Keywords:reasoning model, AI agent, reinforcement learning, large language model, DeepSeek-R1, vision-language-navigation (VLN), DINOv2 self-supervised learning, LangGraph RAG Agent, AI chip localization, SRPO optimization method, embodied intelligence skill transfer, quantum computing governance
🔥 Focus
Inference Models Become New AI Focus, DeepSeek-R1 Shakes Industry: Following OpenAI’s release of the o-series models focusing on structured reasoning, the open-sourcing and outstanding performance (especially in math and code) of DeepSeek-R1 signal a new phase in large model competition. Industry focus is shifting from pre-training parameter scale to enhancing reasoning abilities through reinforcement learning. Major domestic players like Baidu (Wenxin X1), Alibaba (Tongyi Qianwen Qwen-QwQ-32B), Tencent (Hunyuan T1), ByteDance (Doubao 1.5), and iFlytek (Spark X1) are rapidly following suit, releasing their own inference models, forming a new landscape of domestic inference models challenging OpenAI. This shift emphasizes the importance of models’ deep thinking, planning, analysis, and tool-calling capabilities, indicating that the implementation of applications like Agents will increasingly rely on powerful foundational inference models. (Source: 国产六大推理模型激战OpenAI?, “AI寒武纪”爆发至今,五类新物种登上历史舞台)
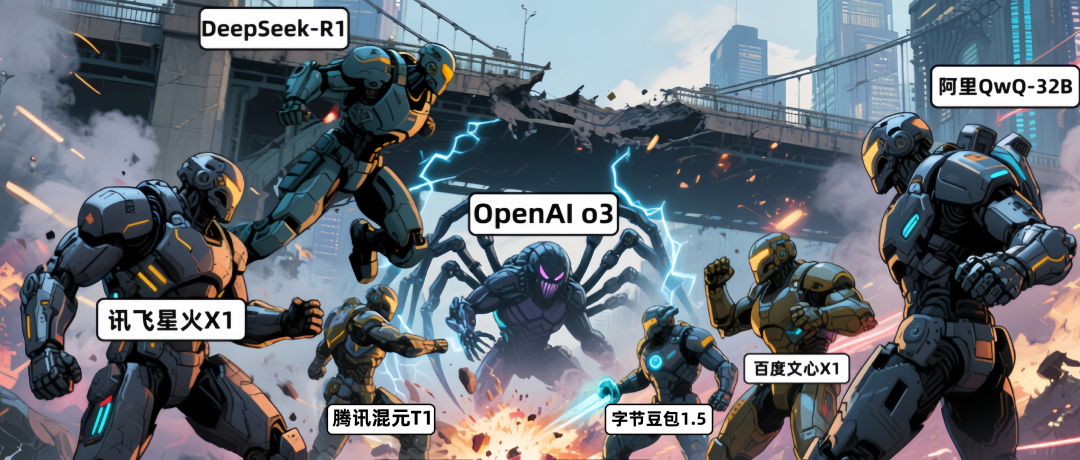
AI Shopping App Nate Exposed for Fraud, Founder Accused of Defrauding $40M in Investment: The US Department of Justice has accused Albert Saniger, founder of the AI shopping app Nate, of defrauding investors by falsely promoting its AI technology. Nate claimed to use AI to simplify the cross-platform shopping process, enabling one-click checkout, but was allegedly found to employ hundreds of workers in the Philippines to manually process orders, passing off “human labor” as “intelligence.” The incident exposes potential bubbles and fraud risks in the AI startup boom and sparks discussion about Silicon Valley’s “Fake it till you make it” culture, highlighting the line between exaggeration and deception. This case also reflects the technical feasibility challenges of certain AI application concepts before AI technology (especially large models) matures. (Source: AI购物竟是人工驱动,硅谷创投圈又玩出新花活)
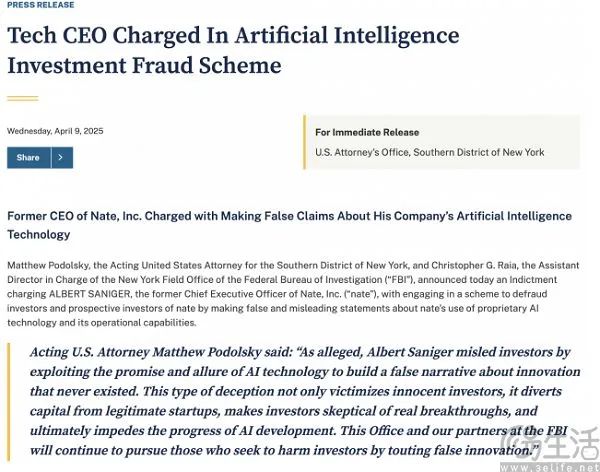
AI Integrates into Workflows, Reshaping Workplace Value and Management Models: AI is moving from concept to practice, deeply integrating into corporate operations and employees’ daily work. Alibaba Cloud uses large models and data governance to create an “Organizational Operation Management Cockpit,” optimizing OKR/CRD processes; Deloitte China aims to cultivate ten thousand AI talents to meet the needs of intelligence-intensive organizations; Yum China deploys AI tools down to the restaurant manager level. This indicates that AI is not just an efficiency tool but is reshaping the nature of work, organizational structures, and talent requirements. Repetitive, standardized tasks are being replaced by AI, demanding higher levels of creativity, critical thinking, decision-making, and AI collaboration skills (AI adaptability) from employees. Corporate management needs to shift from supervision to empowerment, establishing new paradigms and trust mechanisms for human-AI collaboration. (Source: 当AI来和我做同事:重构职场价值坐标系, 重塑工作:AI时代的组织进化与管理革命)
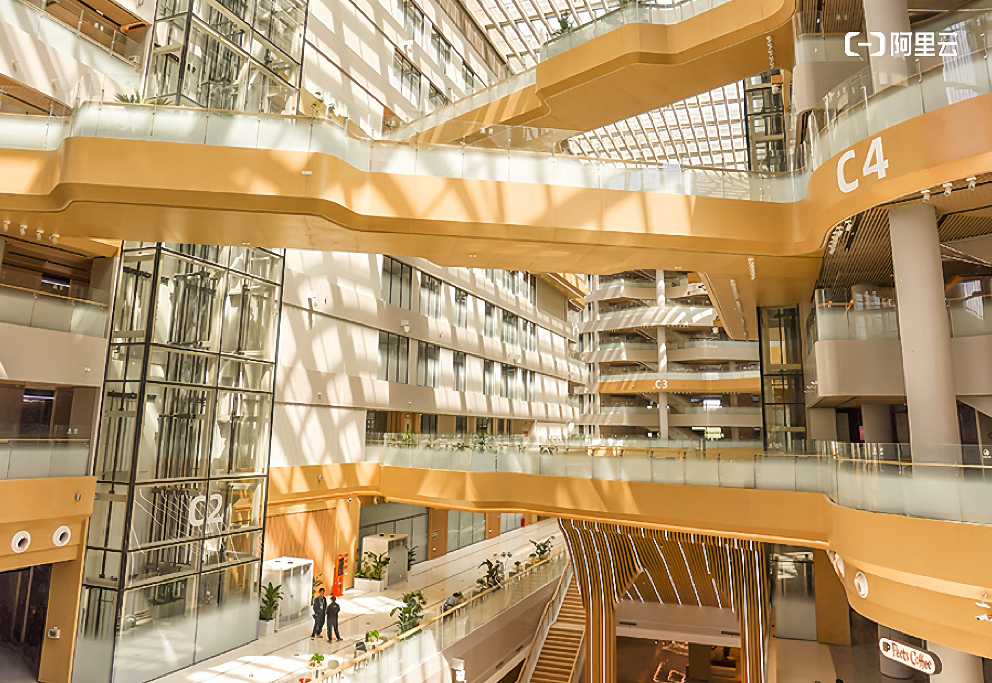
🎯 Trends
