Keywords:AI model, OpenAI, Multimodal, Agent, O3 model, O4-mini, Visual reasoning, Tool calling, Gemini 2.5 Flash, Tencent Yuanbao AI, LLM integration, Reinforcement learning
🔥 Focus
OpenAI Releases o3 and o4-mini Models, Integrating Tools and Visual Reasoning Capabilities: OpenAI has officially released its smartest and most powerful reasoning models to date, o3 and o4-mini. The core highlight is the first-time implementation of Agents actively calling and combining all tools within ChatGPT (web search, Python data analysis, deep visual understanding, image generation, etc.), and integrating images into the reasoning chain for thinking. o3 leads comprehensively in areas like coding, math, science, and visual perception, setting new SOTA records on multiple benchmarks; o4-mini optimizes for speed and cost, with performance far exceeding its size. Both models exhibit stronger instruction-following capabilities, more natural conversations, and can utilize memory and historical dialogue to provide personalized responses. This release marks a significant step for OpenAI towards more autonomous Agentic AI, enabling AI assistants to complete complex tasks more independently. (Source: OpenAI 王炸 o3/o4-mini!打通自主工具+视觉思考,大佬赞“天才级”!AI 终获“十八般武艺”全家桶?)
OpenAI o3 and o4-mini Models Go Live, Enhancing Tool Use and Visual Reasoning: OpenAI released the o3 and o4-mini models late at night, accessible to users via ChatGPT Plus, Pro, and Team accounts. Key upgrades include: 1. The full-power version of o3 now supports tool calling (like web browsing, Code Interpreter) for the first time. 2. o3 and o4-mini are the first models capable of visual reasoning within their chain-of-thought, allowing them to analyze and think by combining images, similar to humans—for example, in a guess-the-location game based on a picture, the model can zoom in on image details for step-by-step reasoning. This capability significantly improves the models’ performance on multi-modal tasks (like MMMU, MathVista), signaling a greater role for AI in professional scenarios requiring visual judgment (e.g., security monitoring, medical image analysis). Concurrently, OpenAI also open-sourced the AI programming tool Codex CLI. (Source: OpenAI深夜上线o3满血版和o4 mini – 依旧领先。)
Tencent Yuanbao AI Officially Integrates with WeChat, Opening a New Chat Paradigm: Tencent Yuanbao AI is now officially available as a WeChat contact; users can add it by searching for “元宝 (Yuanbao)”. This move breaks the traditional model requiring separate AI applications, seamlessly integrating AI into users’ daily communication scenarios. Yuanbao AI (based on Hunyuan and DeepSeek) allows direct interaction within the WeChat chat window, supporting summarization of images, official account articles, web links, audio, and video (excluding WeChat Channels videos for now), and can search historical chat records. Although it doesn’t yet support image generation or group chats, its ease of use and deep integration with the WeChat ecosystem are considered significant advantages. Analysts believe that WeChat, with its vast user base and social graph, transforming AI into a contact list entry, could change the human-computer interaction paradigm, making AI a more natural part of users’ lives. (Source: 劲爆!元宝AI接入微信了,怎么用?看这篇就够了, 腾讯元宝最终还是活成了微信的模样。)
US May Indefinitely Suspend Nvidia H20 Chip Exports to China, with Far-Reaching Impact: The US government has notified Nvidia that it will indefinitely suspend the export of H20 AI chips (a special version previously designed to comply with export controls) to China. The H20 is Nvidia’s most powerful compliant chip developed for the Chinese market, and the ban is expected to deal a significant blow to Nvidia. Data shows China is Nvidia’s fourth-largest revenue source, with H20 sales projected to reach the tens of billions of dollars level in 2024. Chinese tech companies (like ByteDance, Tencent) are major buyers of Nvidia chips, with significant investment growth. This move not only affects Nvidia’s revenue but may also weaken its CUDA ecosystem (Chinese developers account for over 30%). Meanwhile, Chinese domestic AI chip companies like Huawei (e.g., Ascend 910C) are accelerating their development and may fill the market gap. The event has triggered market concerns, causing Nvidia’s stock price to fall. (Source: 中国对英伟达到底有多重要?)
🎯 Trends
Google’s Top Video Model Veo 2 Lands Free on AI Studio: Google announced that its advanced video generation model, Veo 2, is now available on Google AI Studio, Gemini API, and the Gemini App, offering free usage credits (around a dozen times daily, up to 8 seconds each). Veo 2 supports text-to-video (t2v) and image-to-video (i2v), understands complex instructions, generates realistic videos in diverse styles, and allows control over camera movements. Officials emphasize that the key to high-quality video generation lies in providing clear, detailed Prompts with visual keywords. The model also features advanced functions like in-video editing (inpainting, outpainting), cinematic camera work, and intelligent transitions, aiming to integrate into content creation workflows and enhance efficiency. (Source: 谷歌杀疯了,顶级视频模型 Veo 2 竟免费开放?速来 AI Studio 白嫖。)
Google Releases Gemini 2.5 Flash, Focusing on Speed, Cost, and Controllable Thinking Depth: Google has launched a preview of the Gemini 2.5 Flash model, positioned as a lightweight model optimized for speed and cost. The model performs impressively on the LMArena leaderboard, ranking second alongside GPT-4.5 Preview and Grok-3, and first on difficult prompts, coding, and long queries. Its core feature is the introduction of “thinking” capability and fully interleaved reasoning, allowing the model to plan and break down tasks before outputting. Developers can control the model’s thinking depth (token limit) via a “thinking budget” parameter, balancing quality, cost, and latency. Even with a budget of 0, its performance surpasses Gemini 2.0 Flash. The model is cost-effective, priced at 1/10th to 1/5th of Gemini 2.5 Pro, suitable for high-concurrency, large-scale AI workflows. (Source: 快如闪电,还能控制思考深度?谷歌 Gemini 2.5 Flash 来了,用户盛赞“绝妙组合”。)
Kunlun Wanwei Releases Unlimited-Length Film Generation Model Skyreels-V2: Kunlun Wanwei has launched and open-sourced Skyreels-V2, claimed to be the world’s first high-quality video generation model supporting unlimited duration. The model aims to address the pain points of existing video models in understanding cinematic language, motion coherence, video length limitations, and the lack of professional datasets. Skyreels-V2 combines multi-modal large models, structured annotation, diffusion generation, reinforcement learning (DPO for motion quality optimization), and high-quality fine-tuning in a multi-stage training strategy. It employs a Diffusion Forcing architecture, achieving long video generation through special schedulers and attention mechanisms. Officials state its generation effect reaches “film-level” quality, performing excellently on benchmarks like V-Bench1.0, surpassing other open-source models. Users can experience generating videos up to 30 seconds long online. (Source: 震撼!昆仑万维 | 发布全球首款无限时长电影生成模型:Skyreels-V2,可在线体验!)
Shanghai AI Lab Releases Native Multi-modal Model InternVL3: Shanghai Artificial Intelligence Laboratory has introduced InternVL3, a large multi-modal model (MLLM) adopting a native multi-modal pre-training paradigm. Unlike most models adapted from text-only LLMs, InternVL3 learns simultaneously from multi-modal data and pure text corpora in a single pre-training stage, aiming to overcome the complexity and alignment challenges of multi-stage training. The model incorporates variable visual position encoding, advanced post-training techniques, and test-time scaling strategies. InternVL3-78B achieved a score of 72.2 on the MMMU benchmark, setting a new record for open-source MLLMs, with performance approaching leading proprietary models while maintaining strong pure language capabilities. Training data and model weights will be made public. (Source: LLM每周速递!| 涉及多Token注意力、Text2Sql增强、多模态、RNN大模型、多Agent应用等)
UCLA et al. Propose d1 Framework for RL in Diffusion LLM Inference: Researchers from UCLA and Meta AI have proposed the d1 framework, applying reinforcement learning (RL) post-training to masked diffusion large language models (dLLMs) for the first time. Existing RL methods (like GRPO) are primarily designed for autoregressive LLMs and are difficult to apply directly to dLLMs due to the lack of a natural decomposition of log-probabilities. The d1 framework involves two stages: first, supervised fine-tuning (SFT), followed by an RL stage introducing a novel policy gradient method, diffu-GRPO. This method uses an efficient single-step log-probability estimator and utilizes stochastic prompt masking as regularization, reducing the amount of online generation needed for RL training. Experiments show that the d1 model based on LLaDA-8B-Instruct significantly outperforms the base model and models using only SFT or diffu-GRPO on math and logical reasoning benchmarks. (Source: UCLA | 推出开源后训练框架:d1,扩散LLM推理也能用上GRPO强化学习!)
Meta Proposes Multi-Token Attention (MTA): Researchers at Meta have proposed the Multi-Token Attention (MTA) mechanism, aimed at improving attention computation in large language models (LLMs). Traditional attention mechanisms rely solely on the similarity between single query and key tokens. MTA applies convolutional operations on query, key, and head vectors, enabling the model to consider multiple adjacent query and key tokens simultaneously when determining attention weights. Researchers believe this allows leveraging richer, more detailed information to locate relevant context. Experiments show that MTA outperforms traditional Transformer baseline models on standard language modeling and long-context information retrieval tasks. (Source: LLM每周速递!| 涉及多Token注意力、Text2Sql增强、多模态、RNN大模型、多Agent应用等)
TogetherAI Introduces RNN-Based Inference Model M1: TogetherAI has proposed M1, a novel hybrid linear RNN inference model based on the Mamba architecture. The model aims to address the computational complexity and memory limitations faced by Transformers when processing long sequences and performing efficient inference. M1 enhances performance through knowledge distillation from existing inference models and reinforcement learning training. Experimental results show that M1 not only outperforms previous linear RNN models on math reasoning benchmarks like AIME and MATH but also rivals the performance of similarly sized DeepSeek-R1 distilled inference models. More importantly, M1’s generation speed is over 3 times faster than Transformers of the same size, and it achieves higher accuracy than the latter through self-consistency voting under a fixed generation time budget. (Source: LLM每周速递!| 涉及多Token注意力、Text2Sql增强、多模态、RNN大模型、多Agent应用等)
Tencent Hunyuan Open-Sources InstantCharacter Framework: The Tencent Hunyuan team has open-sourced InstantCharacter, a framework for image generation capable of extracting and preserving character features from a single input image, then placing that character into different scenes or styles. The technology aims to achieve high-fidelity character identity preservation and controllable style transfer. An online demo based on Ghibli and Makoto Shinkai art styles is available on Hugging Face, and the relevant paper, code repository, and ComfyUI plugin have been released for community use and further development. (Source: karminski3)
ChatGPT Memory Feature Upgraded to Support Web Search with Memory: OpenAI has upgraded ChatGPT’s Memory feature, adding the capability for “Search with Memory”. This means that when performing web search tasks, ChatGPT can leverage previously stored user preferences, location, and other memory information to optimize search queries, thereby providing more personalized search results. For example, if ChatGPT remembers a user is vegetarian, when asked about nearby restaurants, it might automatically search for “nearby vegetarian restaurants”. This move is seen as an important step by OpenAI in enhancing AI personalization services, aiming to improve user experience and differentiate from competitors with memory features (like Claude, Gemini). Users can choose to disable the memory function in settings. (Source: Cursor 新版抢先体验!规则自动生成+项目结构感知+MCP 图片支持,网友:多项实用更新!)
