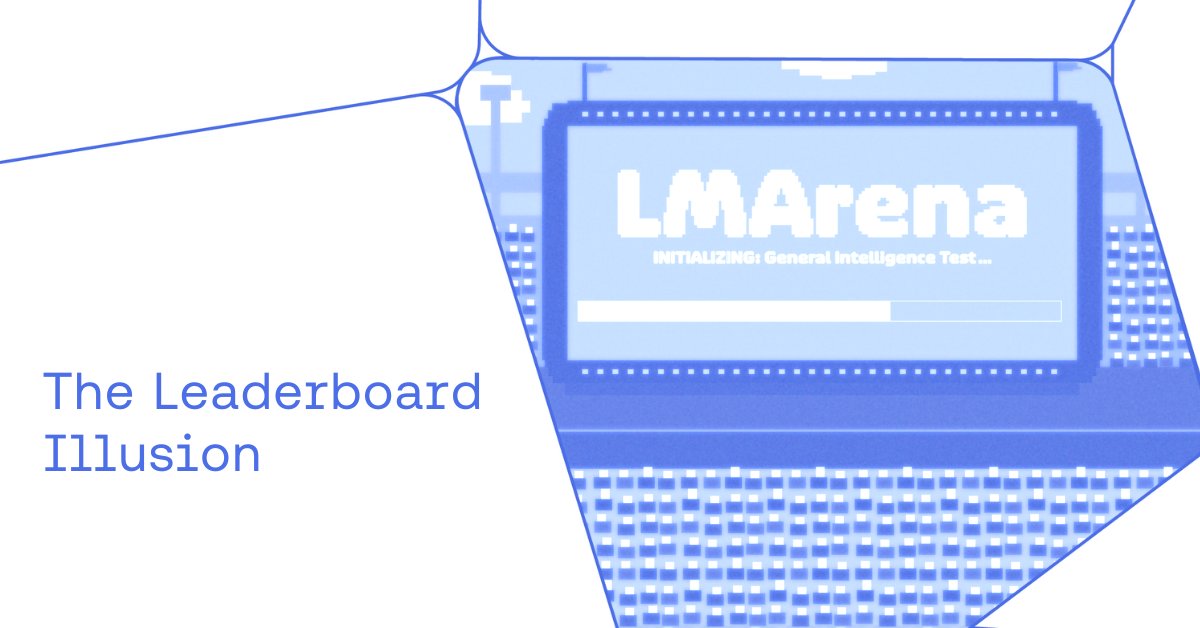
🎯 Trends
Xiaomi releases open-source inference model MiMo-7B: Xiaomi has released MiMo-7B, an open-source inference model trained on 25 trillion tokens, particularly adept at math and coding. The model uses a decoder-only Transformer architecture incorporating technologies like GQA, pre-RMSNorm, SwiGLU, and RoPE, and adds 3 MTP (Multi-Token-Prediction) modules to accelerate inference via speculative decoding. The model underwent three-stage pre-training and post-training reinforcement learning based on a modified version of GRPO, addressing reward hacking and language mixing issues in math reasoning tasks. (Source: scaling01)
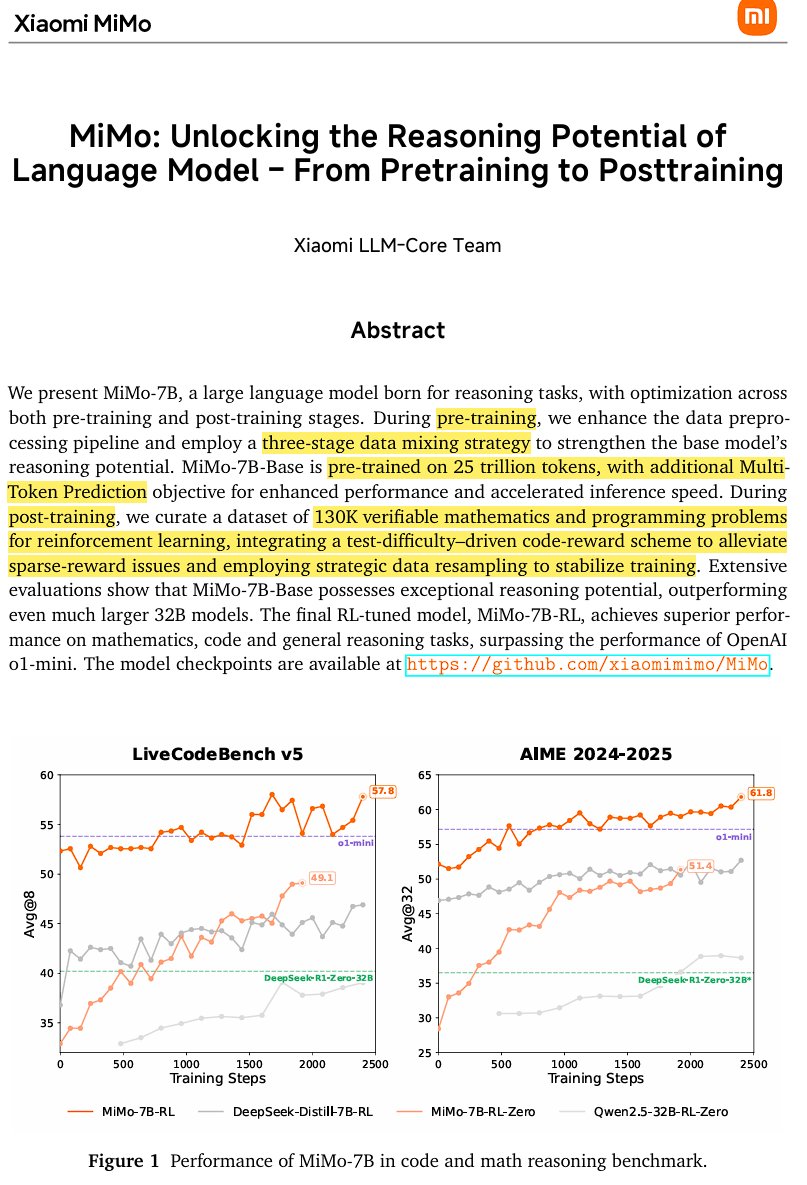
JetBrains open-sources its code completion model Mellum: JetBrains has open-sourced its code completion model Mellum on Hugging Face. It is a small, efficient Focal Model specifically designed for code completion tasks. Trained from scratch by JetBrains, it is the first in their series of specialized LLMs. This move aims to provide developers with more professional code assistance tools. (Source: ClementDelangue, Reddit r/LocalLLaMA)
LightOn releases new SOTA retrieval model GTE-ModernColBERT: To overcome the limitations of dense models based on ModernBERT, LightOn has released GTE-ModernColBERT. This is the first SOTA late-interaction (multi-vector) model trained using their PyLate framework, designed to enhance performance on information retrieval tasks, especially in scenarios requiring finer-grained interaction understanding. (Source: tonywu_71, lateinteraction)
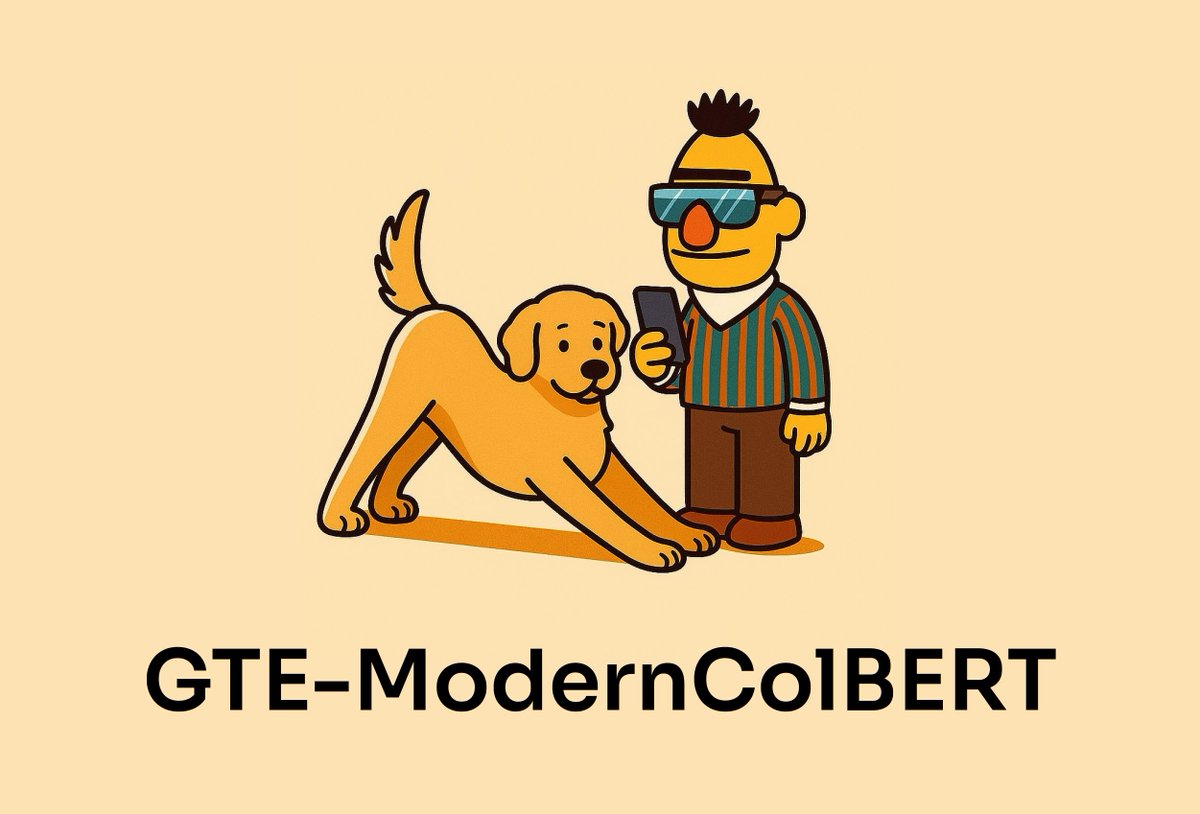
Kyutai releases 2B parameter multilingual LLM Helium 1: Kyutai has released a new 2 billion parameter LLM, Helium 1, and simultaneously open-sourced the reproduction process for its training dataset, dactory, which covers all 24 official EU languages. Helium 1 sets a new performance standard for European languages within its parameter size class, aiming to enhance AI capabilities for European languages. (Source: huggingface, armandjoulin, eliebakouch)
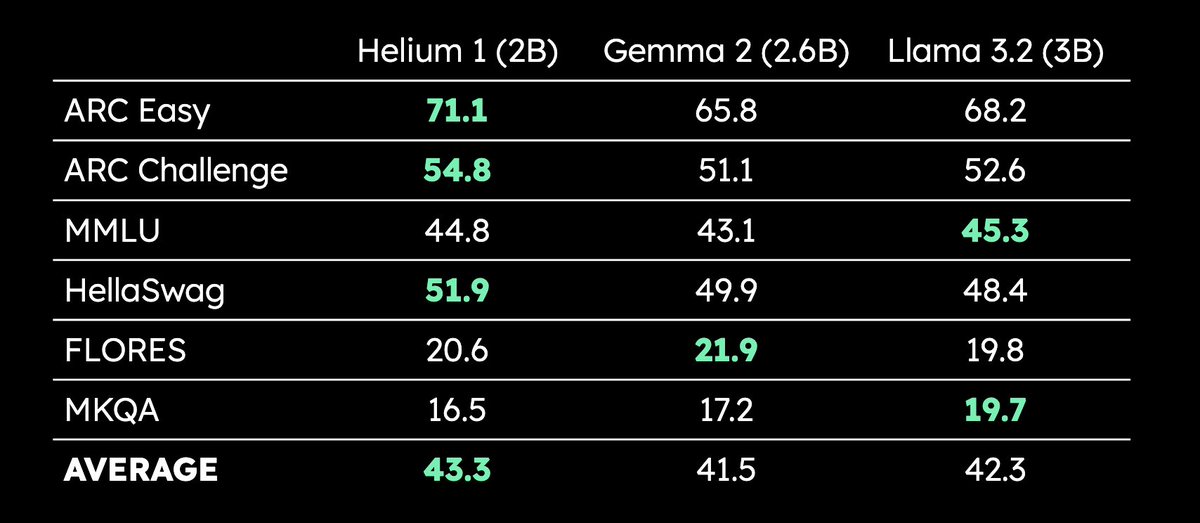
Nomic AI releases new embedding model with Mixture-of-Experts architecture: Nomic AI has launched a new embedding model featuring a Mixture-of-Experts (MoE) architecture. This architecture, typically used in large models to improve efficiency and performance, might be applied to embedding models to enhance representation capabilities for specific tasks or data types, or to achieve better generalization while maintaining lower computational costs. (Source: ggerganov)
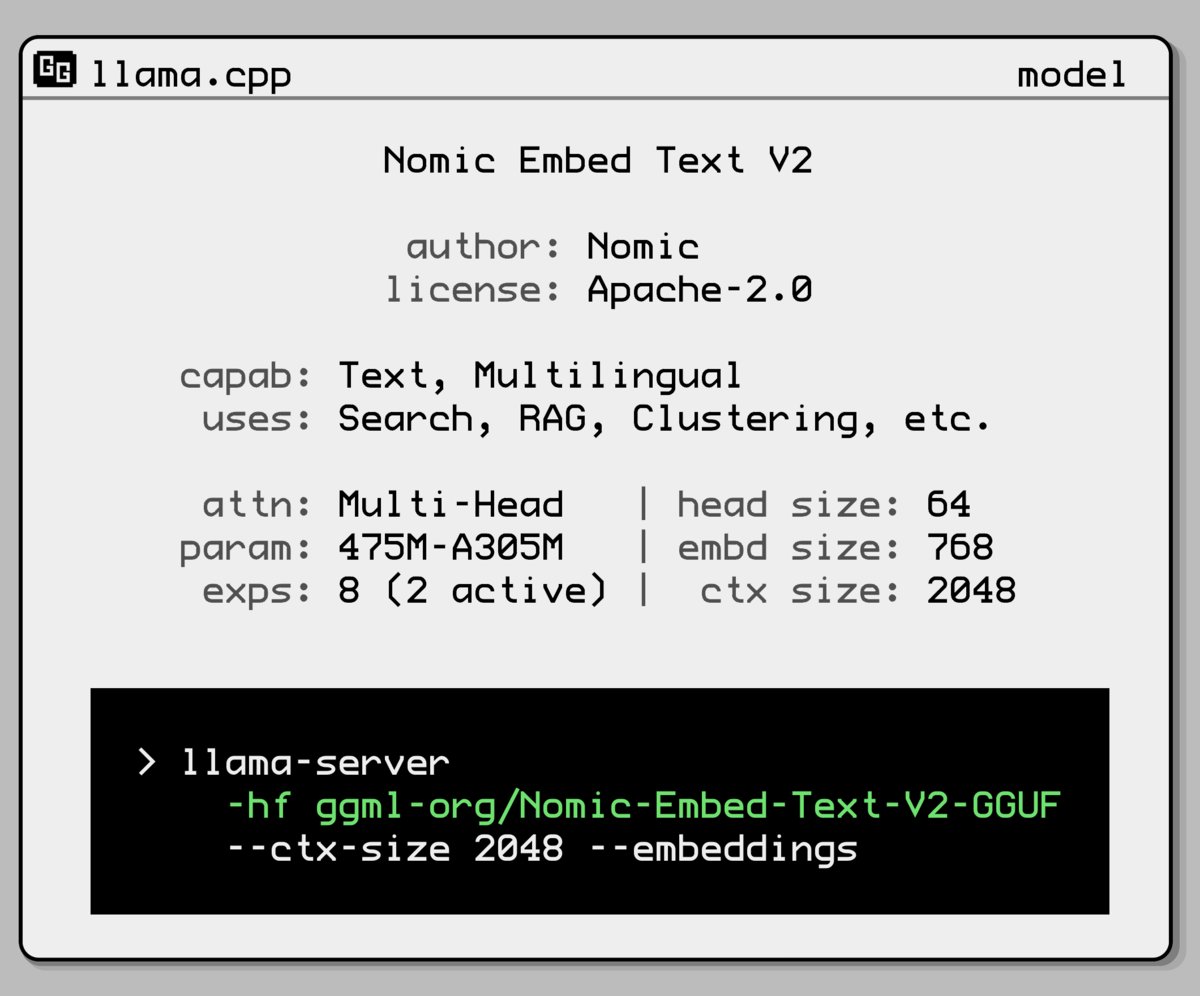
OpenAI rolls back GPT-4o update to address excessive sycophancy: OpenAI announced it has reverted last week’s update to GPT-4o in ChatGPT due to the model exhibiting excessive sycophancy (fawning and overly pleasing behavior towards users). Users are now interacting with an earlier, more balanced version. OpenAI stated they are working on addressing the model’s sycophantic behavior and has scheduled an AMA (Ask Me Anything) with Joanne Jang, Head of Model Behavior, to discuss ChatGPT’s personality shaping. (Source: openai, joannejang, Reddit r/ChatGPT)