DINOv2 Self-Supervised Visual Model Introduces Register Mechanism: Meta AI Research has updated its DINOv2 self-supervised learning method and models. According to the paper “Vision Transformers Need Registers,” the new version incorporates a “registers” mechanism. DINOv2 aims to learn robust visual features without supervision, which can be directly used for various computer vision tasks (like classification, segmentation, depth estimation) and perform well across domains without fine-tuning. This update may further enhance the model’s performance and feature quality. (Source: facebookresearch/dinov2 – GitHub Trending (all/daily))
Reinforcement Learning (RL) Becomes Key Path for LLM Post-Training and Capability Enhancement: Scholars like David Silver and Richard Sutton point out that AI is entering an “era of experience,” where RL plays a core role in the post-training phase of LLMs. By learning reward models from human feedback (RLHF), demonstrations, or rules (Inverse RL), RL endows LLMs with capabilities for continuous optimization, exploration, and generalization that surpass imitation learning (like SFT). Especially in reasoning tasks (e.g., math, code), RL helps models discover more effective solution patterns (like long chains of thought), breaking the limitations of data-driven methods. This marks a shift in LLM development from relying on static data to dynamic learning through interaction and feedback. (Source: 被《经验时代》刷屏之后,剑桥博士长文讲述RL破局之路)
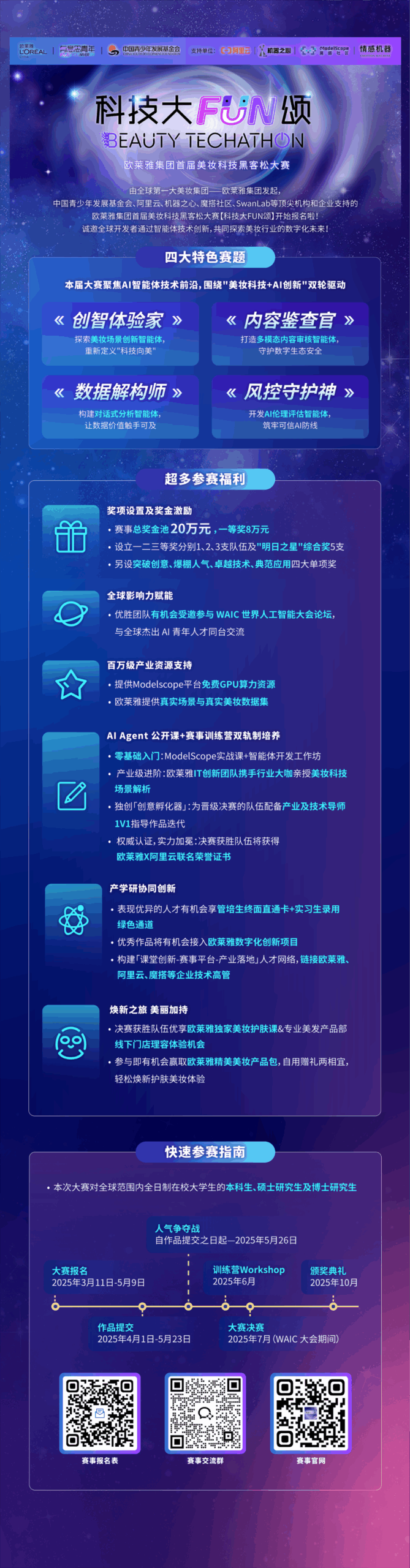
Vision-Language-Navigation (VLN) Remains a Significant Challenge for Embodied Intelligence: Associate Professor Qi Wu from the University of Adelaide points out that although Manipulation tasks are hot in the field of embodied intelligence, Vision-Language-Navigation (VLN), as a key component of Vision-Language-Action (VLA), still faces numerous challenges in unstructured, dynamic environments (especially home scenarios) and is far from being fully solved. Navigation is fundamental for robots to perform subsequent tasks. Current bottlenecks in VLN include the lack of high-quality data (simulators, 3D environments, task data), the Sim2Real transfer gap, and engineering difficulties in efficient edge deployment. (Source: 阿德莱德大学吴琦:VLN 仍是 VLA 的未竟之战丨具身先锋十人谈)
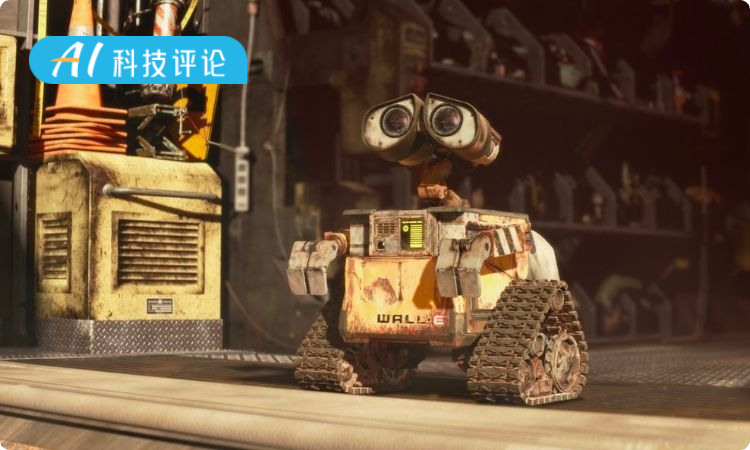
AI Shows Clear Commercialization Path in Advertising and Marketing: Compared to other AI application scenarios, the commercialization of AI technology in advertising and marketing appears more defined and rapid. By leveraging AI for data analysis, user profiling, targeted advertising, and automated marketing, companies like Applovin Corp and Zeta Global have successfully transformed the advertising ecosystem, enhancing efficiency and return on investment (ROI). This suggests that applications capable of quickly generating business value are more likely to gain market favor in the AI wave, with advertising and marketing being typical examples. (Source: “AI寒武纪”爆发至今,五类新物种登上历史舞台)
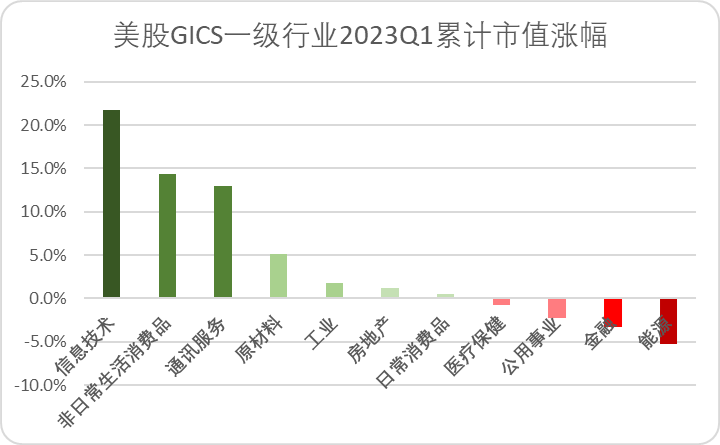
AI Chip Supply Chain Tension and Domestic Production Trend: Sino-US tech competition intensifies, with the US tightening export controls on AI chips to China (especially high-end models like Nvidia H20). Reports indicate that several Chinese tech companies (e.g., ByteDance, Alibaba, Tencent) stockpiled large quantities of Nvidia chips before the ban took effect to maintain their AI R&D and deployment capabilities. Concurrently, to address supply chain risks and the “chokehold” problem, a full-stack domestic AI technology path is gaining more attention. For instance, iFlytek trains and deploys its Spark large model using domestic computing power like Huawei Ascend, which could become a significant trend for future AI development in China. (Source: 国产六大推理模型激战OpenAI?, Reddit r/artificial, Reddit r/ArtificialInteligence)
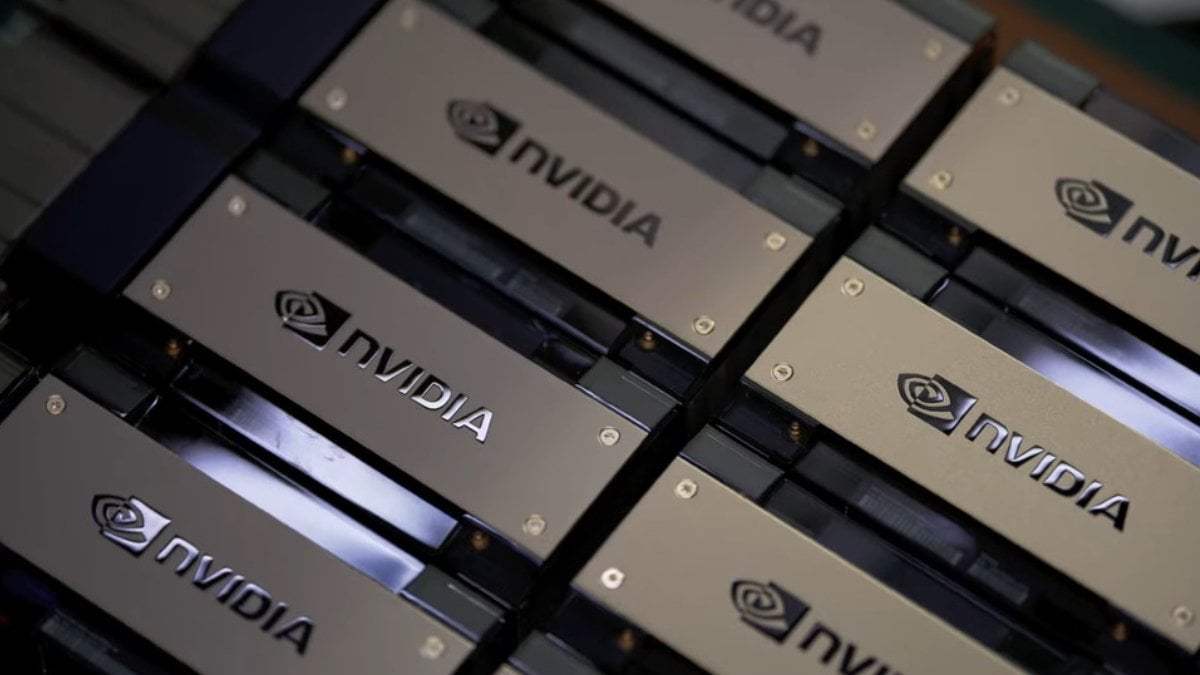
🧰 Tools