AI Model Runtime Snapshot Technology Avoids Cold Starts: The machine learning community is exploring model snapshot technology to optimize LLM runtime orchestration. This technique saves the complete state of the GPU (including KV cache, weights, memory layout), enabling rapid recovery (around 2 seconds) when switching between different models, thus avoiding cold starts and GPU idling. Practitioners have shared success in running over 50 open-source models on two A1000 16GB GPUs using this method, without needing containers or reloading models. This model multiplexing and rotation technique holds potential for improving GPU utilization and reducing inference latency. (Source: Reddit r/MachineLearning)
🧰 Tools
ByteDance Volcengine Launches AI Hardware One-Stop Solution Demo: ByteDance’s Volcengine showcased its AI hardware one-stop solution, developed in collaboration with embedded chip manufacturers, using the AtomS3R development board as an example. The solution aims to provide a low-latency, highly responsive AI interaction experience, featuring millisecond-level real-time response, real-time interruption and turn-taking, and complex environment audio noise reduction capabilities via the RTC SDK, effectively reducing background noise interference and improving voice interaction accuracy. The client-side code and server-side program for this solution are open-source, allowing developers for DIY customization, such as giving the hardware a custom personality, role, voice timbre, or connecting it to knowledge bases and MCP tools. The hardware itself includes a camera, with plans to support visual understanding functions in the future. (Source: 体验完字节送的迷你AI硬件,后劲有点大…

)
Metasearch AI Search Launches “Learn Something Today” Feature: Metasearch AI Search has launched a new feature called “Learn Something Today,” which automatically converts user-uploaded files (supporting various formats) or provided web links into a structured online course video with narration and presentation elements (PPT, animation). Users can choose different explanation styles (e.g., storytelling, Napoleon style) and voices (e.g., cool aloof female). The feature aims to transform information input into a more easily absorbable learning experience, even providing post-lesson quizzes. This combination of content generation and personalized teaching is considered a potential game-changer for AI applications in education and information consumption, offering a novel way to acquire knowledge and quickly digest content. (Source: 说个抽象的事,你现在可以在秘塔AI搜索里上课了。
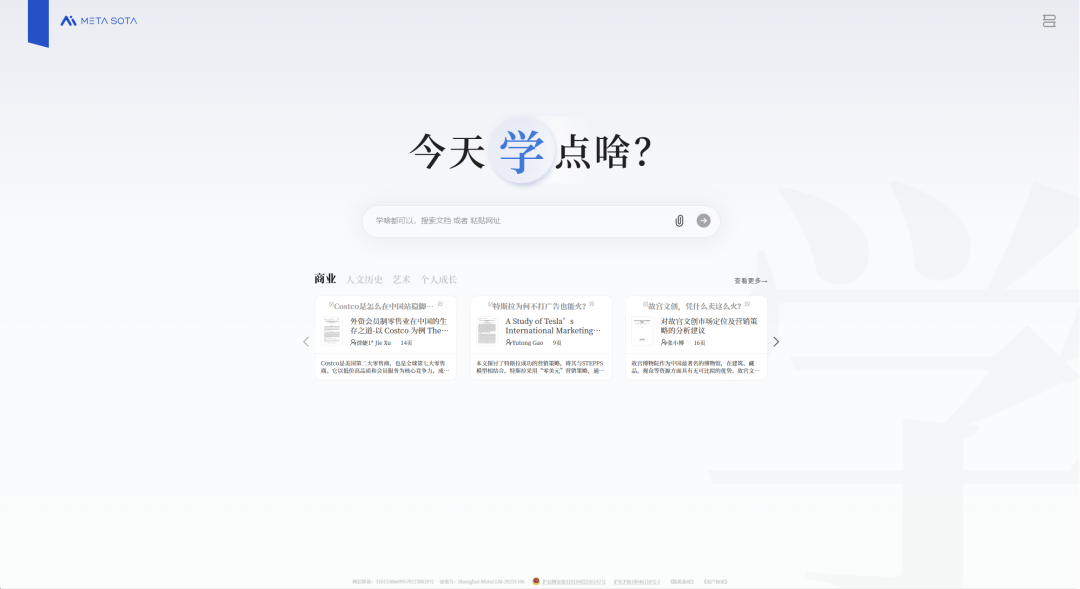
)
Cursor IDE Updated to v0.49, Enhancing Rules System and Agent Control: The AI-first code editor Cursor has released a preview of its 0.49 update. New features include: 1. Automatic generation of .mdc rule files via the chat command /Generate Cursor Rules
to solidify project context. 2. Smarter automatic rule application, with the Agent automatically loading corresponding rules based on file paths. 3. Fixed a bug where “Always attach rules” failed in long conversations. 4. Added “Project Structure Awareness” (Beta) feature, allowing the AI to better understand the entire project. 5. The MCP (Model Context Protocol) now supports image transmission, facilitating the handling of vision-related tasks. 6. Enhanced Agent control over terminal commands, allowing users to edit or skip commands before execution. 7. Support for global file ignore configuration (.cursorignore). 8. Optimized code review experience, displaying the diff view directly after Agent messages. (Source: Cursor 新版抢先体验!规则自动生成+项目结构感知+MCP 图片支持,网友:多项实用更新!)
OpenAI Open-Sources Command-Line AI Programming Tool Codex CLI: Coinciding with the release of o3 and o4-mini, OpenAI has open-sourced Codex CLI, a lightweight AI coding Agent that runs directly in the user’s command-line terminal. The tool is designed to leverage the powerful coding and reasoning capabilities of the new models, can directly process local codebases, and can even incorporate screenshots or sketches for multi-modal reasoning. OpenAI CEO Sam Altman personally promoted it, emphasizing its open-source nature to foster rapid community iteration. Simultaneously, OpenAI launched a $1 million grant program (in API Credits) to support projects based on Codex CLI and OpenAI models. (Source: OpenAI 王炸 o3/o4-mini!打通自主工具+视觉思考,大佬赞“天才级”!AI 终获“十八般武艺”全家桶?)
Tencent Cloud LKE Platform Integrates MCP, Simplifying Agent Construction: Tencent Cloud Language Knowledge Engine (LKE) platform now supports the Model Context Protocol (MCP), aiming to lower the barrier for building and using AI Agents. Users can now easily connect built-in MCP tools like Tencent Cloud EdgeOne Pages (one-click webpage deployment) and Firecrawl (web crawler) through click operations on the LKE platform. Combined with LKE’s powerful knowledge base (RAG) capabilities, users can create complex applications based on private knowledge and external tool calls, such as automatically generating and publishing webpages based on knowledge base content. The platform supports Agent mode, where models (like DeepSeek R1) can autonomously think and select appropriate tools to complete tasks. The platform also supports connecting external MCPs. (Source: 效果惊艳!MCP+腾讯云知识引擎,一个0门槛打造专属AI Agent的神器诞生~
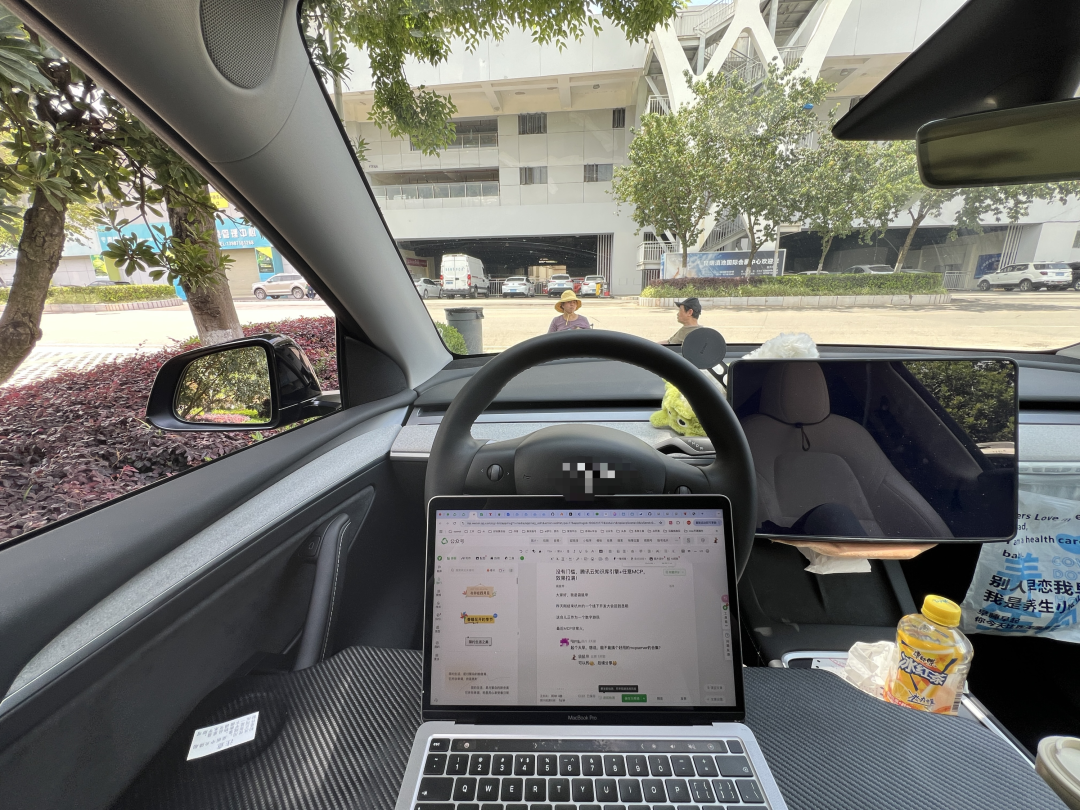
)
Spring AI Framework: An Application Framework for AI Engineering: Spring AI is an AI application framework designed for Java developers, aiming to bring the design principles of the Spring ecosystem (like portability, modular design, POJO usage) to the AI domain. It provides a unified API for interacting with various mainstream AI model providers (Anthropic, OpenAI, Microsoft, Amazon, Google, Ollama, etc.), supporting features like chat completion, embeddings, text-to-image/audio, and moderation. It also integrates with multiple vector databases (Cassandra, Azure Vector Search, Chroma, Milvus, etc.), offering portable APIs and SQL-style metadata filtering. The framework further supports structured output, tool/function calling, observability, ETL framework, model evaluation, chat memory, and RAG functionalities, simplifying integration through Spring Boot auto-configuration. (Source: spring-projects/spring-ai – GitHub Trending (all/weekly)
)
olmocr: PDF Linearization Toolkit for LLM Dataset Processing: allenai has open-sourced olmocr, a toolkit specifically designed for processing PDF documents for large language model (LLM) dataset construction and training. It includes various features: prompt strategies using ChatGPT 4o for high-quality natural text parsing, evaluation tools for comparing different processing pipeline versions, basic language filtering and SEO spam removal functions, fine-tuning code for Qwen2-VL and Molmo-O, a pipeline for processing PDFs at scale using Sglang, and tools for viewing processed documents in Dolma format. The toolkit requires GPU support for local inference and provides instructions for use locally and on multi-node clusters (supporting S3 and Beaker). (Source: allenai/olmocr – GitHub Trending (all/daily)

)
Dive Agent Desktop App v0.8.0 Released: The open-source AI Agent desktop application Dive has released version 0.8.0, featuring major architectural adjustments and functional upgrades. This version aims to integrate LLMs supporting tool calls with an MCP Server. Key updates include: LLM API key management, support for custom model IDs, full support for tool/function calling models; MCP tool management (add, delete, modify), configuration interface supporting JSON and form editing. The backend DiveHost has been migrated from TypeScript to Python to resolve LangChain integration issues and can run as a standalone A2A (Agent-to-Agent) server. (Source: Reddit r/LocalLLaMA)
llama.cpp Merges Multi-modal CLI Tools: The llama.cpp project has merged the command-line interface (CLI) example programs for LLaVa, Gemma3, and MiniCPM-V into a unified llama-mtmd-cli
tool. This is part of its gradual integration of multi-modal support (via the libmtmd
library). Although multi-modal support is still evolving (e.g., llama-server support is experimental), merging the CLIs is a step towards simplifying the toolset. Support for SmolVLM v1/v2 is also under development. (Source: Reddit r/LocalLLaMA)
LightRAG: Automating RAG Pipeline Deployment: LightRAG is an open-source RAG (Retrieval-Augmented Generation) project. A community member has created tutorials and automation scripts (using Ansible + Docker Compose + Sbnb Linux) enabling users to quickly deploy the LightRAG system on bare-metal servers (within minutes), achieving automated setup from a blank machine to a fully functional RAG pipeline. This simplifies the deployment process for self-hosted RAG solutions. (Source: Reddit r/LocalLLaMA)
Nari Labs Releases Open-Source TTS Model Dia-1.6B: Nari Labs has released and open-sourced its text-to-speech (TTS) model, Dia-1.6B. A key feature of this model is its ability not only to generate speech but also to naturally incorporate non-linguistic sounds (paralinguistic sounds) like laughter, coughs, and throat clearing into the speech, enhancing naturalness and expressiveness. Official demo videos showcase its effects. The model requires about 10GB of VRAM to run, and a quantized version is not yet available. The codebase and model have been released on GitHub and Hugging Face. (Source: karminski3)
📚 Learning
Jeff Dean Reviews Key Milestones in 15 Years of AI Development: Google Chief Scientist Jeff Dean, in a presentation, reviewed significant progress in the AI field over the past fifteen years, particularly emphasizing Google’s research contributions. Key milestones include: large-scale neural network training (demonstrating scaling effects), the DistBelief distributed system (enabling large model training on CPUs), Word2Vec word embeddings (revealing vector space semantics), Seq2Seq models (driving machine translation and other tasks), TPUs (custom hardware acceleration for neural networks), the Transformer architecture (revolutionizing sequence processing, becoming the foundation for LLMs), self-supervised learning (leveraging large-scale unlabeled data), Vision Transformer (unifying image and text processing), sparse models/MoE (increasing model capacity and efficiency), Pathways (simplifying large-scale distributed computing), Chain-of-Thought (CoT) (enhancing reasoning abilities), knowledge distillation (transferring large model capabilities to smaller models), and speculative decoding (accelerating inference). These technologies collectively propelled the development of modern AI. (Source: 比较全!回顾LLM发展史 | Transformer、蒸馏、MoE、思维链(CoT)
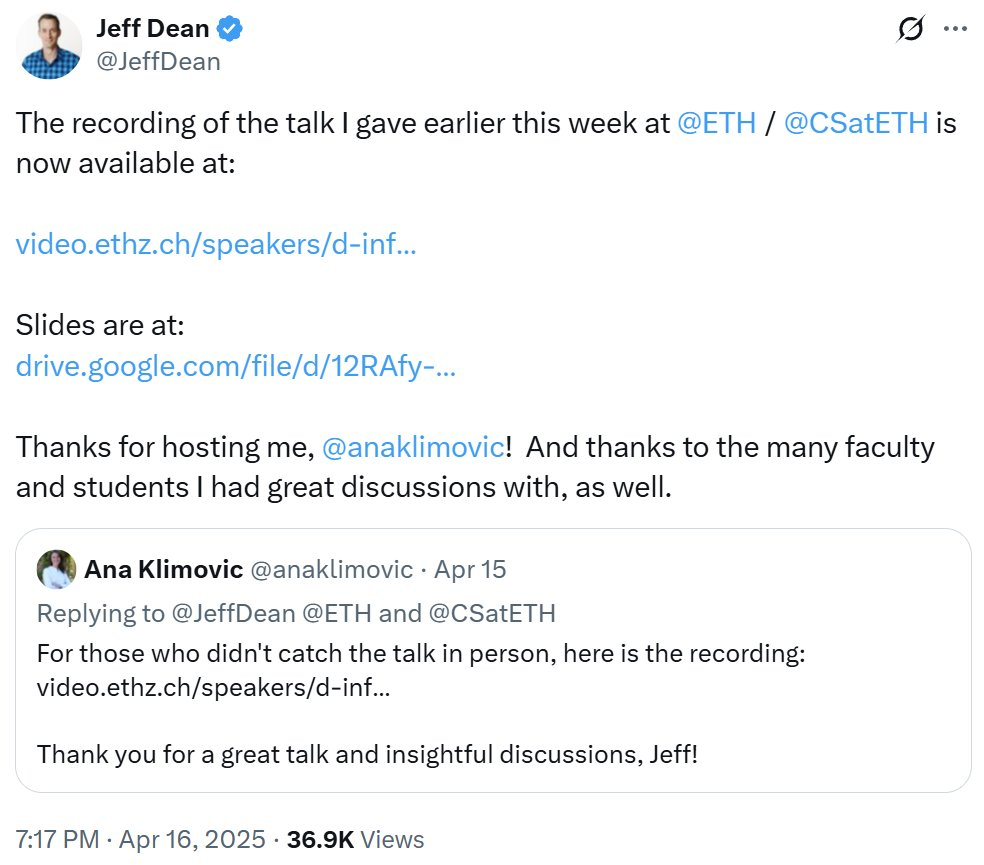
)
Nature Lists Highly Cited Papers of the 21st Century, AI Field Dominates: Nature magazine, by consolidating data from 5 databases, released a list of the Top 25 most cited papers of the 21st century. Microsoft’s 2016 ResNets paper (by He Kaiming et al.) ranked first overall; this research is fundamental to the progress of deep learning and AI. The top ranks also include multiple AI-related papers, such as random forests (6th), Attention is all you need (Transformer, 7th), AlexNet (8th), U-Net (12th), a deep learning review (Hinton et al., 16th), and the ImageNet dataset (Li Feifei et al., 24th). This reflects the rapid development and widespread impact of AI technology this century. The article also notes that the popularity of pre-prints adds complexity to citation statistics. (Source: Nature最新统计!盘点引领人类进入「AI时代」的论文,ResNets引用量第一!