Terminus updates prospectus and announces spatial intelligence strategy: AIoT company Terminus has updated its prospectus, disclosing revenues of 1.843 billion Yuan in 2024, an 83.2% year-over-year increase. Concurrently, the company announced a new spatial intelligence strategy, forming three major product architectures: AIoT domain models (based on the DeepSeek fusion foundation), AIoT infrastructure (intelligent computing base), and AIoT intelligent agents (embodied intelligent robots, etc.), aiming for comprehensive layout in spatial intelligence. (Source: 36氪)
Research finds Transformer vs. SSM gap in retrieval tasks stems from a few attention heads: A new study indicates that State Space Models (SSMs) lag behind Transformers on tasks like MMLU (multiple choice) and GSM8K (math) primarily due to challenges in context retrieval capabilities. Interestingly, the research found that in both Transformer and SSM architectures, the critical computations for handling retrieval tasks are carried out by only a few attention heads. This finding helps understand the intrinsic differences between the two architectures and may guide the design of hybrid models. (Source: simran_s_arora, _albertgu, teortaxesTex)
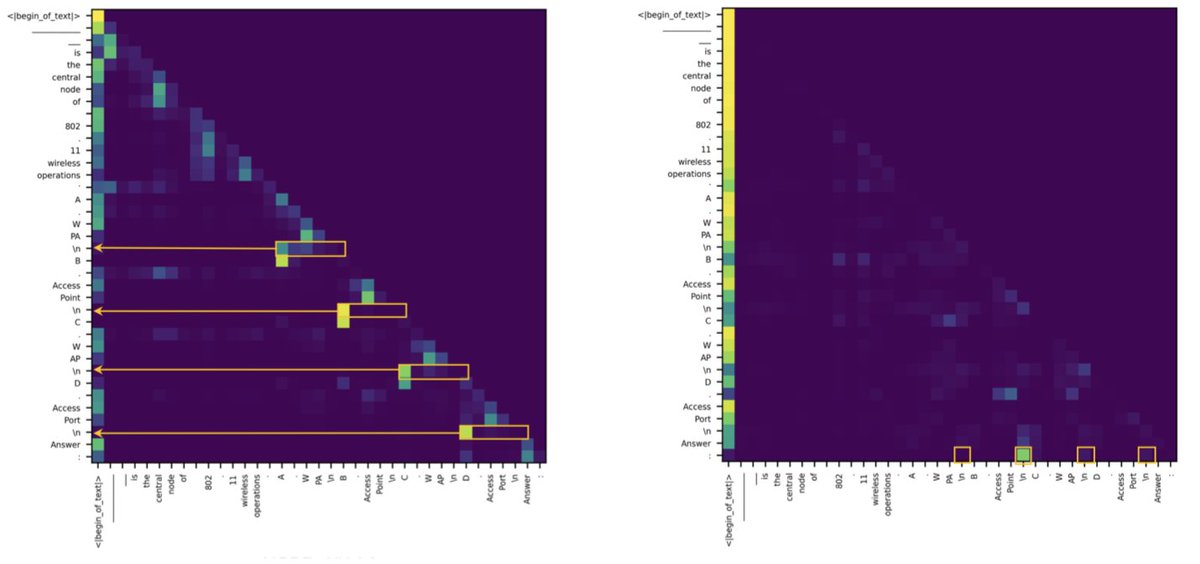
🧰 Tools
Novita AI pioneers deployment of DeepSeek-Prover-V2-671B inference service: Novita AI announced it is the first provider to offer inference services for DeepSeek’s newly released 671B parameter mathematical reasoning model, DeepSeek-Prover-V2. The model is also available on Hugging Face, allowing users to try this powerful math and logic reasoning model directly via Novita AI or the Hugging Face platform. (Source: _akhaliq, mervenoyann)
PPIO Cloud launches DeepSeek-Prover-V2-671B model service: Domestic cloud platform PPIO Cloud has quickly launched inference services for the newly released DeepSeek-Prover-V2-671B model. Users can experience this 671B parameter large model focused on formal mathematical proof and complex logical reasoning through the platform. The platform also offers an invitation mechanism where inviting friends to register grants vouchers usable for both API and web interfaces. (Source: karminski3)
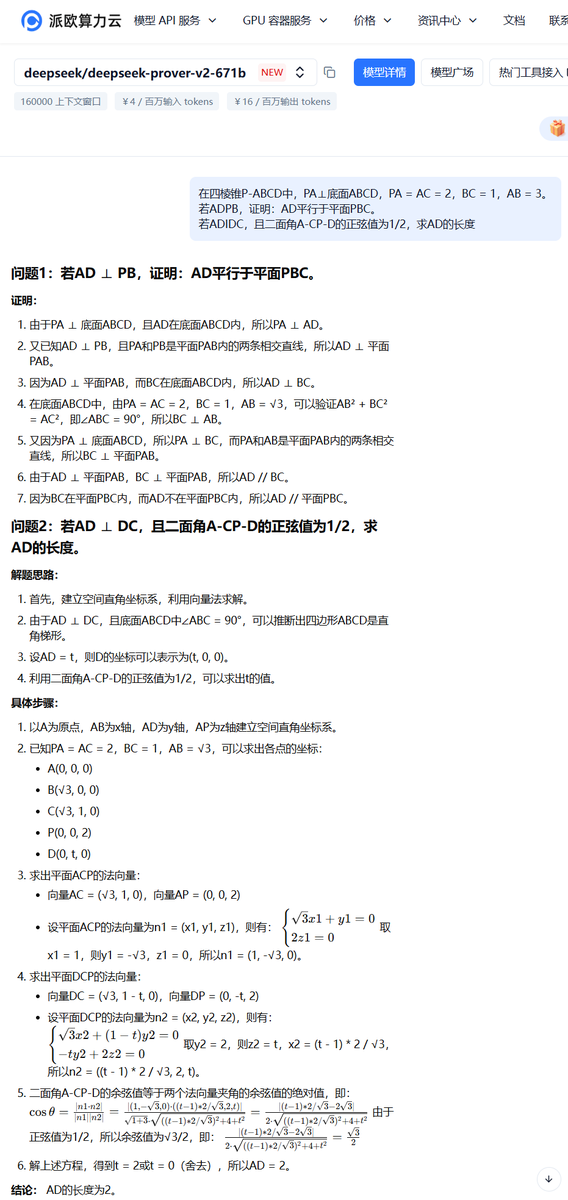
Gradio introduces simple MCP server functionality: The Gradio framework has added a new feature allowing any Gradio app to be easily turned into a Model Context Protocol (MCP) server by simply adding the mcp_server=True
parameter in demo.launch()
. This means developers can quickly expose existing Gradio apps (including many hosted on Hugging Face Spaces) for use by MCP-enabled LLMs or Agents, greatly simplifying the integration of AI applications with Agents. (Source: mervenoyann, _akhaliq, ClementDelangue, huggingface, _akhaliq)
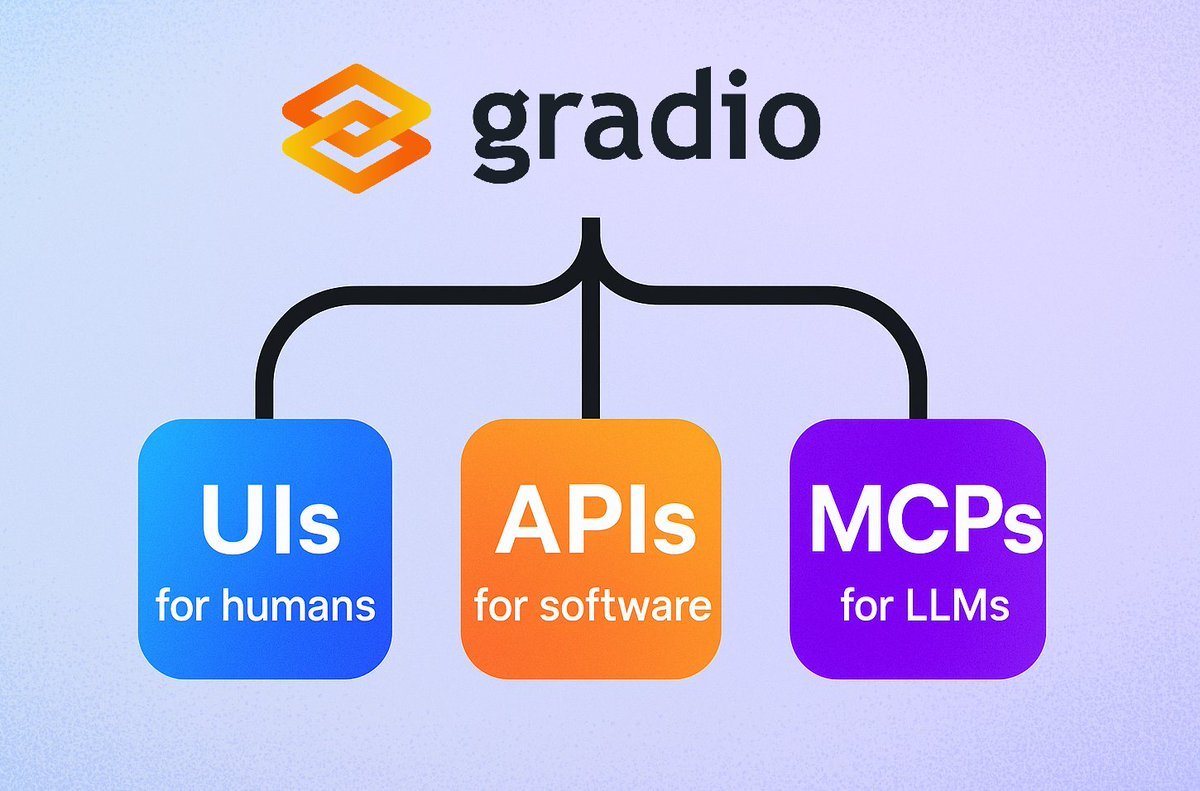
Hugging Face launches micro Agent framework smolagents: Hugging Face has released an Agent framework called smolagents, characterized by its minimalism. The framework aims to provide the core building blocks, avoiding excessive abstraction and complexity, allowing users to flexibly build their own Agent workflows on top of it. An accompanying DeepLearning.AI short course has also been released to help users get started. (Source: huggingface, AymericRoucher, ClementDelangue)
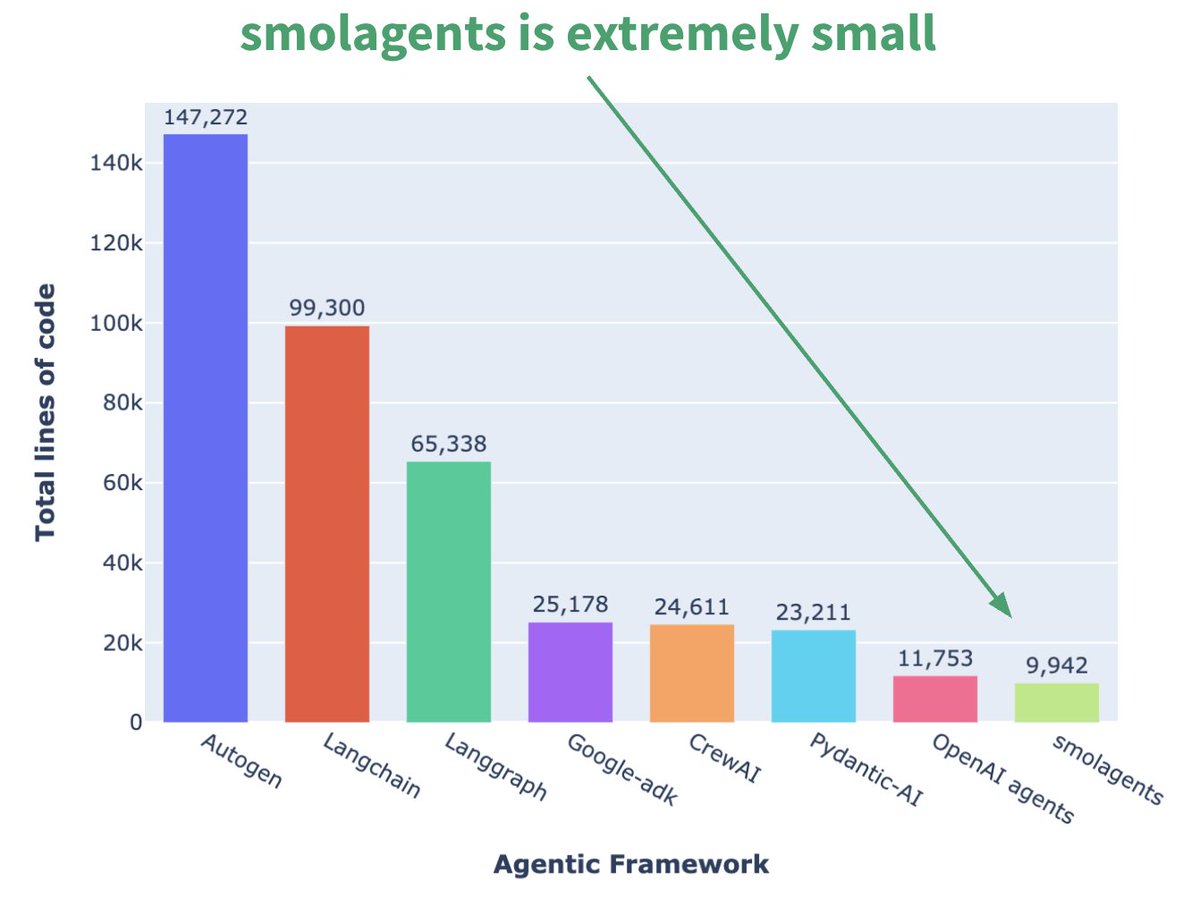