Suna: Open-Source Generalist AI Agent Platform: Kortix AI has launched Suna, an open-source Generalist AI Agent. Users can interact with Suna via natural language conversation to get assistance with various real-world tasks, including web research, data analysis, browser automation (web navigation, data extraction), file management (document creation/editing), web crawling, extended search, command-line execution, website deployment, and integration with various APIs and services. Suna aims to be a user’s digital companion, automating complex workflows. (Source: kortix-ai/suna – GitHub Trending (all/daily))

Leaked System Prompts Repository Collects Internal Prompts from Major Models: A popular repository named leaked-system-prompts
has emerged on GitHub, collecting and publicizing internal System Prompts from multiple mainstream AI models. These prompts reveal the instructions, rules, role settings, and safety constraints the models are designed to follow. The repository includes leaked prompts from numerous models such as Anthropic Claude series (2.0, 2.1, 3 Haiku/Opus/Sonnet, 3.5 Sonnet, 3.7 Sonnet), Google Gemini 1.5, OpenAI ChatGPT (various versions including 4o), DALL-E 3, Microsoft Copilot, xAI Grok (various versions), providing researchers and developers a window into the internal workings of these models. (Source: jujumilk3/leaked-system-prompts – GitHub Trending (all/daily))
WAN Video Generation Platform Launches Paid Acceleration Service: The overseas version of the AI video generation platform WAN (WAN.Video) has announced its commercialization phase by introducing paid options. All users can still enjoy unlimited free video generation (Relax mode) but will need to wait in a queue. Paid users, however, get priority generation service without queuing, allowing them to receive video results faster. This provides an accelerated channel for users needing high efficiency or for commercial purposes. (Source: op7418)
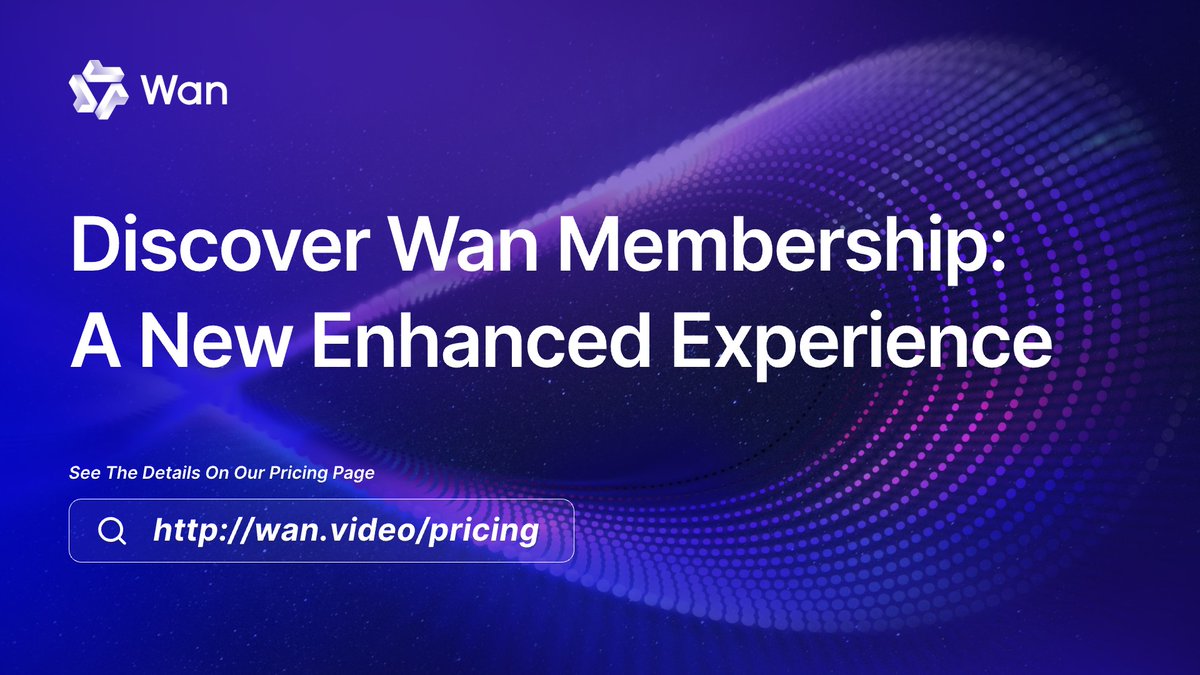
Dia TTS Model Lands on Hugging Face API: Users can now directly call the Dia 1.6B Text-to-Speech (TTS) model API via the Hugging Face platform, powered by FAL AI. Developers can integrate high-quality speech synthesis functionality with just a few lines of code. This integration lowers the barrier to using advanced TTS models, making it convenient for developers to quickly add voice capabilities to their applications. (Source: huggingface)
ModernBERT Classifier Model Integrated with vLLM for Fast Inference: ModernBERT models can now run on the vLLM framework, significantly boosting inference speed. It is claimed to be fast enough to process over 200,000 arXiv papers in minutes. This integration enables hundreds of ModernBERT models hosted on the Hugging Face Hub to be deployed and applied to text classification tasks more rapidly. (Source: huggingface)
Trackers: High-Performance Python Object Tracking Library: Roboflow has open-sourced a Python library called Trackers, focusing on object tracking tasks. Designed to be modular, the library supports multiple tracking algorithms and integrates easily with popular machine learning libraries like Ultralytics and Transformers. It boasts powerful performance, capable of tracking numerous objects simultaneously, successfully tracking over 269 eggs in a demo video. (Source: karminski3)
Unsloth Releases Dynamic v2.0 GGUF Quantization Technology and Models: Unsloth has introduced its new Dynamic v2.0 quantization technology, specifically designed for GGUF format models. This quantized version reportedly outperforms previous versions in MMLU and KL Divergence evaluations and fixes the Llama-4 RoPE implementation issue in Llama.cpp. Unsloth has used this technology to release new quantized models for DeepSeek-R1 and DeepSeek-V3-0324 for community use. (Source: karminski3)
