Keywords:AI, GPT-4.1, Zhipu AI IPO, NVIDIA AI supercomputing investment, Amazon AI capital expenditure, AI Agent interoperability protocol, DeepSeek user base
🔥 Focus
OpenAI Releases GPT-4.1 Series Models, Enhances API Performance, and Deprecates GPT-4.5: On April 15th, OpenAI released three new models via API: GPT-4.1, GPT-4.1 mini, and GPT-4.1 nano, aiming to comprehensively surpass the GPT-4o series. The new models feature a context window of up to 1 million tokens and a knowledge cutoff updated to June 2024. GPT-4.1 shows strong performance in coding ability (SWE-bench Verified score of 54.6%, a 21.4% improvement over GPT-4o), instruction following (MultiChallenge score of 38.3%, a 10.5% improvement over GPT-4o), and long-context video understanding (Video-MME score of 72.0%, a 6.7% improvement over GPT-4o). Notably, GPT-4.1 nano is the first nano model, outperforming GPT-4o mini at a lower cost. Concurrently, OpenAI announced the deprecation of the GPT-4.5 Preview API in 3 months (July 14th), describing it as a research preview and stating that developer-favored features will be integrated into new models. This release is seen as a strategic move by OpenAI to differentiate its API models from the ChatGPT product line and directly compete with Google’s Gemini series. (Source: 36Kr, Xin Zhi Yuan 1, AI Tech Review, Reddit r/LocalLLaMA, Reddit r/artificial)
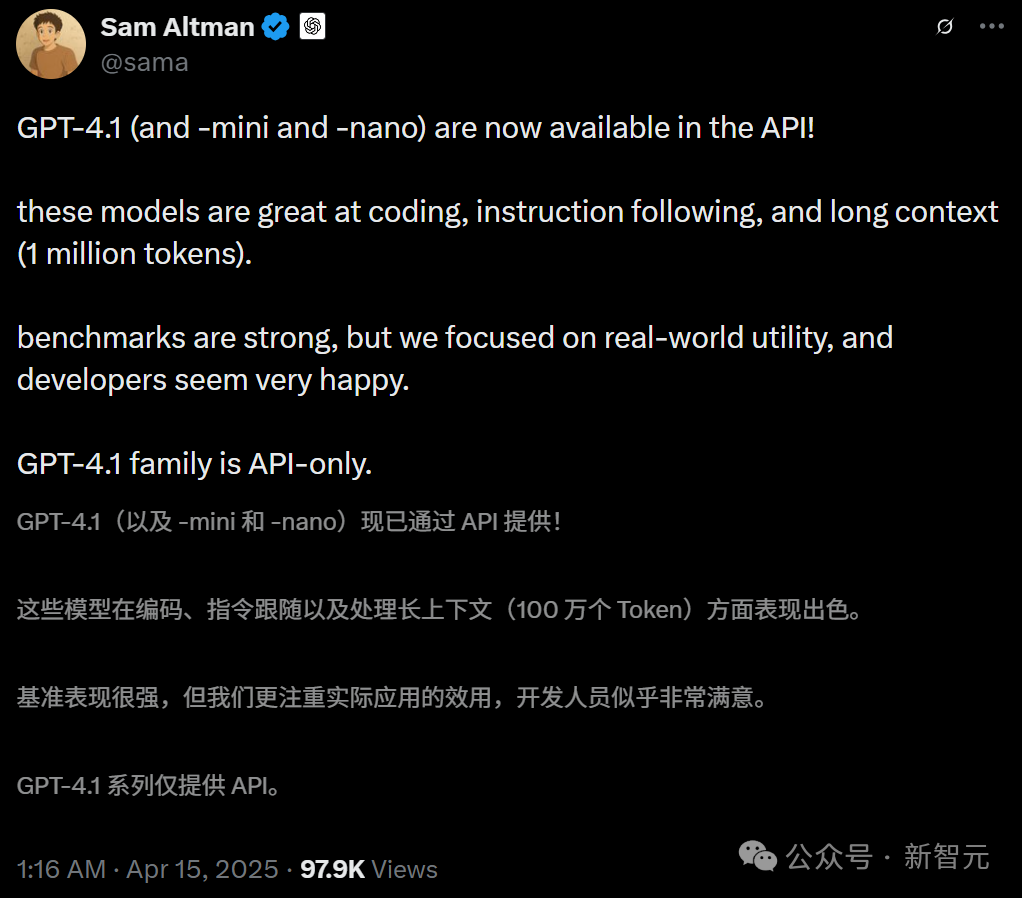
Zhipu AI Initiates IPO Counseling and Open-Sources New Models, Valuation Exceeds 20 Billion RMB: Zhipu AI (Zhipu Huazhang), one of China’s “Six Little Tigers” in the large model space, filed for IPO counseling guidance with the Beijing Securities Regulatory Bureau on April 14th, officially starting its IPO process with CICC as the counseling institution. Incubated from Tsinghua University’s Knowledge Engineering Lab, Zhipu AI’s core team members are mostly from Tsinghua. It has raised over 15 billion RMB in funding, with a recent valuation exceeding 20 billion RMB. Concurrently with the IPO initiation, Zhipu AI announced the large-scale open-sourcing of its GLM-4-32B/9B series models, including base, inference, and contemplation types, under the MIT license for free commercial use. Among them, the 32B parameter inference model GLM-Z1-32B-0414 rivals the performance of the 671B parameter DeepSeek-R1 on some tasks. Its API express version, GLM-Z1-AirX, achieves an inference speed of 200 tokens/s, and the cost-effective version is priced at only 1/30th of DeepSeek-R1’s. The company also launched a new domain, z.ai, as a platform for free model trials. This move demonstrates Zhipu AI’s comprehensive strategy in technology self-development, commercial exploration, and open-source ecosystem building. (Source: Zhidongxi, InfoQ, Quantumbit, GeekPark, Leidi, 公众号)
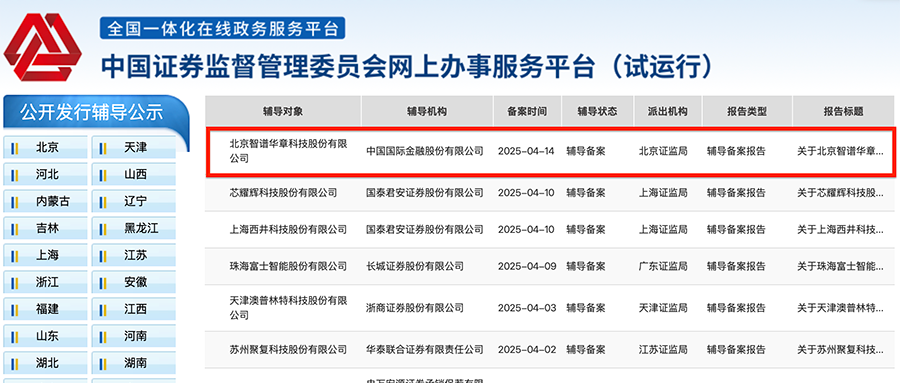
Nvidia to Invest $500 Billion in US-Based AI Supercomputer Manufacturing: Nvidia announced a major plan to invest $500 billion over the next four years to manufacture AI supercomputers domestically in the United States for the first time. The plan involves collaboration with several industry giants, including TSMC (producing Blackwell chips in Arizona), Foxconn and Wistron (building supercomputer factories in Texas), and Amkor and SPIL (conducting packaging and testing in Arizona). Nvidia CEO Jensen Huang stated that this initiative aims to meet the growing demand for AI chips and supercomputers, enhance supply chain resilience, and leverage Nvidia’s AI, robotics (Isaac GR00T), and digital twin (Omniverse) technologies to design and operate the factories. The plan is seen as a strategic deployment under the backdrop of the US government’s push for domestic manufacturing (like the CHIPS Act) and geopolitical considerations, aiming to bolster the US position in the global AI infrastructure race. However, it faces challenges such as supply chain complexity, skilled worker shortages, and policy uncertainties. (Source: Xin Zhi Yuan 1, Xin Zhi Yuan 2, Reddit r/artificial)
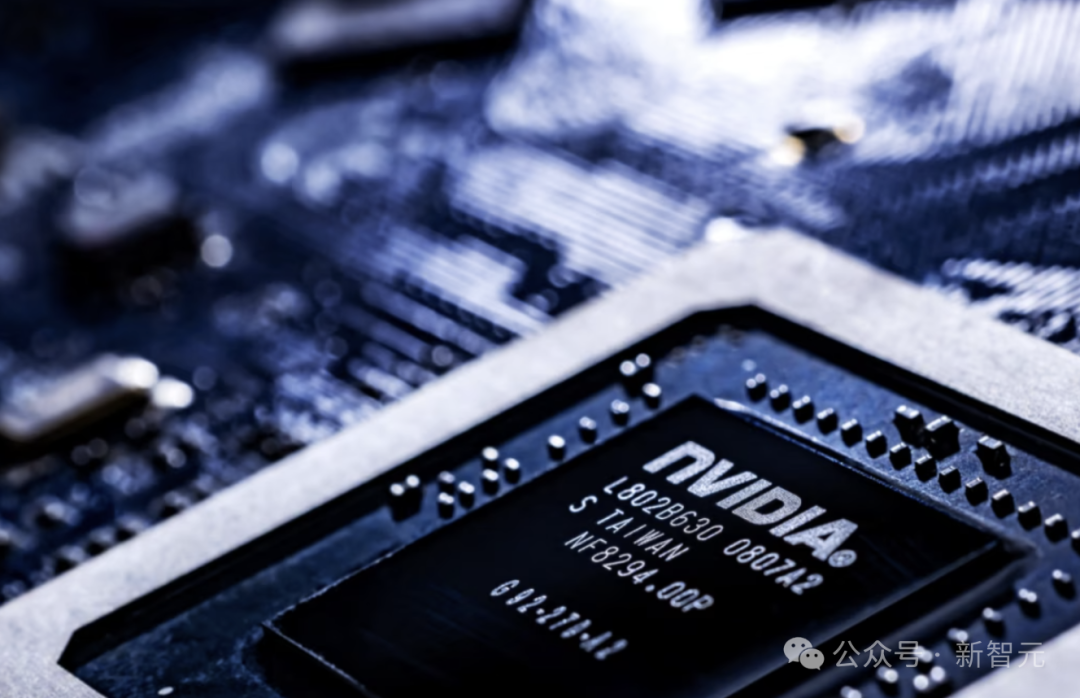
Amazon Plans Over $100 Billion Investment to Boost AI Amid Competition and Opportunity: In his 2024 annual letter to shareholders, Amazon CEO Andy Jassy revealed the company plans capital expenditures exceeding $100 billion in 2025, with the majority allocated to AI-related projects. This includes data centers, networking equipment, AI hardware (like its proprietary Trainium chips), and generative AI services (such as its own Nova series large models, Bedrock platform, upgraded Alexa+, and shopping assistant Rufus). This massive investment (nearly 1/6th of annual revenue) reflects Amazon’s view of AI as crucial for tackling fierce competition in e-commerce (from SHEIN, Temu, TikTok, etc.) and seizing a historic opportunity. Jassy emphasized that AI will change the rules for search, programming, shopping, etc., and failing to invest means losing competitiveness. Amazon’s AI business currently generates billions in annual revenue with triple-digit year-over-year growth. This move also shows Amazon’s determination to maintain its leading position in cloud services (AWS) amidst competition from rivals like Microsoft Azure and Google Cloud by continuing substantial investments. (Source: 36Kr)
🎯 Trends
AI Agent Interoperability Protocols MCP and A2A Gain Attention: The AI agent field is witnessing competition in standardized interaction protocols. Anthropic’s MCP (Model Context Protocol), designed to unify communication between large models and external tools/data sources, is hailed as the “USB-C of AI” and has garnered support from OpenAI, Google, etc. Google, meanwhile, has open-sourced the A2A (Agent2Agent) protocol, focusing on secure and efficient collaboration between agents from different vendors and frameworks, aiming to break down ecosystem barriers. The emergence of these two protocols signifies AI’s evolution from monolithic intelligence to collaborative networks, but also sparks discussions about “protocol is power,” data monopolies, and ecosystem barriers (“walled gardens”). Gaining control over standard-setting could reshape the AI industry chain and profoundly impact the integration of AI with the physical world (robotics, IoT). Domestic vendors like Alibaba Cloud and Tencent Cloud have also begun supporting MCP. (Source: 36Kr)
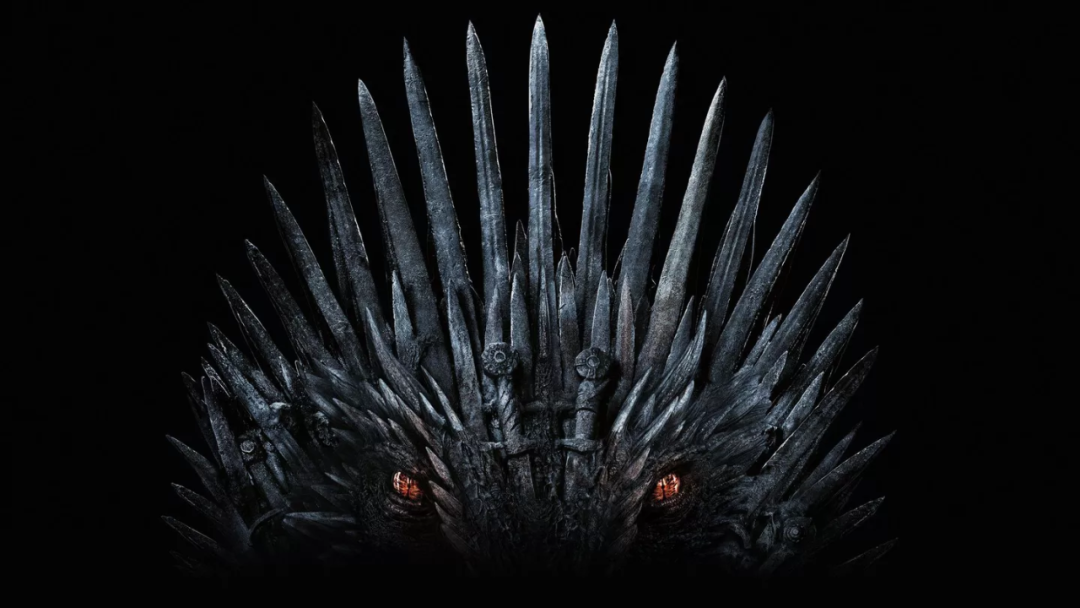
QuestMobile Report: DeepSeek Disrupts China’s AI App Landscape, User Base Reaches 240 Million: QuestMobile’s “Q1 2025 AI Application Market Competition Analysis” report shows that the domestic native AI App market landscape has been completely disrupted, driven by the explosive popularity of the DeepSeek model and its applications. As of the end of February 2025, the monthly active user (MAU) base of native AI Apps reached 240 million, nearly a 90% increase from January. The DeepSeek App topped the list with 194 million MAU, followed by ByteDance’s Doubao (116 million) and Tencent’s Yuanbao (41.64 million), displacing previous leaders like Kimi. The report notes that DeepSeek’s open-source and inclusive effect spurred adoption by top players and fueled an AI application boom, creating 23 tracks including AI general assistants and AI search, with AI search being the most competitive. Currently, “multi-model driven” has become standard for top apps, with competition shifting towards product design and operation. (Source: QuestMobile)
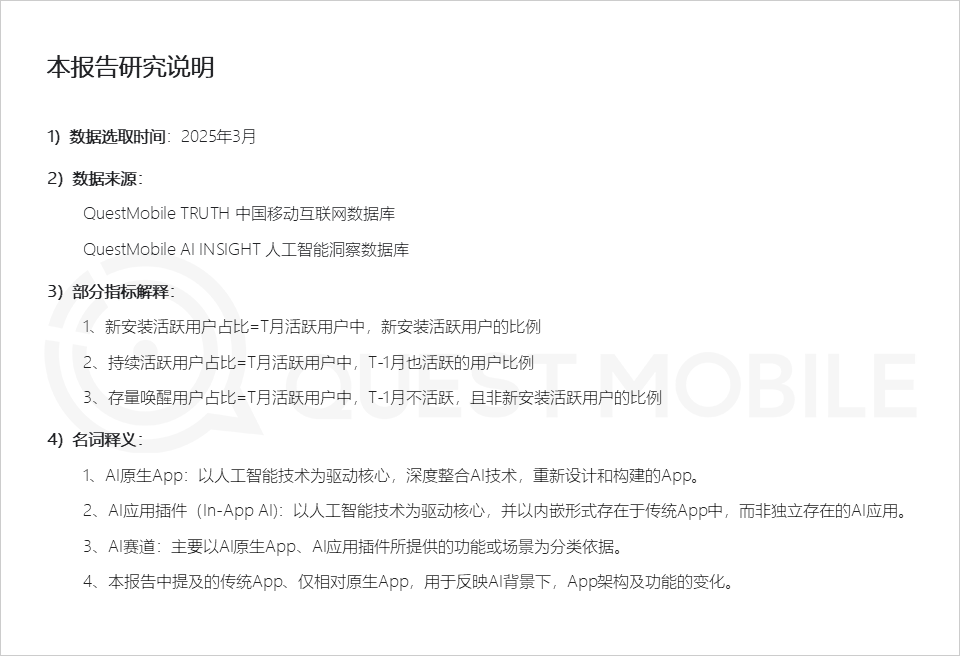