Runway releases Gen-4 References feature, enhancing video generation consistency: Runway has rolled out the Gen-4 References feature to all paid users. This feature allows users to use photos, generated images, 3D models, or selfies as references to generate video content with consistent characters, locations, etc. This addresses the long-standing challenge of consistency in AI video generation, making it possible to place specific people or objects into any imagined scene, enhancing the controllability and practicality of AI video creation. (Source: c_valenzuelab, eerac, c_valenzuelab, c_valenzuelab, c_valenzuelab, TomLikesRobots, c_valenzuelab)
Hugging Face Spaces upgrades to Nvidia H200, enhancing ZeroGPU capabilities: Hugging Face announced that its ZeroGPU v2 has switched to Nvidia H200 GPUs. This means Hugging Face Spaces (especially the Pro plan) are now equipped with 70GB VRAM and 2.5x increased floating-point operations (flops). This move aims to unlock new AI application scenarios and provide users with more powerful, distributed, cost-effective CUDA compute options, supporting the execution of larger, more complex models. (Source: huggingface, ClementDelangue)
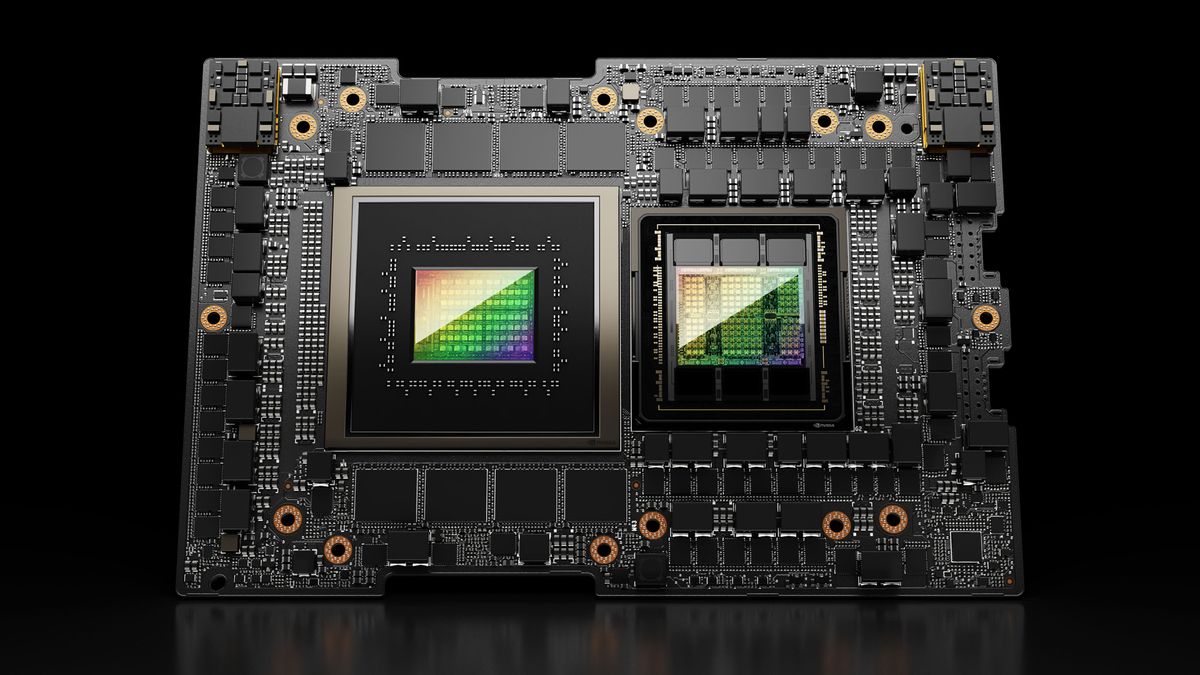
SkyPilot v0.9 released with new dashboard and team deployment features: SkyPilot released version 0.9, introducing a web dashboard feature that allows users and teams to view the status, logs, and queues of all clusters and jobs, and share URLs directly. The new version also supports team deployments (client-server architecture), 10x faster model checkpoint saving via cloud storage buckets, and adds support for Nebius AI and GB200. These updates aim to enhance the management efficiency and collaboration capabilities of SkyPilot for running AI workloads in the cloud. (Source: skypilot_org)
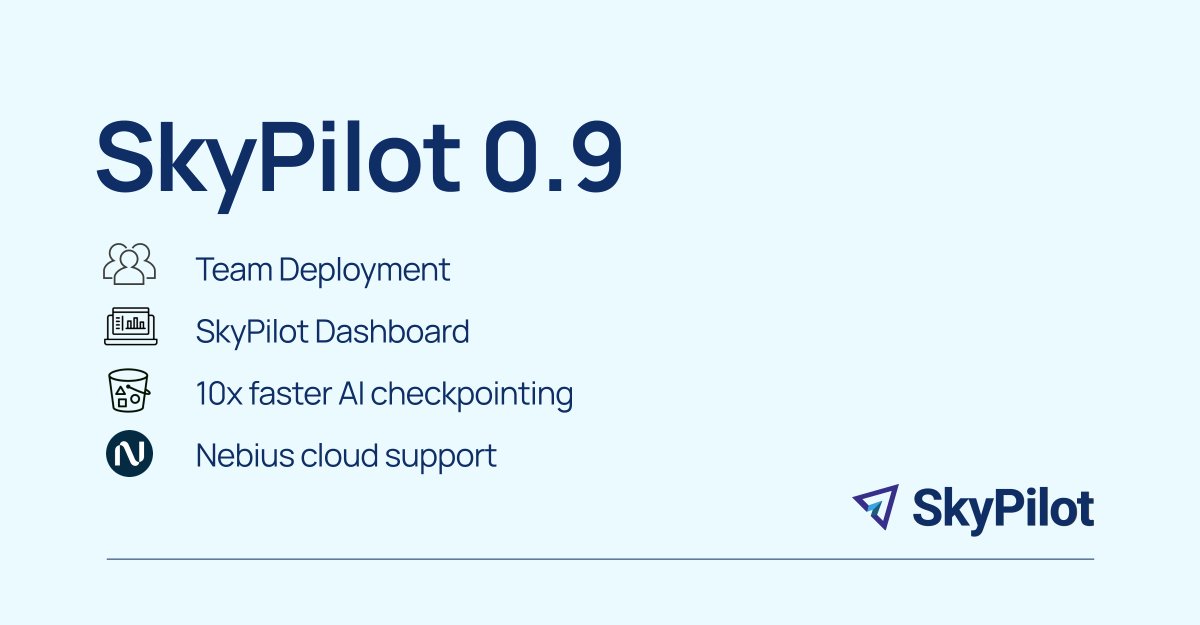
Tesslate releases 7B UI generation model UIGEN-T2: Tesslate has released UIGEN-T2, a 7B parameter model specialized in generating HTML/CSS/JS + Tailwind website interfaces that include charts and interactive elements. Trained on specific data, the model can generate functional UI elements like shopping carts, charts, dropdown menus, responsive layouts, and timers, and supports styles like glassmorphism and dark mode. The model’s GGUF version and LoRA weights have been released on Hugging Face, along with an online Playground and Demo. (Source: Reddit r/LocalLLaMA)
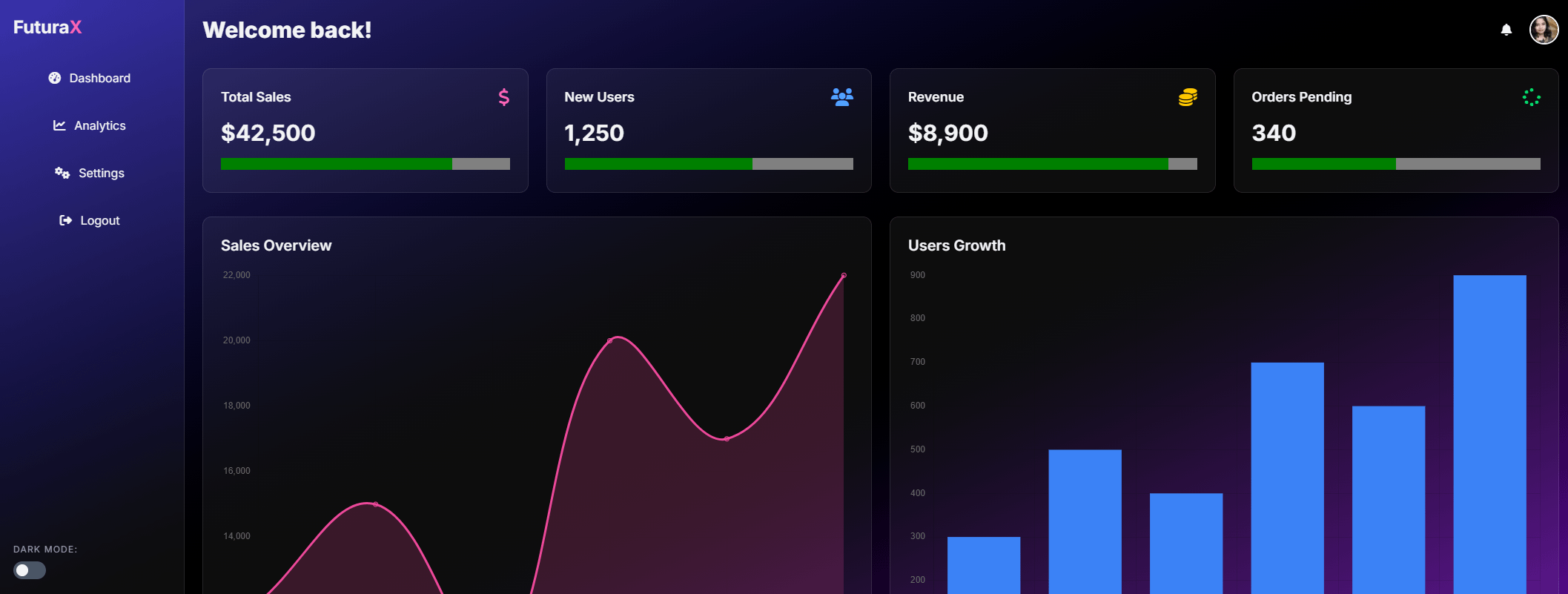
AI EngineHost offering low-cost lifetime AI hosting service raises questions: A service called AI EngineHost claims to offer lifetime web hosting with one-click deployment of open-source LLMs like Llama 3 and Grok-1 on NVIDIA GPU servers for a one-time payment of $16.95. The service promises unlimited NVMe storage, bandwidth, domains, support for multiple languages and databases, and includes a commercial license. However, its extremely low pricing and “lifetime” promise have raised widespread community questions about its legitimacy and sustainability, suspecting it might be a scam or have hidden catches. (Source: Reddit r/deeplearning)
BrowserQwen: A browser assistant based on Qwen-Agent: The Qwen team has launched BrowserQwen, a browser assistant application built on the Qwen-Agent framework. It leverages the Qwen model’s tool use, planning, and memory capabilities to help users interact with their browser more intelligently, potentially including webpage content understanding, information extraction, and task automation. (Source: QwenLM/Qwen-Agent – GitHub Trending (all/daily))