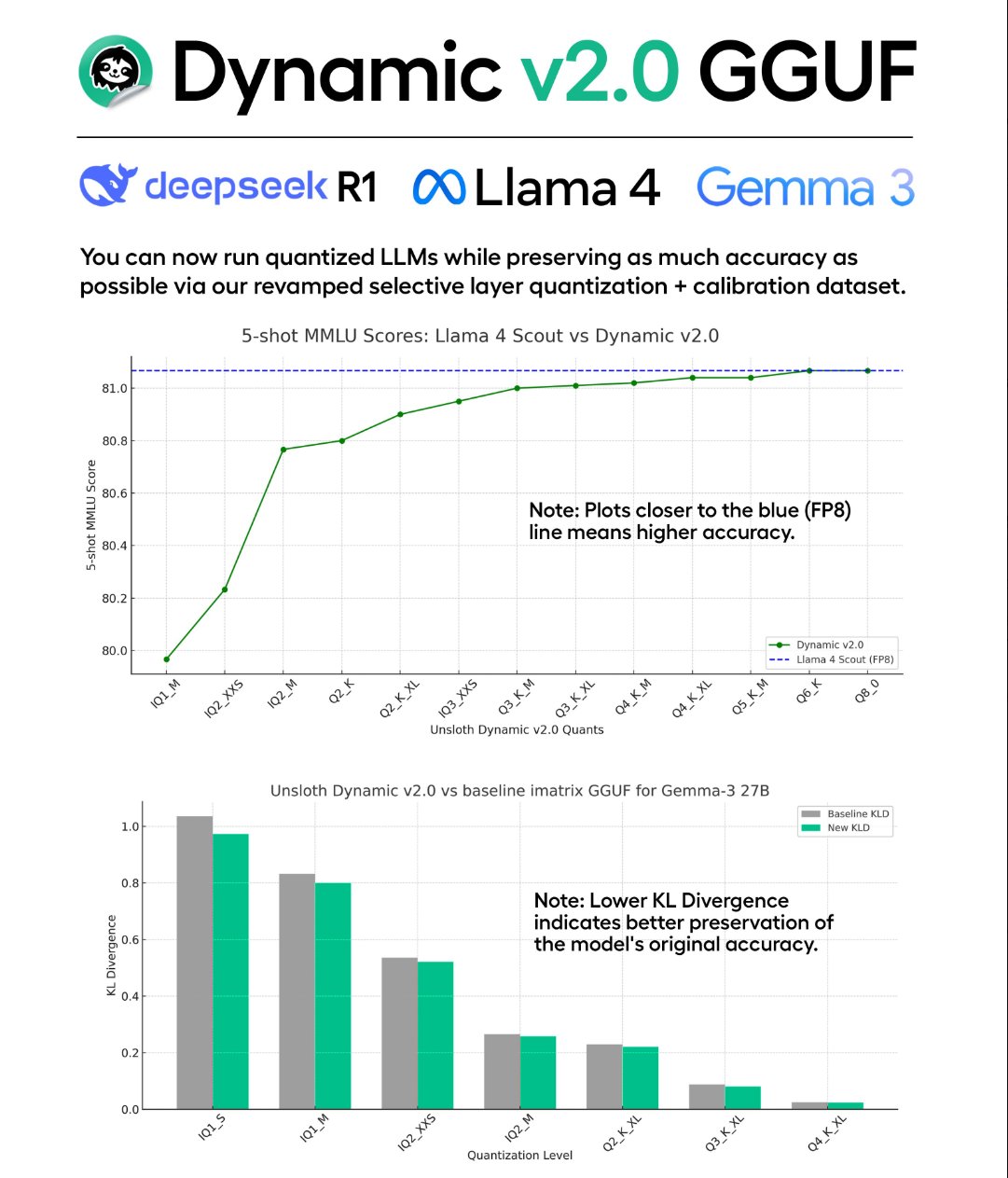
Perplexity iOS Voice Assistant Integrates System Functions: Perplexity’s iOS app has updated its voice assistant feature, enabling it to invoke more system-level actions. Users can now use voice commands to have the Perplexity assistant book restaurants, navigate using Apple Maps, create reminders, search and play Apple Music or podcasts, and hail rides. This brings the Perplexity assistant closer in functionality to native system assistants like Siri, enhancing its utility. (Source: AravSrinivas)
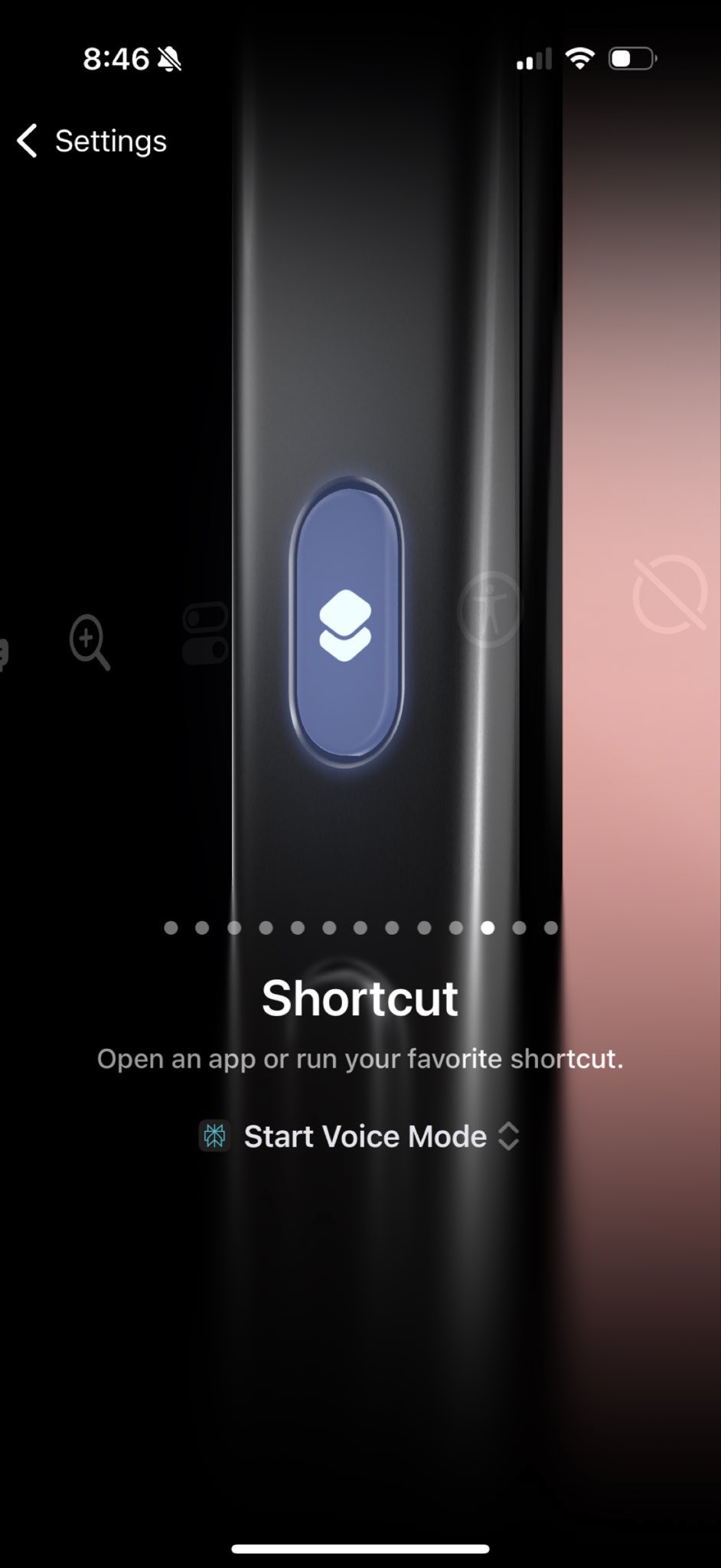
VS Code MCP Server Extension Released, Connecting Claude to Local Dev Environment: Developer Juehang Qin has released a VS Code extension that turns VS Code into an MCP (Model Context Protocol) server. This allows AI assistants like Claude to directly access and manipulate the user’s currently open workspace in VS Code, including reading/writing files and viewing code diagnostics (like errors and warnings). When the user switches projects, the extension automatically exposes the new workspace, facilitating seamless collaboration for the AI assistant across different projects. (Source: Reddit r/ClaudeAI)
📚 Learning
DINOv2: Meta Open-Sources Self-Supervised Visual Feature Learning Method: Meta AI Research has open-sourced the DINOv2 project, including PyTorch code and pre-trained models. DINOv2 is a self-supervised learning method designed to learn powerful, general-purpose visual features that excel on various computer vision tasks (e.g., image classification, semantic segmentation, depth estimation) without requiring fine-tuning for downstream tasks. The project provides detailed documentation, model download links, and related papers, serving as a crucial resource for research and application of self-supervised visual learning. (Source: facebookresearch/dinov2 – GitHub Trending (all/daily))
HD-EPIC: High-Detail First-Person Video Dataset Released: Researchers have introduced the HD-EPIC dataset, containing 41 hours of first-person video recorded in real kitchen environments. A key feature of this dataset is its extremely detailed multi-modal annotations, covering recipe steps, ingredient nutritional information (via weight records), fine-grained action descriptions (content, manner, reason), 3D scene digital twins, object movement trajectories (2D/3D), hand/object masks, gaze tracking, and object-scene interactions. The dataset aims to provide a high-quality benchmark for first-person vision understanding, embodied intelligence, and human-computer interaction research. (Source: CVPR 2025 | HD-EPIC定义第一人称视觉新标准:多模态标注精度碾压现有基准)

SRPO: Optimization Method to Address Cross-Domain RL Training for LLM Reasoning: Addressing performance bottlenecks and efficiency issues encountered when using RL methods like GRPO to train LLMs on mixed math and code tasks, the Kuaishou Kwaipilot team proposed SRPO (Two-Stage History Resampling Policy Optimization). This method uses math data in the first stage to stimulate deep thinking, introduces code data in the second stage to develop programmatic thinking, and incorporates history resampling techniques to solve the zero variance reward signal problem. Experiments show that SRPO surpasses DeepSeek-R1-Zero-Qwen-32B on AIME24 and LiveCodeBench with only 10% of the training steps, offering an efficient path for enhancing cross-domain reasoning capabilities. (Source: DeepSeek-R1-Zero被“轻松复现”?10%训练步数实现数学代码双领域对齐)