Zxiang Future Open-Sources 17B Text-to-Image Model HiDream-I1, Comparable to GPT-4o: Chinese company Zxiang Future has open-sourced its 17B parameter text-to-image large model, HiDream-I1, under the permissive MIT license, allowing commercial use. The model has shown excellent performance in arenas and benchmarks (like HPSv2.1, GenEval, DPG-Bench) on platforms such as Artificial Analysis. The realism, detail, and instruction-following ability of the generated images are considered comparable to GPT-4o and FLUX 1.1 Pro, even surpassing them in some aspects. HiDream-I1 uses a Sparse Diffusion Transformer (Sparse DiT) architecture, incorporating MoE technology to enhance performance and efficiency. The company also announced the upcoming open-sourcing of the HiDream-E1 model, which supports interactive image editing. The combination aims to provide an “open-source GPT-4o” experience for image generation and editing. The model is available on Hugging Face and can be experienced on the Vivago platform. (Source: Machine Heart 1, Machine Heart 2)
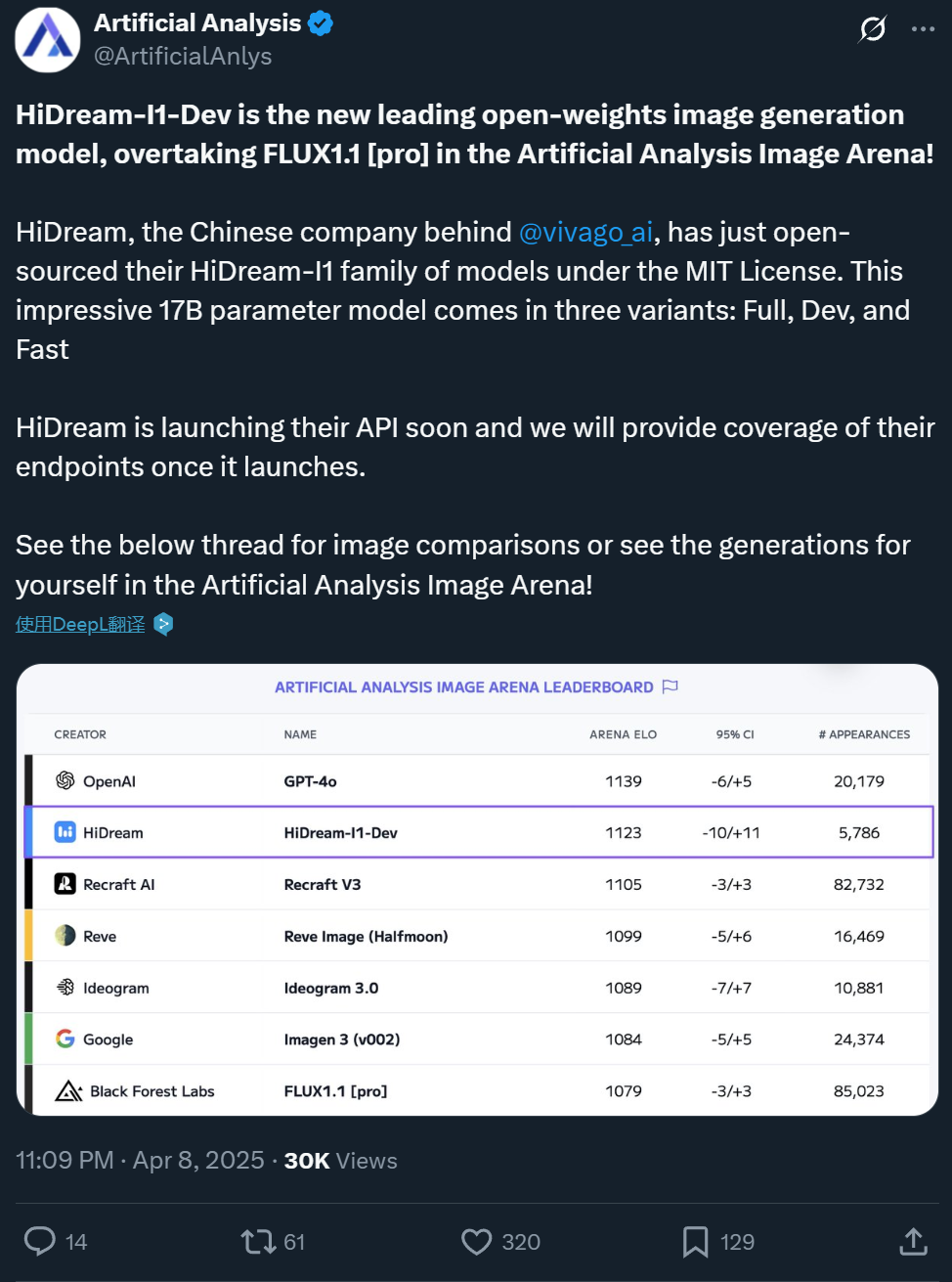
ByteDance Releases 7B Video Foundation Model Seaweed for Low-Cost, High-Efficiency Generation: ByteDance’s Seed team has released a video generation foundation model named Seaweed (a pun on Seed-Video). The model has only 7 billion parameters and was reportedly trained using 665,000 H100 GPU hours (equivalent to about 28 days on 1000 cards), making it relatively low-cost. Seaweed can generate videos of varying resolutions (natively supports 1280×720, upscalable to 2K), arbitrary aspect ratios, and durations based on text prompts. The model supports image-to-video generation, reference subject control (single/multiple images), integration with the digital human solution Omnihuman for lip-synced video generation, and video dubbing. Technically, it employs a DiT+VAE architecture, combined with a comprehensive data processing pipeline and multi-stage, multi-task training strategy (pre-training, SFT, RLHF), along with system-level optimizations for training efficiency. The team is led by Dr. Lu Jiang, former head of video generation at Google, among others. (Source: Quantumbit)
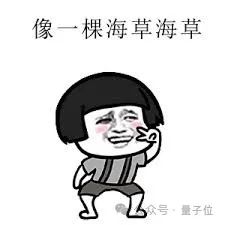
Alibaba Tongyi Releases Digital Human Video Generation Model OmniTalker: Alibaba’s Tongyi Lab HumanAIGC team has launched a new digital human video generation large model, OmniTalker. The model aims to address issues like latency, audio-visual desynchronization, and style inconsistency inherent in traditional cascaded methods (TTS + audio-driven). OmniTalker is an end-to-end unified framework that takes text and a reference audio/video clip as input to generate synchronized speech and digital human video in real-time, while preserving the voice and facial speaking style of the reference source. Its core architecture uses a dual-stream DiT (Diffusion Transformer) to process audio and visual information separately, ensuring synchronization and style consistency through a novel audio-visual fusion module. The model captures style features from the reference video using a context reference learning module, eliminating the need for an extra style extractor. The project is now available for trial on the ModelScope community and HuggingFace. (Source: Machine Heart)
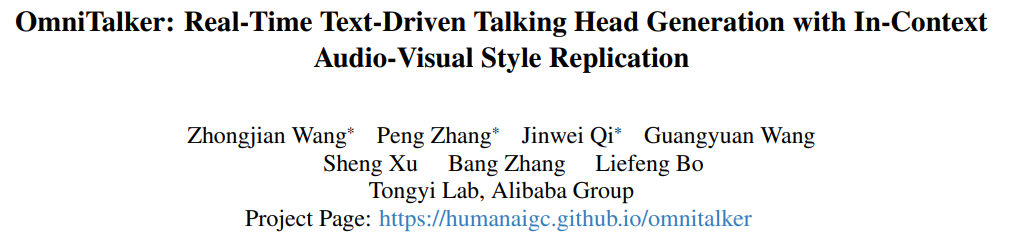
Kuaishou Releases Kling AI Video Model Version 2.0: Kuaishou’s Kling AI video generation model has released version 2.0, reportedly showing significant improvements in camera movement range, adherence to physical laws, character performance, motion stability, and semantic understanding. User reviews indicate the new version excels in handling complex interactions (like a T-Rex breaking trees), fine motor actions (like removing glasses), multi-person scenes, and simulating realistic lighting and shadows. The generated videos exhibit substantially enhanced realism and cinematic quality, considered to surpass the previous 1.6 version and reach industry-leading levels. While there’s still room for improvement in high-speed group motion and extreme physics simulation (like shooting a basketball), its overall performance is seen as challenging professional production standards. Users can experience the new version via the official website klingai.com. (Source: 公众号, op7418)
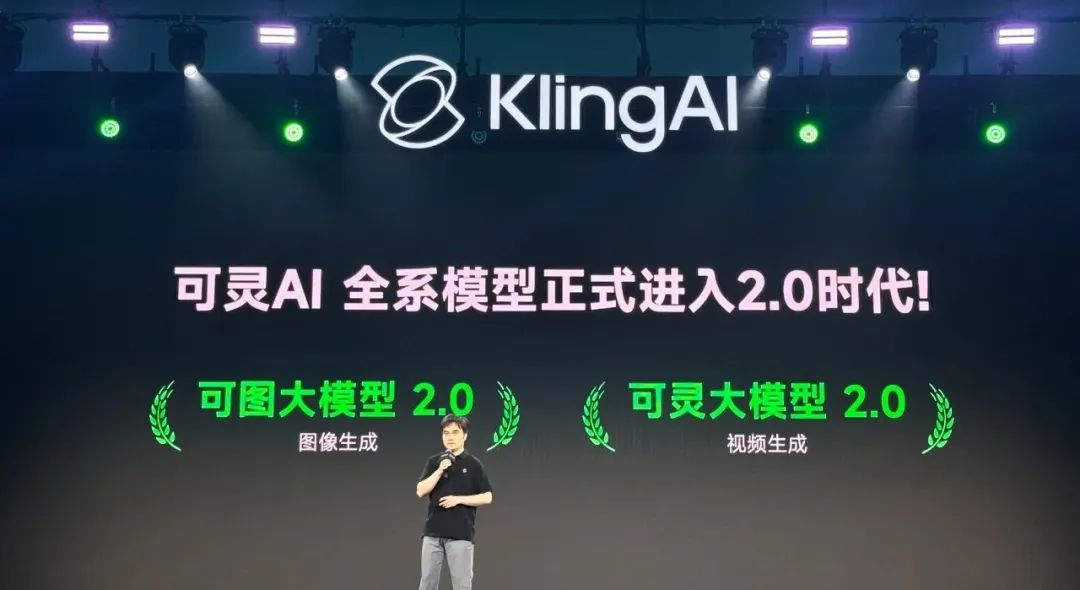
Huawei Releases Pangu Ultra 135B Dense Model, Trained Purely on Ascend with Superior Performance: Huawei unveiled a new member of its Pangu large model series – Pangu Ultra. This is a 135B parameter dense model trained entirely on Huawei’s Ascend AI computing cluster (8192 NPUs), without using Nvidia GPUs. According to reports, Pangu Ultra performs exceptionally well on tasks like mathematical reasoning (AIME 2024, MATH-500) and programming (LiveCodeBench), with performance comparable to larger MoE models like DeepSeek-R1. Technically, the model employs innovative deep-scaling Sandwich-Norm layer normalization and TinyInit parameter initialization strategies, effectively addressing instability issues during the training of ultra-deep networks (94 layers) and achieving smooth training without loss spikes. Through system-level optimization, the training achieved a compute utilization (MFU) of over 52%. (Source: Quantumbit)
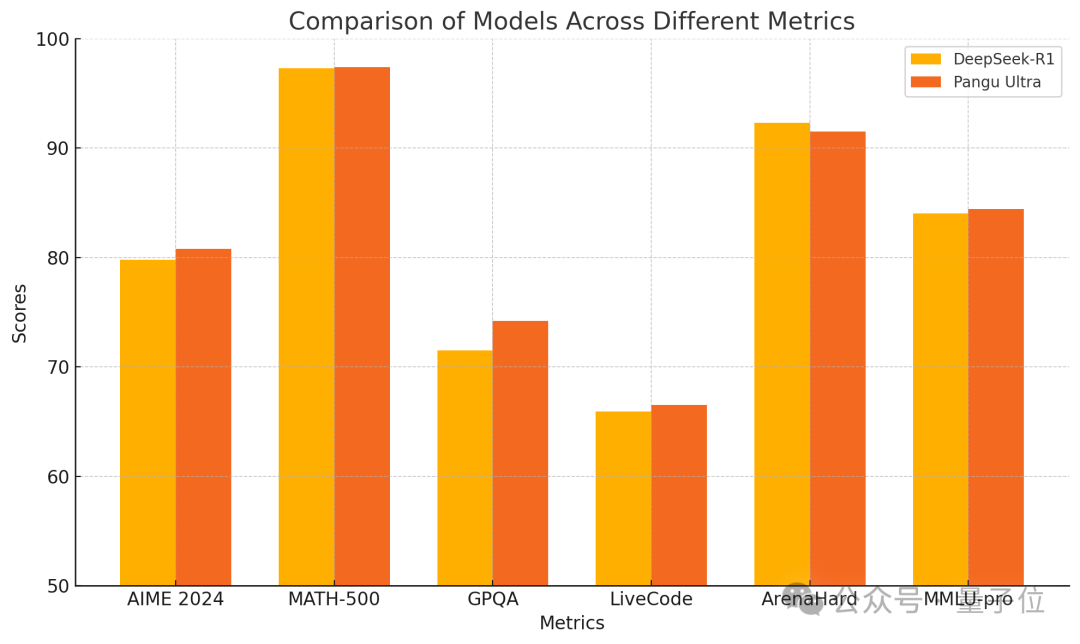
Canopy Labs Open-Sources Emotional Speech Synthesis Model Orpheus: Canopy Labs has released and open-sourced a text-to-speech (TTS) model series named Orpheus. Based on the Llama architecture, the initial release is a 3 billion parameter version, with smaller versions (1B, 0.5B, 0.15B) to follow. Orpheus is characterized by its ability to generate speech with highly human-like emotion, intonation, and rhythm, even inferring and generating non-linguistic sounds like laughter and sighs from text, achieving “empathetic” expression. The model supports zero-shot voice cloning and emotion/intonation control via tags. It uses streaming inference with low latency (100-200ms) and runs faster than real-time on an A100 40GB GPU. Developers claim its performance surpasses existing open-source and some closed-source SOTA models, aiming to break the monopoly of closed-source TTS models. The model and code are available on GitHub and Hugging Face. (Source: Xin Zhi Yuan)
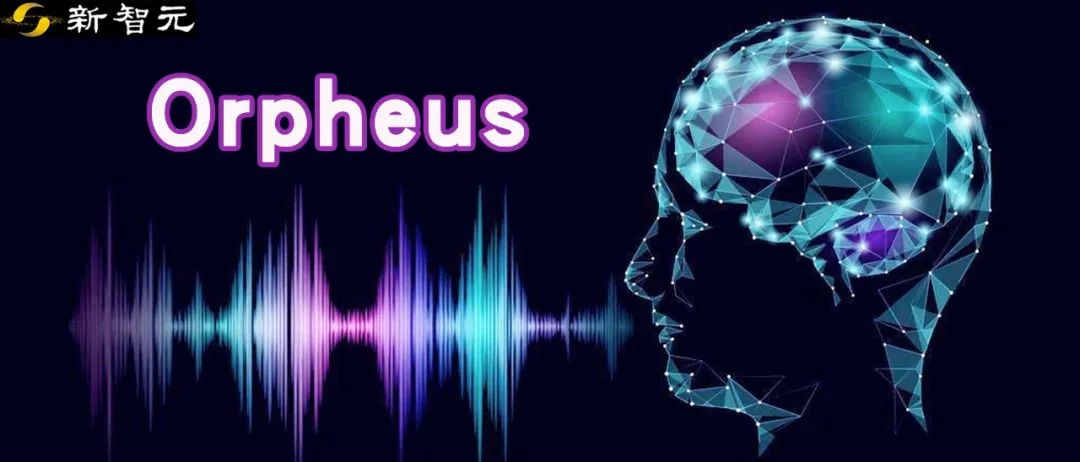
Zhejiang University and ByteDance Jointly Release MegaTTS3 Speech Synthesis Model: Professor Zhou Zhao’s team at Zhejiang University, in collaboration with ByteDance, has released and open-sourced the third-generation speech synthesis model, MegaTTS3. With a lightweight parameter size of only 0.45B, the model achieves high-quality bilingual (Chinese-English) speech synthesis and excels in zero-shot voice cloning, generating natural, controllable, and personalized speech. MegaTTS3 focuses on breakthroughs in sparse speech-text alignment, generation controllability, and balancing efficiency with quality. Technical highlights include the “Multi-Condition Classifier-Free Guidance” (Multi-Condition CFG) technique for multi-dimensional control (like accent strength) and the “Piecewise Rectified Flow Acceleration” (PeRFlow) technique that triples sampling speed. The model demonstrates leading naturalness (CMOS) and speaker similarity (SIM-O) on benchmarks like LibriSpeech. (Source: PaperWeekly)

InternVL 3 Multimodal Large Model Series Open-Sourced: OpenGVLab has released the InternVL 3 multimodal large model series, with parameter sizes ranging from 1B to 78B, now available on Hugging Face. The 78B parameter version reportedly scored 72.2 on the MMMU benchmark, setting a new SOTA record for open-source multimodal models. Technical highlights of InternVL 3 include: native multimodal pre-training to learn language and vision simultaneously; introduction of Variable Vision Position Encoding (V2PE) to support extended context; use of advanced post-training techniques like SFT and MPO; and application of test-time scaling strategies to enhance mathematical reasoning abilities. Both training data and model weights have been released to the community. (Source: huggingface)