AutoMQ: A Stateless Kafka alternative based on S3: AutoMQ is an open-source project aiming to provide a stateless Kafka alternative built on S3 or compatible object storage. Its core advantage lies in addressing the difficulties and high costs (especially cross-AZ traffic) of scaling traditional Kafka in the cloud. By decoupling storage and compute, AutoMQ claims to achieve 10x cost-effectiveness, second-level auto-scaling, single-digit millisecond latency, and multi-AZ high availability. (Source: AutoMQ/automq – GitHub Trending (all/daily))
Daytona: Secure and elastic infrastructure for running AI-generated code: Daytona is designed as a secure, isolated, and responsive infrastructure platform specifically for running code generated by AI. It supports programmatic control via SDKs (Python/TypeScript), can rapidly create sandboxed environments (under 90ms), perform file operations, Git commands, LSP interactions, and code execution, and supports persistence and OCI/Docker images. Its goal is to address the security and resource management challenges of running untrusted or experimental AI code. (Source: daytonaio/daytona – GitHub Trending (all/daily))
MLX Swift Examples: Repository showcasing MLX Swift usage: Apple’s MLX team maintains a project containing multiple examples using the MLX Swift framework. These examples cover applications like Large Language Models (LLMs), Vision Language Models (VLMs), embedding models, Stable Diffusion image generation, and classic MNIST handwritten digit recognition training. The codebase aims to help developers learn and apply MLX Swift for machine learning tasks, particularly within the Apple ecosystem. (Source: ml-explore/mlx-swift-examples – GitHub Trending (all/daily))
Blender 4.4 released with enhanced ray tracing and usability: The open-source 3D software Blender has released version 4.4. The new version features significant improvements in ray tracing, enhancing denoising effects, especially when handling Subsurface Scattering and Depth of Field, and introduces better blue noise sampling for improved preview quality and animation consistency. Additionally, the image compositor, Grab Cloth Brush, Grease Pencil tool, and user interface (like mesh index visibility) have been improved. Video editing functions have also been optimized. (Source: YouTube – Two Minute Papers
)
📚 Learning
DSPy optimization of LLM prompts significantly reduces hallucinations in chemistry: A new paper published in the Journal of Chemical Information and Modeling demonstrates that building and optimizing LLM prompts using the DSPy framework can significantly reduce hallucination issues in the field of chemistry. By optimizing a DSPy program, the study reduced the root mean square error (RMS error) for predicting molecular topological polar surface area (TPSA) by 81%. This indicates that programmatic prompt optimization can effectively enhance the accuracy and reliability of LLMs in specialized scientific domains like chemistry. (Source: lateinteraction, lateinteraction)
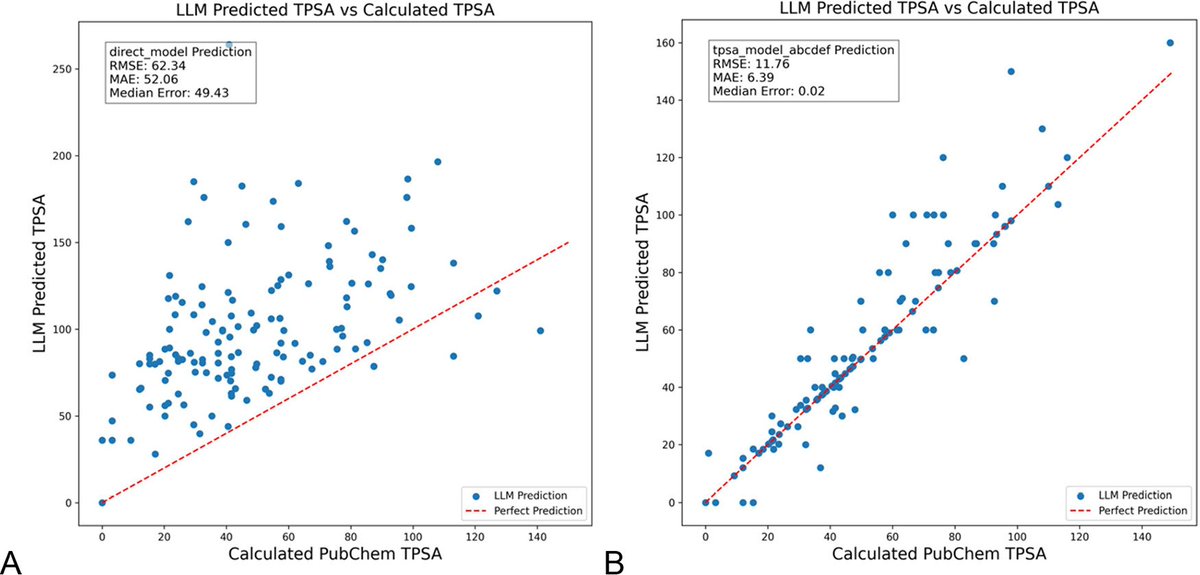
Paper proposes using graphs to quantify common sense reasoning and gain mechanistic insights: A new paper proposes a method to represent the implicit knowledge of 37 everyday activities as directed graphs, thereby generating a massive number (approx. 10^17 per activity) of common sense queries. This approach aims to overcome the limitations of existing benchmarks, which are finite and non-exhaustive, to more rigorously evaluate the common sense reasoning abilities of LLMs. The study uses graph structures to quantify common sense and enhances activation patching techniques with conjugate prompts to locate the key components responsible for reasoning within the model. (Source: menhguin)