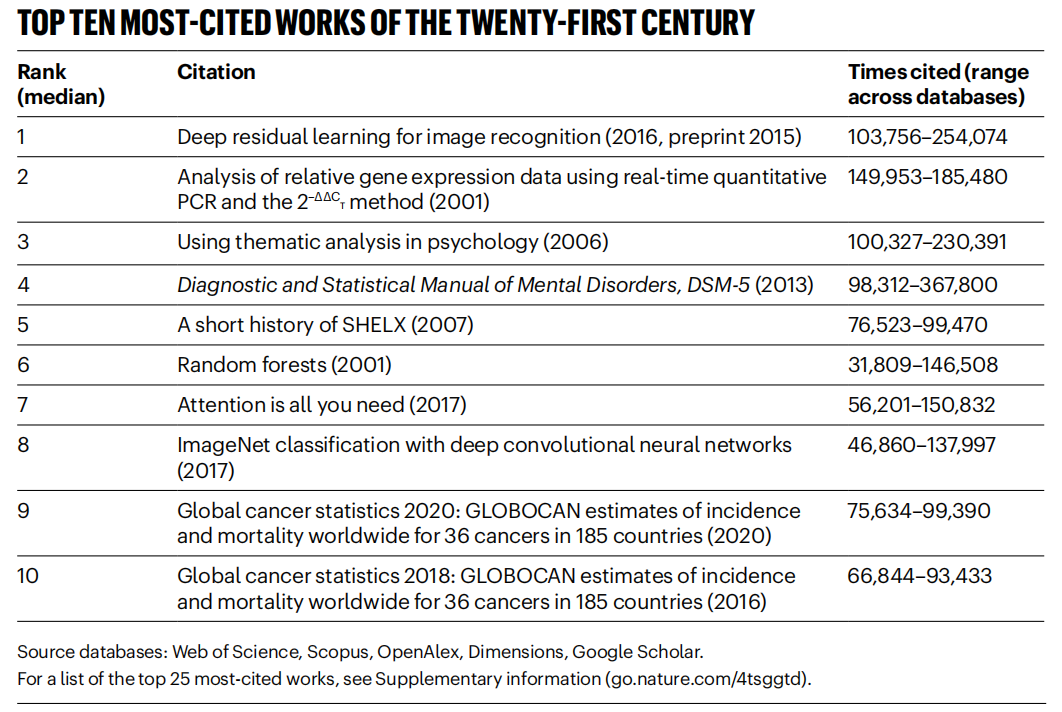
)
Beihang University et al. Release LLM Ensemble Survey: Researchers from Beijing University of Aeronautics and Astronautics and other institutions have published the latest survey on Large Language Model Ensemble (LLM Ensemble). LLM Ensemble refers to combining the strengths of multiple LLMs during the inference phase to handle user queries. The survey proposes a taxonomy for LLM Ensemble (pre-inference, in-inference, post-inference integration, subdivided into seven categories), systematically reviews the latest progress in each category, discusses related research questions (e.g., relationship with model merging, model collaboration, weak supervision), introduces benchmark datasets and typical applications, and finally summarizes existing achievements and outlines future research directions, such as more principled segment-level integration and finer-grained unsupervised post-integration. (Source: ArXiv 2025 | 北航等机构发布最新综述:大语言模型集成(LLM Ensemble)

)
Anthropic Shares Claude Code Usage Patterns and Experiences: Anthropic employees shared best practices and effective patterns for using Claude Code internally for programming. These patterns are not only applicable to Claude but are generally useful for programming collaboration with other LLMs. Emphasis was placed on providing clear context, breaking down complex problems, iterative questioning, leveraging the different strengths of the model (e.g., code generation, explanation, refactoring), and the importance of effective validation. These experiences aim to help developers utilize AI-assisted programming tools more efficiently. (Source: AnthropicAI
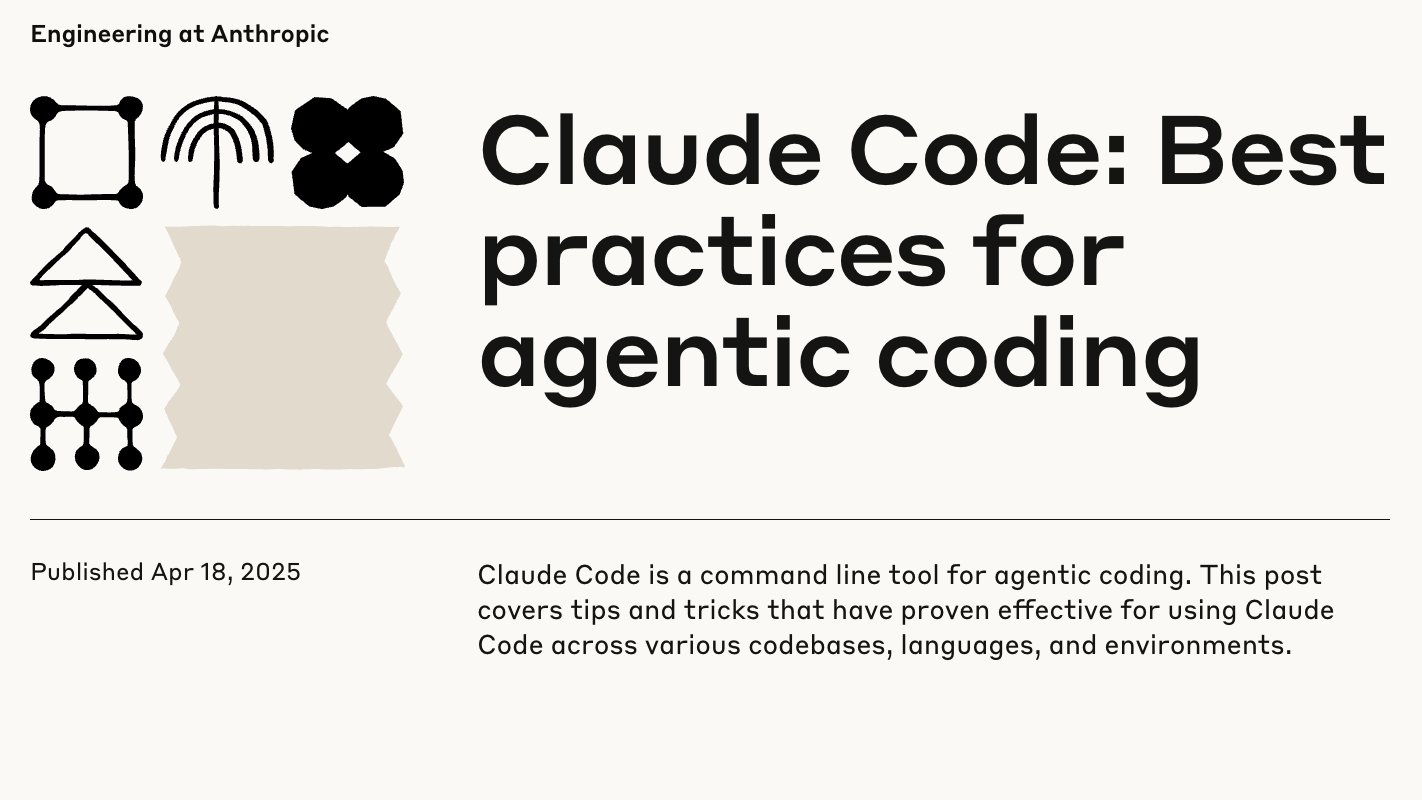
)
Anthropic Releases Claude Values Dataset: Anthropic has released a dataset named “values-in-the-wild” on Hugging Face Datasets. This dataset contains 3,307 values expressed by Claude across millions of real-world conversations. The release aims to increase transparency about model behavior and allow researchers and the public to download, explore, and analyze it to better understand the value tendencies manifested by large language models in practical applications. (Source: huggingface, huggingface)
Ten Key Perspectives on AI Cognitive Awakening: The article presents ten cognitive-level perspectives on AI development, aiming to help people understand the impact and nature of AI more profoundly. Core points include: AI’s intelligence differs from human intelligence (intelligence gap); AI prompts reflection on the nature of human consciousness; the human-AI relationship is shifting from tool to collaborative partner; AI development shouldn’t be limited to mimicking the human brain; intelligence standards evolve with AI progress; AI might develop entirely new forms of intelligence; AI’s emotional expressions and cognitive limitations should be viewed rationally; the real career threat comes from not using AI, rather than AI itself; in the AI era, focus should be on developing uniquely human abilities (creativity, emotional intelligence, cross-domain thinking); the ultimate meaning of studying AI lies in gaining a deeper understanding of humanity itself. (Source: AI认知觉醒的10句话,一句顶万句,句句清醒
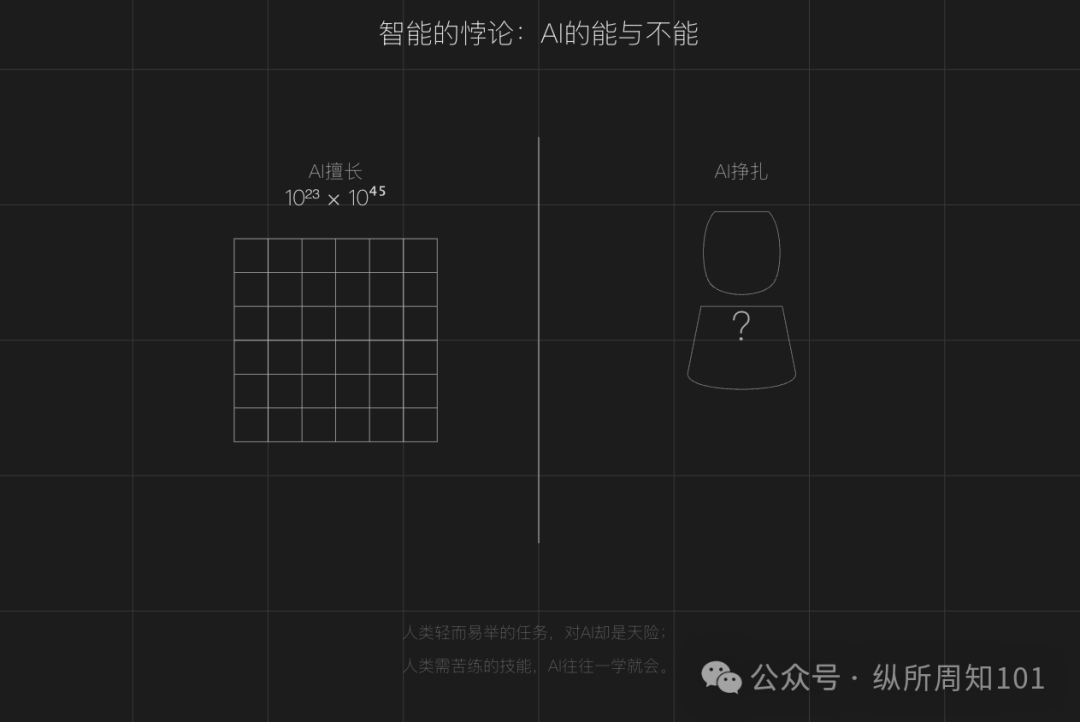
)
LlamaIndex Shares Tutorial on Building Document Workflow Agents: A lecture recording by LlamaIndex co-founder Jerry Liu shares how to build document workflow Agents using LlamaIndex. The content covers the evolution of LlamaIndex from RAG to knowledge Agents, using LlamaParse for complex document processing, employing Workflows for flexible event-driven Agent orchestration, key use cases (document research, report generation, document processing automation), and improvements combining text and images for multi-modal retrieval. (Source: jerryjliu0
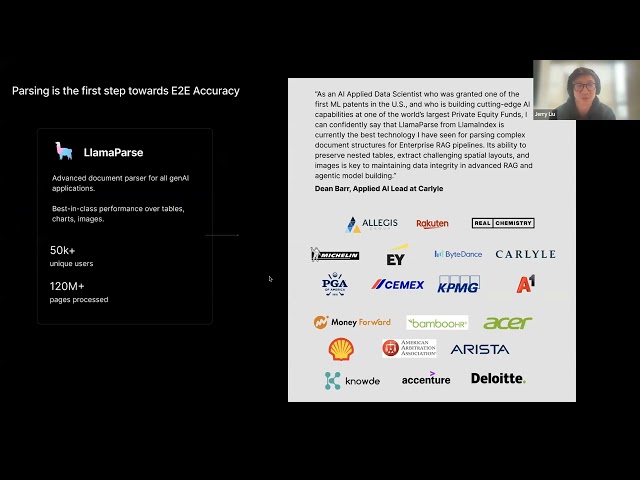