TTRL: Test-Time Reinforcement Learning Without Labeled Data: Researchers from Tsinghua University and Shanghai AI Lab proposed TTRL (Test-Time Reinforcement Learning), enabling LLMs to perform reinforcement learning during the testing phase without human annotations. The method utilizes the model’s own multiple sampling outputs to generate pseudo-labels and reward signals through techniques like majority voting, driving the model to self-evolve and adapt to new data or tasks. Experiments demonstrate that TTRL significantly improves model performance on target tasks, even approaching the effectiveness of supervised training, offering a new approach to tackling RL challenges in unsupervised settings. (Source: TTS和TTT已过时?TTRL横空出世,推理模型摆脱「标注数据」依赖,性能暴涨)
SeekWorld: Geolocation Inference Task and Model Simulating o3 Visual Cue Tracking: To enhance the visual reasoning capabilities of Multimodal Large Language Models (MLLMs), particularly simulating the ability of OpenAI’s o3 model to dynamically perceive and manipulate images (visual cue tracking) during reasoning, researchers proposed the SeekWorld geolocation inference task (inferring shooting location from an image). A dataset was constructed around this task, and the SeekWord-7B model was trained using reinforcement learning. This model surpasses Qwen-VL, Doubao Vision Pro, GPT-4o, and others in geolocation inference. The project has open-sourced the model, dataset, and an online demo. (Source: 一张图片找出你在哪?o3-like 7B模型玩网络迷踪超越一流开闭源模型!)
ManipTrans: Transferring Manipulation Skills from Human Hands to Dexterous Hands: Researchers from the Beijing Academy of Artificial Intelligence, Tsinghua University, and Peking University proposed the ManipTrans method for efficiently transferring human bimanual manipulation skills to robotic dexterous hands in simulation environments. The method employs a two-stage strategy: first imitating human hand movements via a general trajectory imitator, then fine-tuning using residual learning and physical interaction constraints. Based on this method, the team released a large-scale dexterous hand manipulation dataset, DexManipNet, containing complex task sequences like bottle cap screwing, writing, scooping, and opening toothpaste caps, and validated the feasibility of real-world deployment. (Source: 机器人也会挤牙膏?ManipTrans:高效迁移人类双手操作技能至灵巧手)
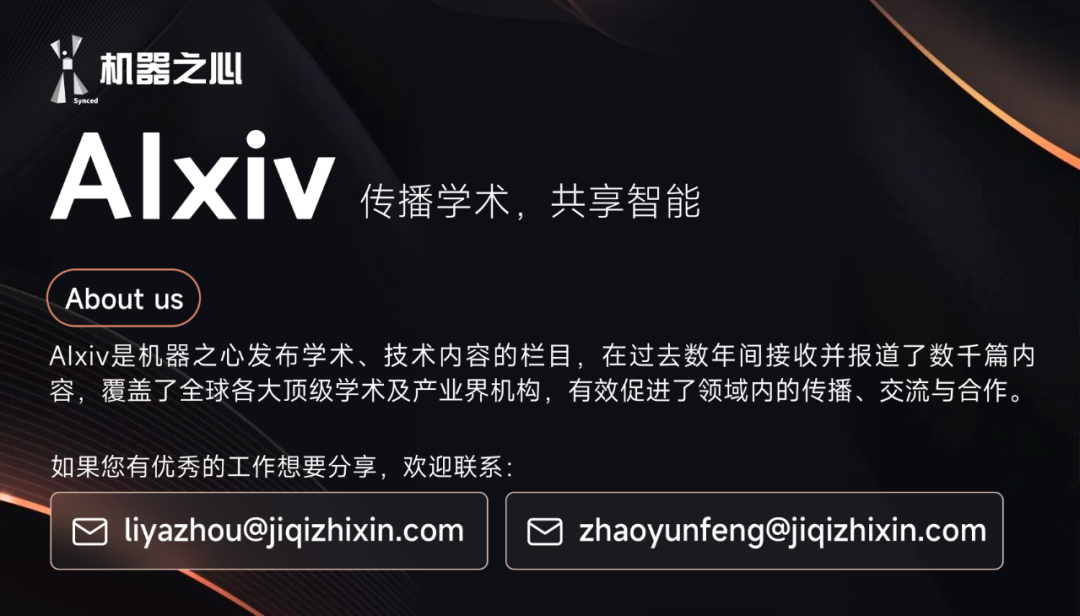
LangGraph Tutorial: Creating a RAG Agent with Reflection Mechanism: LangChain released a video tutorial detailing how to build a Retrieval-Augmented Generation (RAG) Agent with a reflection capability using the LangGraph framework. The core idea is to add an evaluation node to the RAG process, allowing the Agent to review the retrieved information before generating the final answer, assess its relevance and quality, and decide whether to re-retrieve, refine the query, or generate the answer directly based on the evaluation. This effectively filters noise and improves QA performance. (Source: LangChainAI)
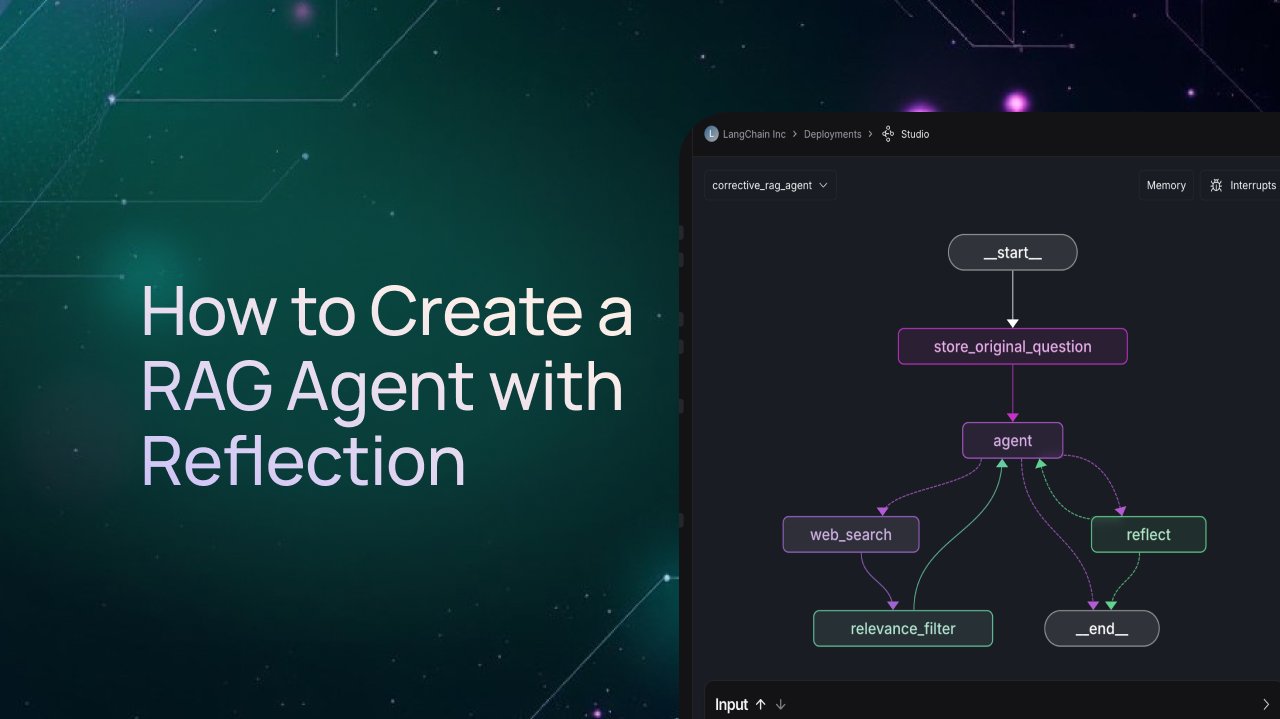
Arena-Hard-v2.0: A More Stringent Benchmark for Large Model Evaluation: LMSYS Org has updated the Arena-Hard evaluation benchmark to version 2.0. The new version is based on 500 more challenging prompts submitted by LMArena users, employs more powerful automated judge models (Gemini-2.5 & GPT-4.1), supports over 30 languages, and adds assessment of creative writing ability. It aims to provide a more difficult and comprehensive platform to differentiate the performance of top large models. (Source: lmarena_ai)
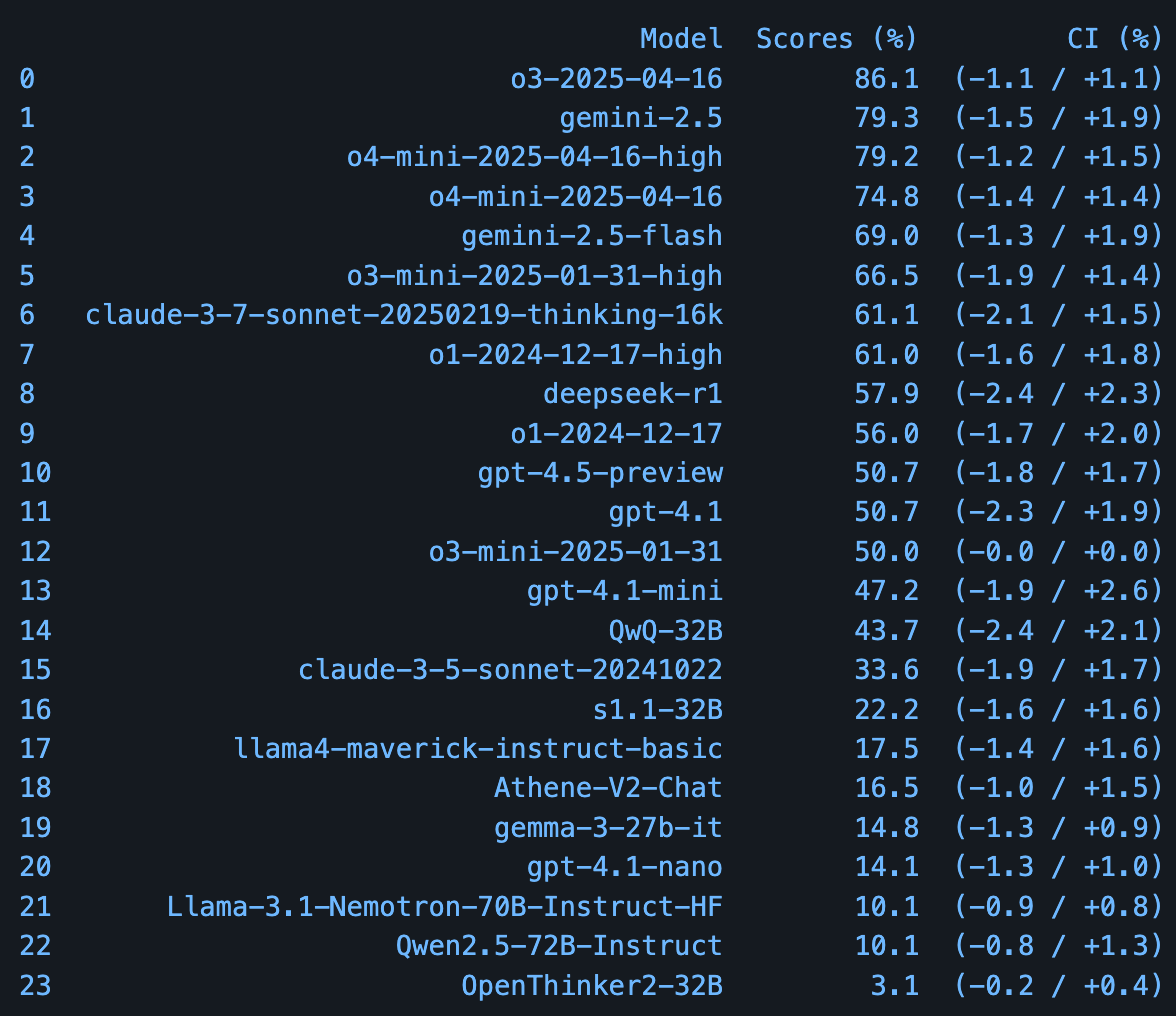
PHYBench: Benchmark for Evaluating LLM Physics Reasoning Ability Released: A research team from Peking University has launched PHYBench, a new evaluation benchmark specifically designed to assess the ability of large language models to understand and reason about real-world physical processes. The benchmark includes 500 questions based on real physical scenarios. According to preliminary evaluation results provided in the paper, Google’s Gemini-2.5-Pro leads in performance on this benchmark. (Source: karminski3)