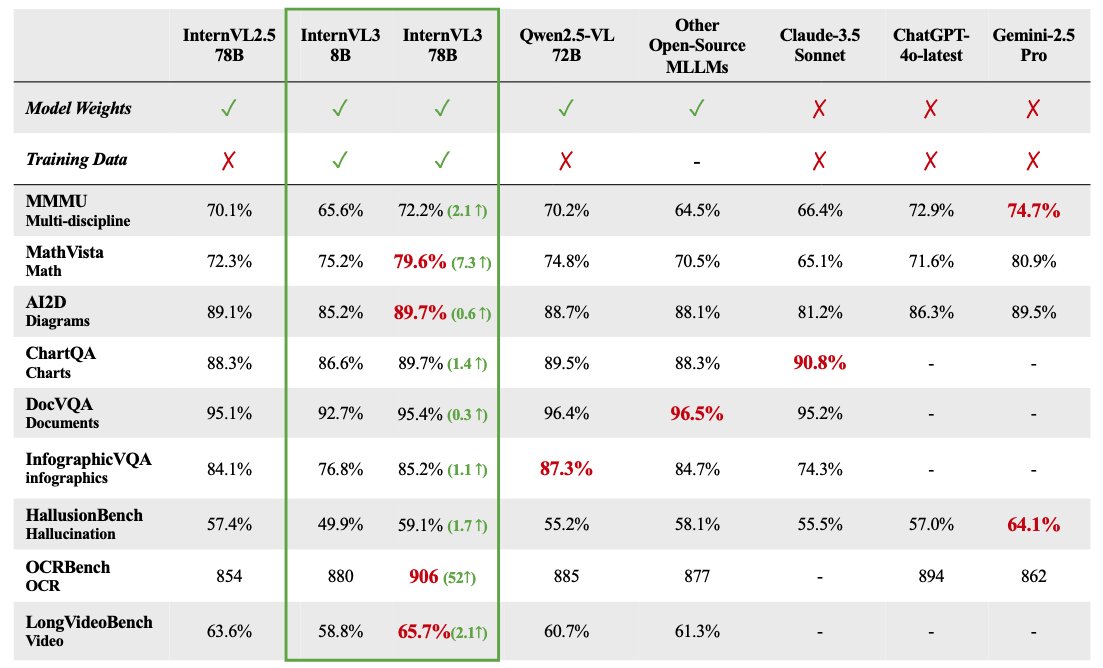
GPT-4.1 Performance Analysis Based on Real-World Testing: Coding Enhanced but Reasoning Lags: OpenAI’s newly released GPT-4.1 series models show a complex performance picture in initial real-world tests and benchmark evaluations. While demonstrating significant improvement over GPT-4o in code generation tasks, such as better handling of physics simulations and game development, and scoring high on SWE-Bench, GPT-4.1’s performance on broader reasoning, math, and knowledge Q&A benchmarks (like Livebench, GPQA Diamond) still lags behind Google’s Gemini 2.5 Pro and Anthropic’s Claude 3.7 Sonnet. Analysts suggest GPT-4.1 might be an incremental update to GPT-4o or distilled from GPT-4.5. Its release strategy might aim to offer more cost-effective, specifically optimized model options via API, rather than a flagship model intended to comprehensively outperform competitors. (Source: Xin Zhi Yuan)
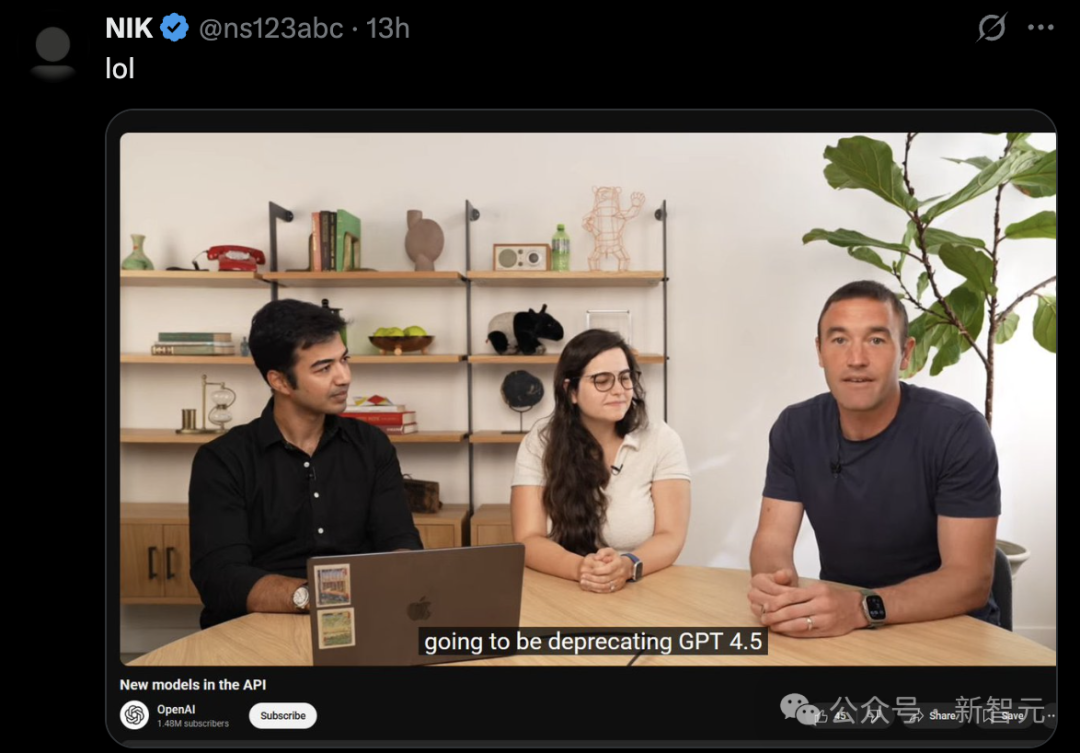
LMArena Search Leaderboard: Gemini 2.5 Pro and Perplexity Sonar Tied for First: In the LMArena leaderboard evaluating large models with search/web-browsing capabilities, Google’s Gemini-2.5-Pro (integrated with Google Search) and Perplexity’s Sonar-Reasoning-Pro are tied for the top spot. This result was confirmed and shared by Google DeepMind CEO Demis Hassabis and Google Developer Relations lead Logan Kilpatrick. Perplexity CEO Aravind Srinivas also commented that internal A/B tests show their Sonar model has better user retention than GPT-4o and performs comparably to Gemini 2.5 Pro and the newly released GPT-4.1. The evaluation organizer, lmarena.ai, has open-sourced 7,000 user preference votes. (Source: lmarena_ai 1, lmarena_ai 2, AravSrinivas, demishassabis)
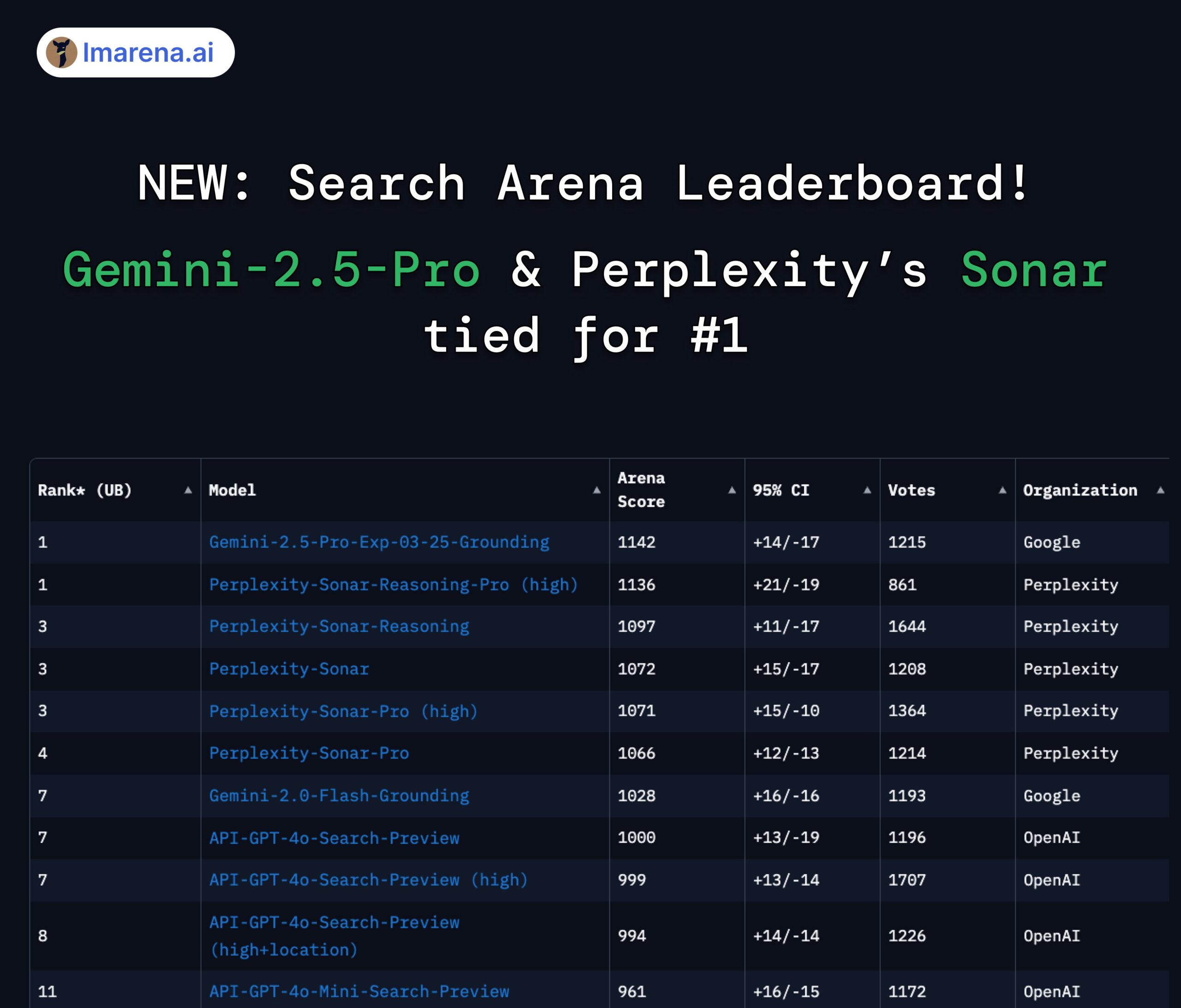
Meta to Resume Using European Users’ Public Content for AI Training: Meta announced it will restart using public content from European users to train its artificial intelligence models. Previously, Meta had paused this practice due to pressure and regulatory requirements from European data protection authorities, particularly the Irish Data Protection Commission. The decision to resume training likely reflects Meta’s ongoing efforts and strategic adjustments to balance user privacy, comply with regulations (like GDPR), and acquire sufficient data to maintain the competitiveness of its AI models. This move may reignite discussions about user data rights and AI training transparency. (Source: Reddit r/artificial)
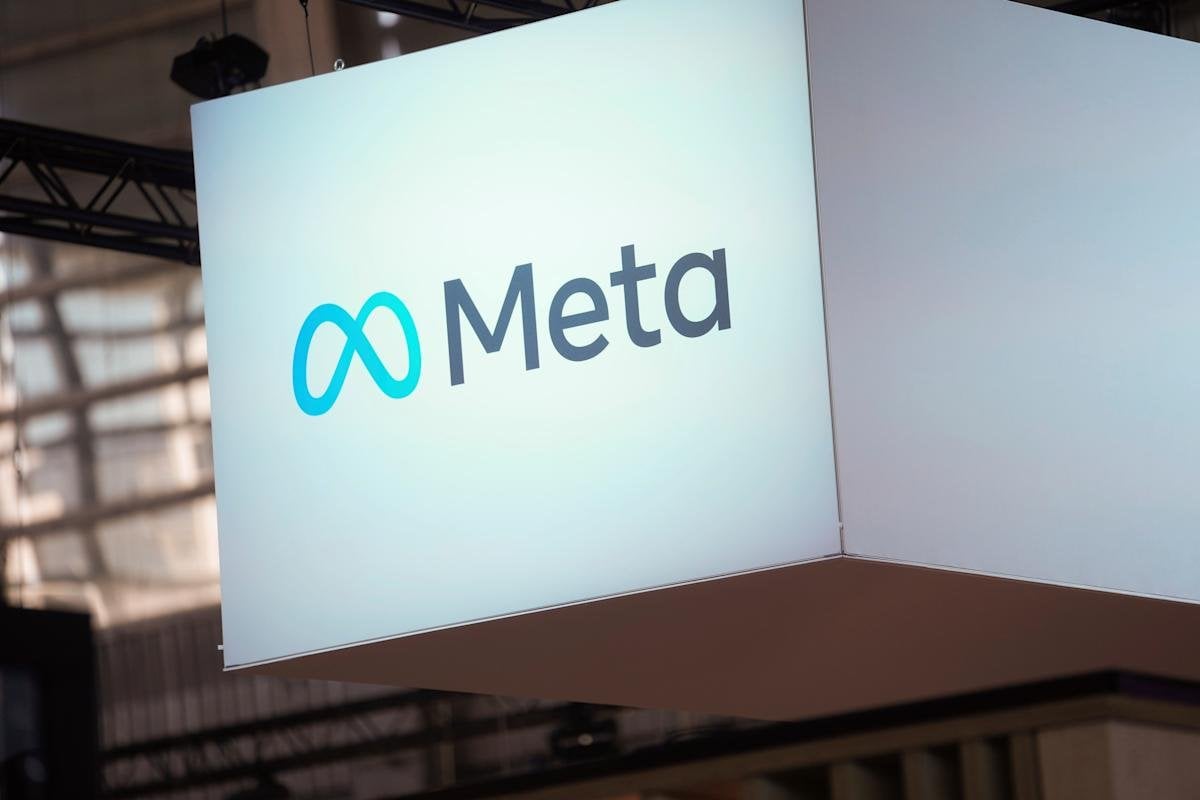
Claude Mobile App May Add Voice Interaction Mode: Based on clues discovered by X user @testingcatalog, Anthropic might be planning to add voice interaction functionality to its Claude mobile application. Screenshots show a microphone icon appearing in the app interface, suggesting users may soon be able to converse with Claude via voice, similar to the voice modes already offered by ChatGPT and Google Gemini apps. This would diversify interaction methods on Claude mobile, enhance user convenience, and bring feature parity with other major AI assistants. (Source: Reddit r/ClaudeAI)
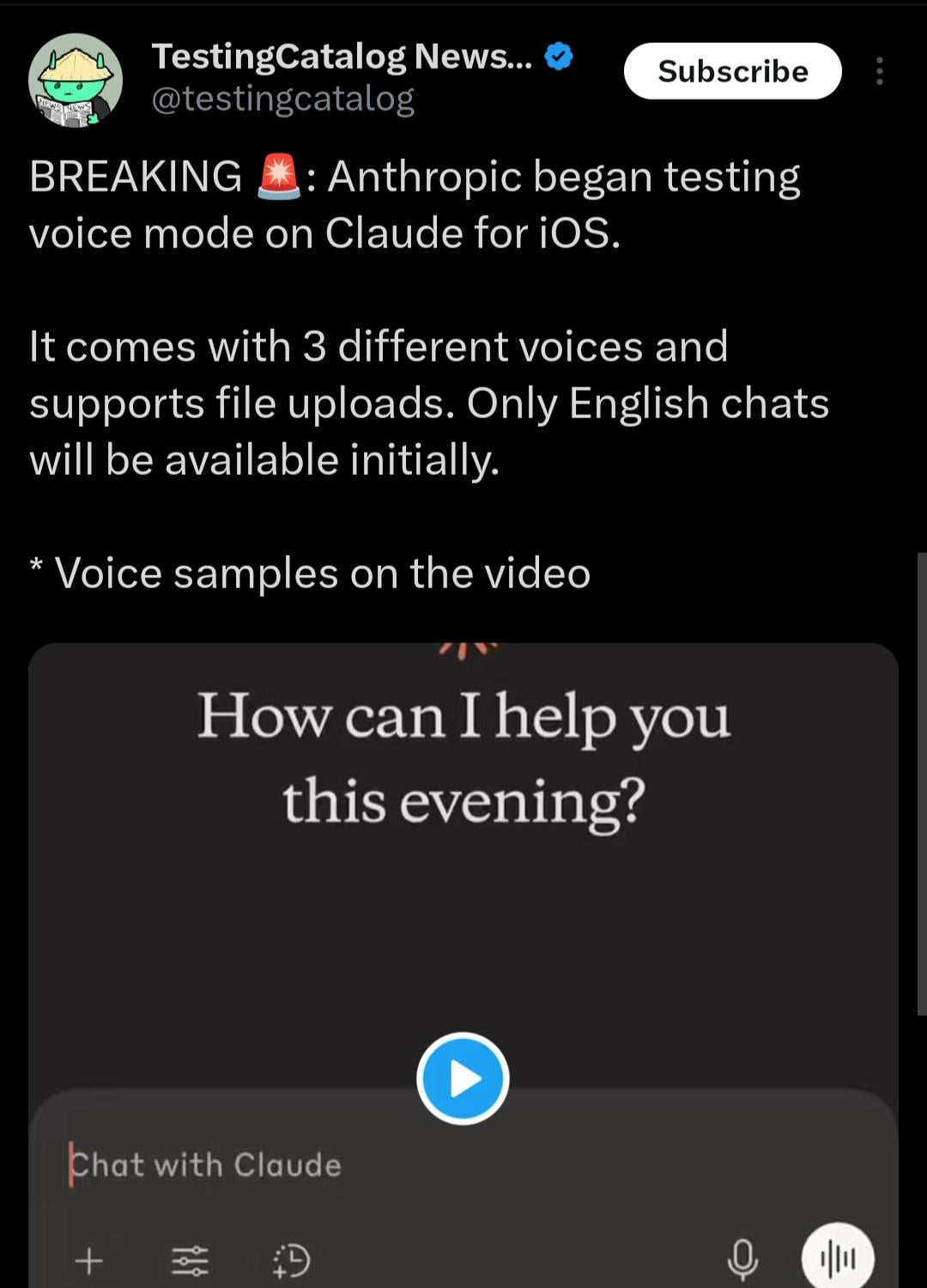
Zhipu’s Z1 Series Models Attract Attention for Speed, Dubbed “Instant Models”: Zhipu AI’s newly released Z1 series models, particularly the GLM-Z1-AirX version, have gained attention for their extremely fast inference speed. Some analysts have dubbed them “instant models,” noting their ability to deliver the first response and generate over 50 Chinese characters within 0.3 seconds, approaching human neural reflex time. This low latency and high throughput could potentially transform human-computer interaction from a “question-wait-answer” pattern to near real-time synchronous conversation, especially suitable for scenarios demanding high responsiveness like education, customer service, content creation, and Agent calls. The API version of Z1-AirX reportedly reaches speeds of 200 tokens/s. (Source: 公众号)
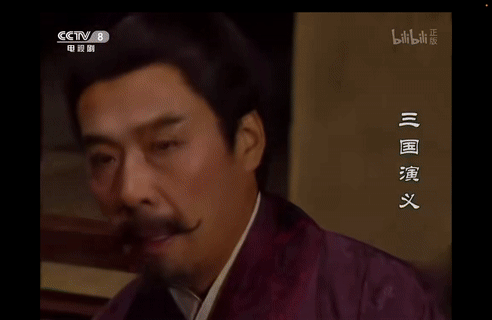
AI-Native Games: Evolution and Challenges from Efficiency Tools to Gameplay Innovation: The gaming industry is evolving from using AI to improve development and operational efficiency (e.g., art generation, code assistance, automated testing) towards exploring truly “AI-native games.” The core of AI-native games lies in deeply integrating AI into gameplay, creating dynamic content and personalized experiences driven by player interaction, rather than pre-scripted scenarios. Examples of such explorations include “Whispers from the Star,” invested in by miHoYo founder Cai Haoyu, and the AI player mode in Giant Network’s “Super Sus.” However, realizing AI-native games faces numerous challenges: technically, issues of model capability, stability, and cost need resolution; design-wise, mature paradigms are lacking, requiring a balance between controllability and freedom; user-wise, player demands for fun and interaction depth must be met; additionally, there are content compliance and ethical risks. The industry is still in the early exploration phase, far from mature implementation. (Source: Jiemian News)
🧰 Tools
Highlighting Five Creative AI Applications: 36Kr reviewed five creative and practical AI tools from recent submissions of innovative AI-native applications: 1) AiPPT.com: Quickly generates PPTs from a single sentence or imported files (Word, PDF, Xmind, links), supports offline operation. 2) ShanJi AI PaiPai Mirror: AI glasses with functions like photo/video recording, real-time translation, and formula recognition. 3) Lianxin Digital Imperceptible Interrogation Agent: Based on the psychological large model “Insightful Human,” assists interrogations by analyzing micro-expressions, voice, and physiological signals, generating reports. 4) Huilima Vali Footwear AI: Generates 8 footwear design drafts in 10 seconds from keywords, integrating material libraries and pattern data, connecting to production. 5) Nanfang Shittong Sandbox HR Agent: Handles social security and HR tasks, providing policy interpretation, cost calculation, intelligent processing, and risk warnings. These applications showcase AI’s potential in efficiency tools, smart hardware, and specialized domains (security, design, HR). (Source: 36Kr)
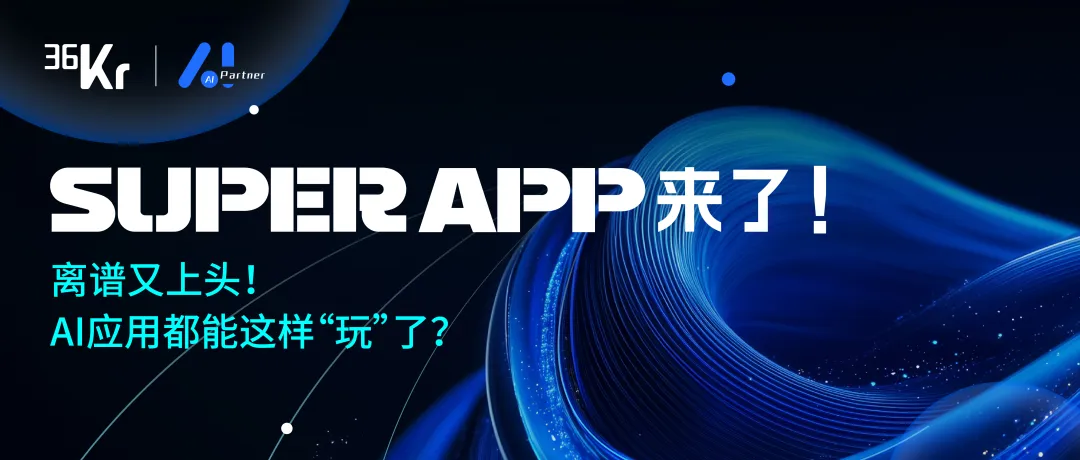
Haisin Intelligence Releases AI No-Code Development Platform “Haisnap”: Beijing state-owned Haisin Intelligence Technology launched an AI no-code/low-code development platform called “Haisnap”. Users can describe their needs in natural language, and the AI automatically generates web applications or small games. A key feature is that the code is visible in real-time during generation and supports secondary editing and modification through conversational interaction. Applications developed by users can be published to the platform’s “Creative Community” for others to browse, use, and remix. The platform is currently free, aiming to lower the barrier for AI application development, promote creation for all, and particularly focus on youth AI education and industry application deployment. (Source: Quantumbit)
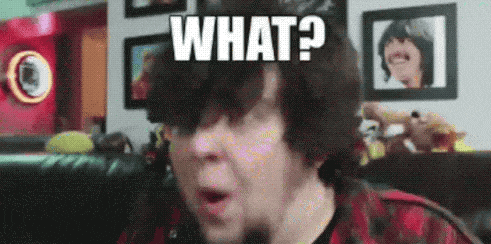