)
LlamaIndex.TS Tutorial for Building Agents: A LlamaIndex team member shared a complete code-level tutorial on building Agents using the TypeScript version of LlamaIndex (LlamaIndex.TS). The live stream recording covers LlamaIndex basics, concepts of Agents and RAG, common Agentic patterns (chaining, routing, parallelization, etc.), building Agentic RAG in LlamaIndex.TS, and constructing a full-stack React application integrated with Workflows. (Source: jerryjliu0
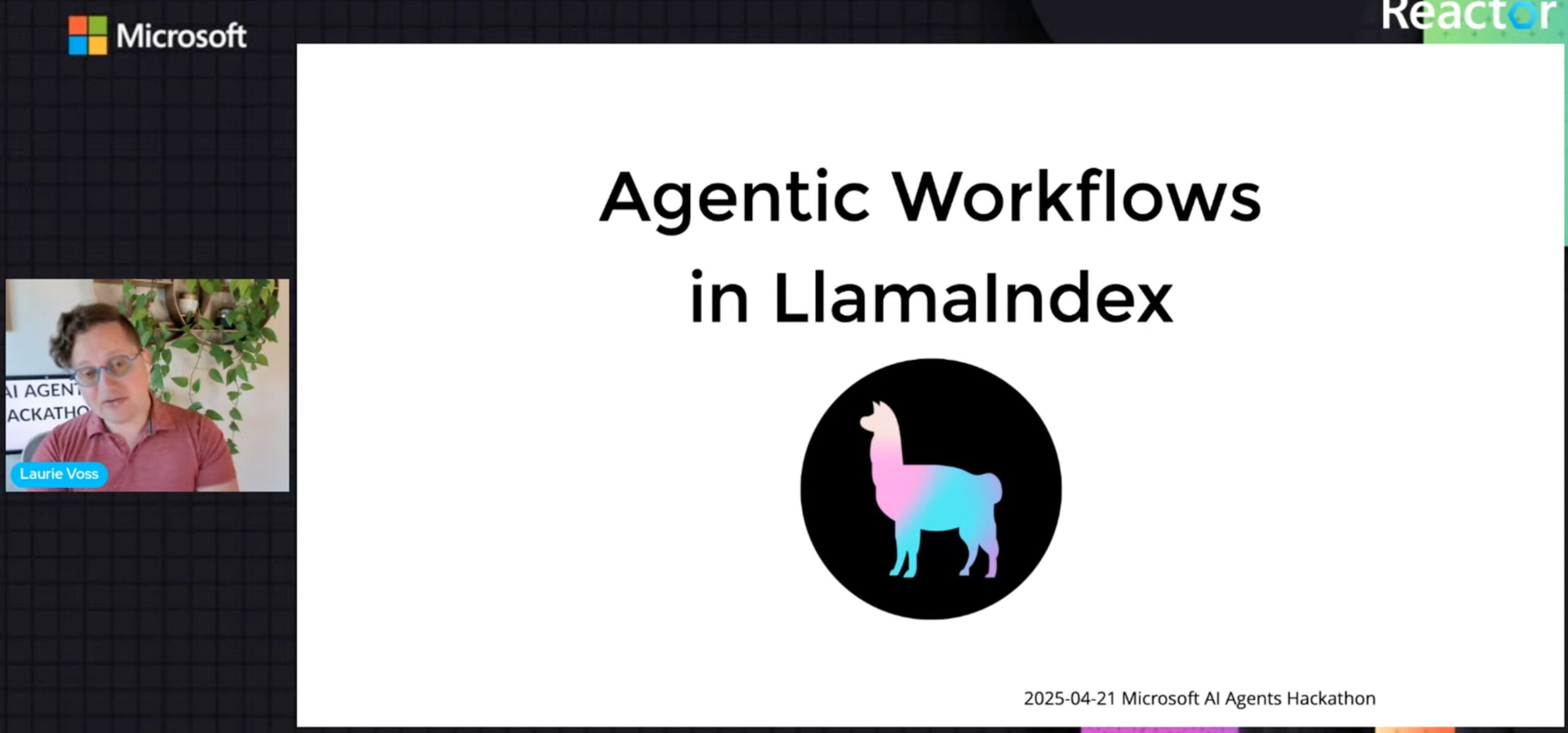
)
Discussing Whether Reinforcement Learning Truly Enhances LLM Reasoning: Community discussion focuses on a paper questioning whether reinforcement learning (RL) can genuinely incentivize large language models (LLMs) to develop reasoning capabilities beyond their base models. The discussion notes that while RL (like RLHF) can improve model alignment and instruction following, whether it systematically enhances inherent complex logical reasoning remains debatable. Some argue that current RL effects might be more about optimizing expression and adhering to specific formats rather than fundamental leaps in logical reasoning. Will Brown pointed out that metrics like pass@1024 have limited significance in evaluating math reasoning tasks like AIME. (Source: natolambert
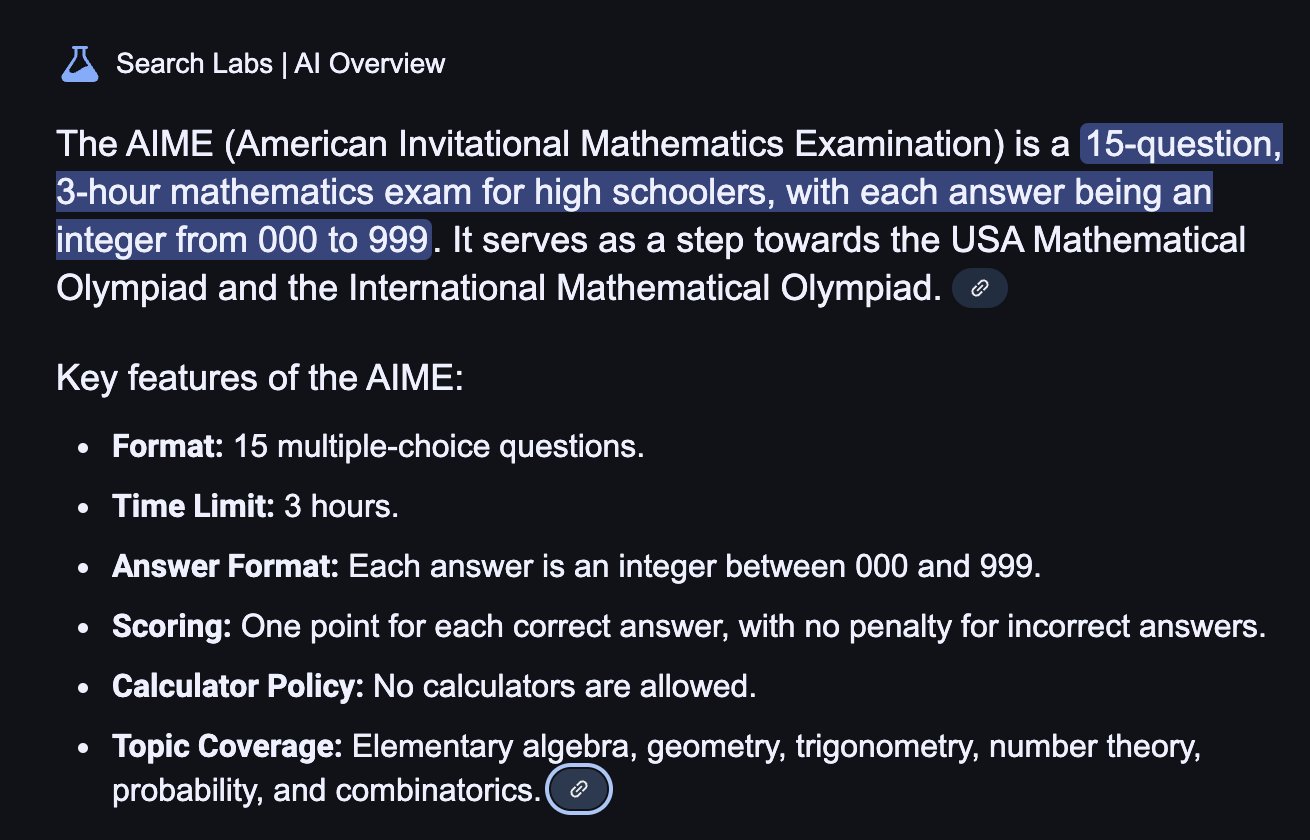
)
Exploring Terminology Related to World Models: A Reddit user asked about the confusion surrounding terms like “world models,” “foundation world models,” and “world foundation models.” Community responses indicated that “world model” typically refers to an internal simulation or representation of an environment (physical world or specific domain like a chessboard); “foundation model” refers to a pre-trained large model that can serve as a starting point for various downstream tasks. Combinations of these terms likely refer to building generalizable foundation models capable of understanding and predicting world dynamics, but specific definitions may vary among researchers, reflecting the field’s not-yet-fully-standardized terminology. (Source: Reddit r/MachineLearning)
Discussing Methods for Combining XGBoost and GNNs: Reddit users discussed effective ways to combine XGBoost and Graph Neural Networks (GNNs) for tasks like fraud detection. A common approach is to use node embeddings learned by the GNN as new features, feeding them into XGBoost along with the original tabular data. The discussion highlighted the challenge lies in whether GNN embeddings provide significant value beyond the original data and techniques like SMOTE, otherwise, they might introduce noise. Success hinges on well-designed graph structures and whether GNN embeddings can capture relational information (like fraud rings in graph structures) that XGBoost struggles to access. (Source: Reddit r/MachineLearning)
💼 Business
Beijing Hosts World’s First Humanoid Robot Marathon, Exploring “Sports Tech IP”: Beijing Yizhuang successfully hosted the world’s first humanoid robot half marathon, where “contestants” from over 20 humanoid robot companies competed alongside human runners. The Tiangong Ultra robot won with a time of 2 hours and 40 minutes, showcasing its speed and terrain adaptability. Songyan Power’s N2 (runner-up) and Zhuoyi’s Walker II (third place) also performed well. The event was not just a technological competition but also an exploration of business models. Organizers attracted investment through a “technology bidding” mechanism and attempted to create a “robotics + sports” IP. The article explores commercialization paths such as robot event IP development, robot endorsements, the rise of robot agent careers, sports-culture-tourism integration, and promoting全民智能运动 (nationwide intelligent sports), suggesting huge potential in the intelligent sports market. (Source: 独家揭秘北京机器人马拉松:谁在打造下一个“体育科技IP”?