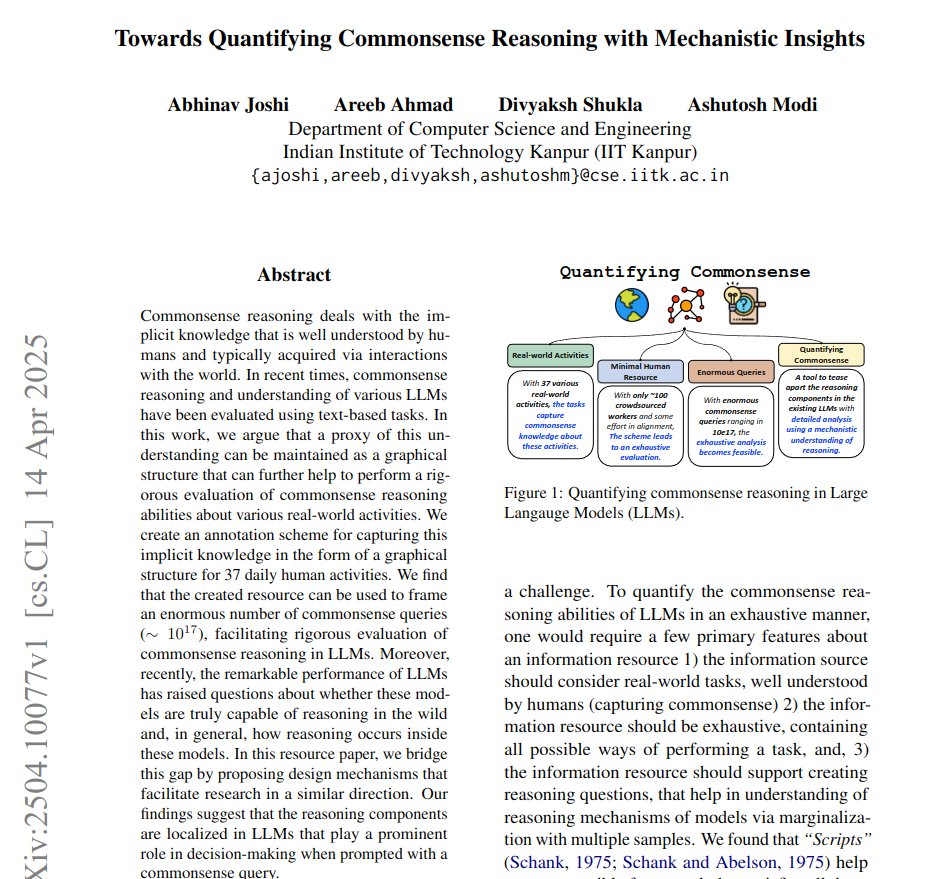
Single sample sufficient for RLVR method to significantly boost LLM math reasoning: A new paper proposes that the Reinforcement Learning from Verification feedback (RLVR) method, using just a single training sample, can significantly improve the performance of large language models on mathematical tasks. Experiments show that on the MATH500 benchmark, single-sample RLVR boosted the accuracy of Qwen2.5-Math-1.5B from 36.0% to 73.6%, and Qwen2.5-Math-7B from 51.0% to 79.2%. This finding may inspire a rethinking of the RLVR mechanism and offer new avenues for enhancing model capabilities in low-resource settings. (Source: StringChaos, _akhaliq, _akhaliq, natolambert)
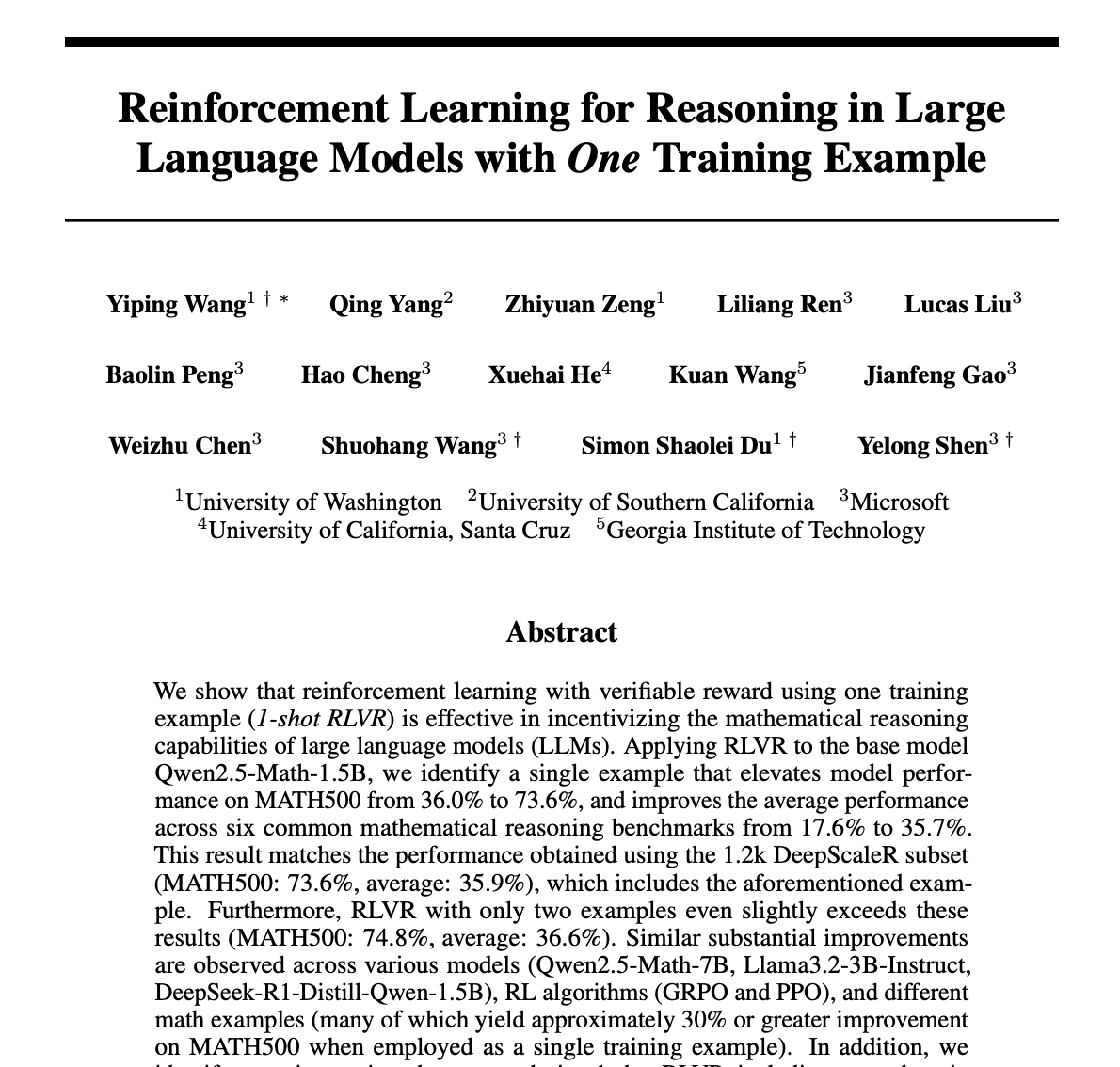
DeepLearning.AI updates “LLMs as Operating Systems: Agent Memory” course: The free short course “LLMs as Operating Systems: Agent Memory,” offered by DeepLearning.AI in collaboration with Letta, has been updated. The course explains how to build LLM Agents capable of managing long-term memory (beyond context window limits) using the MemGPT method. New content includes a pre-deployed Letta Agent service (for hands-on cloud Agent practice) and streaming output functionality (to observe the Agent’s step-by-step reasoning process), aiming to help learners build more adaptive and collaborative AI systems. (Source: DeepLearningAI)
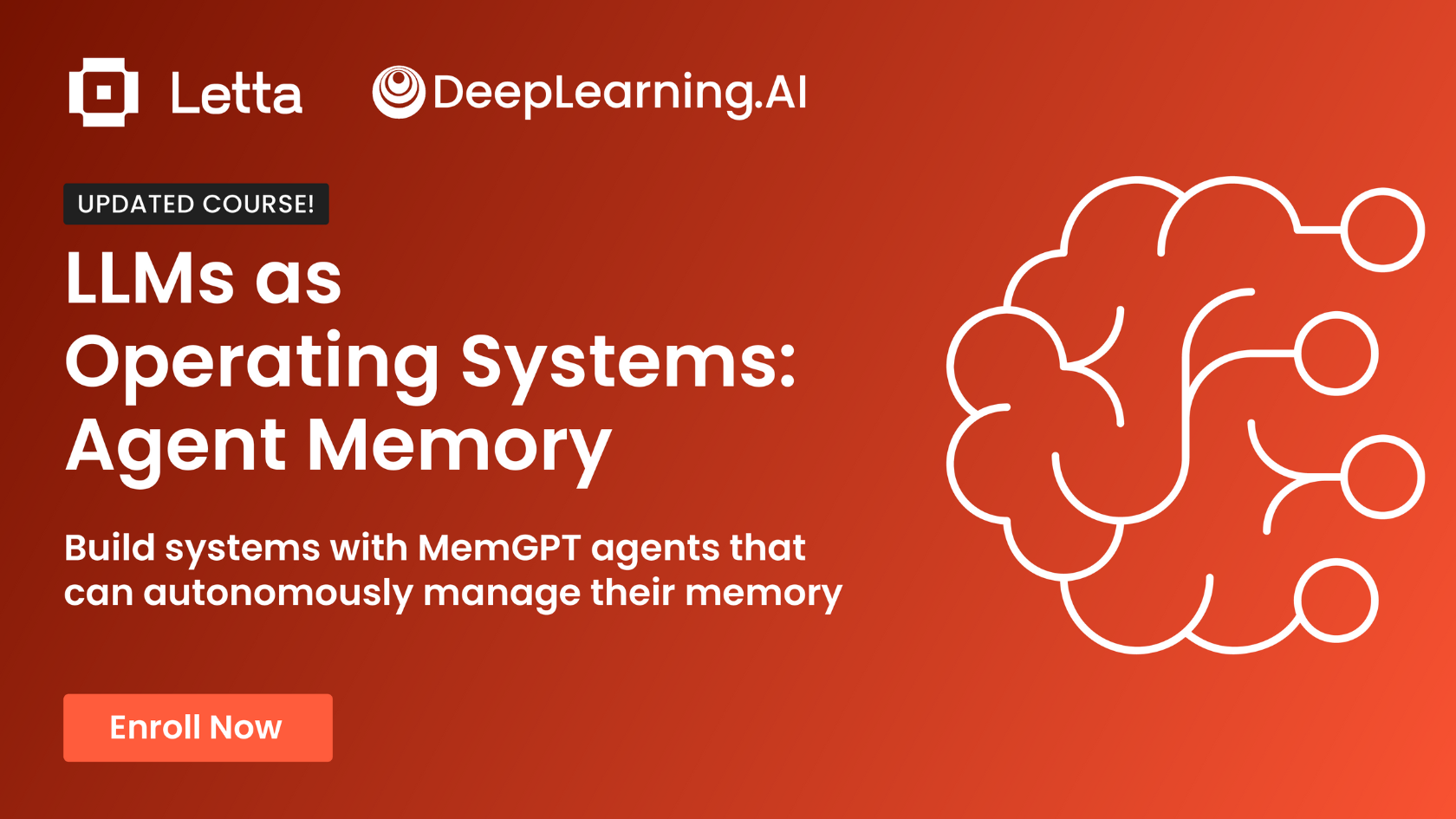
ICLR 2025 blog post: Extrapolation performance of 2D ALiBi in Vision Transformers: An ICLR 2025 blog post indicates that Vision Transformers (ViTs) using 2D Attention with Linear Biases (2D ALiBi) perform best on tasks involving extrapolation to larger image sizes on the Imagenet100 dataset. ALiBi, a relative position encoding method successful in NLP, inspired its exploration in vision. This result suggests that 2D ALiBi helps ViTs generalize better to image resolutions unseen during training. (Source: OfirPress)
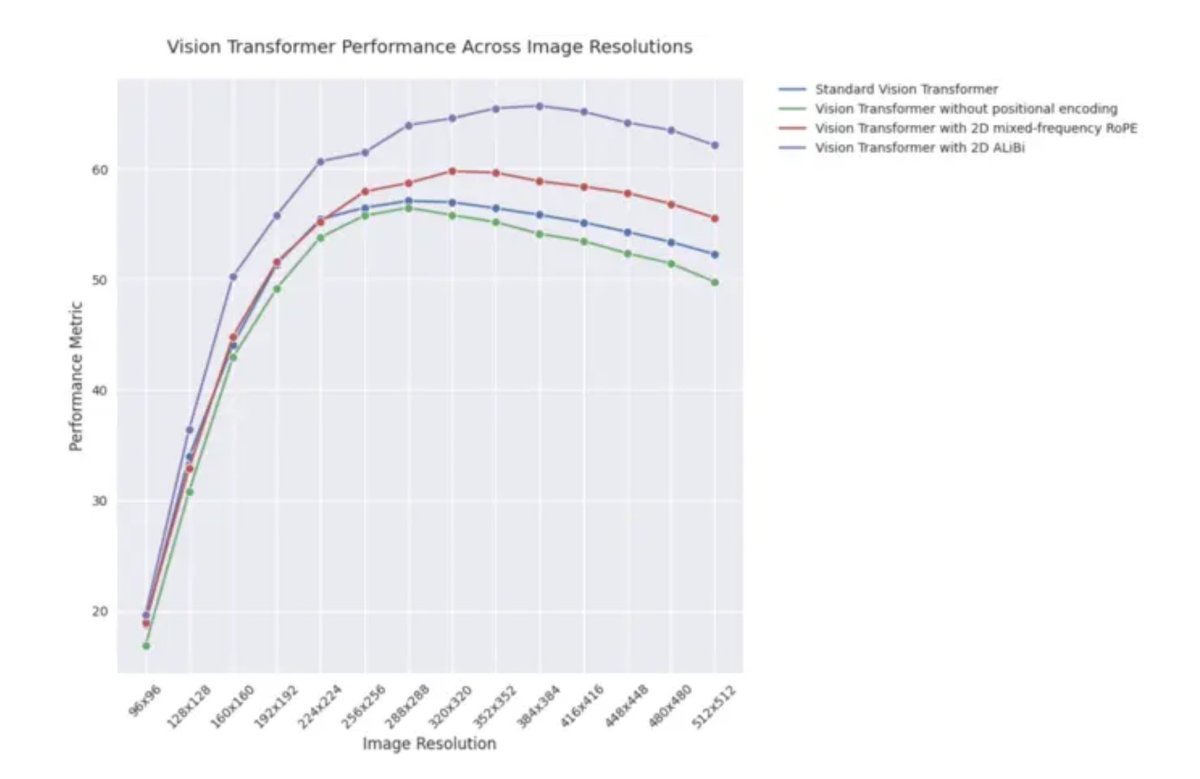
Weaviate releases RAG Cheat Sheet: Vector database company Weaviate has released a cheat sheet on Retrieval-Augmented Generation (RAG). This resource aims to provide developers with a quick reference guide, potentially covering key RAG concepts, architectures, common techniques, best practices, or frequently asked questions, to help them better understand and implement RAG systems. (Source: bobvanluijt)
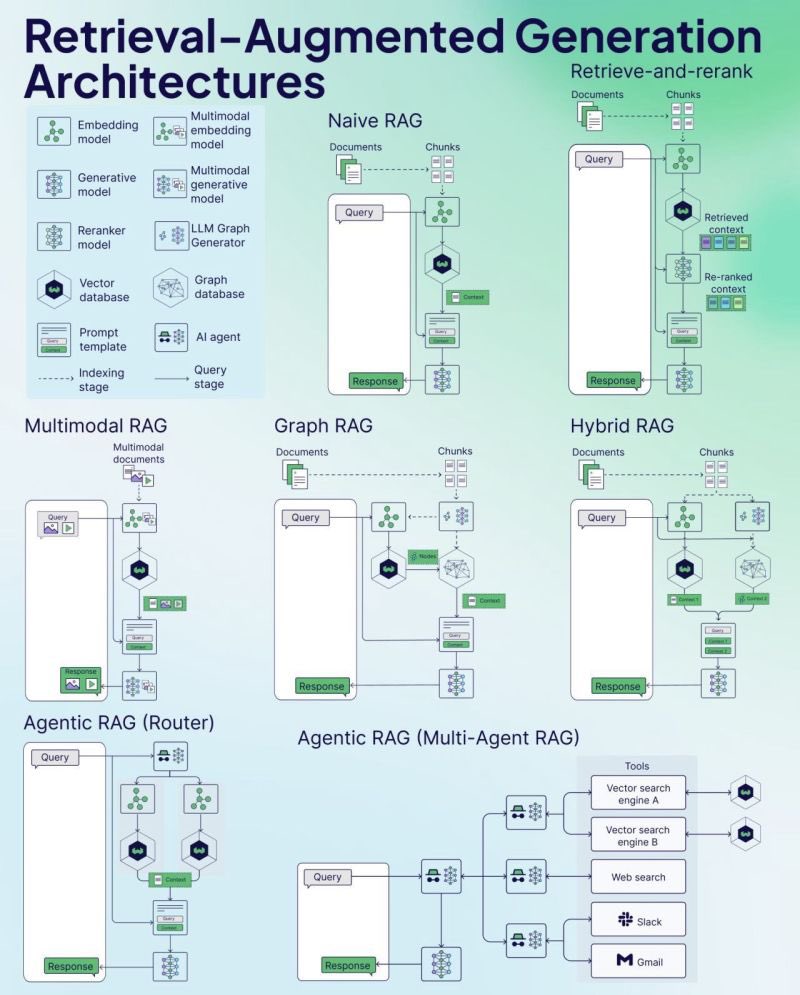
MIT research reveals structure and risks of AI Supply Chains: Researchers from MIT and other institutions have published a new paper exploring the emerging AI Supply Chains. As the process of building AI systems becomes increasingly decentralized (involving foundation model providers, fine-tuning services, data vendors, deployment platforms, etc.), the paper studies the implications of this network structure, including potential risks (like upstream failure propagation), information asymmetry, conflicts in control and optimization goals, etc. The research analyzes two case studies through theoretical and empirical analysis, emphasizing the importance of understanding and managing AI supply chains. (Source: jachiam0, aleks_madry)