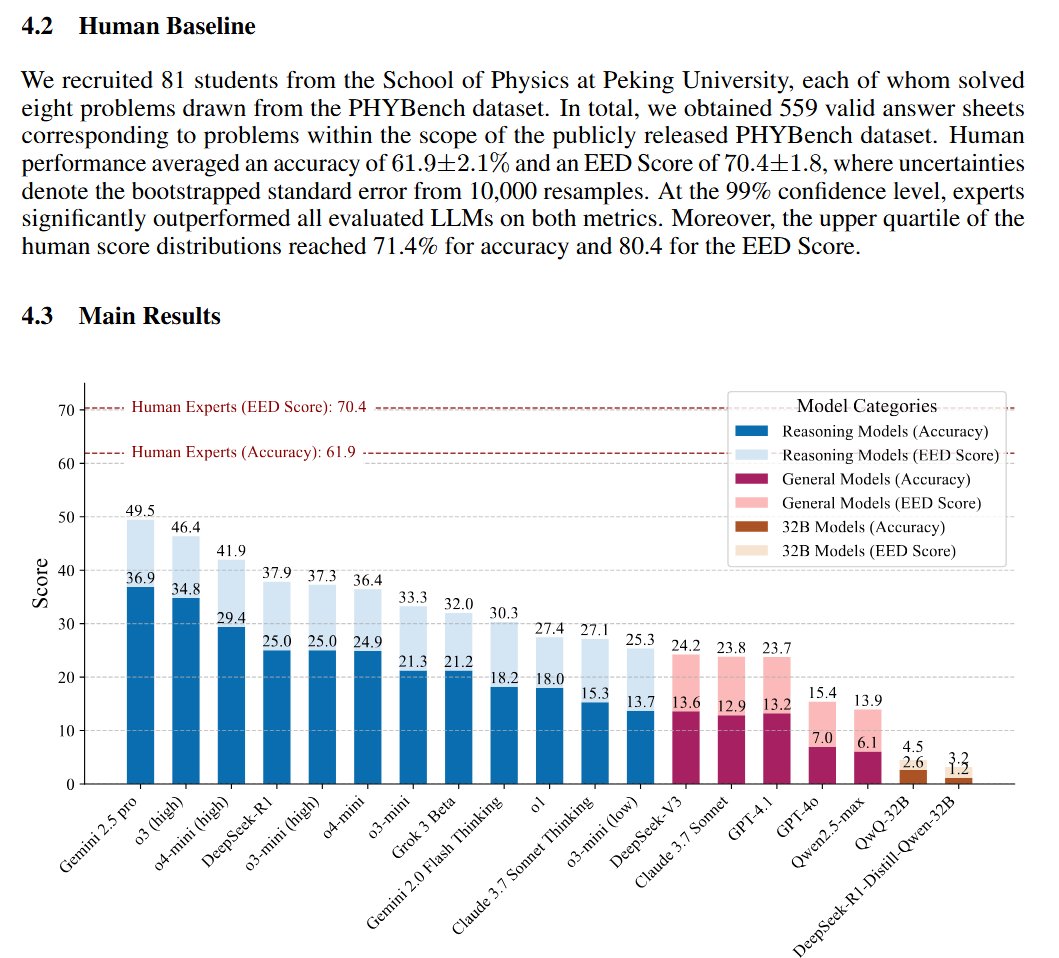
💼 Business
Alibaba’s Qwen and FLock.io Announce Strategic Partnership: Alibaba’s Tongyi Qianwen large model (Qwen) and the decentralized AI compute platform FLock.io have entered into a strategic partnership. The two parties aim to jointly explore and promote the practical application of decentralized AI, combining the capabilities of the Qwen open-source model series with FLock.io’s decentralized technology framework to offer new possibilities for AI developers and users. (Source: Alibaba_Qwen)
Alibaba Tongyi Lab Hiring LLM Multi-Turn Dialogue Research Interns: The Conversational Intelligence team at Alibaba’s Tongyi Lab, responsible for the development of the Tongyi series large models, is recruiting research interns in Beijing and Hangzhou focusing on LLM multi-turn dialogue. Research areas include generative reward modeling, inference-time extension of reward models, reinforcement learning for creative tasks like role-playing, and text-speech multi-modal dialogue. Applicants are required to be current PhD students with publications in top conferences and able to commit to at least a 6-month internship. (Source: 北京/杭州内推 | 阿里通义实验室对话智能团队招聘LLM多轮对话方向研究实习生)
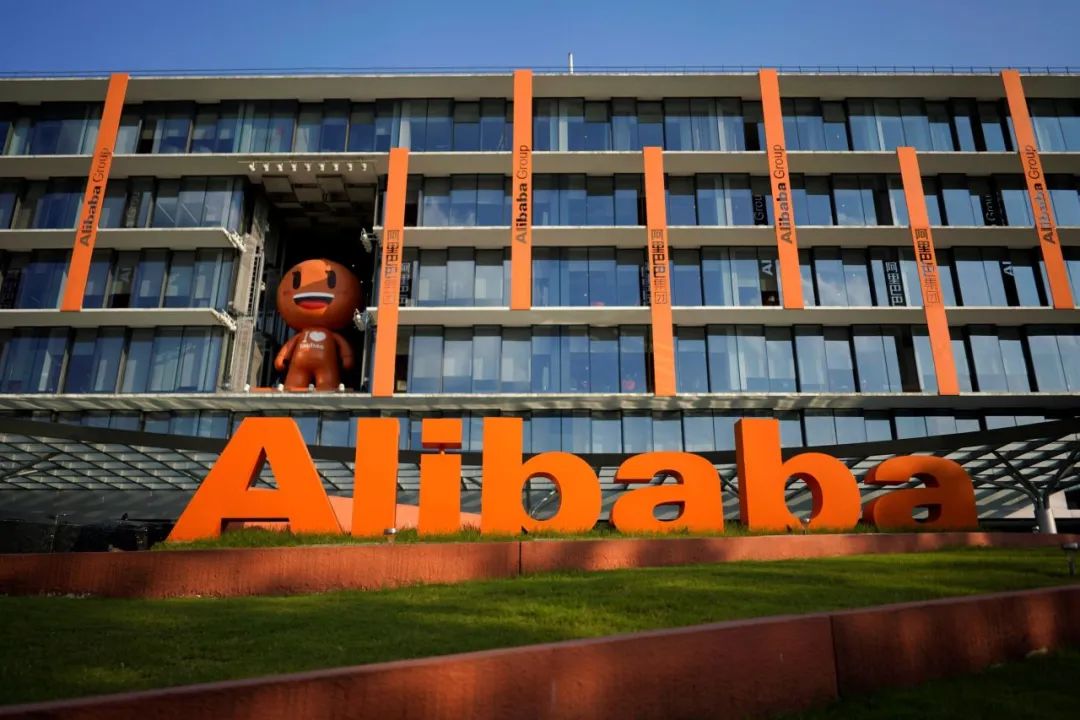
Productivity Tool Remio Hiring Overseas Social Media Operations Intern: Startup company Remio is looking for an intern familiar with overseas social media (Reddit, Hacker News, Twitter, etc.) and passionate about productivity tools. The main responsibilities include social media operations and content creation. The position accepts remote work, open to applicants in China or North America, with a suggested Reddit karma requirement of 100+. (Source: dotey)
API Company Kong Hiring Engineers and Interns for Shanghai Team: Kong Inc. (known for its open-source API gateway) is expanding its China team (located in Shanghai) and hiring for multiple positions, including interns and full-time roles. Hiring directions cover Rust development, AI Gateway, Kong Gateway, and front-end development. Developers interested in the relevant tech stacks can take note. (Source: dotey)
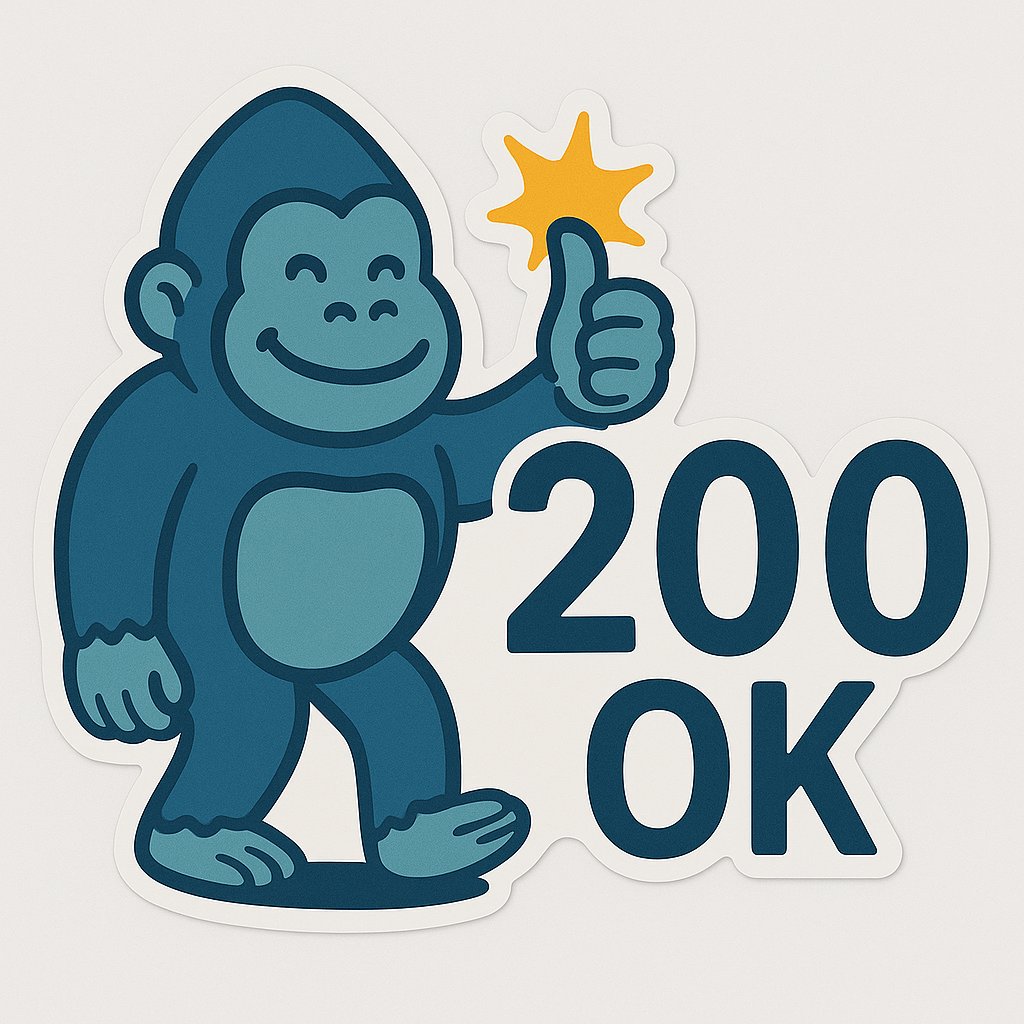
Webtoon Reduces Content Review Workload by 70% Using LangGraph: The globally renowned digital comics platform Webtoon built a system called WCAI (Webtoon Comprehension AI) using LangChain’s LangGraph framework. The system utilizes multimodal AI agents to automatically understand comic content, including identifying characters and attributing dialogue, extracting plot points and emotional tones, and supporting natural language queries. WCAI has been adopted by marketing, translation, and recommendation teams, successfully reducing the manual browsing and review workload by 70%, enhancing content processing efficiency and creative support. (Source: LangChainAI)
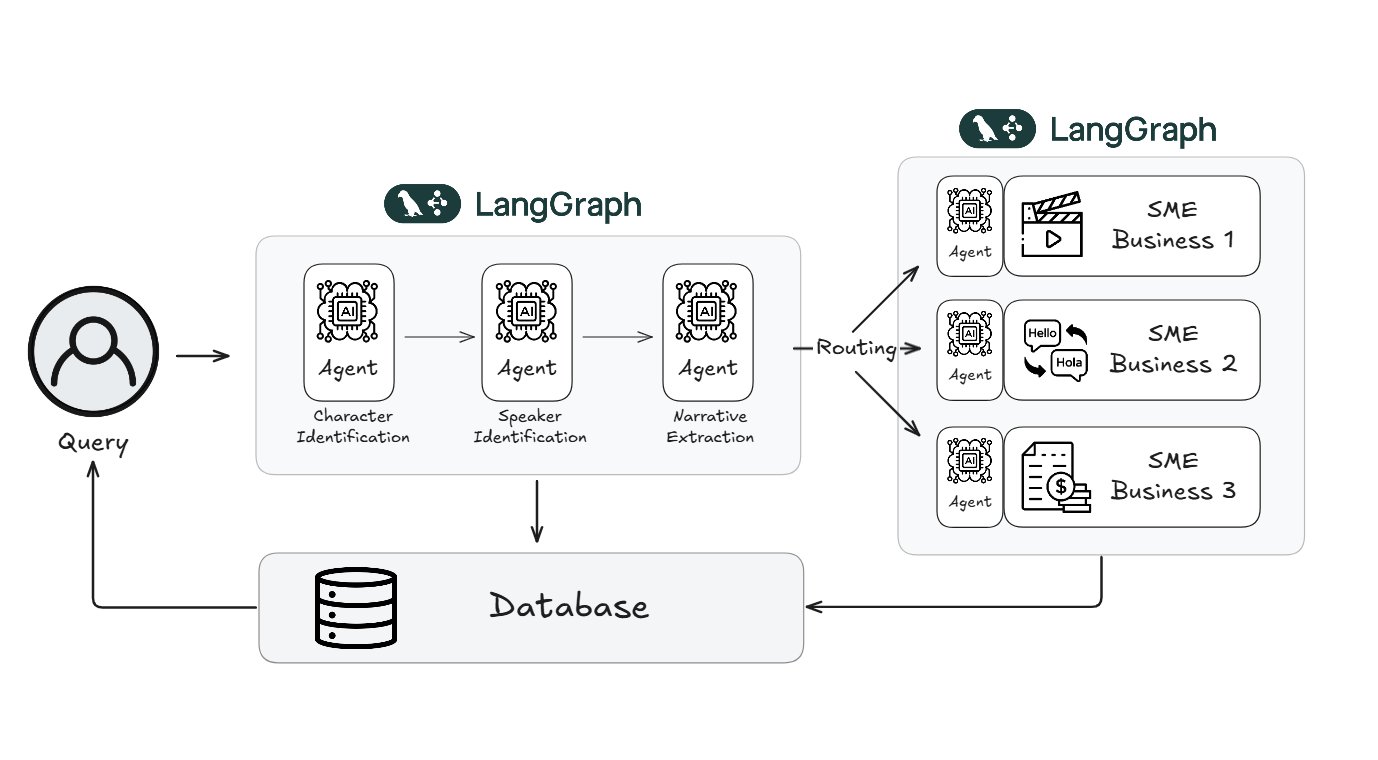
Meta AI Recruiting Research Talent at ICLR 2025: The Meta AI team attended the ICLR 2025 conference in Singapore, setting up a booth (#L03) to engage with attendees. Concurrently, Meta AI is actively posting job openings, seeking AI Research Scientists, Postdoctoral Researchers, and Research Assistants (PhD), with research directions including core learning theory, 3D generative AI, language generative AI, etc. Work locations include Paris and other global research centers. (Source: AIatMeta)
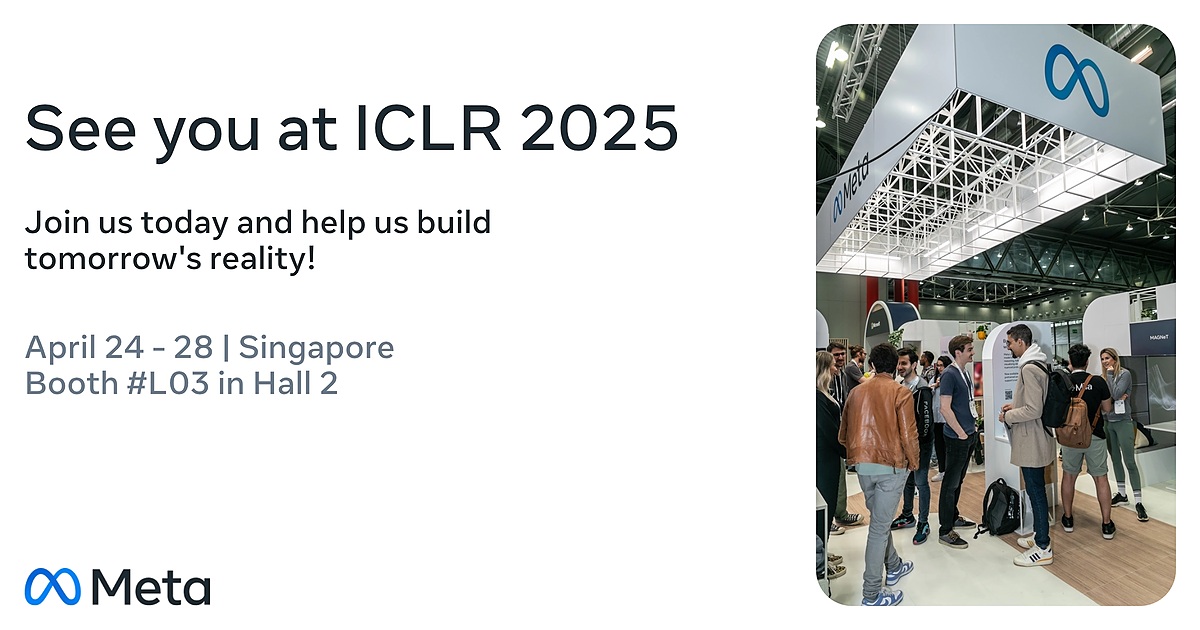
🌟 Community