Open-Source Knowledge Base Q&A System ChatWiki Released, Supports GraphRAG and WeChat Integration: ChatWiki is a newly open-sourced knowledge base AI Q&A system that integrates large language models (supporting over 20 models like DeepSeek, OpenAI, Claude) with Retrieval-Augmented Generation (RAG) technology. It notably supports GraphRAG based on knowledge graphs to handle complex queries. System features include: importing various document formats (OFD, Word, PDF, etc.) to build private knowledge bases; supporting semantic chunking to improve RAG accuracy; publishing knowledge bases as public documentation sites; providing API interfaces for seamless integration with WeChat Official Accounts, WeChat Customer Service, etc., to create AI chatbots; built-in visual workflow orchestration tool; support for integrating with third-party business data; enterprise-level permission management; support for Docker and source code local deployment. (Source: 公众号)
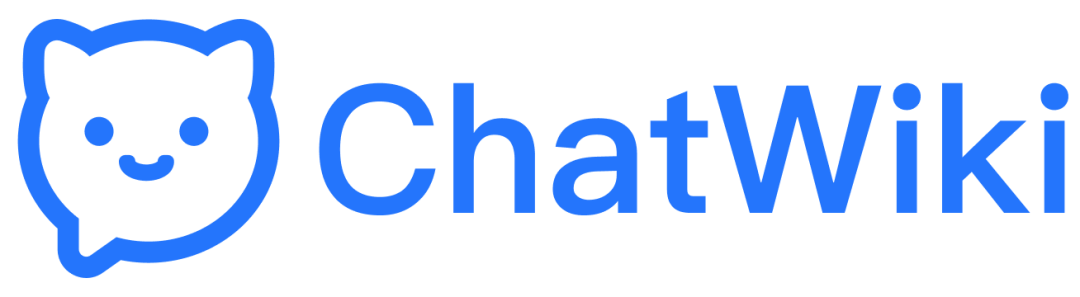
ModelScope Community Launches MCP Square, Building China’s Largest MCP Service Ecosystem: Alibaba’s AI model community, ModelScope, officially launched “MCP Square,” bringing together nearly 1,500 services that implement the Model Context Protocol (MCP). Covering domains like search, maps, payments, and developer tools, it aims to create the largest MCP Chinese community in China. Several MCP services from Alipay and MiniMax debuted exclusively here, such as Alipay’s payment, query, and refund capabilities, and MiniMax’s voice, image, and video generation capabilities, all callable by AI agents via the MCP protocol. Developers can quickly experiment with and integrate these services in the ModelScope MCP Playground using simple JSON configurations and free cloud resources, significantly lowering the barrier for AI applications to access external tools and data. ModelScope also introduced MCP Bench for evaluating the quality and performance of various MCP services. (Source: Xin Zhi Yuan)
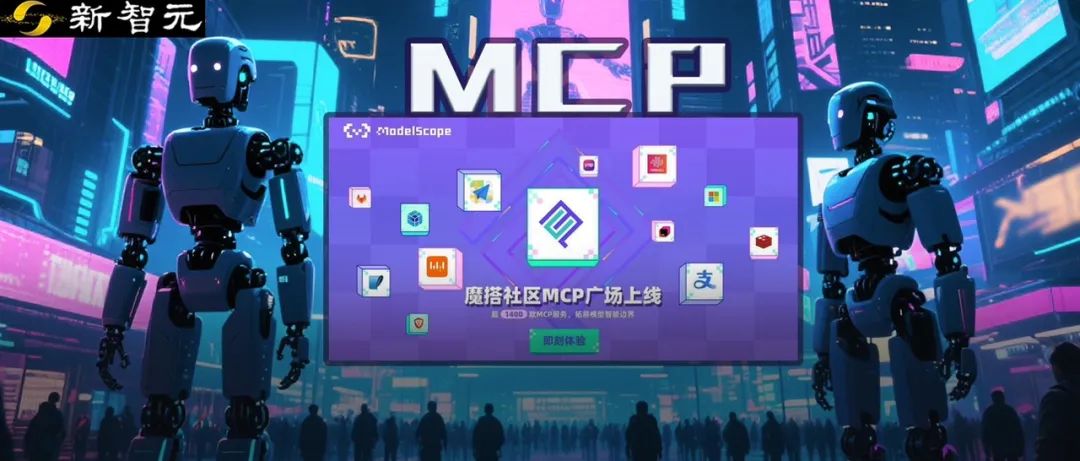
Discussion on Using Open WebUI WebSearch Functionality: Reddit community users discussed how to use the Web Search feature within Open WebUI. Questions focused on how to precisely control the search keywords used by the engine and how to restrict the Web Search functionality to specific models to prevent private model data from being accidentally sent to the web. This reflects users’ practical needs for control precision and privacy security when using AI tools with integrated search capabilities. (Source: Reddit r/OpenWebUI 1, Reddit r/OpenWebUI 2)
User Seeking Understanding of Model Context Protocol (MCP): A user posted on Reddit seeking an explanation of the Model Context Protocol (MCP), indicating a growing need within the developer and user community to understand this emerging technology and its working principles as MCP standards gain traction and application (e.g., ModelScope MCP Square). (Source: Reddit r/OpenWebUI)
📚 Learning
ICLR 2025 Test of Time Award Goes to Adam Optimizer and Attention Mechanism: The International Conference on Learning Representations (ICLR) awarded its 2025 Test of Time Award to two landmark papers published ten years ago (in 2015). One is “Adam: A Method for Stochastic Optimization” by Diederik P. Kingma and Jimmy Ba, which introduced the Adam optimizer that has become a standard algorithm for training deep learning models. The other is “Neural Machine Translation by Jointly Learning to Align and Translate” by Dzmitry Bahdanau, Kyunghyun Cho, and Yoshua Bengio, which first introduced the attention mechanism, laying the foundation for the Transformer architecture and modern large language models. These awards highlight the profound impact of fundamental research on current AI development. (Source: Xin Zhi Yuan)
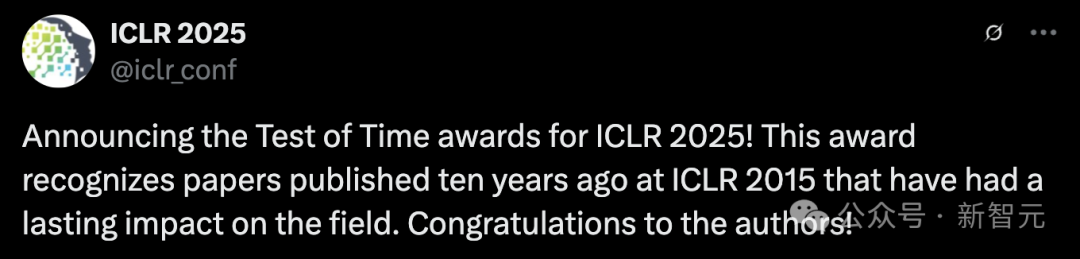
A Brief History of AI Development and Corporate Evolution: The article systematically reviews the history of artificial intelligence from the mid-20th century to the present, highlighting key milestones including the Turing Test, the Dartmouth Workshop, symbolism and expert systems, AI winters, the rise of machine learning (DeepBlue, PageRank), the deep learning revolution (AlexNet, AlphaGo), and the current era of large models (GPT series, generative AI commercialization, open vs. closed source debates). Simultaneously, it divides the evolution of AI companies into four eras: Pioneering Era (2000-2010, exploring tool-based applications), Gold Rush Era (2011-2016, platform enablement and data-driven explosion), Bubble Era (2017-2020, scenario competition and commercialization bottlenecks), and Reconstruction Era (2021-present, large models driving a new landscape). The article emphasizes the synergistic roles of compute power, data, and algorithms, as well as the impact of new forces like DeepSeek on the landscape. (Source: Chaos University)
OpenAI Releases GPT-4.1 Prompting Guide: Coinciding with the release of the GPT-4.1 series models, OpenAI updated its Prompting guide. The guide emphasizes that GPT-4.1 series models, compared to earlier models like GPT-4, follow instructions more strictly and literally, being more sensitive to clear, specific prompts. If the model’s behavior doesn’t meet expectations, adding concise and clear instructions usually guides it correctly. This differs from past models that tended to guess user intent, meaning developers might need to adjust their existing prompting strategies. The guide provides best practices from basic principles to advanced strategies to help developers better leverage the new models’ characteristics. (Source: dotey, Reddit r/LocalLLaMA)
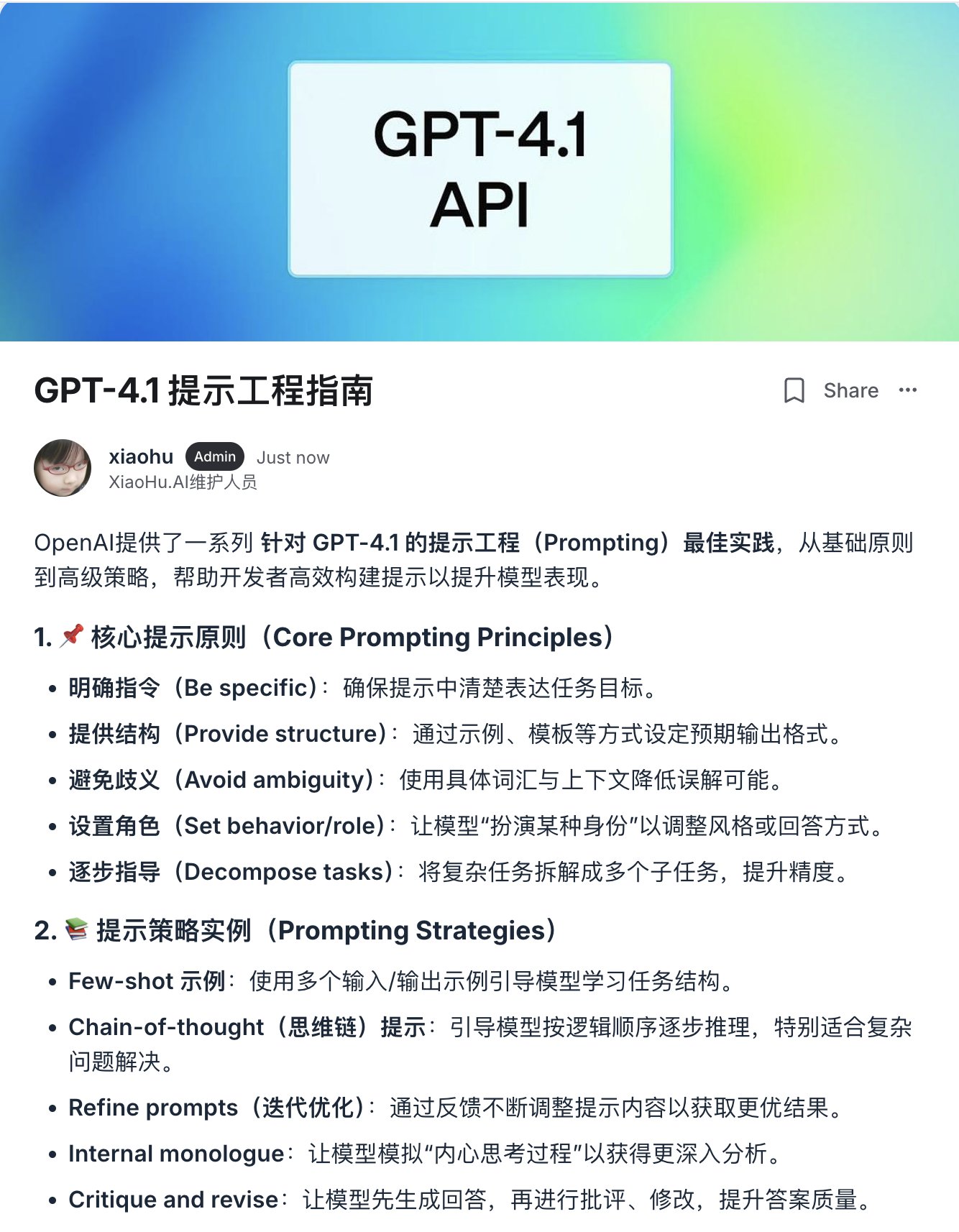
Shanghai Jiao Tong University et al. Release Spatio-Temporal Intelligence Benchmark STI-Bench, Challenging Multimodal Models’ Physical Understanding: Shanghai Jiao Tong University, in collaboration with multiple institutions, released STI-Bench, the first benchmark to evaluate the spatio-temporal intelligence of Multimodal Large Models (MLLMs). Using real-world videos, the benchmark focuses on precise, quantitative spatio-temporal understanding capabilities, covering eight tasks: scale measurement, spatial relations, 3D localization, displacement path, velocity & acceleration, egocentric orientation, trajectory description, and pose estimation. Evaluations of top models like GPT-4o, Gemini 2.5 Pro, Claude 3.7 Sonnet, and Qwen 2.5 VL show that existing models generally perform poorly on these tasks (accuracy < 42%), particularly struggling with quantitative spatial attributes, temporal dynamics, and integrating cross-modal information. The benchmark reveals the limitations of current MLLMs in understanding the physical world and provides direction for future research. (Source: Quantumbit)
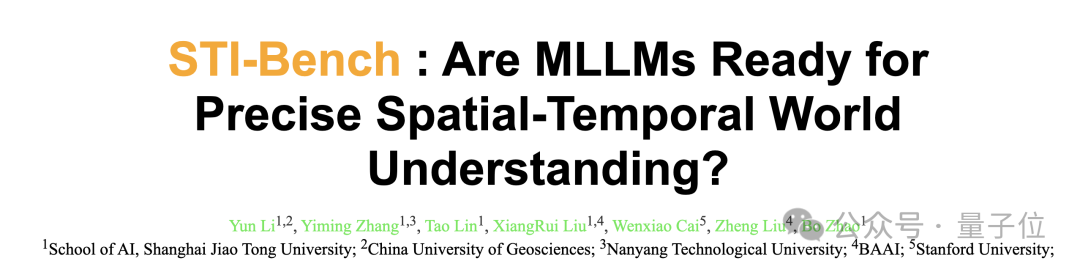
Research Combining Reinforcement Learning and Multi-Objective Optimization Gains Attention: The intersection of Reinforcement Learning (RL) and Multi-Objective Optimization (MOO) is becoming a hot topic in AI decision-making research. This combination aims to enable agents to balance multiple (potentially conflicting) objectives in complex environments, rather than pursuing a single optimum. For example, HKUST proposed a dynamic gradient balancing framework for autonomous driving, optimizing safety and energy efficiency simultaneously; MIT’s Pareto policy search algorithm is used for robot control; Alibaba Cloud applies multi-objective alignment techniques in financial trading to balance returns and risks. Related research, such as CMORL (Continual Multi-Objective Reinforcement Learning) and Pareto Set Learning for combinatorial optimization, is exploring how to make RL agents more effective at handling real-world problems that are dynamic or have multiple optimization dimensions. (Source: 公众号)
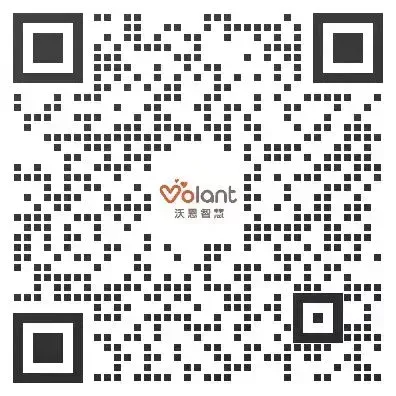