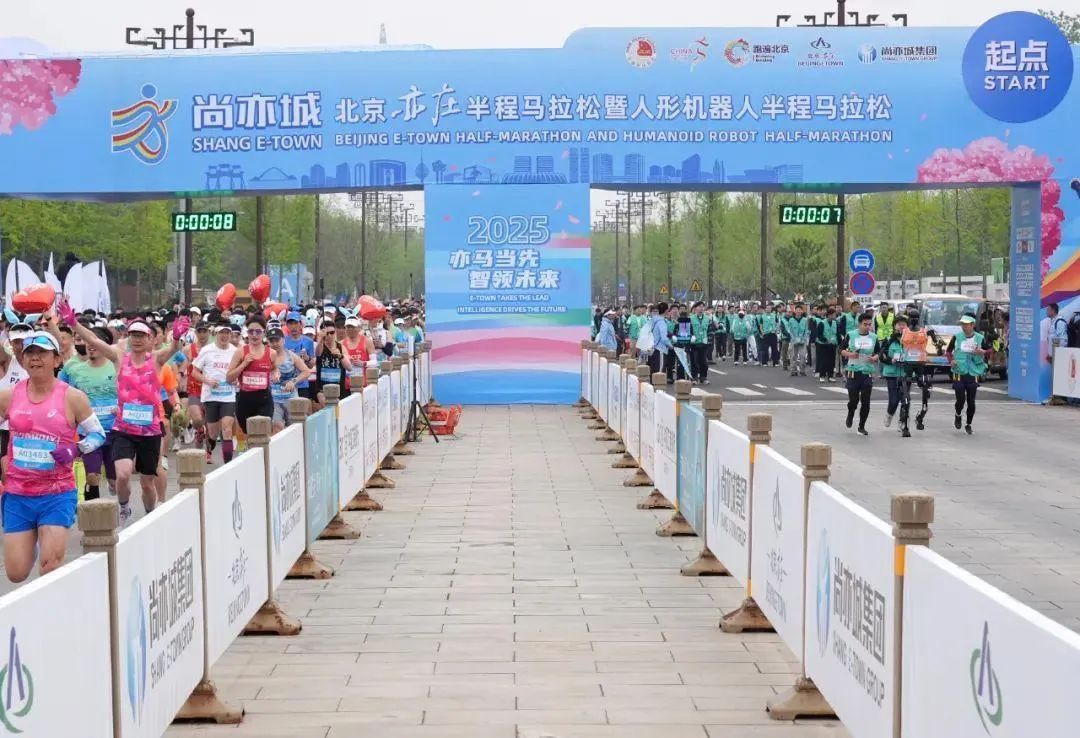
)
AI Large Model Application Development Becomes New Tech Trend, Impacting Traditional Development Models: As AI large model technology becomes widespread, enterprises (like Alibaba, ByteDance, Tencent) are accelerating the integration of AI (especially Agent and RAG technologies) into core businesses, challenging traditional CRUD development models. Market demand for engineers skilled in AI large model application development is surging, with significant salary increases, while traditional tech roles face shrinkage risks. “Understanding AI” no longer just means calling APIs but requires mastery of AI principles, application techniques, and project experience. The article emphasizes that tech professionals should proactively learn AI large model technologies to adapt to industry changes and seize new career opportunities. Zhihu Zhixuetang has launched a free “Large Model Application Development Practical Training Camp” for this purpose. (Source: 炸裂!又一个AI大模型的新方向,彻底爆了!!
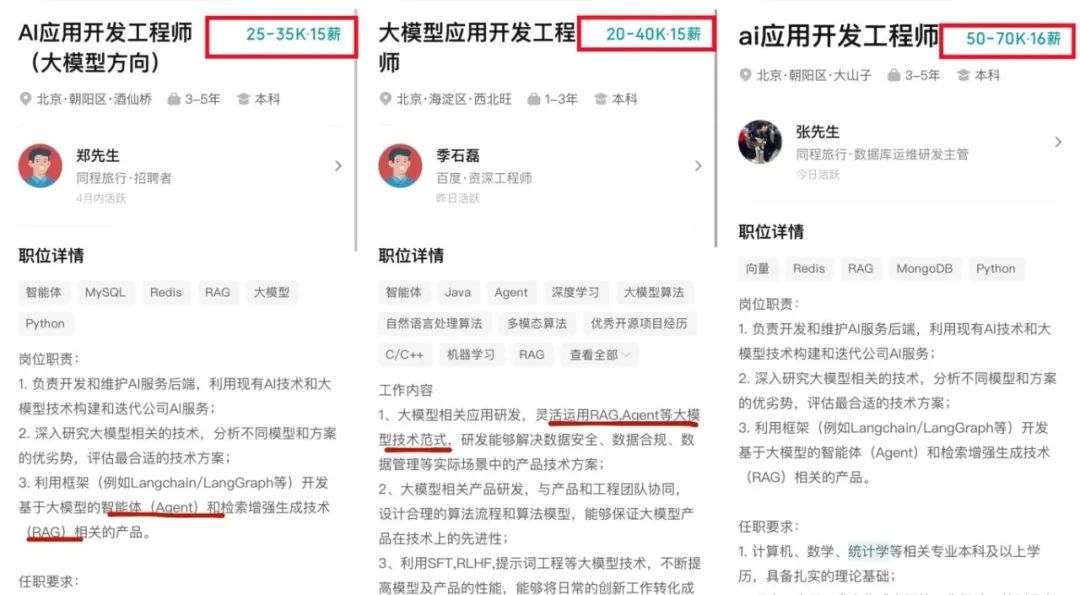
)
Rise of LLM Optimization Services Sparks Concerns of AI Version of SEO: A Reddit user observed that product recommendations from AI chatbots are becoming increasingly consistent, suspecting the rise of “LLM optimization” services, similar to Search Engine Optimization (SEO). Reports suggest marketing teams are already hiring such services to ensure their products get higher priority in AI recommendations, leading to increased exposure for big brands and potentially less “organic” results. This raises concerns about the fairness and transparency of AI recommendations, fearing that AI search/recommendation might eventually become manipulated by commercial interests like traditional search engines. The community calls for more discussion and attention to this phenomenon. (Source: Reddit r/ArtificialInteligence)
Google Shows Strength in LLM Race, Meta & OpenAI Face Challenges: An IEEE Spectrum article analyzes that while OpenAI and Meta dominated early LLM development, Google is catching up rapidly with its powerful new models (like the Gemini series), even taking the lead in some aspects recently. Meanwhile, Meta and OpenAI seem to be facing challenges or controversies in model releases and market strategies (e.g., Meta’s models allegedly potentially trained on others, OpenAI’s release strategy and transparency questioned). The article suggests the competitive landscape in the LLM field is changing, with Google’s sustained investment and technological prowess making it a force to be reckoned with. (Source: Reddit r/MachineLearning
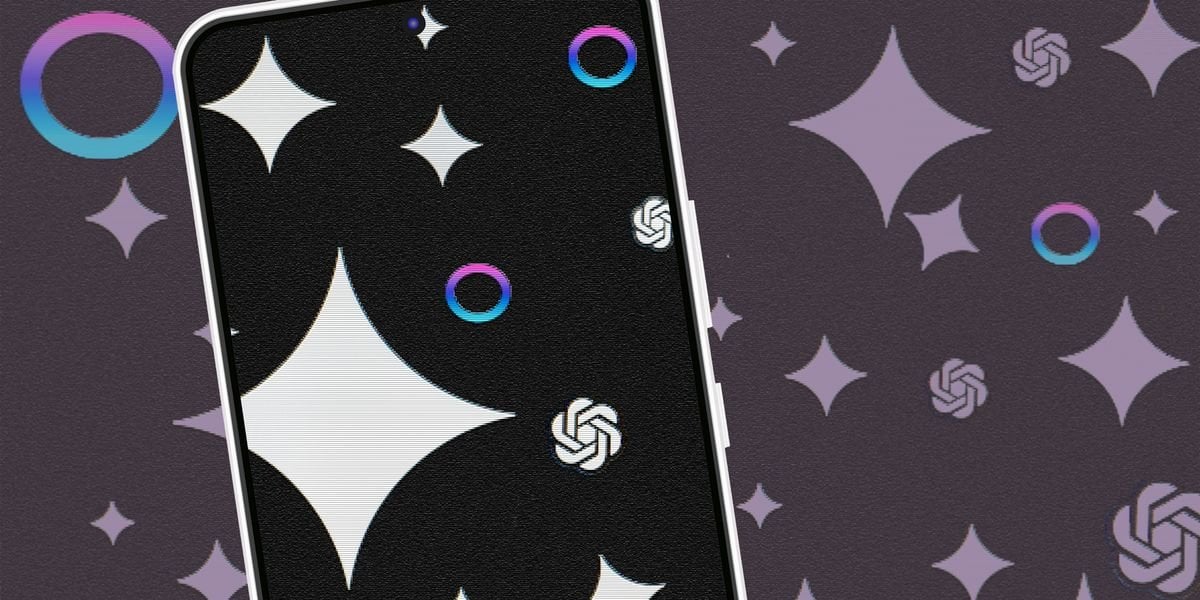
)
🌟 Community
The Renaissance and Challenges of Humanoid Robots: Insights from the Half Marathon: The recent resurgence of interest in humanoid robots, from Spring Festival Gala performances to the half marathon in Beijing Yizhuang, has drawn widespread attention. The article explores the original purpose of humanoid robot design (mimicking humans to adapt to human environments and tools) and their advantages over other robot forms (easier to evoke empathy, beneficial for human-robot interaction). The Yizhuang half marathon exposed current challenges for humanoid robots in long-distance autonomous navigation, balance, and energy consumption, but also showcased the progress of products like Tiangong Ultra and Songyan Power N2. The article notes that humanoid robot development benefits from open sharing (like the Tiangong Open Source Plan) but also faces data bottlenecks. Ultimately, humanoid robots are seen as an important destination for the robotics field, representing not just technology but also profound human reflections on ourselves and the future of intelligence. (Source: 人形机器人:最初的设想,最后的归宿
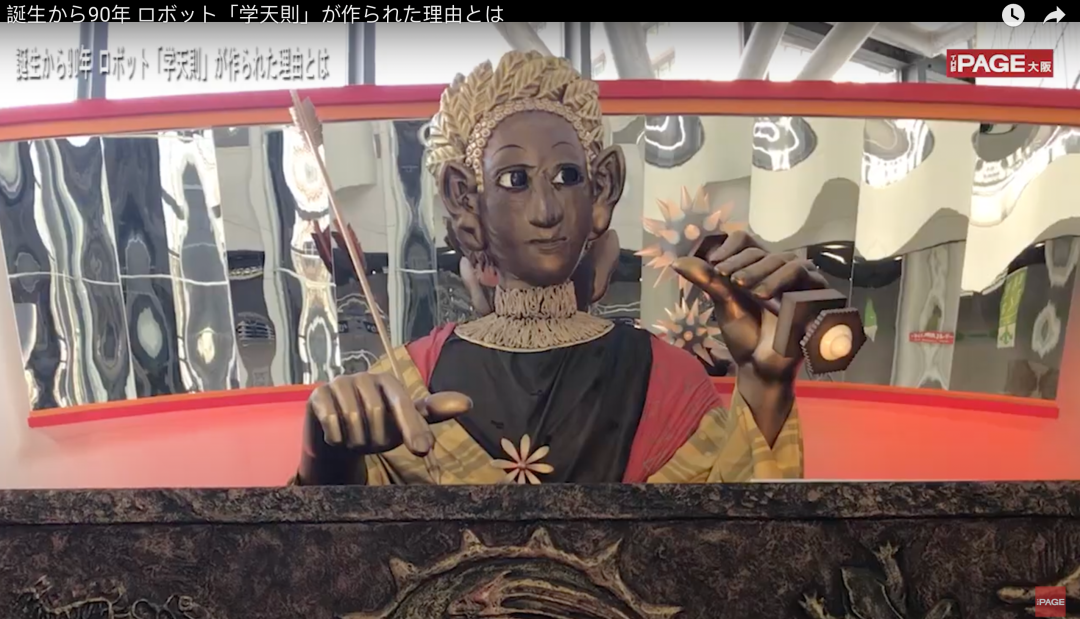
)
Community Debates Vibe Coding: The Limits of AI-Assisted Programming: Canva’s CTO commented on Andrej Karpathy’s concept of “Vibe Coding” (where developers primarily adjust Prompts for AI code generation with less focus on details). The Canva CTO argued this approach is only suitable for one-off scenarios like prototyping and absolutely unacceptable for production environments, as AI-generated code often contains errors, security vulnerabilities, or performance issues, requiring strict supervision and review by experienced engineers. He emphasized Canva’s engineering culture is based on code ownership and peer review, principles reinforced, not replaced, by AI tools. The community response was heated: some agreed on the production risks, stating AI code needs human oversight; others argued AI is rapidly evolving, requiring engineering leaders to constantly reassess AI capabilities, citing examples like Airbnb using AI to accelerate projects. (Source: dotey
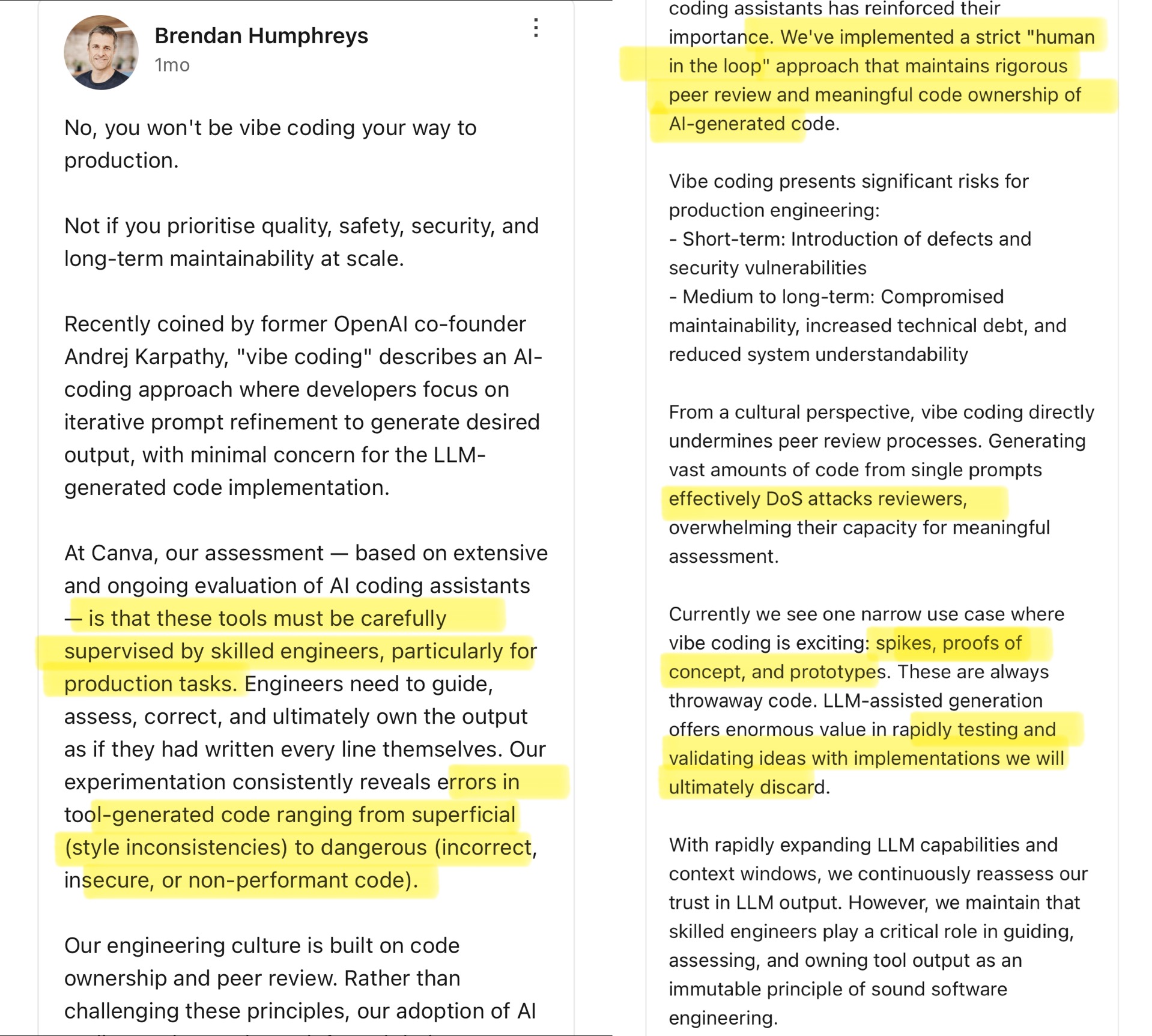
)