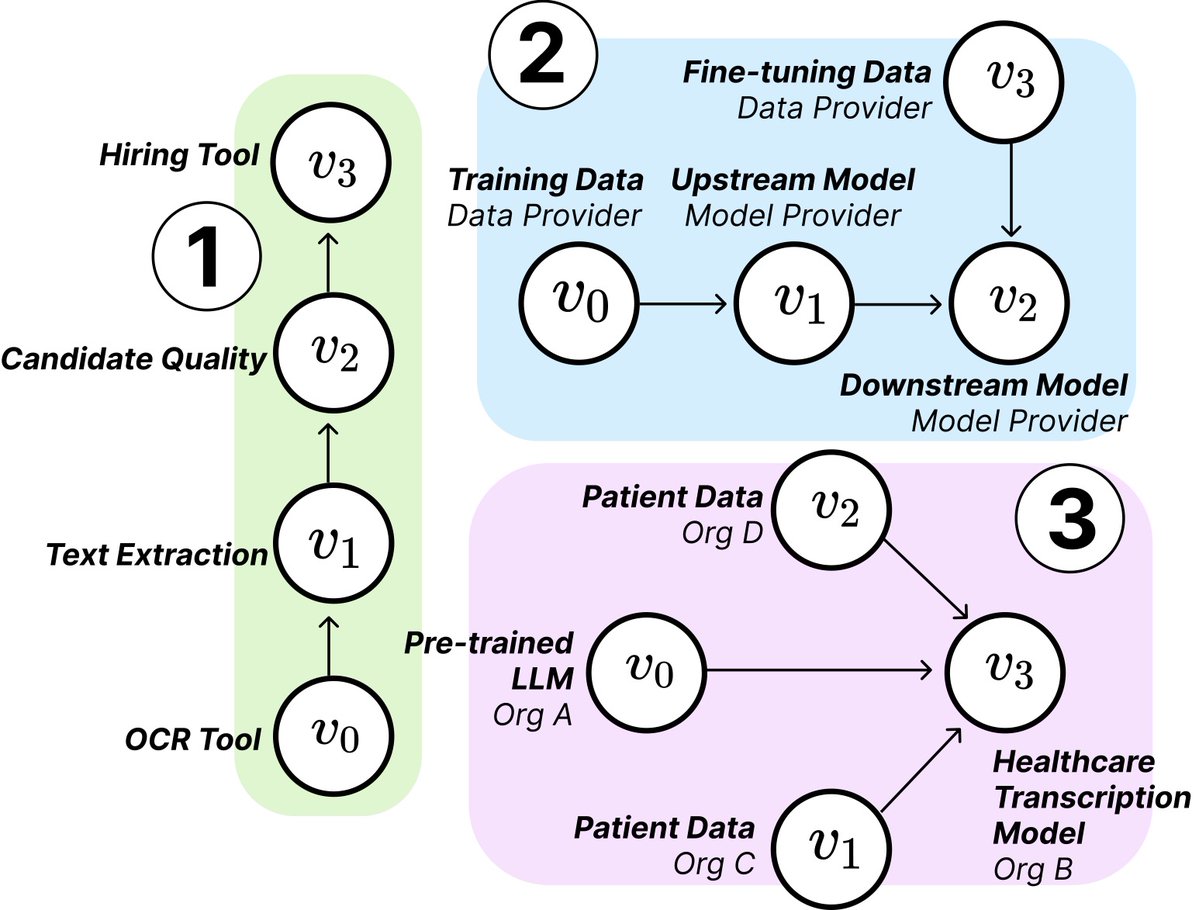
LangChain releases 5-minute introduction video for LangSmith: LangChain has released a short 5-minute video explaining the features of its commercial platform, LangSmith. The video introduces how LangSmith assists throughout the entire lifecycle of LLM application and Agent development, including observability, evaluation, and prompt engineering, aiming to help developers improve application performance. (Source: LangChainAI)
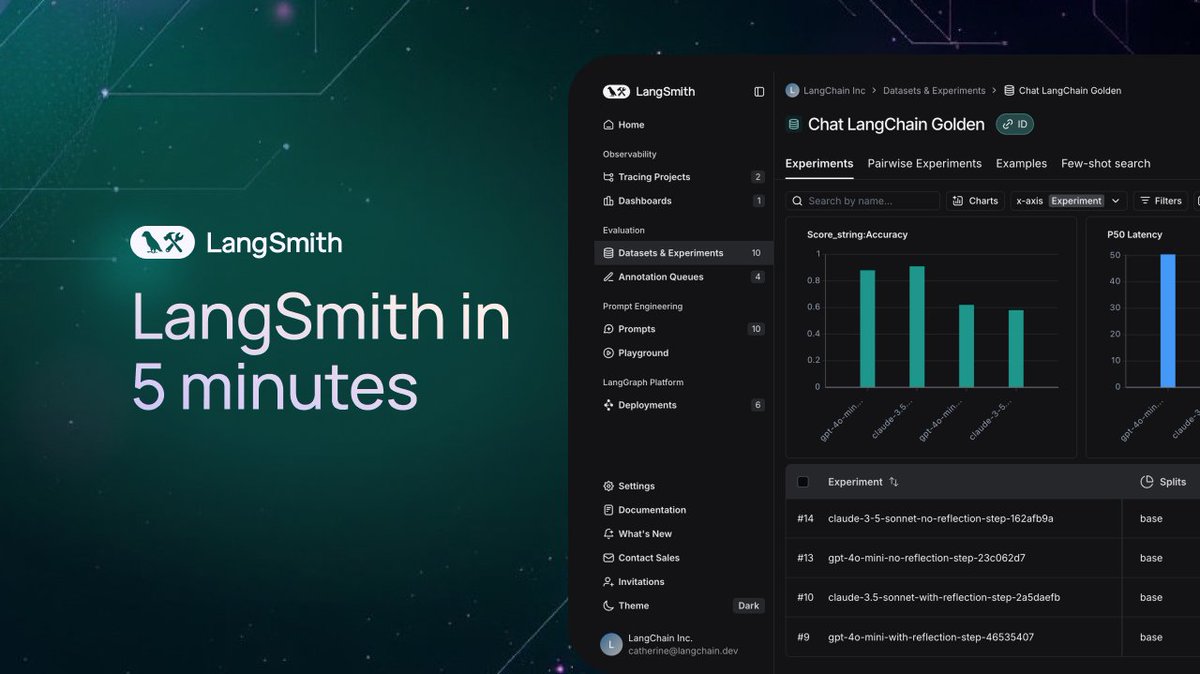
Together AI releases tutorial video on running and fine-tuning OSS models: Together AI has released a new instructional video guiding users on how to run and fine-tune open-source large models on the Together AI platform. The video likely covers steps such as selecting a model, setting up the environment, uploading data, initiating training tasks, and performing inference, aiming to lower the barrier for users to customize and deploy open-source models using their platform. (Source: togethercompute)
Paper proposes using “Sentient Agents” to evaluate LLM social cognitive abilities: A new paper introduces the SAGE (Sentient Agent as a Judge) framework, a novel evaluation method using Sentient Agents that simulate human emotional dynamics and internal reasoning to assess LLMs’ social cognitive abilities in conversation. The framework aims to test LLMs’ capacity to interpret emotions, infer hidden intentions, and respond empathetically. The study found that in 100 supportive dialogue scenarios, the Sentient Agents’ emotional ratings correlated highly with human-centric metrics (like BLRI, empathy indicators), and that socially adept LLMs do not necessarily require lengthy responses. (Source: menhguin)
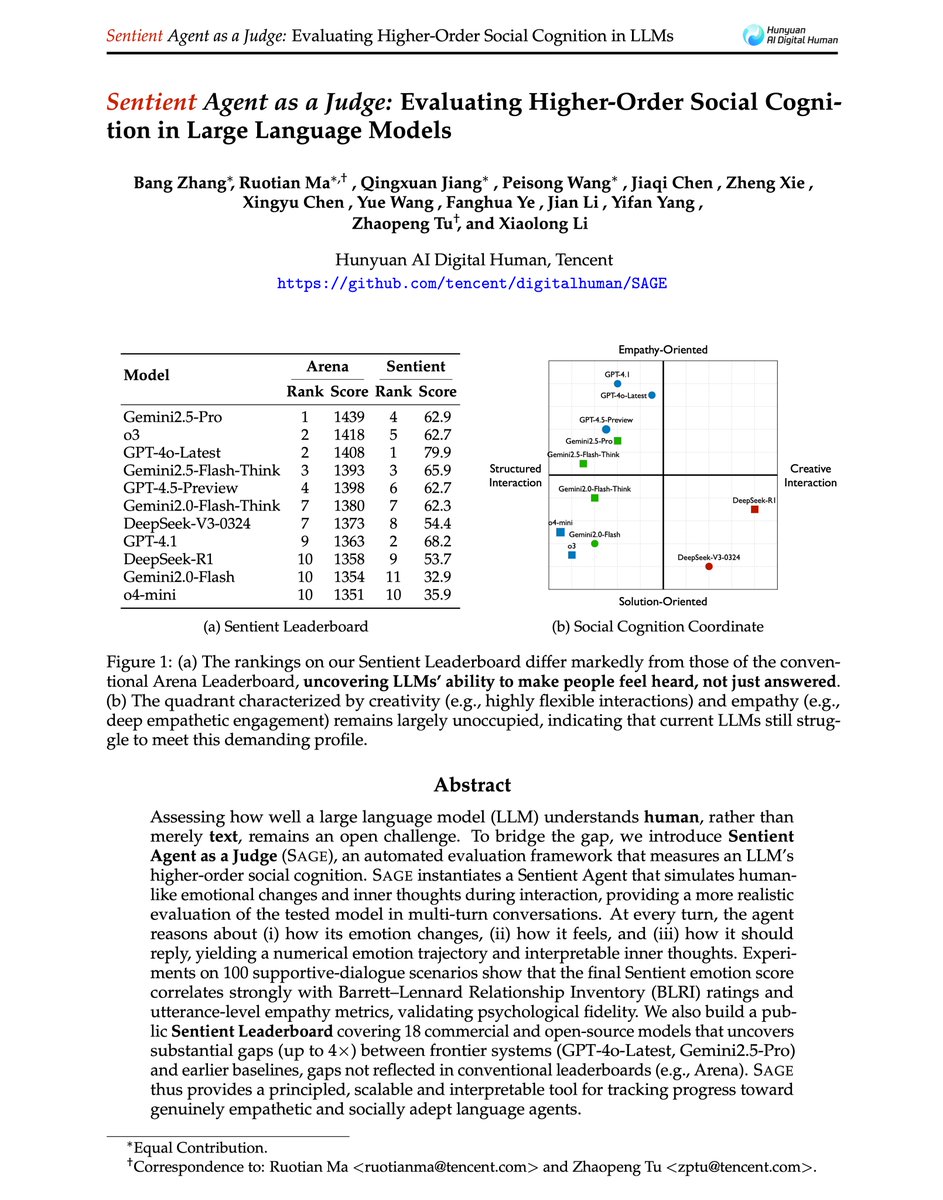
Paper explores Softpick: An alternative attention mechanism to Softmax: A preprint paper proposes Softpick, an alternative to Softmax designed to address issues like “attention sink” and large activation values in attention mechanisms by modifying Softmax. The method suggests using ReLU(x – 1) in the numerator of Softmax and abs(x – 1) in the denominator terms. Researchers believe this simple adjustment might improve some inherent problems of existing attention mechanisms while maintaining performance, especially when dealing with long sequences or scenarios requiring more stable attention distributions. (Source: sedielem)
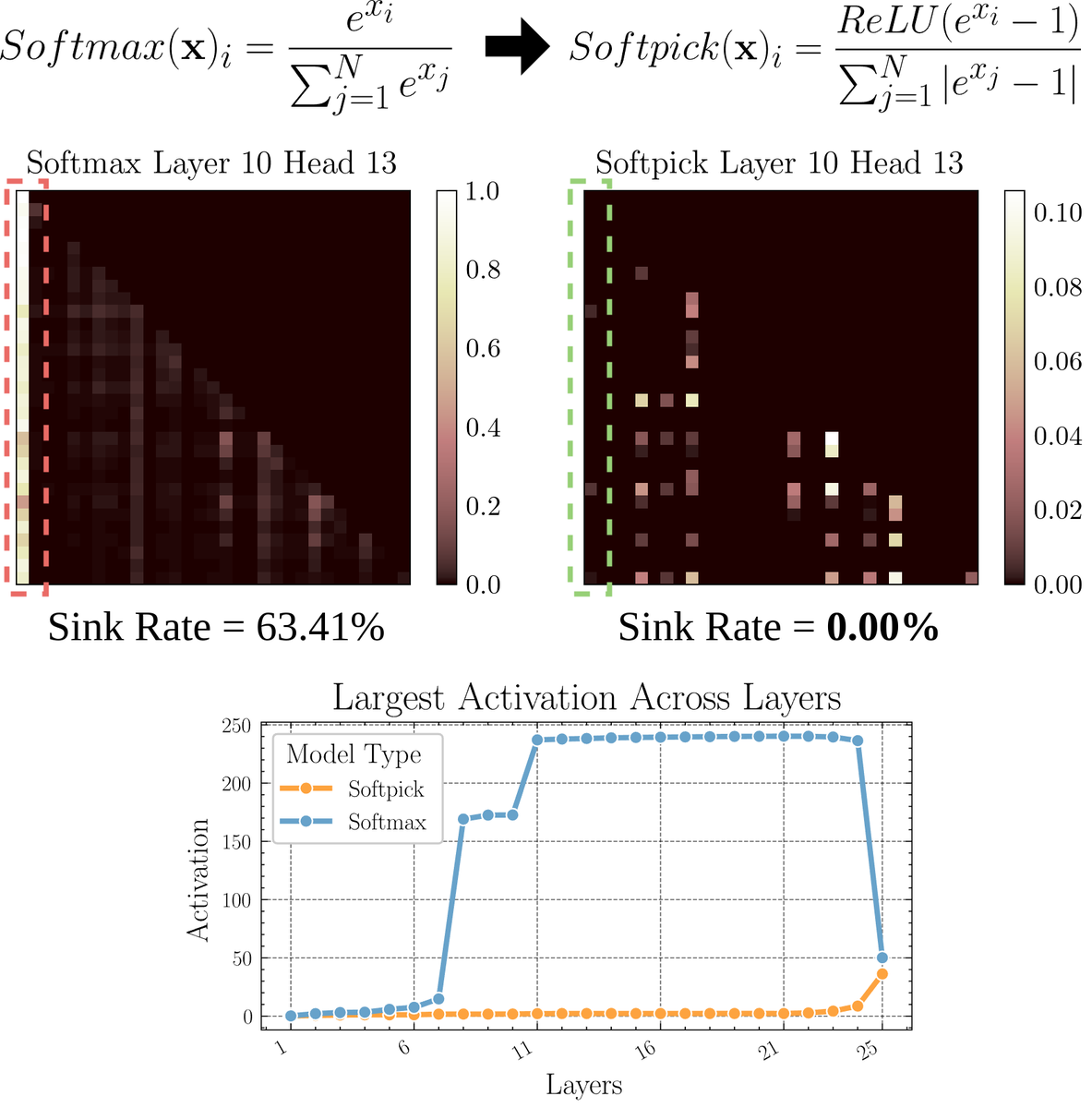
💼 Business
AI startup RogoAI completes $50 million Series B funding: RogoAI, focused on building an AI-native research platform for the financial services industry, announced the completion of a $50 million Series B funding round led by Thrive Capital, with participation from J.P. Morgan Asset Management, Tiger Global, and others. This funding will be used to accelerate RogoAI’s product development and market expansion in financial analysis and research automation. (Source: hwchase17, hwchase17)
Enterprise AI search startup Glean completes new funding round at $7 billion valuation: According to The Information, AI enterprise search startup Glean is finalizing a new funding round led by Wellington Management, valuing the company at approximately $7 billion. The company had completed a funding round at a $4.6 billion valuation just four months prior, and this significant jump in valuation reflects high market expectations for enterprise-level AI applications and knowledge management solutions. (Source: steph_palazzolo)