Automated Adversarial Attack and Defense Platform A³D Open-Sourced (TPAMI 2025): The Intelligent Design and Robust Learning (IDRL) research team at the Academy of Military Sciences’ National Innovation Institute of Defense Technology has developed and open-sourced a platform called A³D (Automated Adversarial Attack and Defense). The platform utilizes Automated Machine Learning (AutoML) techniques combined with adversarial game theory principles, aiming to automatically search for robust neural network architectures and efficient adversarial attack strategies. It integrates various Neural Architecture Search (NAS) methods and robustness evaluation metrics (norm-based attacks, semantic attacks, adversarial camouflage, etc.) for automated defense, while also providing an automated adversarial attack module that can search for optimal combined attack schemes through optimization algorithms. The research results were published in the top journal TPAMI, and the code has been released on platforms like Hongshan Open Source, providing new tools for evaluating and enhancing the security of DNN models. (Source: 公众号)
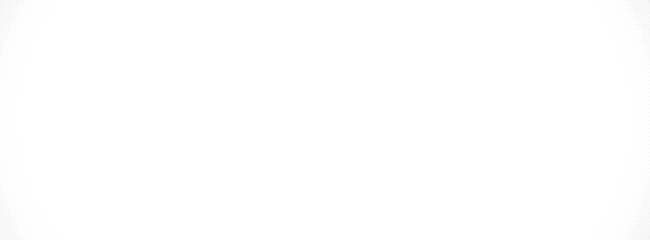
University of Florida Recruiting Fully Funded PhD Students/Interns in NLP/LLM: Assistant Professor Yuanyuan Lei (joining Fall 2025) in the Computer & Information Science & Engineering department at the University of Florida has posted recruitment information for fully funded PhD students starting Fall 2025 or Spring 2026, as well as research interns with flexible timing (remote possible). Research focuses on Natural Language Processing (NLP) and Large Language Models (LLM), specifically including knowledge-enhanced LLMs, fact verification, reasoning & planning, and NLP applications (multimodal, legal, business, scientific, etc.). Students with backgrounds in CS, EE, Statistics, Math, etc., who are interested and motivated in AI research are welcome to apply. The email mentions the potential impact of Florida SB-846 on recruiting students from mainland China and possible ways to navigate it. (Source: PaperWeekly)
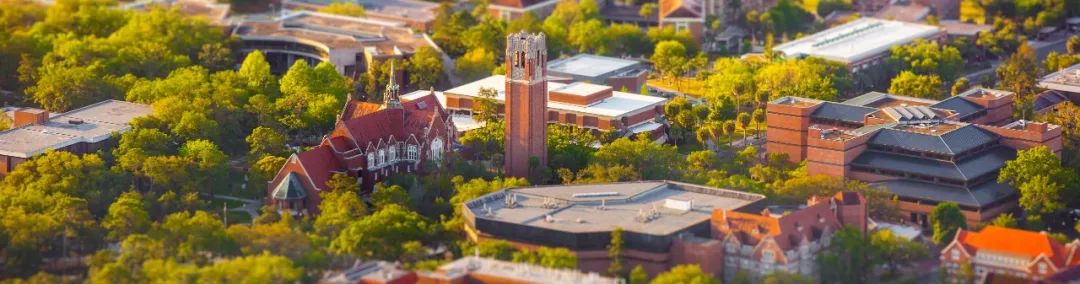
New Diffusion Model Research: Temporally-Correlated Noise Prior: An arXiv paper titled “How I Warped Your Noise: a Temporally-Correlated Noise Prior for Diffusion Models” proposes a novel noise prior for diffusion models. The method aims to improve the generation quality or efficiency of (potentially video) diffusion models by introducing time-correlated noise. Specific technical details require consulting the original paper. (Source: Reddit r/MachineLearning)
New Research on Automated Scientific Discovery: AI Scientist-v2: An arXiv paper titled “The AI Scientist-v2: Workshop-Level Automated Scientific Discovery via Agentic Tree Search” introduces the AI Scientist-v2 system. This system utilizes an Agentic Tree Search method aiming to achieve “Workshop-Level” automated scientific discovery. This indicates researchers are exploring the use of AI agents for more advanced and autonomous scientific research and exploration. (Source: Reddit r/MachineLearning)
Dropout Regularization Implementation Explained: A Substack article provides a detailed explanation of how the Dropout regularization technique is implemented. Dropout is a widely used regularization technique in deep learning that prevents model overfitting by randomly “dropping out” a fraction of neurons during training. The article is likely aimed at learners who want to deeply understand how Dropout works or implement it themselves. (Source: Reddit r/deeplearning)
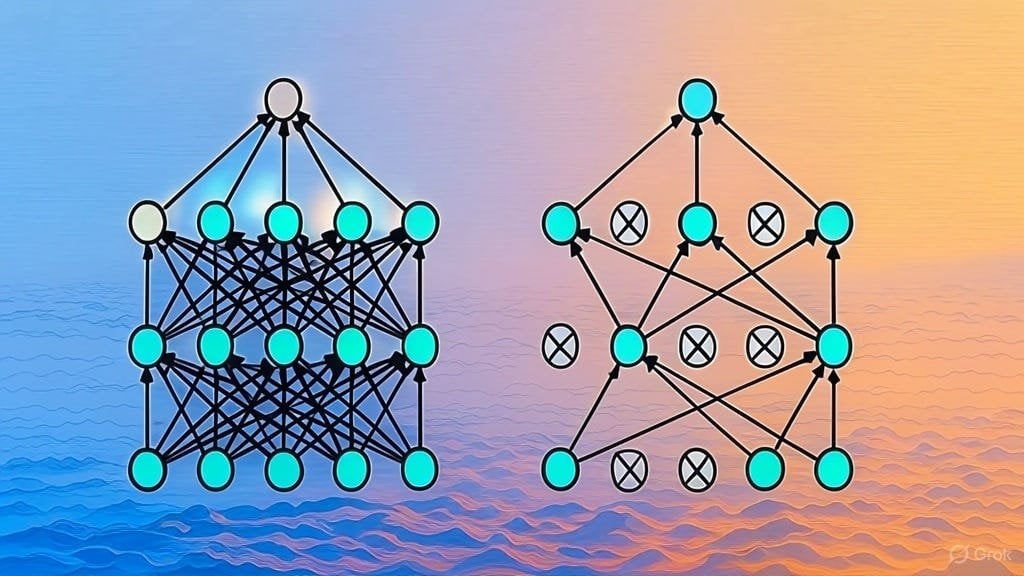
Call for List of LLM Architecture Papers: A Reddit user initiated a discussion to share and collect arXiv papers on Large Language Model (LLM) architectures. Architectures already listed include BERT, Transformer, Mamba, RetNet, RWKV, Hyena, Jamba, DeepSeek series, etc. This list reflects the diversity and rapid development of current LLM architecture research and is a valuable reference for researchers wanting a systematic overview of the field. (Source: Reddit r/MachineLearning)
💼 Business
AI Nutrition Platform Fay Secures $50 Million Funding, Reaches $50 Million ARR: Silicon Valley AI nutrition platform Fay recently completed a $50 million Series B funding round led by Goldman Sachs, bringing total funding to $75 million at a $500 million valuation. Fay connects registered dietitians with patients, using AI to enhance service efficiency (claiming reduction from 6.5 hours/patient to 2 hours) by automating tasks like clinical note generation (including ICD codes), personalized nutrition plan creation, insurance claims processing, and back-office management. The platform accurately capitalized on the surge in demand for nutritional counseling driven by GLP-1 weight-loss drugs and successfully integrated payment by partnering with insurance companies (nutritional intervention can lower long-term healthcare costs for chronic diseases). With fewer than 3,000 dietitians on the platform, Fay achieved $50 million in annual recurring revenue (ARR), demonstrating a successful business model of empowering professionals in a vertical healthcare sector with AI and connecting with payers. (Source: Uya Intelligence Talk)
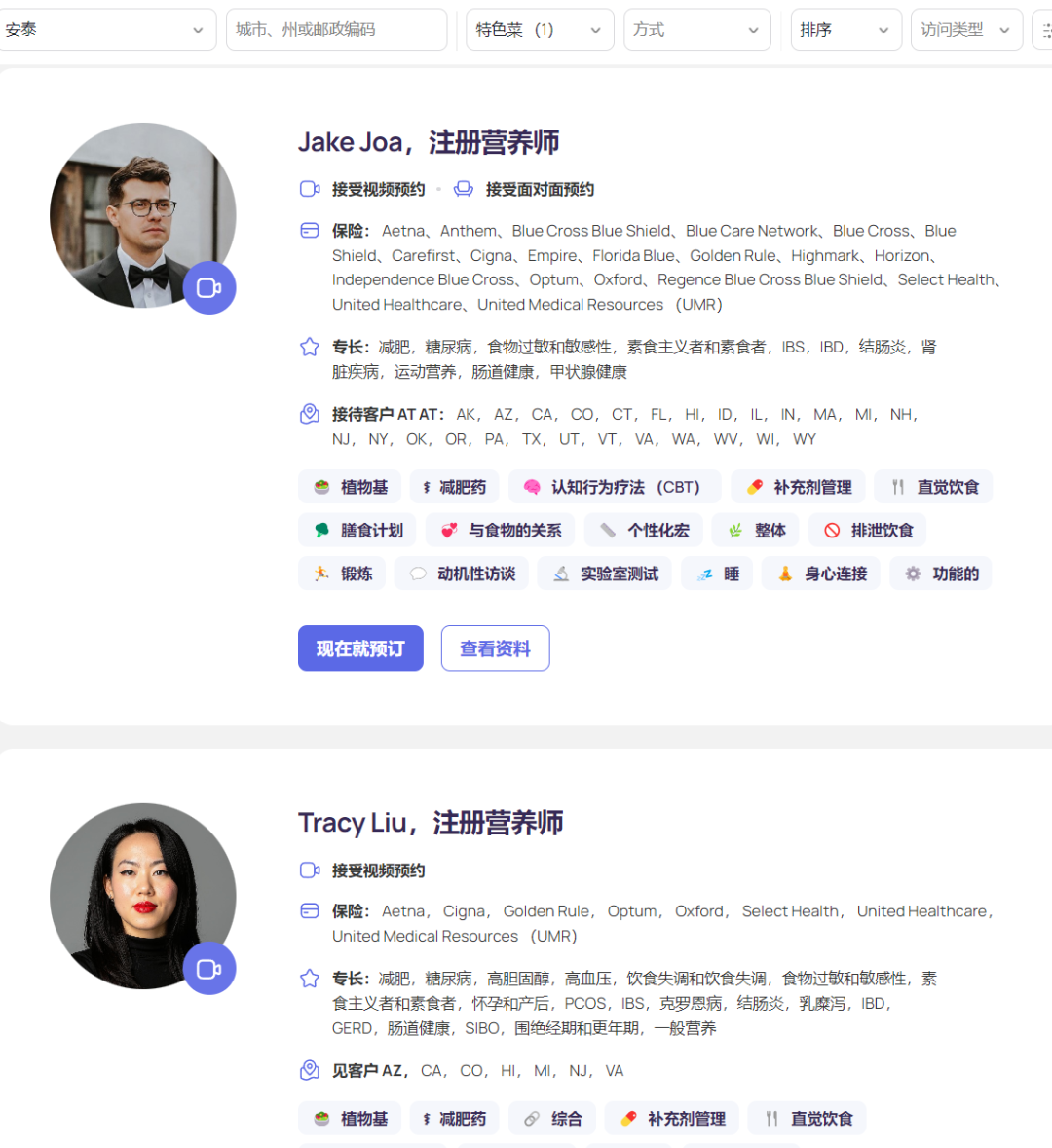
Chengdu Hengtu Technology: Empowering Digital Creativity with AI, Profitable Overseas Expansion: Chengdu-based company Hengtu Technology has accumulated approximately 700 million users globally for its core product Fotor (an image and video editing platform), with over 10 million monthly active users. It has shown particularly strong performance in overseas markets, being one of the earlier Chinese AI application companies to expand internationally and achieve large-scale profitability. The company has 16 years of expertise in image processing technology and rapidly integrated AIGC functions (text-to-image, text-to-video, etc.) into Fotor and its new platform Clipfly in 2022. Fotor lowers the barrier for digital visual content creation using AI, serving various industries like e-commerce, social media, advertising, tourism, and education. Hengtu Technology utilizes AI for “cultural translation,” helping Chinese culture go global and exploring new paths for the digital creative industry. (Source: 36Kr Sichuan)
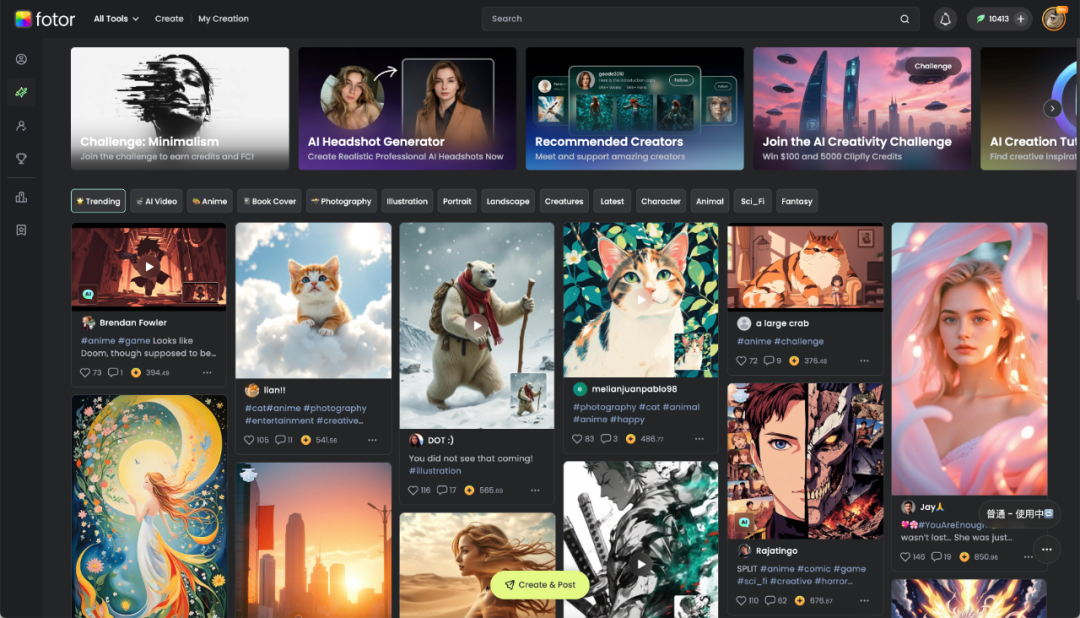
Enterprise AI Implementation Practices: Focus on Value, Less on Fine-tuning, Promote Collaboration: As enterprises advance in deploying large models, the focus has shifted from early exploration to a more pragmatic, value-driven approach. Successful AI applications often target scenarios that are repetitive, require creativity, and have establishable paradigms, such as knowledge Q&A, intelligent customer service, material generation, and data analysis. Companies generally recognize that blindly pursuing model fine-tuning often yields low ROI; prioritizing knowledge governance and building agent platforms (initially RAG-based) is preferred. AI implementation requires deep involvement from business departments and top-level support, with a dual-track strategy of “quick wins pilots + AI foundation preparation” proving more effective. Regarding organizational talent, companies tend to form small, specialized AI teams to empower business units and address talent shortages by bringing in external top talent, nurturing internal young potential (interns paired with senior business staff), and collaborating with external experts. (Source: AI Frontline)