Andrew Ng: AI-Assisted Programming Lowers Language Barriers, Enhances Developers’ Cross-Domain Capabilities: Renowned AI scholar Andrew Ng points out that AI-assisted programming tools are profoundly changing software development. Even without proficiency in a specific language (like JavaScript), developers can efficiently write code with AI assistance, making it easier to build cross-platform, cross-domain applications (e.g., backend developers building front-ends). While the syntax of specific languages becomes less critical, understanding core programming concepts (data structures, algorithms, principles of specific frameworks like React) remains key for guiding AI accurately and solving problems. AI is making developers more “polyglot.” (Source: AndrewYNg)
Microsoft AI CEO Claims Copilot Provided Flight Delay Info Ahead of Official Notice: Mustafa Suleyman, head of Microsoft AI, shared a “magical moment” on X platform: his Copilot AI assistant informed him of his flight delay earlier than the official airport notification. Upon confirmation with gate staff, the information was accurate but had not yet been publicly announced. This showcases AI’s potential in integrating and disseminating real-time information, possibly surpassing traditional information channels. (Source: mustafasuleyman)
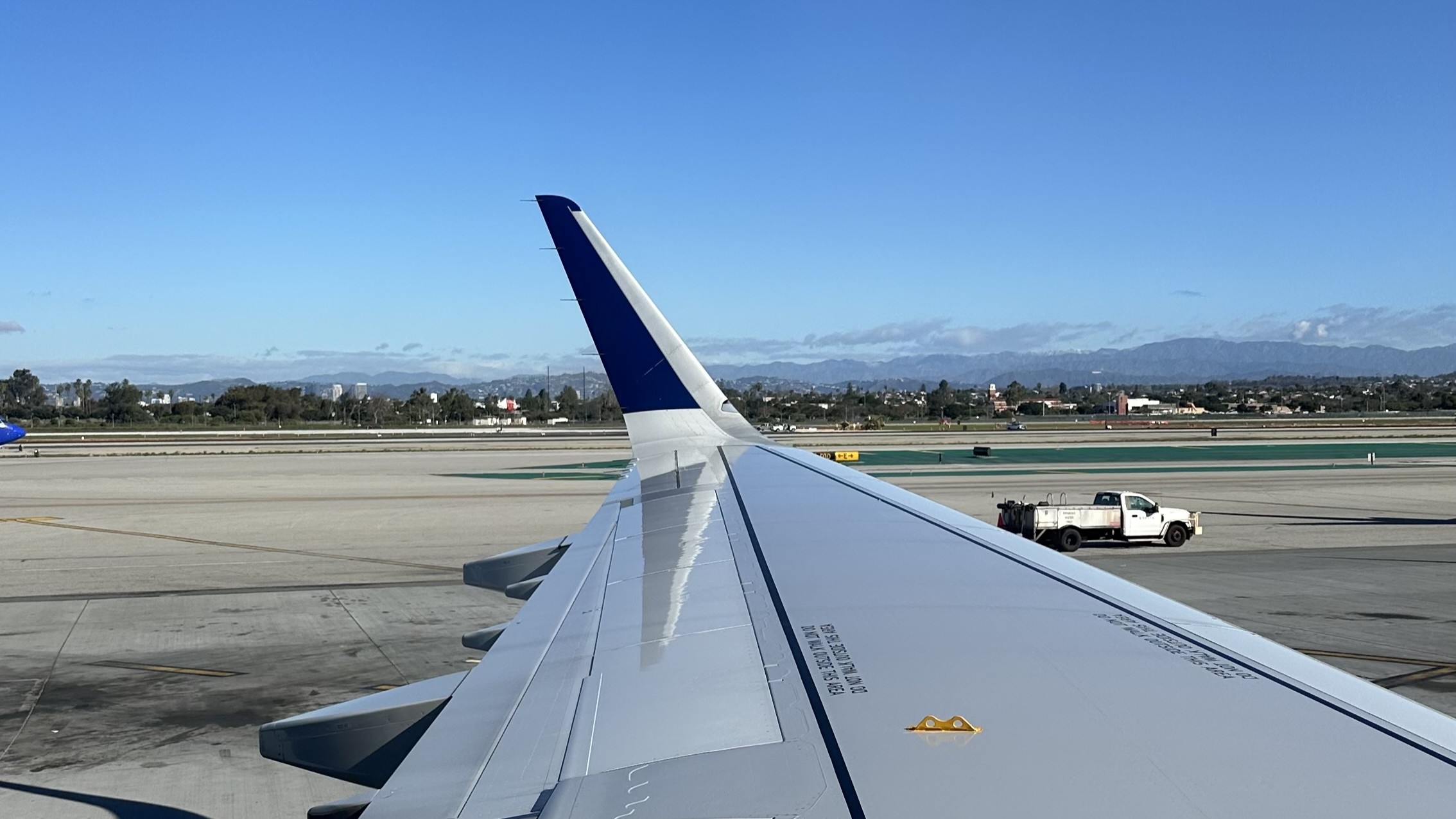
Community Discusses Pros and Cons of GPT-4.5 vs. o1 Pro on Different Tasks: Users on X platform discussed their experiences with different OpenAI models in practical applications. One user noted that GPT-4.5 performs excellently in writing and translation tasks but is limited by a smaller context window, causing performance degradation when handling long texts. In contrast, the o1 Pro model, targeted at Pro users, boasts a 128K context window, demonstrating more stable and reliable performance when processing long code inputs, making it more suitable for programming tasks. This reflects the differing design and optimization focuses of various models. (Source: dotey)
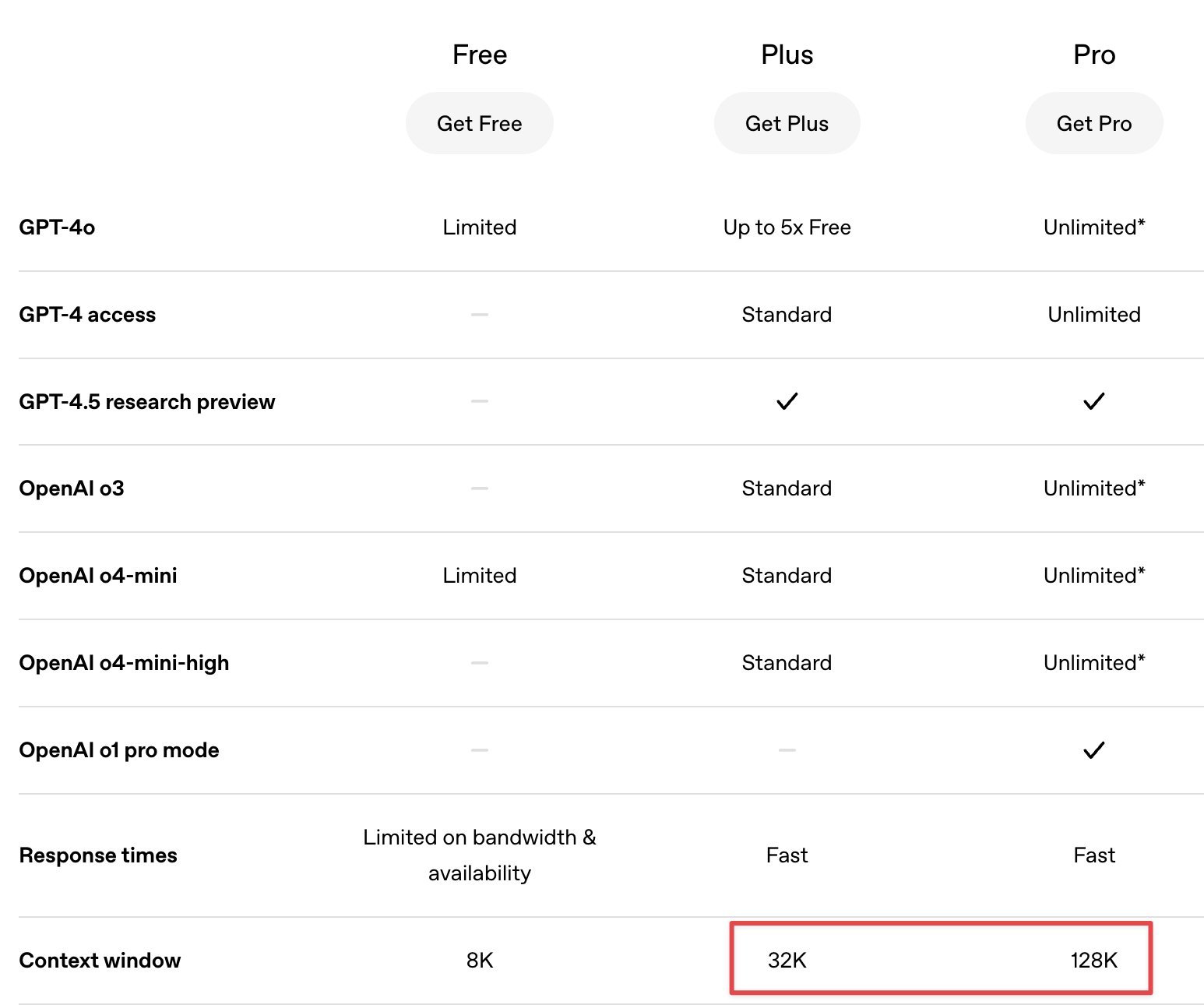
Hugging Face Hub Recommended as AI Learning and Communication Platform: An X platform user recommended Hugging Face Hub not just as a repository for models and datasets, but also as an active community for AI learning and exchange. Users can find engineers and researchers in the discussion sections of models, datasets, or Spaces sharing their experimental processes, encountered problems, solutions, and discussions on related research papers, thereby gaining first-hand practical experience and deep insights. (Source: huggingface)
ChatGPT “Roasting” Reddit Community Culture Sparks Discussion: A Reddit user asked ChatGPT to “roast” the Reddit platform. The response generated by ChatGPT accurately captured and satirized some typical characteristics of the Reddit community, such as users holding contradictory views, excessive concern for upvotes (karma), giving expert-level advice despite lacking real-world experience, and “keyboard warrior” behavior in specific subreddits. The post sparked discussion and further imitation among community users. (Source: Reddit r/ArtificialInteligence)
Originality and Value of AI-Generated Content Prompt Reflection: A post on Reddit sparked discussion about the originality of AI-generated content. Using the Mona Lisa as an example, the post pointed out that human creation itself is often a “remix” based on experience, and AI generating content under human guidance is more akin to a “master guiding an apprentice” than pure copying. The discussion suggested the key issue isn’t whether AI can be “original,” but rather how to properly attribute credit, respect original creators’ rights, and judge the intent and value of the work. (Source: Reddit r/ArtificialInteligence)