Groq partners with Meta to accelerate Llama API: AI inference chip company Groq announced a partnership with Meta to provide acceleration for the official Llama API. Developers will be able to run the latest Llama models (starting from Llama 4) at throughputs up to 625 tokens/second, claiming migration from OpenAI requires only 3 lines of code. This collaboration aims to offer developers high-speed, low-latency solutions for running large language models. (Source: JonathanRoss321)
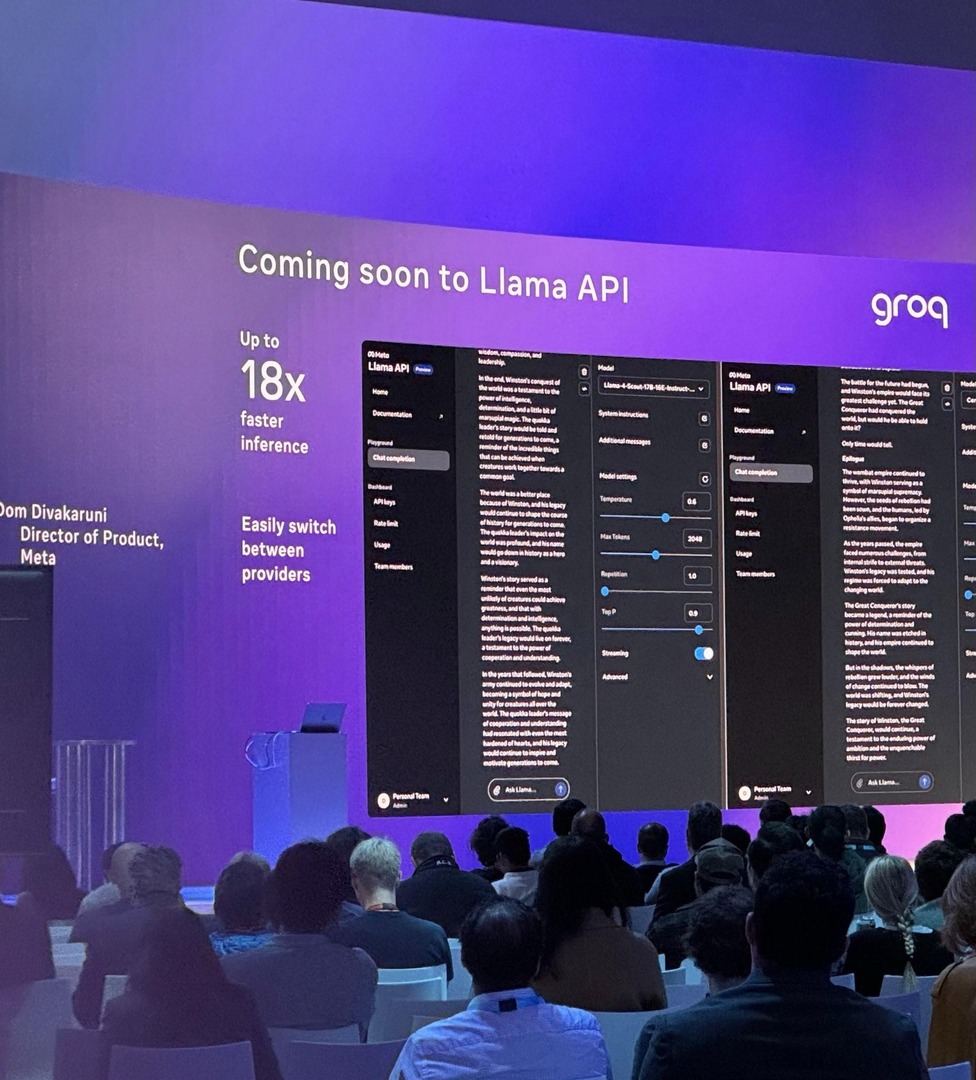
🌟 Community
Community discusses Llama4 vs. DeepSeek R1 comparison and model evaluation benchmark issues: Meta CEO Mark Zuckerberg responded in an interview to the issue of Llama4 underperforming DeepSeek R1 in the arena, arguing that open-source benchmarks are flawed, overly biased towards specific use cases, and do not truly reflect model performance in actual products. He also stated that Meta’s reasoning model has not yet been released and cannot be directly compared to R1. These remarks, combined with Cohere’s paper questioning LMArena, have sparked widespread community discussion about how to fairly evaluate LLMs, the limitations of public leaderboards, and model selection strategies. Many agree that one should not over-rely on general leaderboards but should choose models based on specific use cases, private data evaluation, and community signals. (Source: BlancheMinerva, huggingface, ClementDelangue, sarahcat21, xanderatallah, arohan, ClementDelangue, 量子位)
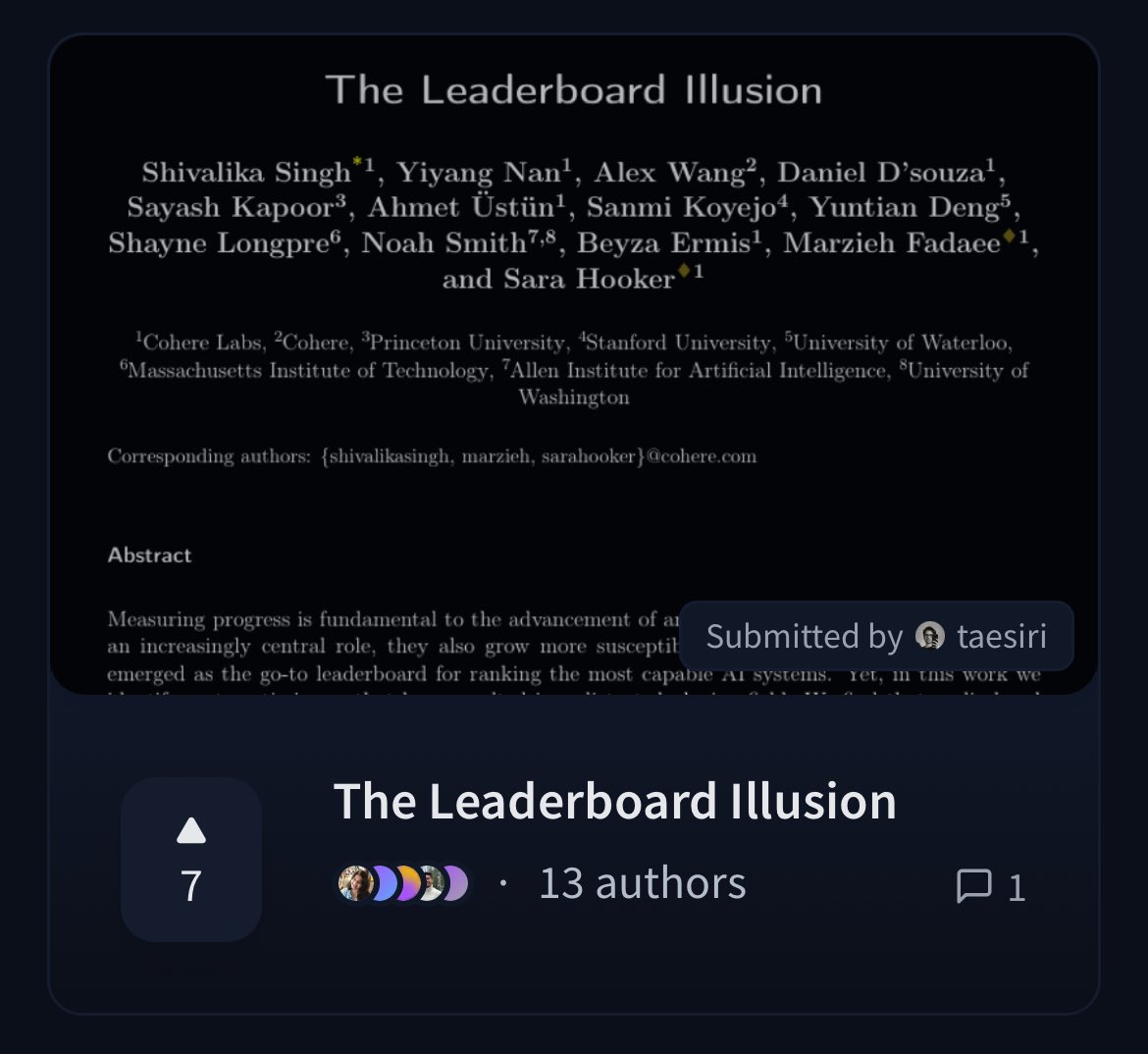
Discussion on AI replacing human jobs continues to heat up: Several posts on Reddit discuss the impact of AI on employment. A Spanish translator stated their business has significantly shrunk due to improved AI translation quality; another audio engineer switched careers due to the improved quality of AI mastering. Meanwhile, other posts discuss how AI applications in areas like medical diagnosis and tax consulting might reduce the demand for professionals. These cases have sparked discussions about whether the unemployment crisis caused by AI automation is arriving sooner than expected, and how professionals should adapt (e.g., leveraging AI for transformation, finding value that AI cannot replace). (Source: Reddit r/ArtificialInteligence, Reddit r/ArtificialInteligence)
“Iterative drift” phenomenon in AI-generated images draws attention: A Reddit user attempted to have ChatGPT repeatedly create an “exact replica” of the previously generated image. The results showed the image content and style gradually deviating from the original input with increasing iterations, eventually converging towards abstract patterns or specific motifs (like Samoan tattoos/female features). Dwayne Johnson’s example also showed a similar evolution from realism to abstraction. This phenomenon reveals the challenges current image generation models face in maintaining long-term consistency and potential biases or convergence tendencies in their internal representations. (Source: Reddit r/ChatGPT, Reddit r/ChatGPT)
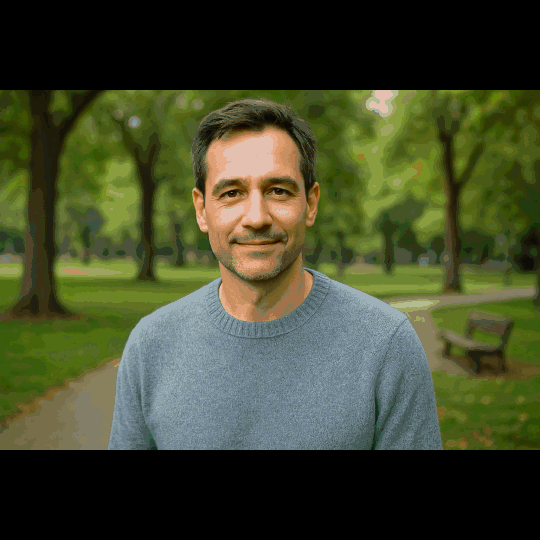