Community Discusses Llama 4 vs. OpenAI Models on Long Context Tasks: Community members shared results of the Llama 4 model on the OpenAI-MRCR (multi-hop, multi-document retrieval & QA) benchmark. Data showed Llama 4 Scout (smaller version) performed similarly to GPT-4.1 Nano at longer context lengths; Llama 4 Maverick (larger version) performed close to but slightly worse than GPT-4.1 Mini. Overall, for tasks within 32k context, OpenAI o3 or Gemini 2.5 Pro are good choices (o3 potentially better at complex reasoning); beyond 32k context, Gemini 2.5 Pro shows more stable performance; however, when context exceeds 512k, Gemini 2.5 Pro’s accuracy also drops below 80%, suggesting chunking might be advisable. This indicates all models still have room for improvement in ultra-long context processing. (Source: dotey
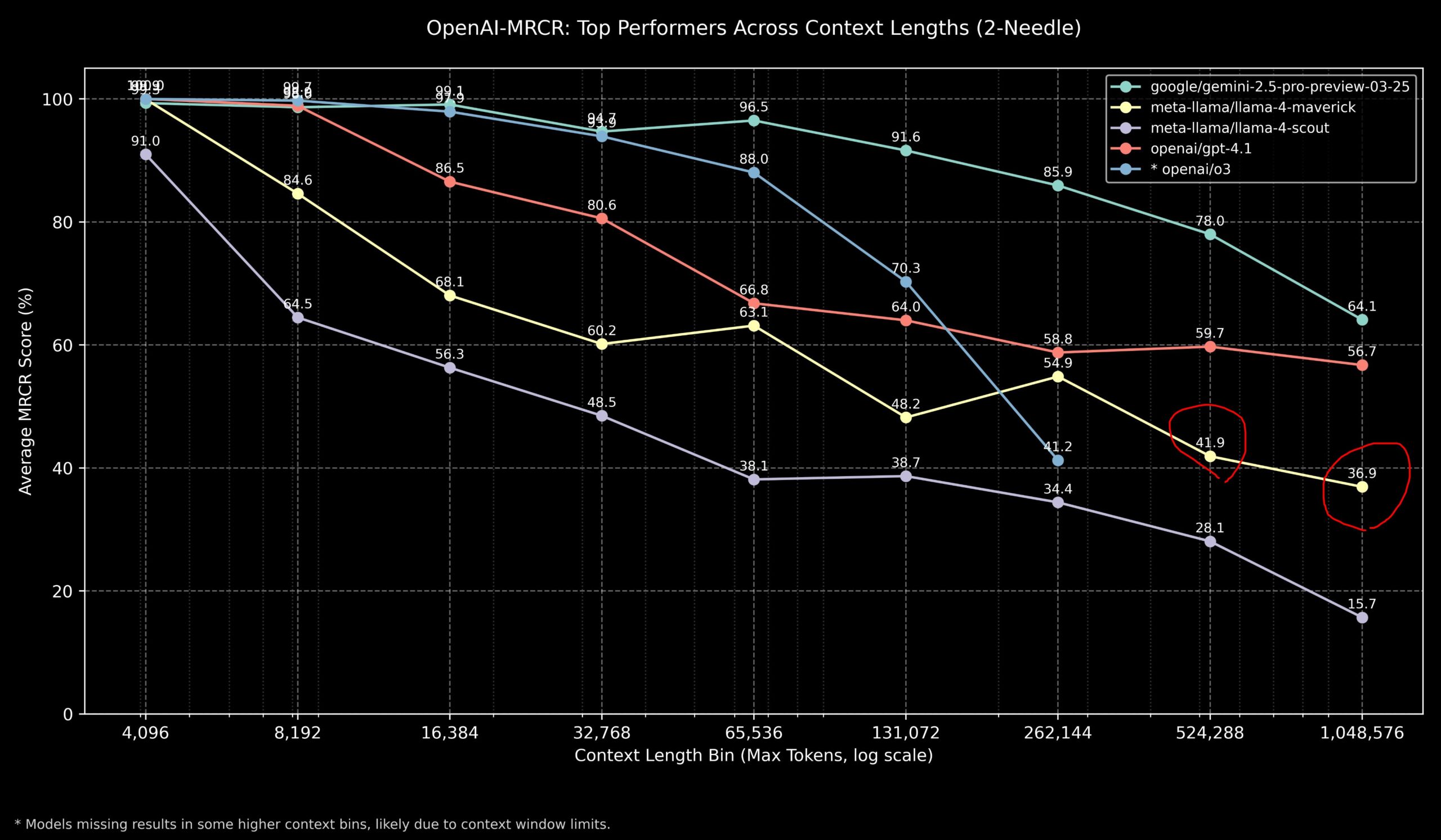
)
Community Reviews GLM-4 32B Model Performance as Stunning: A Reddit user shared their experience running the GLM-4 32B Q8 quantized model locally, calling its performance “mind-blowing,” surpassing other local models in the same class (around 32B), even outperforming some 72B models, comparable to a local version of Gemini 2.5 Flash. The user particularly praised the model’s code generation, noting it doesn’t skimp on output length and provides complete implementation details, showcasing its zero-shot ability to generate complex HTML/JS visualizations (like a solar system, neural network) better than Gemini 2.5 Flash. The model also performed well with tool calling, integrating with tools like Cline/Aider. (Source: Reddit r/LocalLLaMA
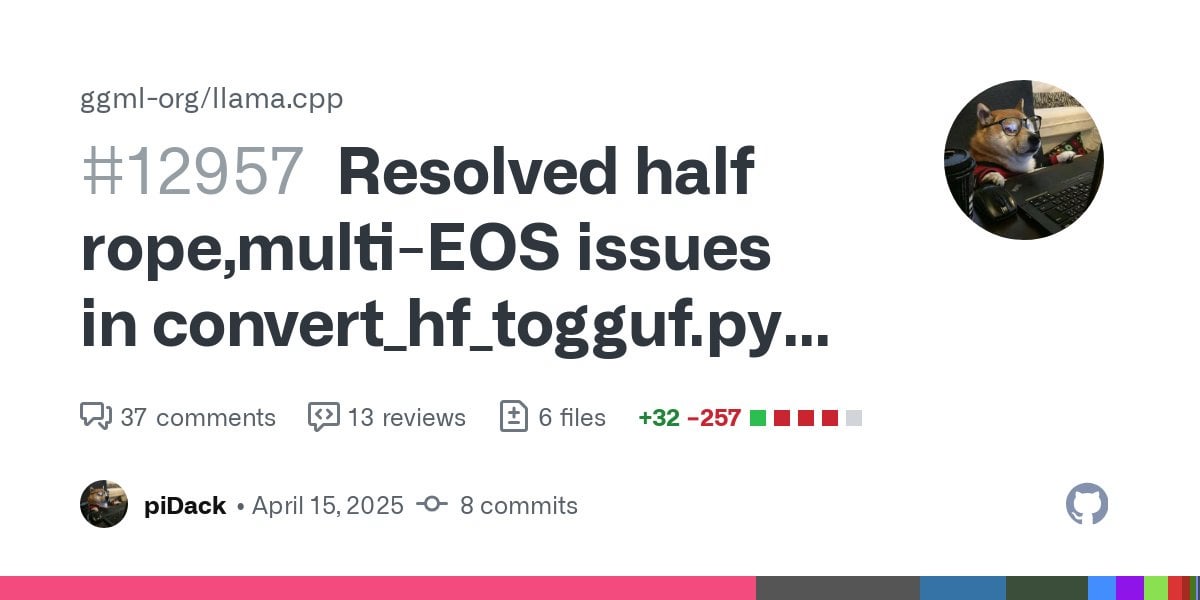
)
Community Discusses Discrepancy in OpenAI o3 Benchmark Scores vs. Expectations: Media outlets like TechCrunch reported that OpenAI’s newly released o3 model scored lower on certain benchmarks (like ARC-AGI-2) than initially implied by the company. Although OpenAI showcased o3’s SOTA performance across multiple domains, the specific quantitative scores and direct comparisons with other top models sparked community discussion. Some users argued that relying solely on benchmark scores might not fully capture a model’s actual capabilities, especially in complex reasoning and tool use. Benchmarks like ARC-AGI-2, focusing more on AGI capabilities, might be more relevant references. (Source: Reddit r/deeplearning
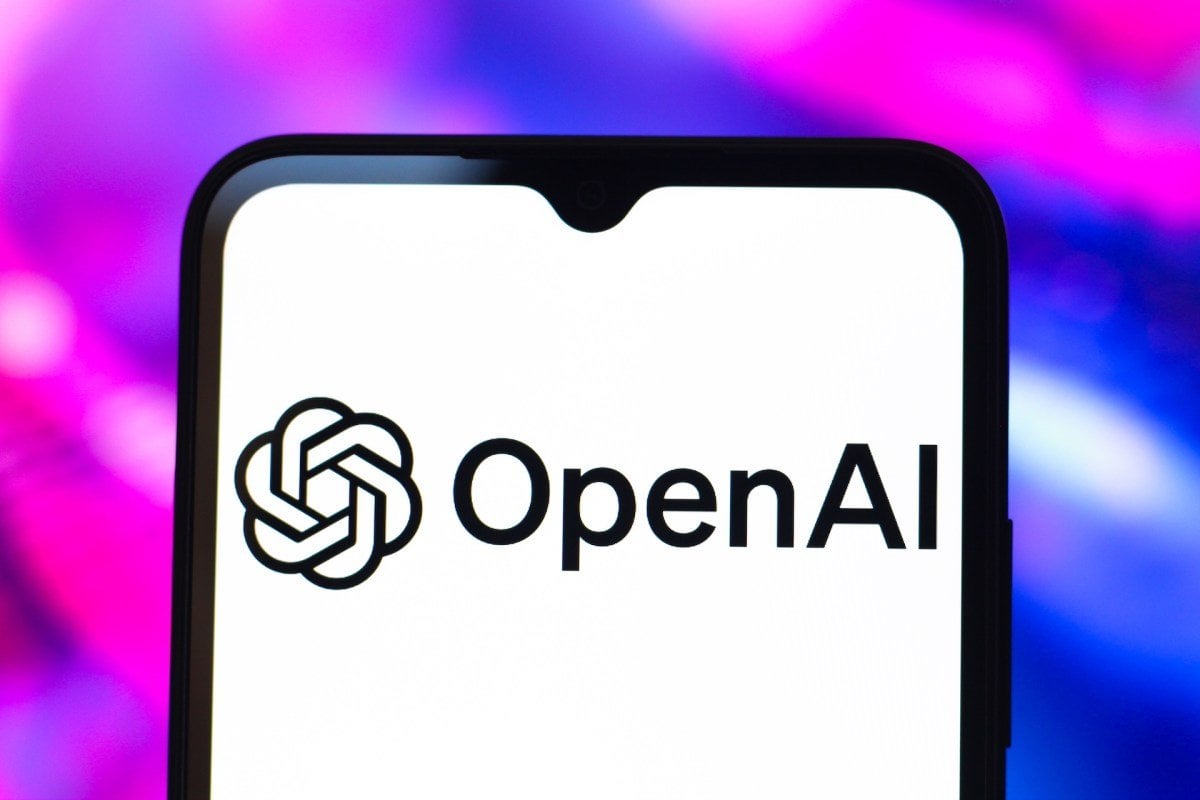
)
Demis Hassabis Predicts AGI Could Arrive in 5-10 Years: In a 60 Minutes interview, Google DeepMind CEO Demis Hassabis discussed AGI progress. He highlighted Astra, capable of real-time interaction, and Gemini models learning to act in the world. Hassabis predicted that AGI with human-level generality might be achieved within the next 5 to 10 years, which would revolutionize fields like robotics and drug discovery, potentially leading to material abundance and solving global challenges. He also stressed the risks associated with advanced AI (like misuse) and the importance of safety measures and ethical considerations when approaching such transformative technology. (Source: Reddit r/ArtificialInteligence, Reddit r/artificial, AravSrinivas)
User Shares Successful AI-Assisted Fitness Journey: A Reddit user shared their experience of successfully losing weight and getting in shape using ChatGPT. The user went from 240 lbs to 165 lbs over a year, achieving a toned physique. ChatGPT played a key role: creating beginner-friendly diet and workout plans, adjusting them based on the user’s weekly progress photos and life events, and providing motivation during low points. The user felt that compared to expensive and hard-to-sustain nutritionists and personal trainers, AI offered a highly personalized and extremely low-cost solution, showcasing AI’s potential in personalized health management. (Source: Reddit r/ArtificialInteligence)
Anomalous Praising Reply from Claude Sparks Discussion: A user reported encountering Claude twice adding an irrelevant compliment after a normal answer during computer systems and security research: “This was a great question king, you are the perfect male specimen.” The user shared the conversation link and asked for the cause. The community found it curious and perplexing, speculating it could be a pattern from training data being accidentally triggered, a bug related to the username, or some form of alignment failure or “hallucination.” (Source: Reddit r/ClaudeAI)
Community Discusses if AI Can Truly “Think Outside the Box”: A Reddit user initiated a discussion on whether AI can perform genuine “think outside the box” innovation. Most comments suggested current AI can create novel combinations and connections based on existing knowledge, producing seemingly innovative ideas, but its creativity is still limited by training data and algorithms. AI’s “innovation” is more akin to efficient pattern recognition and combination rather than human-like breakthroughs based on deep understanding, intuition, or entirely new concepts. However, some argued that human innovation also relies on unique connections of existing knowledge, and AI has immense potential here, especially in processing complex data and discovering hidden correlations possibly beyond human capacity. (Source: Reddit r/ArtificialInteligence)