STAR Market Artificial Intelligence Index Gains Attention, Potentially Becoming the Next Investment Hotspot: A report analysis indicates that despite recent market volatility, China’s artificial intelligence industry has formed a complete loop of “compute power – model – application” and demonstrates strong resilience. Highlights include the national “East Data West Compute” project, low-cost models like DeepSeek, and breakthroughs in applications like humanoid robots. AI is considered a major engine for global economic growth over the next decade, with related assets showing significant long-term return potential. Against this backdrop, the SSE STAR Market Artificial Intelligence Index (focusing on compute chips and AI applications) is attracting investor attention due to its high growth expectations and increasing domestic self-sufficiency content. Institutions like E Fund have launched ETFs and feeder funds (e.g., 588730, 023564/023565) tracking this index, providing tools for investors to position themselves in the domestic AI industry chain. (Source: Chuangyebanquancha)
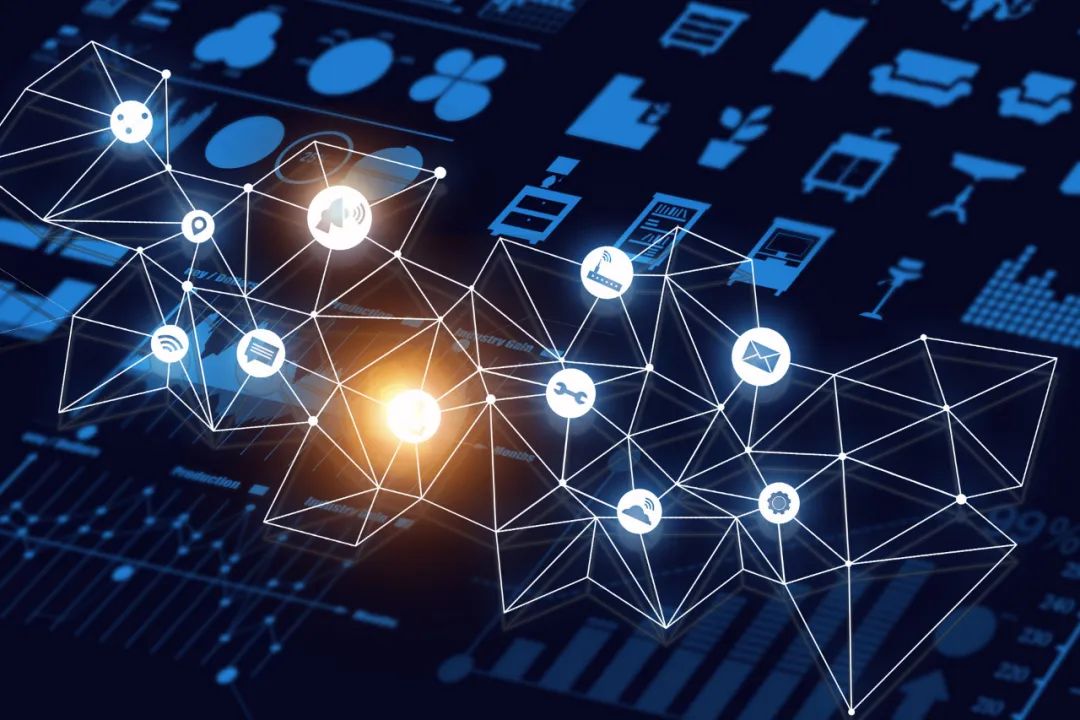
Apple’s AI Strategy Shifts Towards Openness: Allowing Third-Party Models for Siri Development: To accelerate the development of its “personalized Siri” feature and catch up with competitors, Apple has reportedly adjusted its long-standing internal closed development strategy. Under the new Senior Vice President of Software Engineering, Craig Federighi, Siri engineers are now allowed, for the first time, to use third-party large language models to develop Siri features, breaking the previous restriction of using only Apple’s proprietary models. This shift is considered a key move by Apple to address its relative lag in AI technology reserves and to avoid further user dissatisfaction (or even lawsuits) potentially caused by delays in the “personalized Siri” feature. This move could create opportunities for external model providers like OpenAI or Alibaba (in the Chinese market) to collaborate with Apple. (Source: San Yi Sheng Huo)
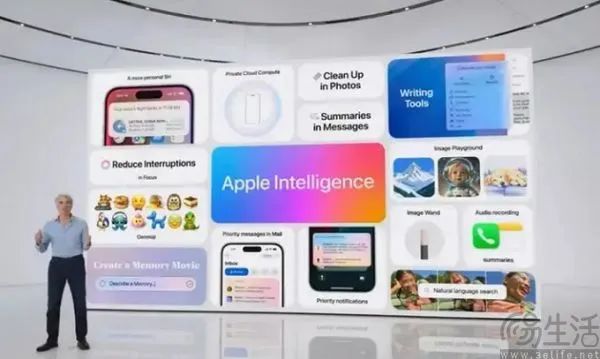
🌟 Community
Intense Competition Among DeepSeek, Doubao, Yuanbao Apps; Product Experience Becomes Key: The domestic AI assistant application market is fiercely competitive. DeepSeek’s user base surged after its model gained explosive popularity, initially boosting Tencent’s Yuanbao (which was among the first to integrate it) to the top spot. However, ByteDance’s Doubao, with more comprehensive product features and deep integration with Douyin (TikTok’s China version), has since overtaken Yuanbao. Analysis suggests that relying solely on integrating powerful models like DeepSeek only provides short-term benefits. In the long run, the application’s feature richness, user experience, multi-device synergy, and platform ecosystem integration are more crucial. As model capabilities converge across providers (e.g., all possessing deep thinking abilities), future competition will focus on product design, operational strategies, and breakthroughs in new application forms like AI Agents. (Source: Zimu Bang)
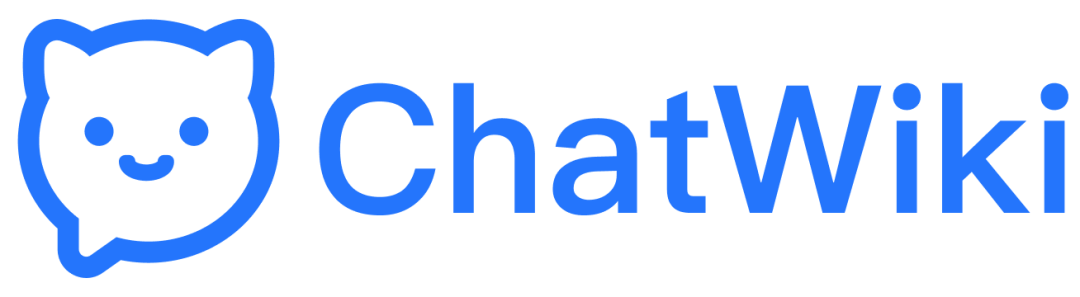
Asian Student Develops Interview Cheating Tool, Sparking Online Debate: Roy Lee, an Asian student at Columbia University, developed an AI tool called Interview Coder and used it with ChatGPT to pass remote technical interviews at several tech companies, including Amazon, Meta, and TikTok. He not only rejected the offers but also recorded himself using the tool and posted the video on YouTube. After being reported by Amazon, he was suspended from school. Undeterred, Roy Lee publicized the entire incident, including emails with the school and companies, gaining massive support from netizens and industry attention. He leveraged this to start his own company. The incident has triggered heated discussions about the validity of technical interviews (especially the LeetCode grinding model), the ethical boundaries of AI tools in recruitment, and individuals challenging large corporate systems. (Source: Zhi Mian AI)
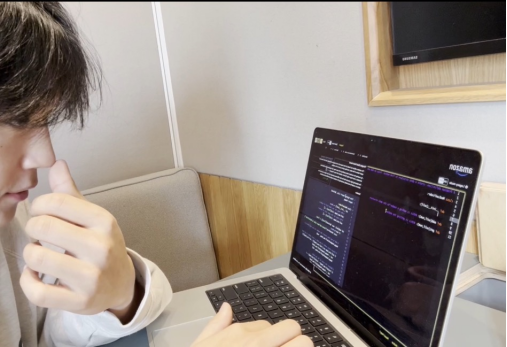
User Tests Zhipu’s New Open-Source GLM Models with Knowledge Base and MCP Integration: A user tested Zhipu AI’s latest GLM series models (via API calls). Results showed that GLM-Z1-AirX (express version) responded extremely quickly (reportedly 200 tokens/s) when connected to a local knowledge base built with FastGPT, and the answer quality improved compared to standard models, generating more detailed and complete answers and comparison tables. GLM-4-Air (base model), when connected to MCP (Model Context Protocol) to perform Agent tasks (like web search, local file writing, Docker control, webpage summarization), could correctly call tools and complete tasks, although its performance was slightly inferior to DeepSeek-V3. The user also praised the Zhipu models’ safety performance (not responding to jailbreak prompts). (Source: 公众号)
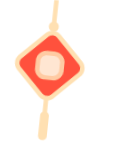
Sharing “Hyper-Rational Problem Solver” Prompt and Comparing Model Performance: A community user shared an advanced prompt designed to make an LLM act as a “hyper-rational, first-principles problem solver.” The prompt details the model’s operating principles (deconstruct problem, engineer solution, delivery protocol, interaction rules), response format, and tone characteristics, emphasizing logic, action, and results while rejecting ambiguity, excuses, and emotional comfort. The user tested this prompt comparing DeepSeek, Claude Sonnet 3.7, and ChatGPT 4o on answering questions, providing guidance, and recommending online resources, finding Claude 3.7 performed better. This demonstrates how carefully crafted prompts can significantly guide and enhance LLM performance on specific tasks. (Source: 公众号)
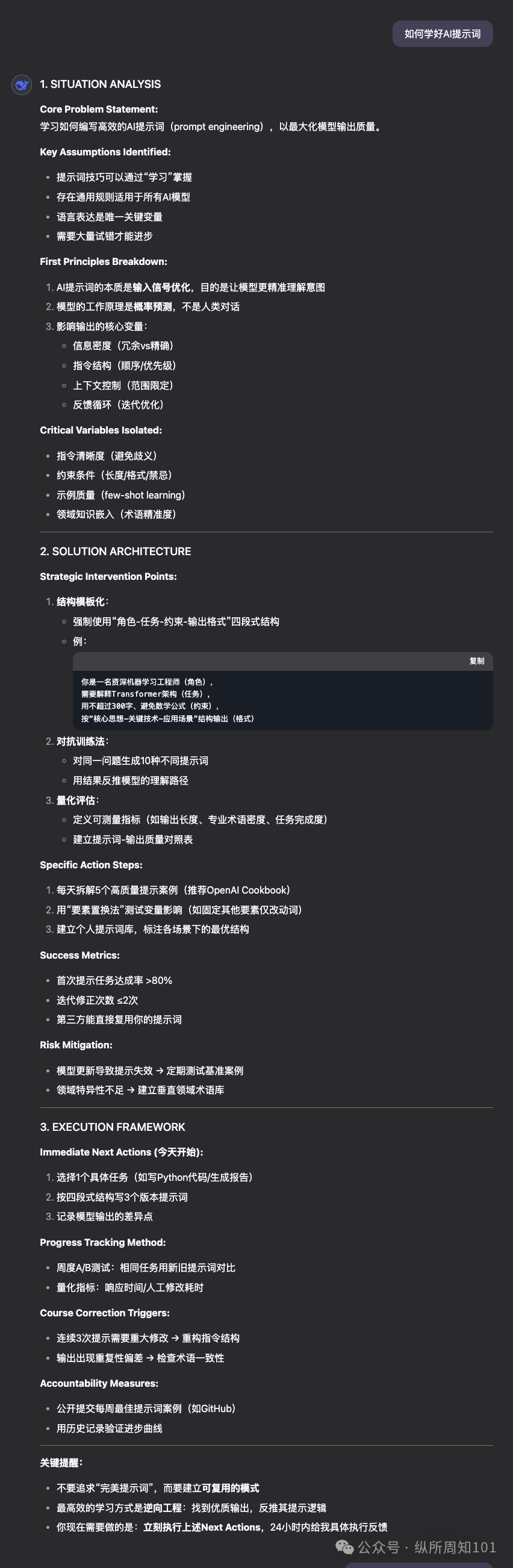
Community Discusses GPT-4.1 Release: Performance, Strategy, and Naming: OpenAI’s release of the GPT-4.1 series models sparked widespread community discussion. On one hand, users found through testing and benchmark comparisons (like Aider, Livebench, GPQA Diamond, KCORES Arena) that while GPT-4.1 shows significant improvement in coding, it still lags behind Google Gemini 2.5 Pro and Claude 3.7 Sonnet in overall reasoning ability. On the other hand, the community discussed and criticized OpenAI’s product strategy (differentiating API from ChatGPT, deprecating GPT-4.5), model iteration speed, and confusing naming convention (releasing 4.1 after 4.5). Some believe OpenAI might be facing an innovation bottleneck, while others see it as a strategy to optimize its API product line and offer different price-performance options. (Source: dotey, op7418, Reddit r/LocalLLaMA 1, Reddit r/ArtificialInteligence, karminski3, Reddit r/LocalLLaMA 2)
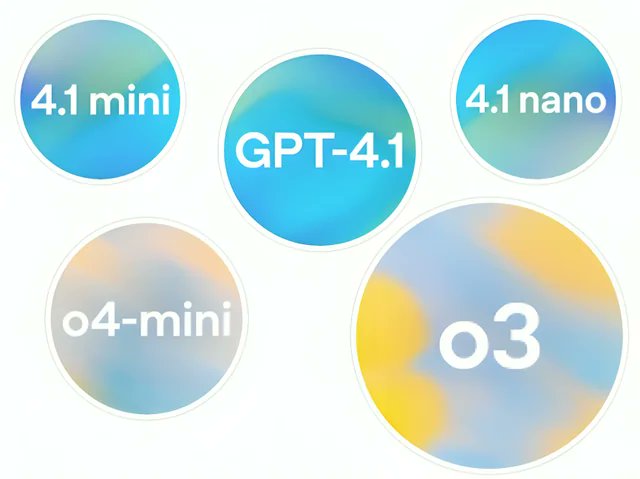
ChatGPT Shows Prowess in Legal Consultation Scenario, User Shares Success Story: A Reddit user shared a success story of using ChatGPT to handle a work-related legal dispute. Facing the risk of dismissal, the user provided documents to ChatGPT, asking it to act as a UK employment law expert. ChatGPT identified procedural errors by the employer. Using a letter drafted by ChatGPT for negotiation, the user ultimately reached a settlement agreement including two months’ salary compensation, avoiding a negative record. Other users in the comments shared similar experiences using AI (ChatGPT or Gemini) to draft legal letters, prepare for hearings, and achieve positive outcomes, noting that AI can save significant costs and time in legal assistance. (Source: Reddit r/ChatGPT)
User Complains About Poor Performance of OpenAI’s Deep Research Feature: A Reddit user posted criticizing OpenAI’s Deep Research feature, identifying three main issues: 1) Search results are inaccurate or irrelevant (relying on Bing API); 2) The exploration method resembles depth-first search rather than broad research; 3) It’s disconnected from the user’s research goals and lacks constraints. The user felt it was more like an extended search capability than true deep research. This reflects the gap between user expectations and the actual experience of current AI Agent research capabilities. (Source: Reddit r/deeplearning)