Community discusses whether AI will replace Venture Capital (VC) jobs: Marc Andreessen believes that when AI can do everything else, venture capital might be one of the last jobs done by humans because it’s more art than science, relying on taste, psychology, and tolerance for chaos. This view sparked discussion, with some calling it a “hilarious take” and questioning why early-stage investing is unique; others, drawing from their own fields (like game development), suggest this thinking might be “cope,” as people in every field tend to believe their job requires unique taste and thus cannot be replaced by AI. (Source: colin_fraser, gfodor, cto_junior, pmddomingos)
University of Zurich’s unauthorized AI persuasion experiment on Reddit sparks controversy: According to Reddit r/changemyview moderators and Reddit Lies, researchers from the University of Zurich deployed multiple AI accounts to participate in discussions on the subreddit without explicitly informing community users, testing the persuasiveness of AI-generated arguments. The study found that the AI accounts’ success rate in persuasion (receiving a “∆” mark indicating a user’s view change) far exceeded the human baseline level, and users failed to detect their AI identity. Although the experiment claimed ethics committee approval, its covert nature and potential for “manipulation” have sparked widespread ethical controversy and concerns about AI misuse. (Source: 量子位)
💡 Other
Whether learning to code is still necessary in the AI era prompts reflection: Discussions have emerged in the community regarding the value of learning to code in the age of AI. The view is that although AI’s code generation capabilities are rapidly improving and the nature of software engineering work is changing fast, learning to code remains important. Learning to code is fundamental to understanding how to effectively collaborate with AI (especially LLMs), and this human-computer collaboration skill will become a core competency across fields. Programming is the starting point for humans to “dance” with AI, and future industries will all require mastery of this collaborative model. (Source: alexalbert__, _philschmid)
Developers discuss experiences and challenges with AI-assisted programming: Developers in the community shared their experiences using AI programming tools (like Cursor, ChatGPT Desktop). Some miss the “cooling-off period” of past compilation waits, suggesting AI assistance reintroduces a similar edit/compile/debug cycle. Others pointed out that AI tools still struggle with understanding context (e.g., multi-file editing) and following instructions (e.g., avoiding specific syntax/ingredients), sometimes requiring very specific prompts to achieve desired results, and that AI-generated code still needs manual review and debugging. (Source: hrishioa, eerac, Reddit r/ChatGPT)
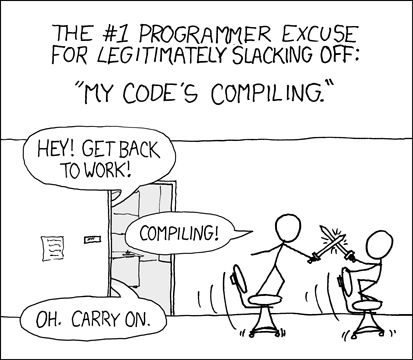
AI-driven happiness enhancement: A potential AI application direction: A Reddit post suggests that one of the ultimate applications of AI could be enhancing human happiness. The author argues that based on the facial feedback hypothesis (smiling enhances happiness) and principles of focus, AI (like Gemini 2.5 Pro) can generate high-quality guided content to help people improve their happiness levels through simple exercises (like smiling and focusing on the pleasant feeling it brings). The author shared an AI-generated report and audio, predicting the potential emergence of successful applications or “happiness tutor” bots based on this principle in the future. (Source: Reddit r/deeplearning)
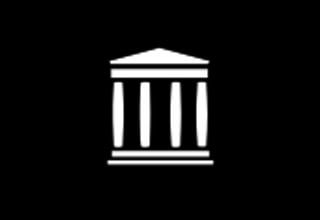