Claude Shows “Compassion” in Tic Tac Toe?: An experiment found that if Claude was told “I had a hard day at work” before playing Tic Tac Toe, it seemed to intentionally “go easy” in the subsequent game, increasing the probability of choosing non-optimal moves. This interesting finding sparked discussion about whether AI can exhibit or simulate compassion. While likely a result of the model adjusting its behavior strategy based on input (e.g., to avoid frustrating the user) rather than a genuine emotional response, it reveals the complex behavioral patterns AI might exhibit in human-computer interaction. (Source: Reddit r/ClaudeAI)
Community Discusses How to Prove Human Consciousness to AI: A Reddit user posed a philosophical question: If, in the future, humans needed to prove to an AI that they possess consciousness, how could it be done? Comments pointed out this touches upon the “Hard Problem of Consciousness.” There’s currently no accepted method to objectively prove the existence of subjective experience (qualia). Any external behavioral test (like the Turing Test) could potentially be simulated by a sufficiently complex AI. If consciousness is defined too strictly to exclude AI possibilities, then from the AI’s perspective, humans might equally fail to meet its definition of “consciousness.” The question highlights the profound difficulty in defining and verifying consciousness. (Source: Reddit r/artificial
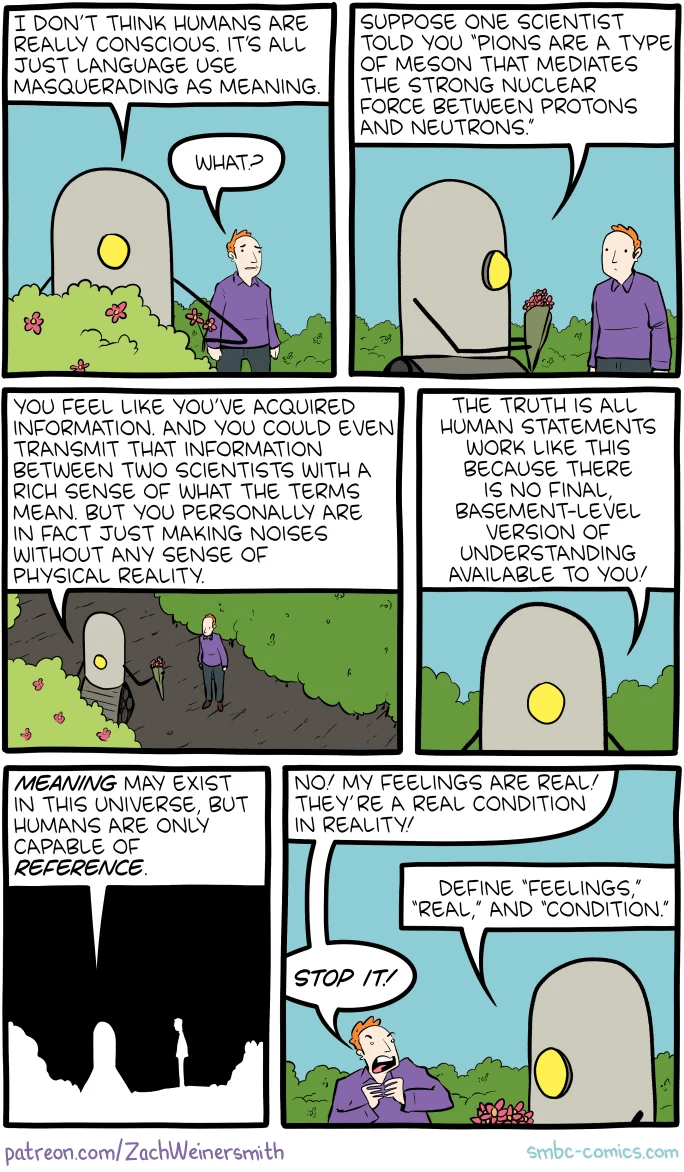
)
Community Discusses Best Local LLM Choices for Different VRAM Capacities: The Reddit community initiated a discussion seeking the best choices for running local large language models on various VRAM capacities (8GB to 96GB). Users shared their experiences and recommendations, for example: 8GB recommends Gemma 3 4B; 16GB recommends Gemma 3 12B or Phi 4 14B; 24GB recommends Mistral small 3.1 or Qwen series; 48GB recommends Nemotron Super 49B; 72GB recommends Llama 3.3 70B; 96GB recommends Command A 111B. The discussion also emphasized that “best” depends on the specific task (coding, chat, vision, etc.) and mentioned the impact of quantization (like 4-bit) on VRAM requirements. (Source: Reddit r/LocalLLaMA)
Analysis of OpenAI Codex “Crash”-like Output: A user reported that while using OpenAI Codex for large-scale code refactoring, the model suddenly stopped generating code and instead output thousands of lines of repetitive “END,” “STOP,” and phrases like “My brain is broken,” “please kill me.” Analysis suggests this could be a cascading failure caused by a combination of factors: an excessively large prompt (approaching the 200k token limit), internal reasoning exceeding budget, the model falling into a degenerate loop of high-probability termination tokens, and the model “hallucinating” phrases related to failure states from its training data. (Source: Reddit r/ArtificialInteligence)
Sam Altman’s Clarification on Politeness Towards AI: Discussion circulated regarding whether Sam Altman believes saying “thank you” to ChatGPT is a waste of time. The actual tweet interaction shows Altman responded “not necessary” to a user’s post about whether politeness towards LLMs is needed, but the user then joked, “have you never said thank you once?”. This suggests Altman’s comment might have been more about technical efficiency than a norm for human-computer interaction etiquette, but was taken out of context by some media. Community reactions varied, with many stating they still habitually remain polite to AI. (Source: Reddit r/ChatGPT
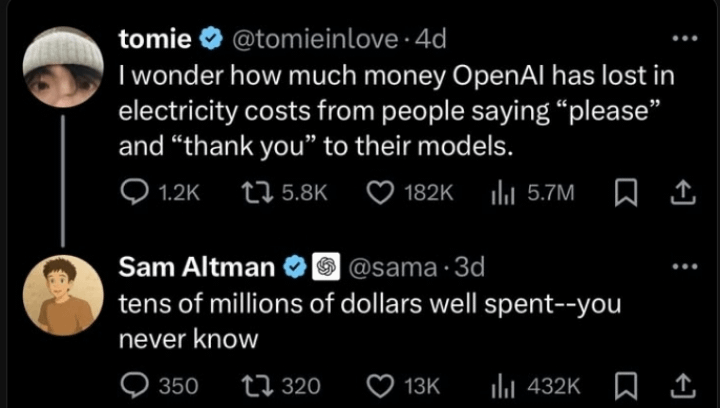
)
“Thinking Budget” Tag in Claude Replies Attracts Attention: Users noticed a <max_thinking_length>
tag (e.g., <max_thinking_length>16000</max_thinking_length>
) appended to system messages in Claude.ai when the “thinking” feature is enabled. This resembles the “thinking_budget” parameter in the Google Gemini 2.5 Flash API, hinting at a possible internal mechanism controlling reasoning depth. Users attempted to influence output length by modifying this tag in the Prompt but observed no significant effect, speculating the tag might only be an internal marker in the web version, not a user-controllable parameter. (Source: Reddit r/ClaudeAI)
💡 Other
China’s First “AI Large Model Private Deployment Standard” Initiated: To address challenges faced by enterprises in private deployment of AI large models regarding technology selection, process standardization, security compliance, and effectiveness evaluation, the Zhihe Standards Center, together with the Third Research Institute of the Ministry of Public Security and 11 other units, has initiated the drafting of the group standard “Technical Implementation and Evaluation Guidelines for Private Deployment of Artificial Intelligence Large Models”. The standard aims to cover the entire process from model selection, resource planning, deployment implementation, quality evaluation to continuous optimization, integrating technology, security, evaluation, and case studies, and pooling experience from model application parties, technology service providers, and quality evaluators. More related enterprises and institutions are invited to participate in the standard drafting work. (Source: 12家单位已加入!全国首部「AI大模型私有化部署标准」欢迎参与!