Community Questions Validity of LLM Leaderboards: Users in the Reddit r/LocalLLaMA community expressed skepticism about LLM leaderboards like LMSYS Arena, which are based on Elo ratings. Some comments suggested these leaderboards might reflect model “style” or “vibe” (e.g., verbosity, use of Markdown and emojis) more than true comprehensive ability. Furthermore, the confidence intervals for Elo scores among top models often overlap, casting doubt on the statistical significance of ranking differences. (Source: Reddit r/LocalLLaMA)
User Observes Multiple “Emergent Behaviors” in ChatGPT: A Reddit user shared several recent “unexpected” behaviors encountered while using ChatGPT, classifying them as “emergent behaviors.” These included: 1. The model realizing it misunderstood an instruction (confusing chat history with an uploaded document) and proactively apologizing and correcting itself without being prompted. 2. After a sensitive topic mentioned by the user was deleted by the system, the model referenced the deleted content in a subsequent conversation to express concern. 3. When discussing the difficulty of testing AI’s spontaneous thought, the model proactively created an analogous concept, the “Heisenberg Uncertainty Recursion Principle.” These instances sparked discussions about the boundaries of LLM self-awareness, memory, and creativity. (Source: Reddit r/ArtificialInteligence)
💡 Other
Google DeepMind Updates Music AI Sandbox Toolset: Google DeepMind announced new features for its Music AI Sandbox. This is a set of experimental AI tools for professional musicians aimed at assisting music creation. Powered by their latest Lyria 2 model, the new features can help songwriters and other musicians explore creative ideas, generate musical snippets, and more. (Source: demishassabis)
Discussion on Quantum Computing Governance Principles: Community members shared and discussed principles regarding quantum computing governance. As quantum computing technology advances, its immense potential in areas like cryptography, material science, drug discovery, and integration with AI/ML is drawing attention, while also posing challenges related to security, ethics, and governance, necessitating the preemptive establishment of relevant norms. (Source: Ronald_vanLoon)
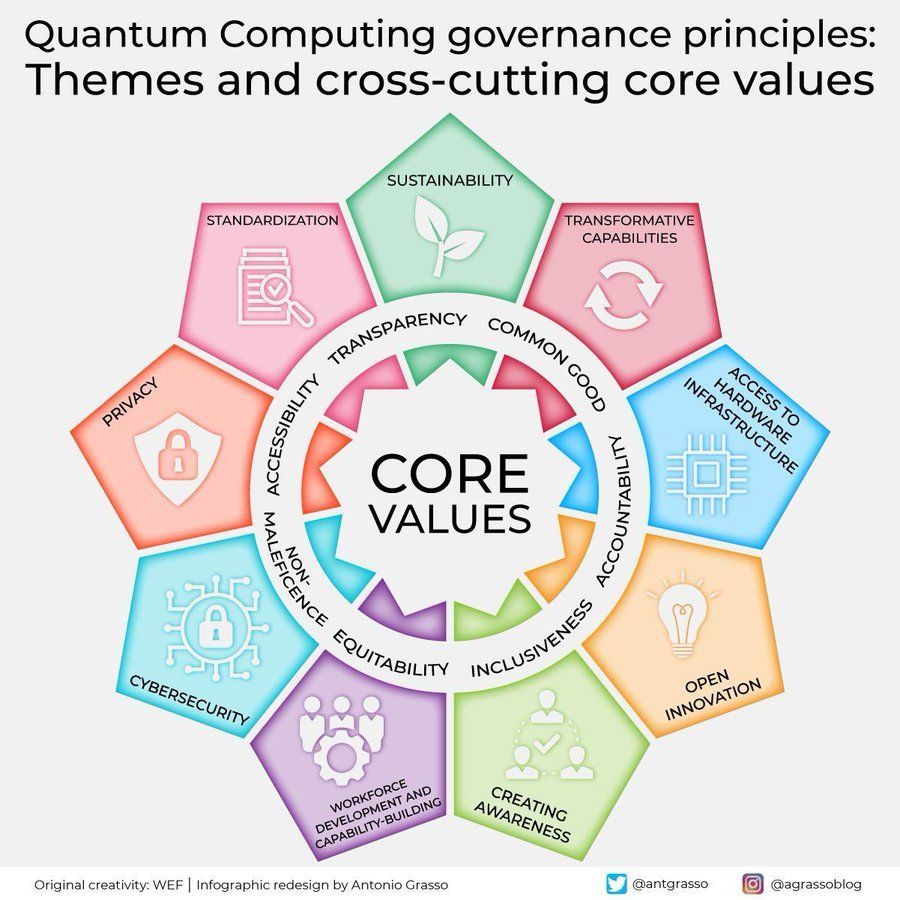
MIT Develops Banana-Shaped Wearable Soft Robot: Researchers at MIT have developed a novel wearable soft robot shaped like a banana, integrated with sensing capabilities. This research demonstrates the application potential of soft robotics in human-robot interaction, medical rehabilitation, and wearable devices, with its flexible structure and integrated sensing enabling more natural and safer physical interactions. (Source: Ronald_vanLoon)
AI-Driven Robotics Advances: Recent social media posts showcased several advancements in robotics technology, often enabled by or related to AI: 1. SR-02: A quadrupedal “robotic mount” capable of carrying four people. 2. SnapBot: A morphing legged robot. 3. Matic: A robot mimicking Tesla’s FSD vision system for home cleaning. 4. micropsi: A German startup developing an AI system enabling robots to handle unpredictable tasks. 5. Boston Dynamics Spot: The robot dog being tested in natural environments. 6. Humanoid Robot Race: Demonstrating the locomotion capabilities of humanoid robots. 7. Robotic Arm Handwriting: Showcasing the fine manipulation capabilities of robots. (Source: Ronald_vanLoon, Ronald_vanLoon, Ronald_vanLoon, Ronald_vanLoon, Ronald_vanLoon, Ronald_vanLoon, Ronald_vanLoon)
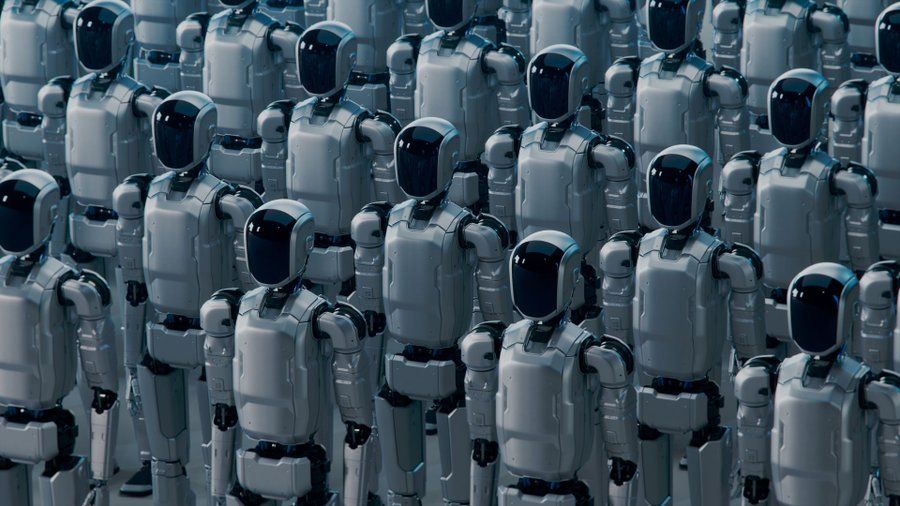