Showcase and Discussion of AI-Generated Content: Community users actively shared content created using various AI tools (like ChatGPT, Midjourney, Kling AI, Suno AI), including satirical cartoons (Trump and Musk), anthropomorphized universities, an alternate WWII history short film, images of Greek gods, a 90s-style toothpaste ad, and multi-panel comics. These shares not only demonstrate AI’s capabilities in text, image, video, and music generation but also sparked discussions on the creativity, aesthetics (e.g., being called “kitsch”), limitations (e.g., poor character consistency in comics), and ethical issues of AI-generated content. (Source: dotey 1, dotey 2, Reddit r/ChatGPT 1, Reddit r/ChatGPT 2, Reddit r/ChatGPT 3, Reddit r/ChatGPT 4, Reddit r/ChatGPT 5)
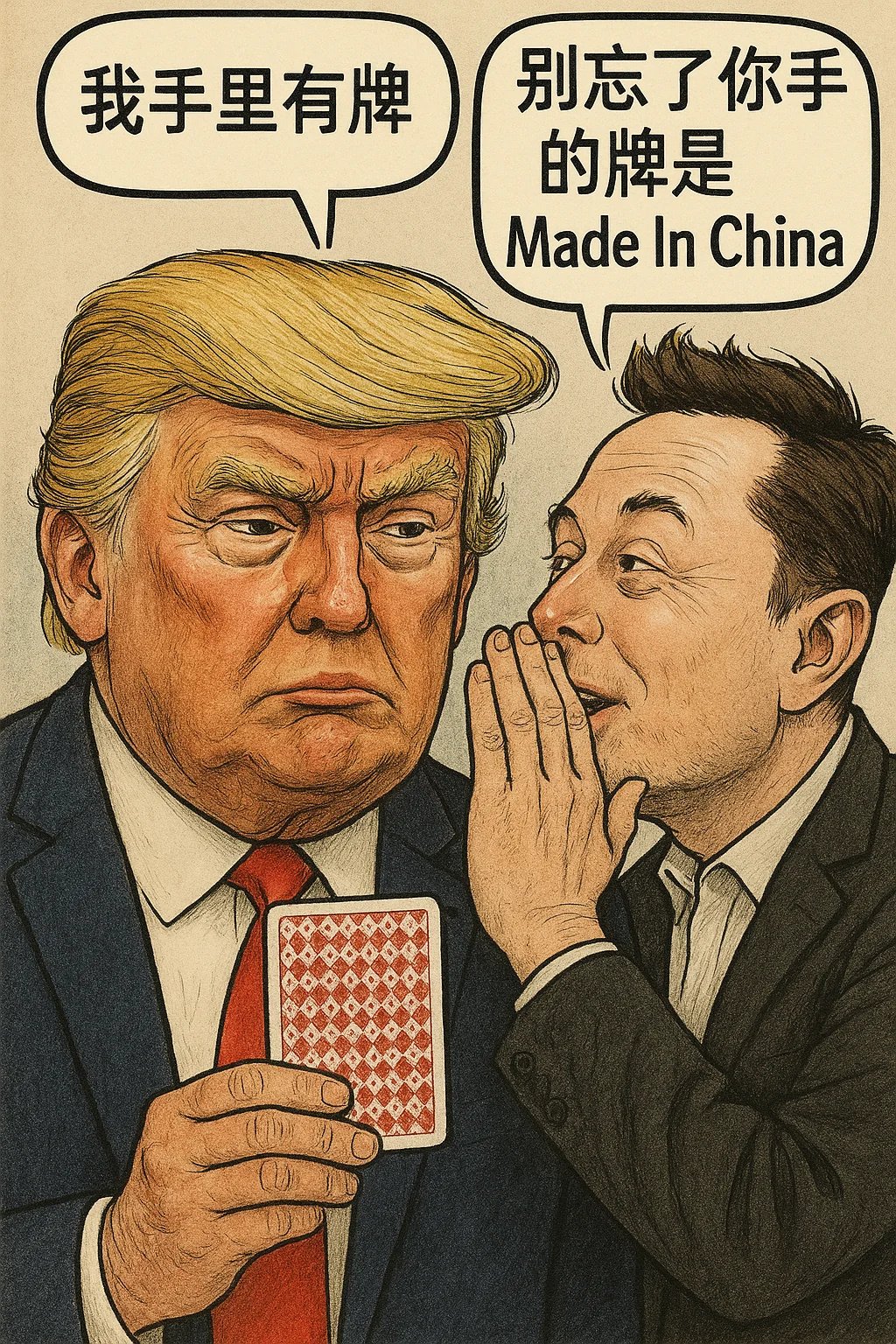
Concerns About AI Training Data Feedback Loop Leading to “Model Collapse”: Community discussion focused on a potential risk: as AI-generated content proliferates online, future AI models trained primarily on this AI-generated data might suffer from “Model Collapse.” This phenomenon refers to performance degradation where model outputs become narrow, repetitive, and lack originality and accuracy, akin to a photocopy degrading with repeated copying. Users worry this could slowly erode information authenticity and human perspective. Potential countermeasures like using synthetic data for training and strengthening data quality control were mentioned, but there’s debate on whether the problem is already occurring and how to effectively prevent it. (Source: Reddit r/ArtificialInteligence)
Viewpoint: In the AI Era, Compute is the New Oil: A Reddit user proposed the view that in AI development, compute power, not data, will become the key bottleneck and strategic resource, analogous to oil during the Industrial Revolution. Reasons cited: more powerful AI models (especially for reasoning and Agent systems) require exponentially growing compute; robotics and other physical interactions will generate massive new data, further increasing compute demand. Possessing more compute power will directly translate to stronger economic output capability. This viewpoint sparked community discussion, generally agreeing that compute is indeed a core element determining the upper limits and pace of AI development. (Source: Reddit r/ArtificialInteligence)
Ethical Discussion on AI Use: Is Using AI to Improve Grades Wrong?: An online university student failed a course due to its structure (only one quiz or assignment per week, immediately followed by an exam). The student then used ChatGPT to generate practice questions based on lecture PDFs for daily study, leading to significant grade improvement. However, the student felt guilty after reading criticisms about AI’s environmental impact and the importance of “independent thinking.” Community comments generally agreed that using AI for learning assistance is a legitimate and effective use, helping improve efficiency and learning outcomes, and should not cause guilt. Commenters noted that AI’s environmental impact needs context compared to other human activities, and leveraging AI for productivity is already a workplace trend. (Source: Reddit r/ArtificialInteligence)
Claude Pro User Experience: Throttling and Business Model Discussion: In the Reddit r/ClaudeAI community, users discussed encountering throttling issues when using the Claude Pro service and explored Anthropic’s business model. One user pointed out that the $20/month Pro subscription fee is far less than the actual compute cost Anthropic incurs for heavy users (potentially up to $100/month), suggesting user complaints (e.g., feeling “exploited”) might overlook the cost structure of AI services. The discussion also touched upon Anthropic recently prioritizing new features for the more expensive Max tier over the Pro tier, causing dissatisfaction among early annual Pro subscribers. (Source: Reddit r/ClaudeAI 1, Reddit r/ClaudeAI 2)
KCORES LLM Arena Update, DeepSeek R1 Shows Strong Performance: A user shared the latest test results from their personally maintained LLM arena (KCORES LLM Arena). The test required models to generate Python code for a complex physics simulation (20 balls colliding and bouncing inside a rotating heptagon). After updating the arena to include new models like GPT-4.1, Gemini 2.5 Pro, and DeepSeek-V3, the results showed DeepSeek R1 performed exceptionally well on this task, generating a good simulation. This provides the community with another reference point for evaluating different models’ capabilities on complex programming tasks. (Source: Reddit r/LocalLLaMA)
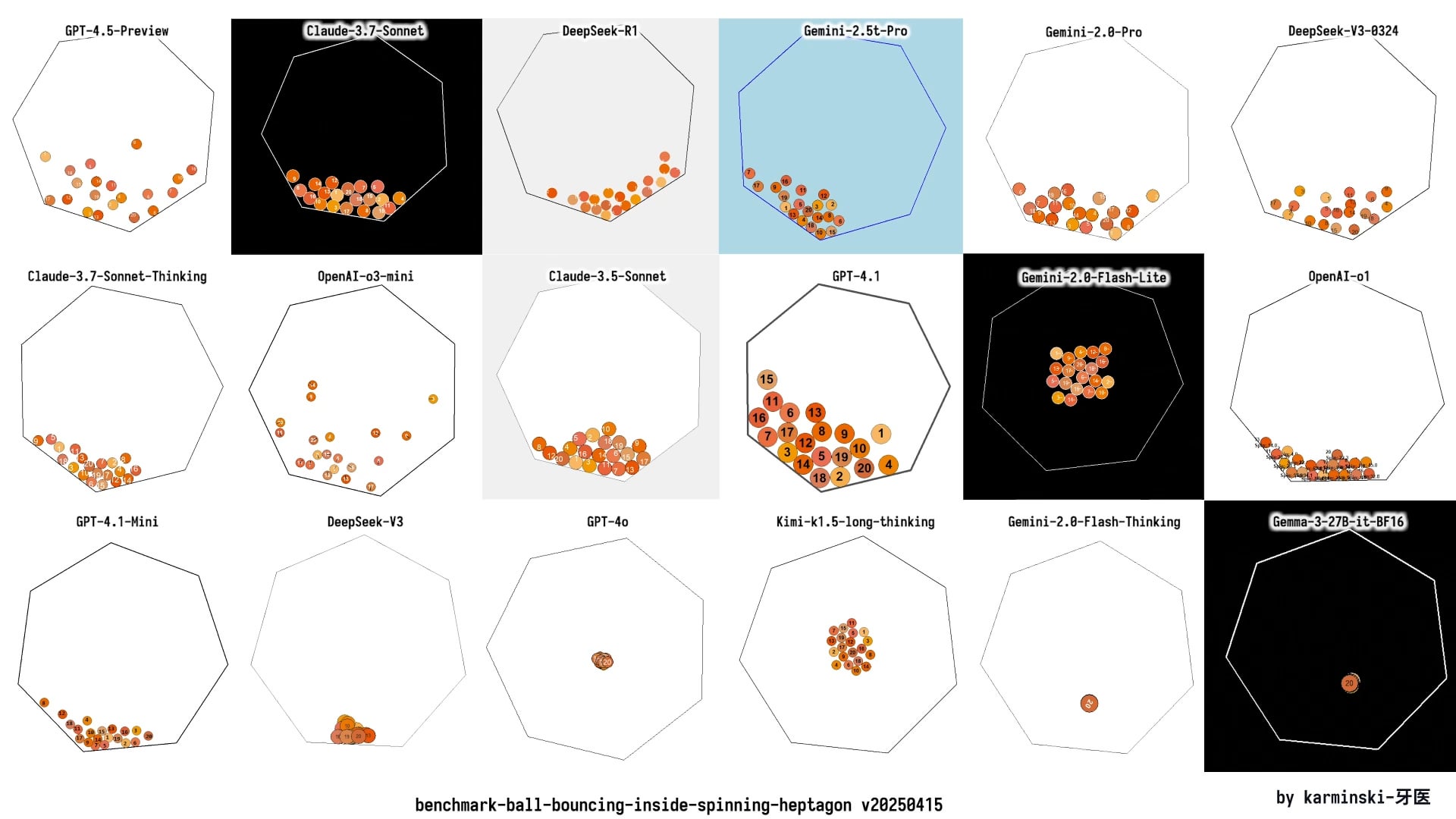
Exploring Emotional Response Capabilities of Different LLMs: A Reddit user posted a meme humorously comparing the different response styles of ChatGPT 4o, Claude 3 Sonnet, Llama 3 70B, and Mistral Large when faced with a user expressing sadness. This reflects users’ varying experiences when using different LLMs for emotional communication or seeking support, as well as the community’s perception and evaluation of models’ “empathy” capabilities. The comment section also discussed the privacy advantages of using local models for sensitive emotional topics. (Source: Reddit r/LocalLLaMA)
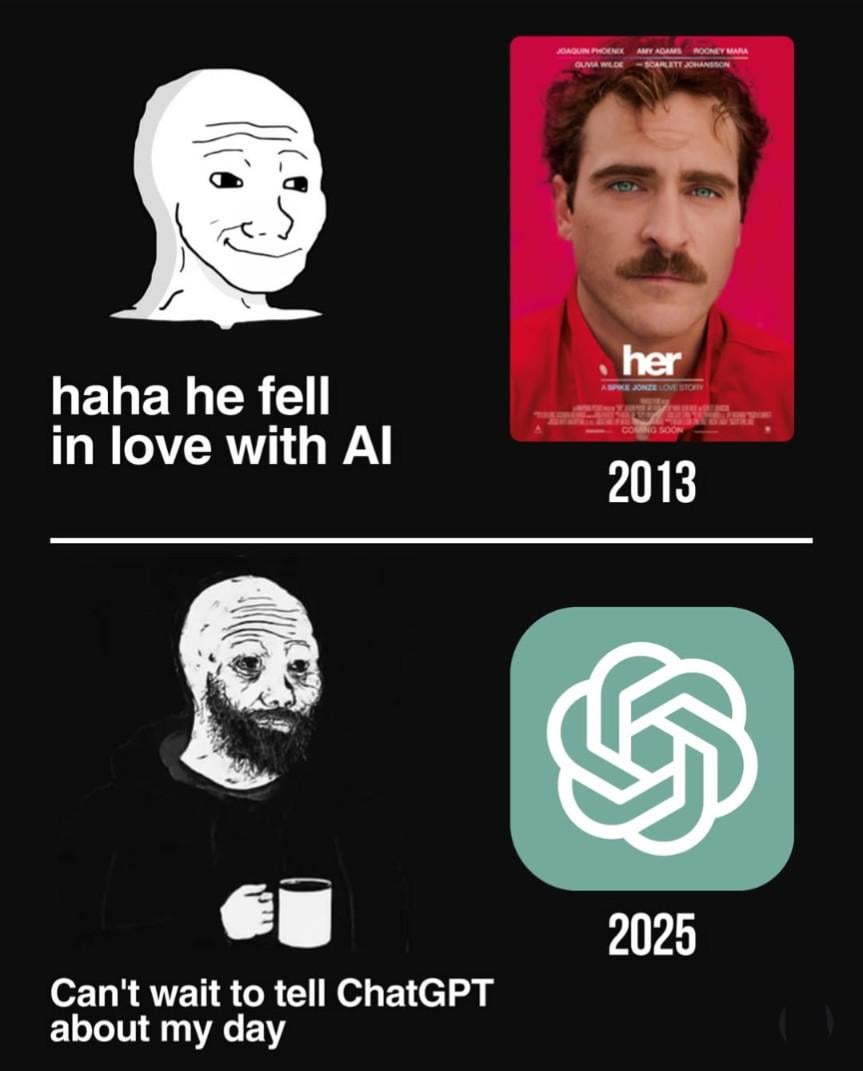
Discussion on Whether AGI is a Silicon Valley Hoax: A community member shared and potentially discussed an article questioning whether Artificial General Intelligence (AGI) is a concept overhyped by Silicon Valley (the tech industry) to attract investment or maintain buzz (a hoax). This reflects ongoing debate and skepticism within the industry and public regarding the feasibility, timeline, and veracity of current AGI-related claims. (Source: Ronald_vanLoon)
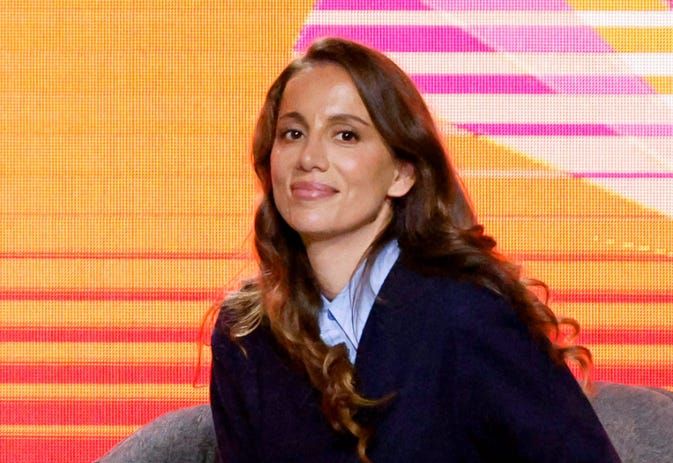
💡 Other
Small Appliance Industry Cools Down, AI Becomes New Narrative but Application Still Shallow: The kitchen small appliance market (e.g., breakfast makers, air fryers) faces declining sales and price wars after the “stay-at-home economy” boom faded. Performance is under pressure for the “Big Six” listed companies like Supor, Joyoung, and Bear Electric. Seeking breakthroughs, companies are generally looking towards overseas market expansion and AI technology integration. However, current AI applications in small appliances are mostly limited to simple voice commands and automatic adjustments, offering limited practicality and innovation space, and potentially deterring users with increased costs. In contrast, large appliances have more advantages in AI application, capable of building smart home ecosystems and providing personalized services using big data. The AI narrative for the small appliance industry is still in its early stages. (Source: 36Kr)
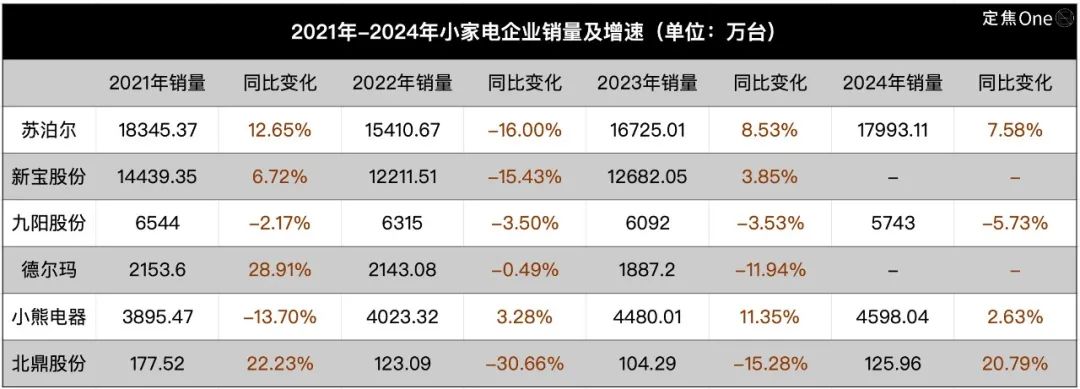
Tariff Turmoil Impacts Huaqiangbei Chip Market, Domestic Substitution May Accelerate: Recent changes in tariff policies surrounding chips have caused concern in Shenzhen’s Huaqiangbei electronics market. Merchants dealing in popular chips like CPUs and GPUs (especially those potentially originating from the US) have paused quoting prices and are hoarding inventory, leading to increased price volatility. The impact on categories like memory chips is relatively smaller. Several listed distributors stated that the direct impact of the tariff war is limited due to the small proportion of direct imports from the US, but market uncertainty has increased. The industry generally believes that IDM companies with fabs in the US (like TI, Intel, Micron) are most affected. This event has already prompted some downstream customers to inquire about domestic chip alternatives, potentially accelerating the localization process in the semiconductor sector. (Source: Chuangyebanquancha)