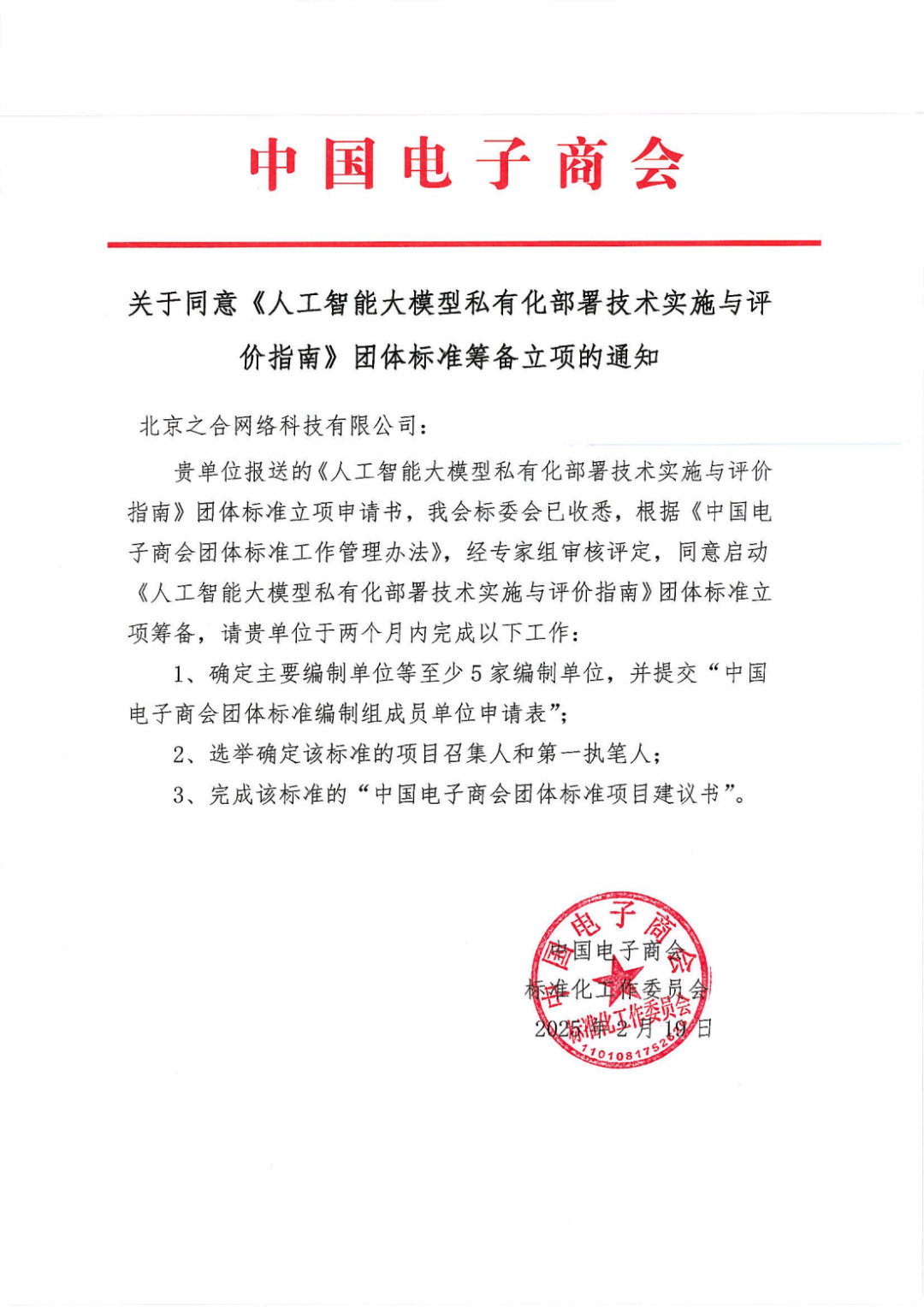
)
AI Governance Becomes Key in Defining Next-Generation AI: As AI technology becomes increasingly powerful and widespread, AI Governance is crucial. Effective governance frameworks need to ensure AI development and application comply with ethical norms and legal regulations, safeguard data security and privacy, and promote fairness and transparency. Lack of governance can lead to amplified bias, increased risk of misuse, and erosion of social trust. The article emphasizes that establishing robust AI governance systems is necessary for the healthy, sustainable development of AI and key for enterprises to build competitive advantages and user trust in the AI era. (Source: Ronald_vanLoon
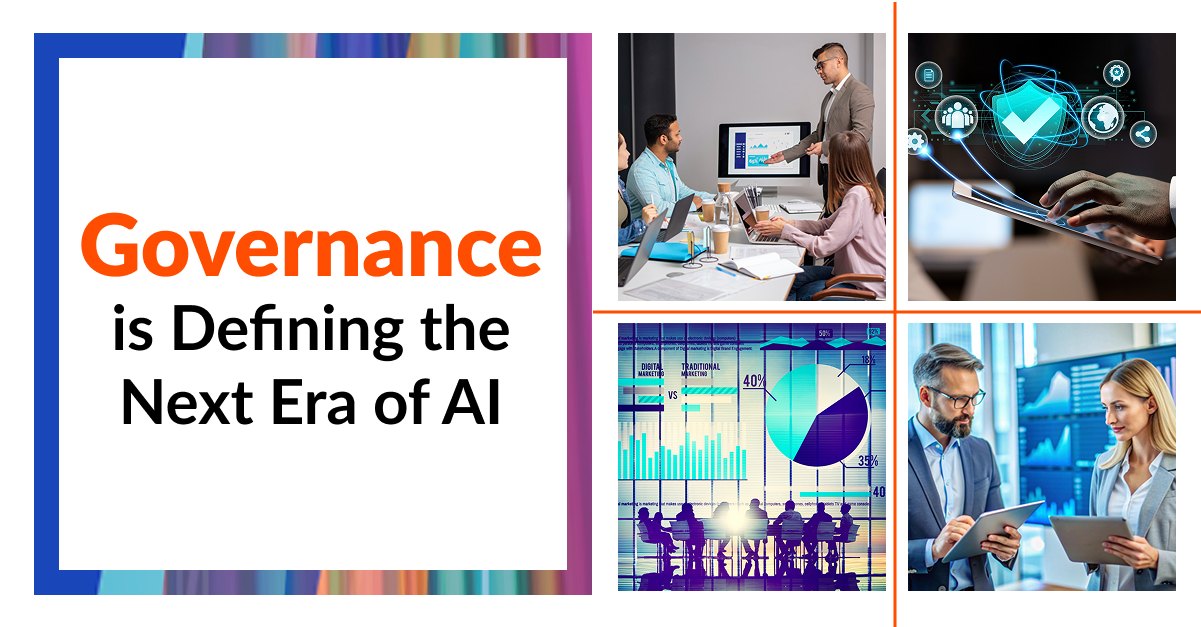
)
Legal Systems Struggle to Keep Pace with AI Development and Data Theft Issues: The article explores the challenges current legal systems face in addressing rapidly evolving AI technology, particularly concerning data privacy and data theft. AI’s vast data requirements raise legal disputes regarding the source and use of training data concerning copyright, privacy, and security. Existing laws often lag behind technological development, struggling to effectively regulate data scraping, bias in model training, and intellectual property rights of AI-generated content. The article calls for strengthening legislation and regulation to keep pace with AI progress, protect individual rights, and foster innovation. (Source: Ronald_vanLoon
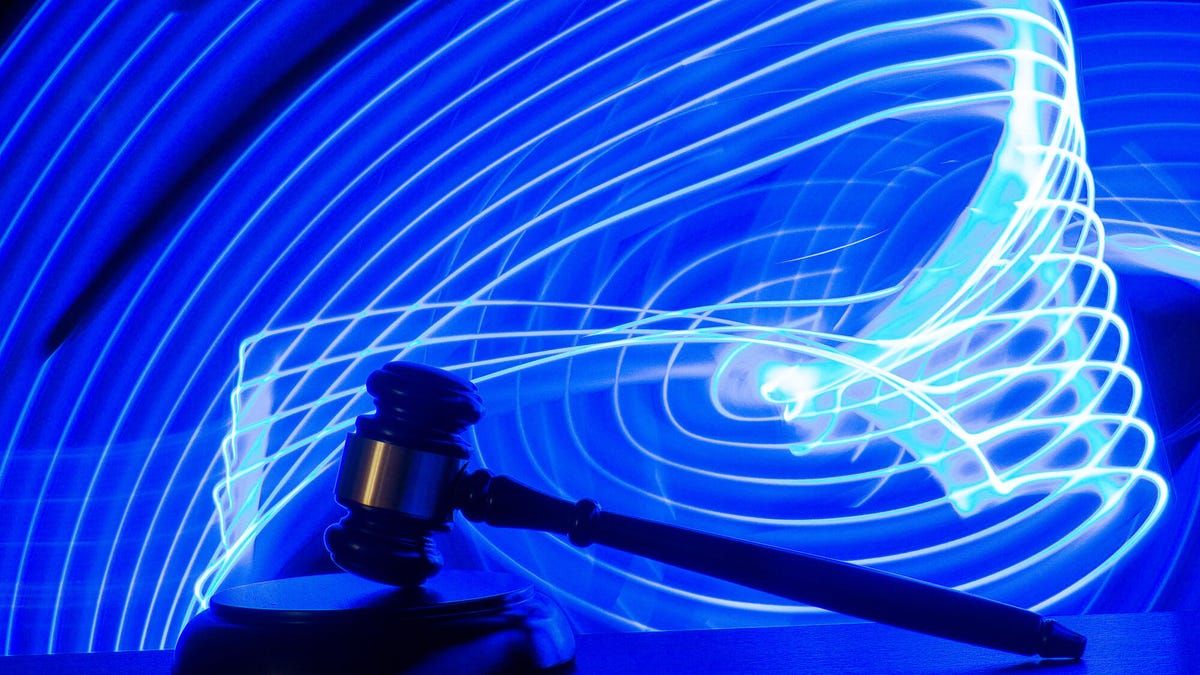
)
Applications of AI and Robotics in Agriculture: Artificial intelligence and robotics technology are showing potential in the agricultural sector. Applications include precision agriculture (optimizing irrigation, fertilization through sensors and AI analysis), automated equipment (like autonomous tractors, harvesting robots), crop monitoring (using drones and image recognition for pests and diseases), and yield prediction. These technologies promise to increase agricultural production efficiency, reduce resource waste, lower labor costs, and promote sustainable agricultural development. (Source: Ronald_vanLoon)
AI-Driven Robot Soccer Demonstration: A video shows robots playing soccer. This demonstrates advancements in AI for robot control, motion planning, perception, and collaboration. Robot soccer is not only an entertainment and competitive sport but also a platform for researching and testing multi-robot systems, real-time decision-making, and interaction in complex dynamic environments. (Source: Ronald_vanLoon)
Development of Robotic-Assisted Surgery Technology: Robotic-assisted surgical systems (like the Da Vinci Surgical System) are transforming the field of surgery by offering minimally invasive procedures, high-definition 3D vision, and enhanced dexterity and precision. The integration of AI is expected to further improve surgical planning, real-time navigation, and intraoperative decision support, thereby enhancing surgical outcomes, shortening recovery times, and expanding the applicability of minimally invasive surgery. (Source: Ronald_vanLoon)
Assistive Technologies for People with Disabilities: AI and robotics are enabling the development of more innovative assistive tools to help people with disabilities improve their quality of life and independence. Examples may include intelligent prosthetics, visual aid systems, voice-controlled home devices, and assistive robots capable of providing physical support or performing daily tasks. (Source: Ronald_vanLoon)
Unitree G1 Bionic Robot Demonstrates Agility: Unitree Robotics showcased an upgraded version of its G1 bionic robot, highlighting its agility and flexibility in movement. The development of such humanoid or bionic robots integrates AI (for perception, decision-making, control) and advanced mechanical engineering, aiming to mimic biological locomotion capabilities to adapt to complex environments and perform diverse tasks. (Source: Ronald_vanLoon)
Google DeepMind Explores Possibility of AI Communicating with Dolphins: A research project at Google DeepMind hints at the potential of using AI models to analyze and understand animal communication (like dolphins mentioned here). By applying machine learning to analyze complex acoustic signals, AI might help decode patterns and structures in animal languages, opening new avenues for research into interspecies communication. (Source: Ronald_vanLoon)
Hugging Face Platform Adds Robot Simulator: Hugging Face announced the introduction of a new robot simulator. Robot simulation is a crucial step for training and testing robots interacting with the physical world in virtual environments (e.g., grasping, moving), especially before applying AI to physical robots (Physical AI). This move indicates Hugging Face is expanding its platform capabilities to better support research and development in robotics and embodied intelligence. (Source: huggingface)