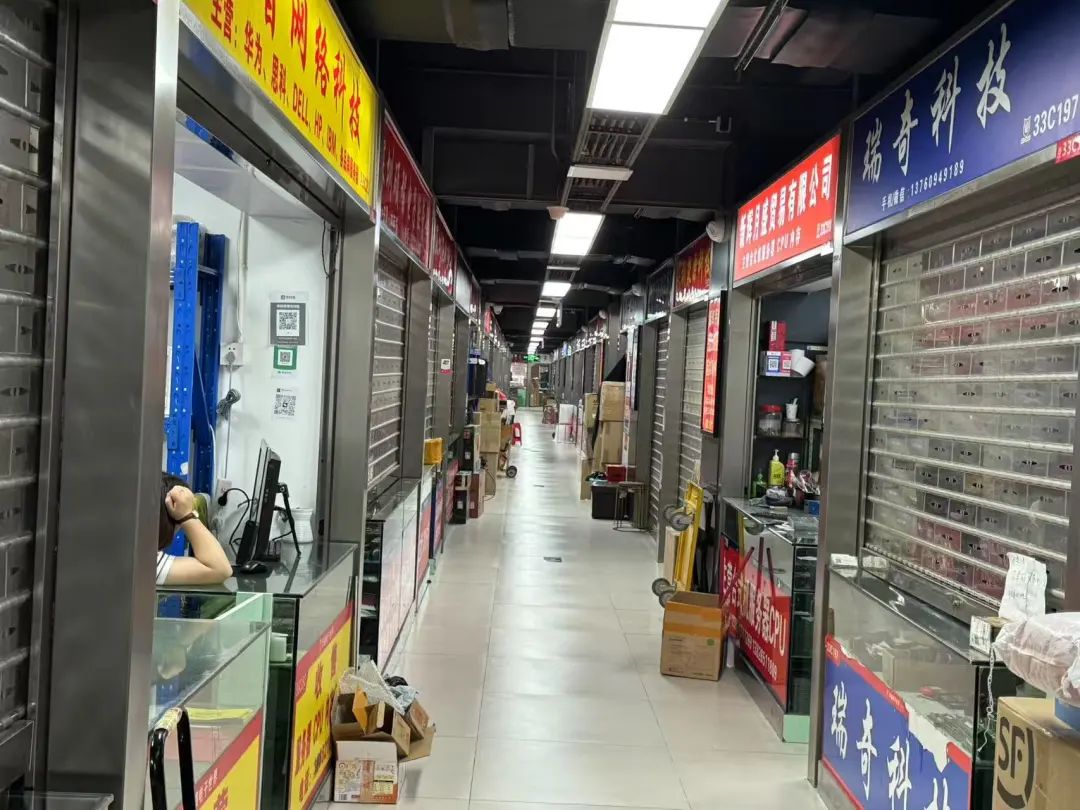
AI Exacerbates Human Crisis of Meaning? Reflecting on the Balance Between Technology and Values: The article explores how the rapid development of artificial intelligence impacts human existential meaning. It argues that AI surpassing humans in professional fields (like Go, medical diagnosis, art creation) exacerbates the crisis of meaning triggered since the Industrial Revolution by factors like labor alienation, crises of faith, and environmental problems. AI might further reinforce the “tool person” dilemma, especially by replacing decision-making capabilities in white-collar jobs. Citing philosophers and sci-fi works (like “Dune,” “Westworld”), the article warns against the risk of technological enslavement, calling for rebuilding value rationality while embracing AI’s technological enhancements. It advocates safeguarding human creativity, emotional connection, and critical thinking through ethical frameworks and humanities education to avoid becoming appendages to our own creations. (Source: Tencent Research Institute)
US-Made iPhone Costs Could Exceed 25,000 Yuan ($3,500): The article analyzes that if the iPhone were entirely produced domestically in the US, its cost would skyrocket, with an estimated selling price potentially reaching $3,500 (about 25,588 RMB), far exceeding current prices. Key reasons include significantly higher costs in the US compared to China for raw material acquisition (like rare earths, refined lithium/cobalt), logistics, factory construction (land, power, environmental approvals), and labor (minimum wage 4-5 times higher than China’s, plus a lack of skilled industrial workers). Apple’s previous model of maintaining high profit margins by squeezing the global supply chain (especially Chinese suppliers with relatively larger profit margins) would be unsustainable in the US. The high production costs would likely be passed on to consumers, potentially shaking Apple’s pricing strategy and market position. (Source: Xinghai Intelligence Bureau)
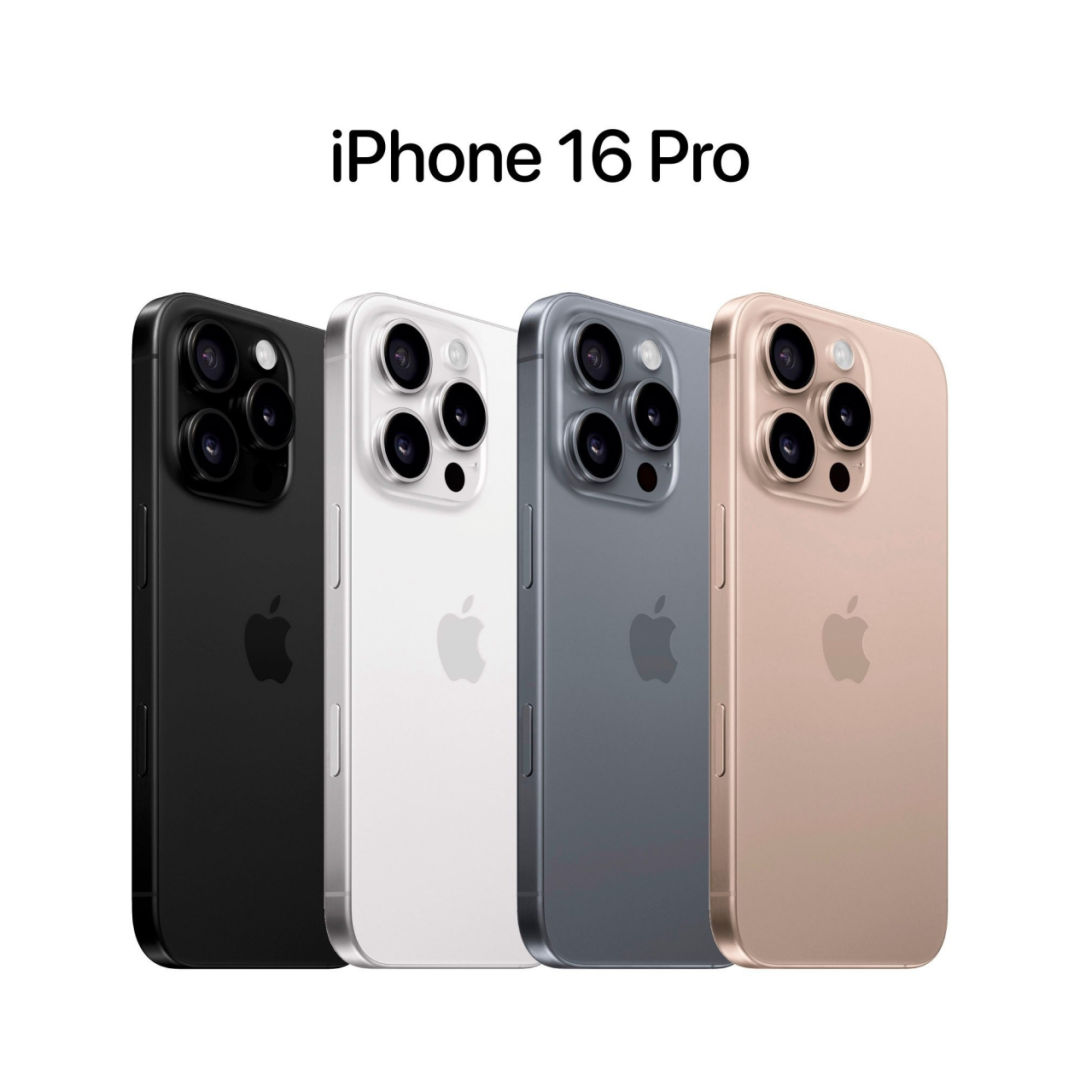
Mathematical Breakthrough: Multiplicity-One Conjecture for Mean Curvature Flow Singularities Proven: The Multiplicity-one conjecture, which puzzled mathematicians for nearly 30 years, was recently proven by Richard Bamler and Bruce Kleiner. The conjecture concerns Mean Curvature Flow (MCF) – a mathematical process describing how surfaces evolve over time to decrease their area most rapidly (like ice melting or sandcastles eroding). The proof indicates that in three-dimensional space, singularities (points where curvature approaches infinity) formed by closed two-dimensional surfaces under MCF are simple. They typically manifest locally as spheres shrinking to a point or cylinders collapsing to a line; complex, multi-layered overlapping singularities do not occur. This breakthrough ensures that MCF can continue to be analyzed after singularities form, providing a more solid theoretical foundation for using MCF to solve important problems in geometry and topology (like the Poincaré conjecture). (Source: Machine Heart)
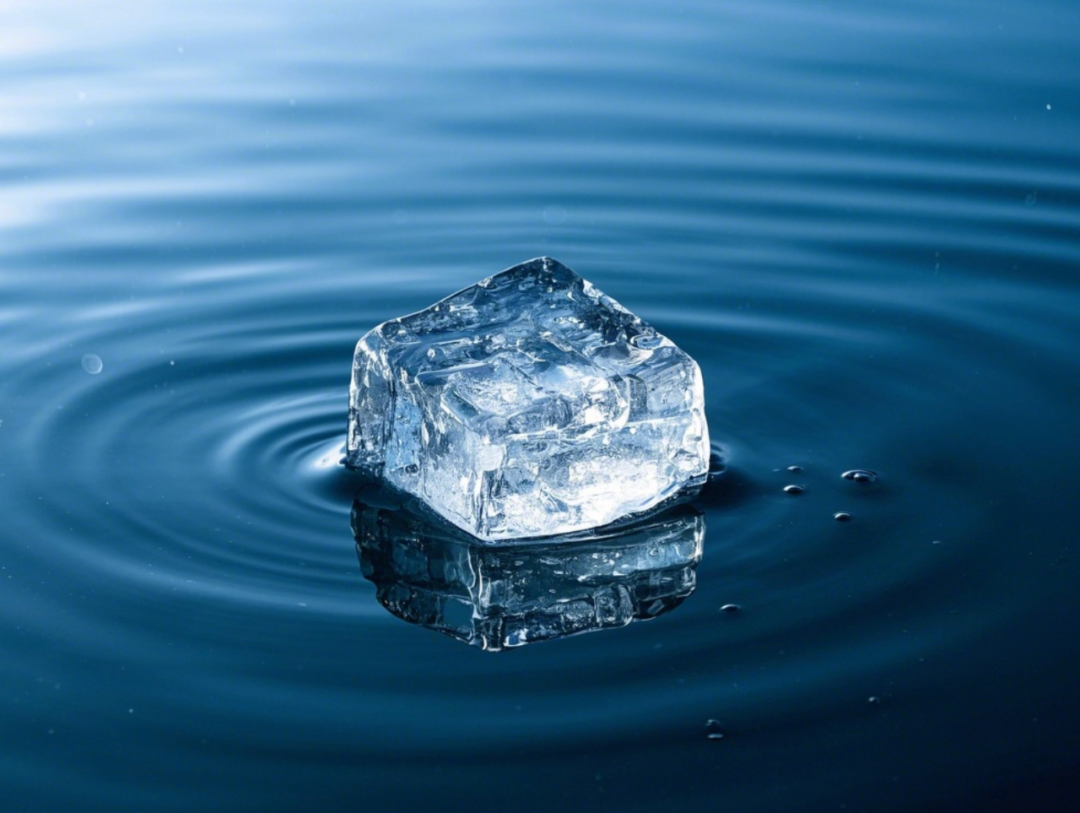
User Shares “Budget” 4x RTX 3090 Local AI Hardware Setup: A Reddit user shared their hardware configuration for running LLMs locally, with a total cost of approximately $4,204. The setup includes four used EVGA RTX 3090 graphics cards ($600 each), an AMD EPYC 7302P server CPU, an Asrock Rack motherboard, 96GB DDR4 RAM, and a 2TB NVMe SSD, assembled in an MLACOM Quad Station Pro Lite open-air case and powered by two 1200W power supplies. This share provides a relatively “economical” reference solution for users wanting to build a home AI workstation with significant compute power (4x 24GB VRAM). (Source: Reddit r/LocalLLaMA)
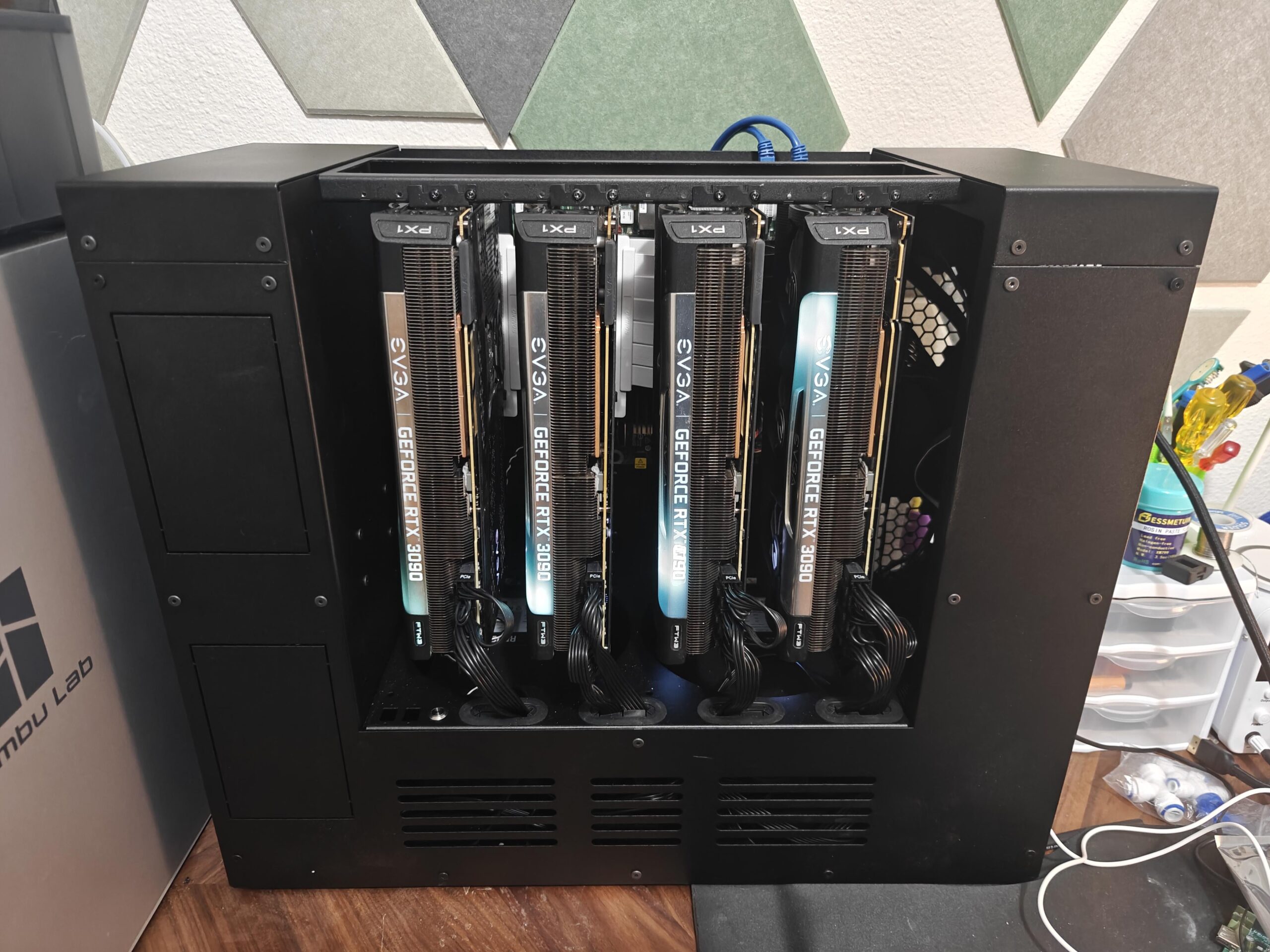
Hackers Attack US Traffic Signals to Display Musk and Zuckerberg Deepfake Messages: Reports indicate that multiple pedestrian crosswalk signal systems in the San Francisco Bay Area were hacked and used to display Deepfake messages generated by AI featuring Elon Musk and Mark Zuckerberg. This incident highlights the vulnerability of public infrastructure to cyberattacks leveraging AI technology and the risk of Deepfake technology being misused for spreading misinformation or conducting pranks. (Source: Reddit r/ArtificialInteligence)
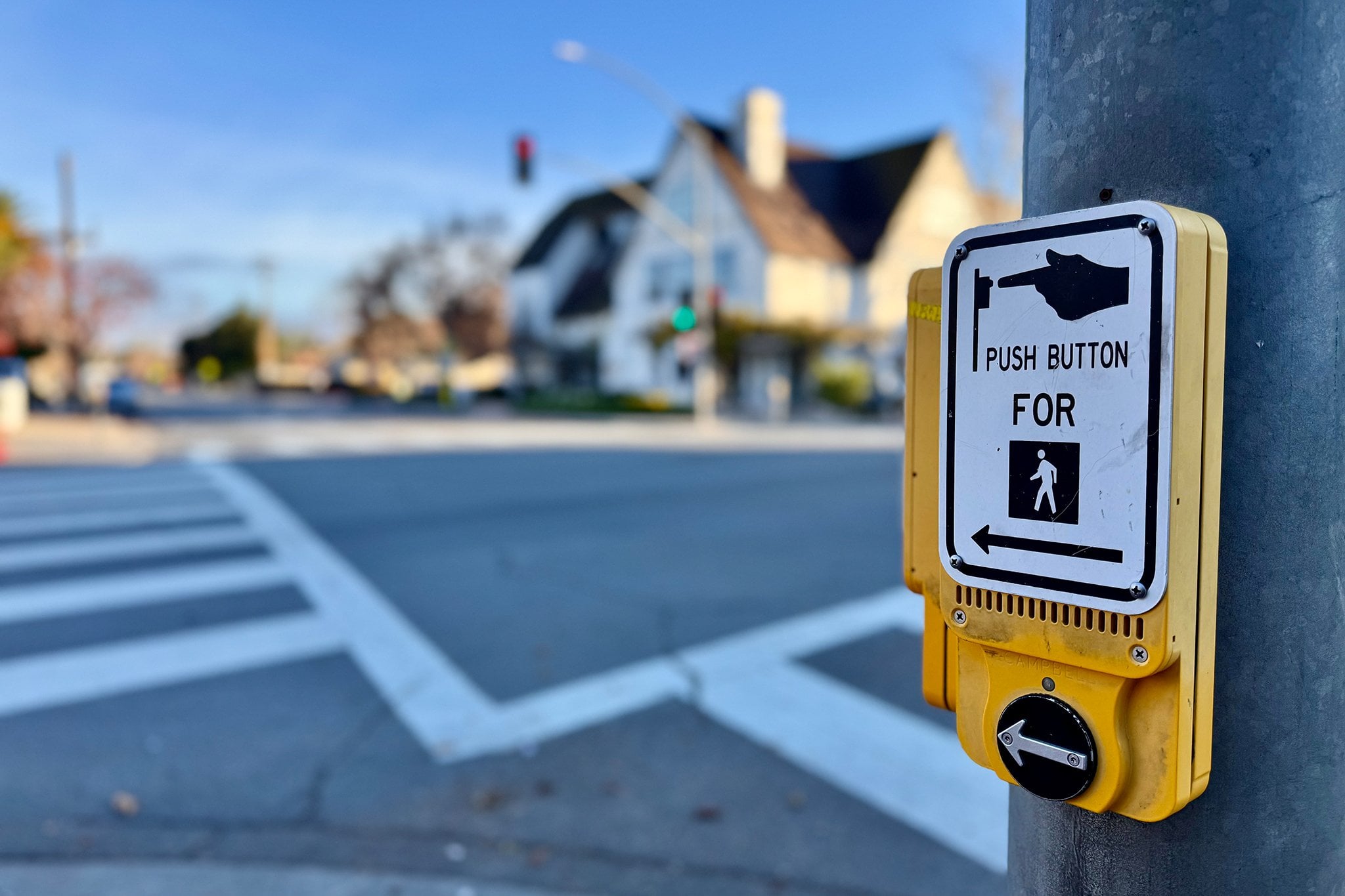
Showcasing Diverse Robotics and Automation Technologies: Social media featured various applications of robotics and automation technology, including: the Booster T1 robot capable of mimicking human movements to perform kung fu; robotic systems for rehabilitation training; a robotic arm making coffee; agricultural robots for rice planting and weeding; an automated system to help shepherds handle sheep; and dancing robots. These examples reflect the widespread application and continuous development of robotics in industry, agriculture, service sectors, medical rehabilitation, and entertainment. (Source: Ronald_vanLoon 1, Ronald_vanLoon 2, Ronald_vanLoon 3, Ronald_vanLoon 4, Ronald_vanLoon 5, Ronald_vanLoon 6)
Showcasing Emerging Technologies and Innovative Products: Social media shared various emerging technologies and innovative products, such as: MIT-developed tiny wireless antennas using light to monitor cellular communication; a single-winged drone mimicking maple seed flight; IoT smart toilets; digital impression technology for dental orthodontics; a device generating electricity from saltwater; dynamic walls that can breathe and move; an Iron Man Cosplay suit; an all-terrain electric snowboard; and techniques using a Flipper Zero device to copy keys. These showcases highlight ongoing technological innovation in diverse fields like communication, energy, health, transportation, construction, and security. (Source: Ronald_vanLoon 1, Ronald_vanLoon 2, Ronald_vanLoon 3, Ronald_vanLoon 4, Ronald_vanLoon 5, Ronald_vanLoon 6, Ronald_vanLoon 7, Ronald_vanLoon 8, Ronald_vanLoon 9)
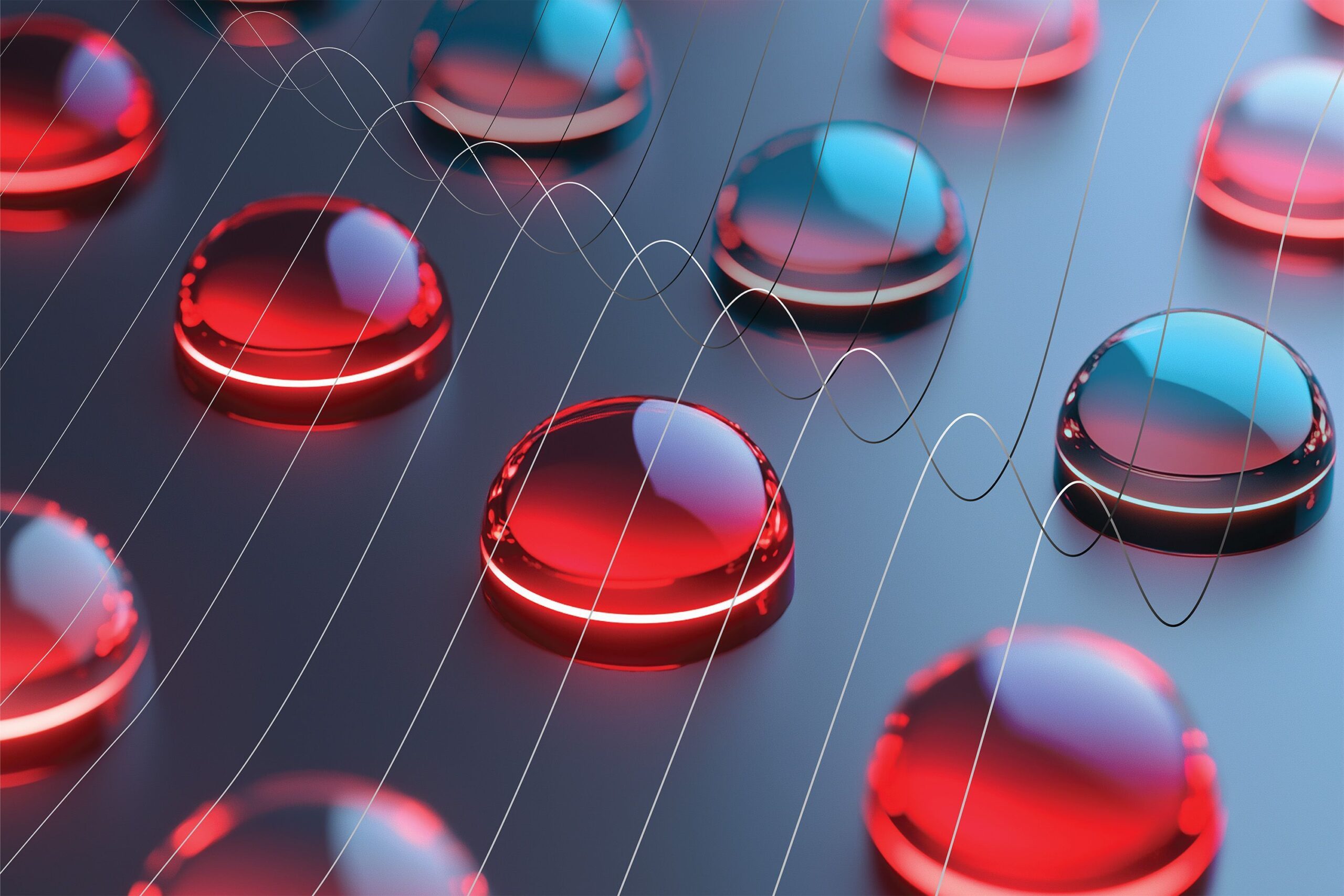
Healthcare Technology Trends: Social media posts and article links mentioned technology applications and development trends in the healthcare sector, including robot-assisted surgery, AI trends and inflection points in healthcare, driving operational excellence with technology (hyperautomation), and the potential transformations brought by AI. These contents reflect the potential and practice of AI, robotics, automation, and other technologies in improving healthcare service efficiency, diagnostic accuracy, and patient experience. (Source: Ronald_vanLoon 1, Ronald_vanLoon 2, Ronald_vanLoon 3, Ronald_vanLoon 4)
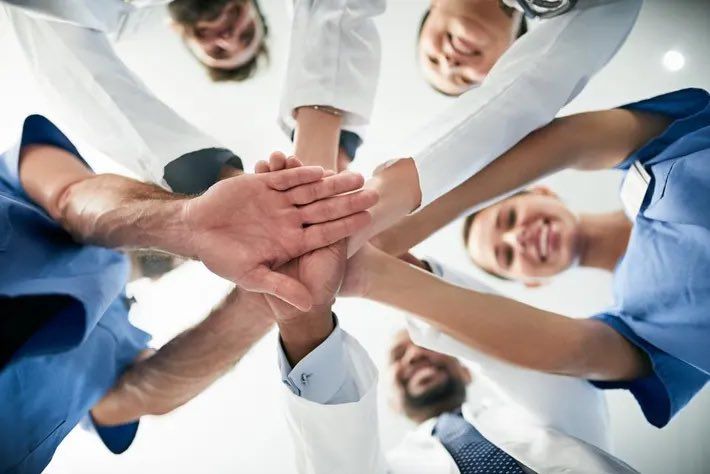
Cybersecurity Related Information: Social media shared cybersecurity-related content, including an infographic on types of password attacks and an article on the importance of recovery capability within 60 minutes after a data breach. This content reminds users to pay attention to cybersecurity risks and response strategies. (Source: Ronald_vanLoon 1, Ronald_vanLoon 2)
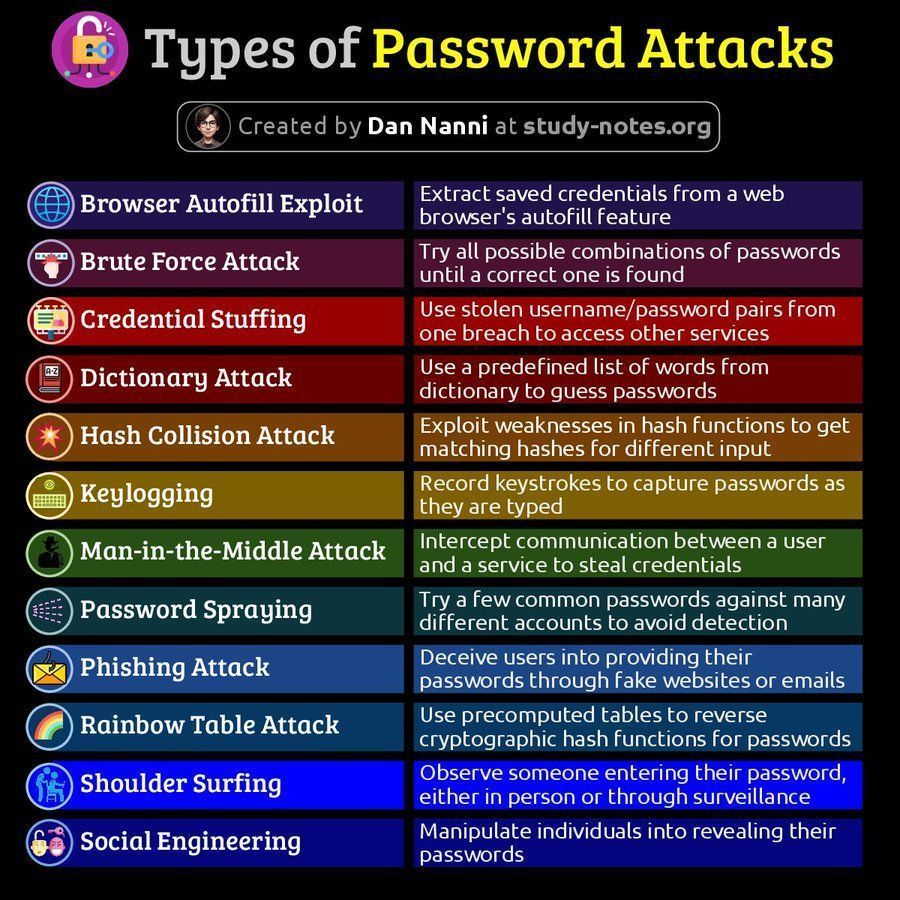
AMD ROCm Platform Discussion: Reddit users discussed the possibility of building a deep learning workstation using dual AMD Radeon RX 7900 XTX GPUs, involving the ROCm (Radeon Open Compute platform) software stack. This reflects user interest and exploration of AMD GPU solutions and their software ecosystem (ROCm) in the Nvidia-dominated AI hardware market. (Source: Reddit r/deeplearning)